User login
This issue of the Journal of Hospital Medicine describes 2 research and quality improvement demonstration projects funded by the Gordon and Betty Moore Foundation. Early detection is central to both projects. This introductory article does not provide a global review of the now voluminous literature on rapid response teams (RRTs), sepsis detection systems, or treatment protocols. Rather, it takes a step back and reassesses just what early detection and quantification of critical illness are. It then examines the implications of early detection and its quantification.
CONCEPTUAL FRAMEWORK
We define severe illness as the presence of acute disease such that a person can no longer expect to improve without dedicated hospital treatment but which is not inevitably associated with mortality, postdischarge morbidity, or major loss of autonomy. In contrast, we define critical illness as acute disease with high a priori risk of mortality, postdischarge morbidity, and major (possibly total) loss of autonomy. We accept that the boundaries between ordinary illness, severe illness, and critical illness are blurred. The basic assumption behind all efforts at early detection is that these edges can be made sharp, and that the knowledge base required to do so can also lead to improvements in treatment protocols and patient outcomes. Further, it is assumed that at least some forms of critical illness can be prevented or mitigated by earlier detection, identification, and treatment.
Research over the last 2 decades has provided important support for this intuitive view as well as making it more nuanced. With respect to epidemiology, the big news is that sepsis is the biggest culprit, and that it accounts for a substantial proportion of all hospital deaths, including many previously considered unexpected hospital deaths due to in‐hospital deterioration.[1] With respect to treatment, a number of studies have demonstrated that crucial therapies previously considered to be intensive care unit (ICU) therapies can be initiated in the emergency department or general medicalsurgical ward.[2]
Figure 1 shows an idealized framework for illness presenting in the emergency department or general medicalsurgical wards. It illustrates the notion that a transition period exists when patients may be rescued with less intense therapy than will be required when condition progression occurs. Once a certain threshold is crossed, the risk of death or major postdischarge morbidity rises exponentially. Unaided human cognition's ability to determine where a given patient is in this continuum is dangerously variable and is highly dependent on the individuals training and experience. Consequently, as described in several of the articles in this issue as well as multiple other publications, health systems are employing comprehensive electronic medical records (EMRs) and are migrating to algorithmic approaches that combine multiple types of patient data.[3, 4] Although we are still some distance from being able to define exact boundaries between illness, severe illness, and critical illness, current EMRs permit much better definition of patient states, care processes, and short‐term outcomes.
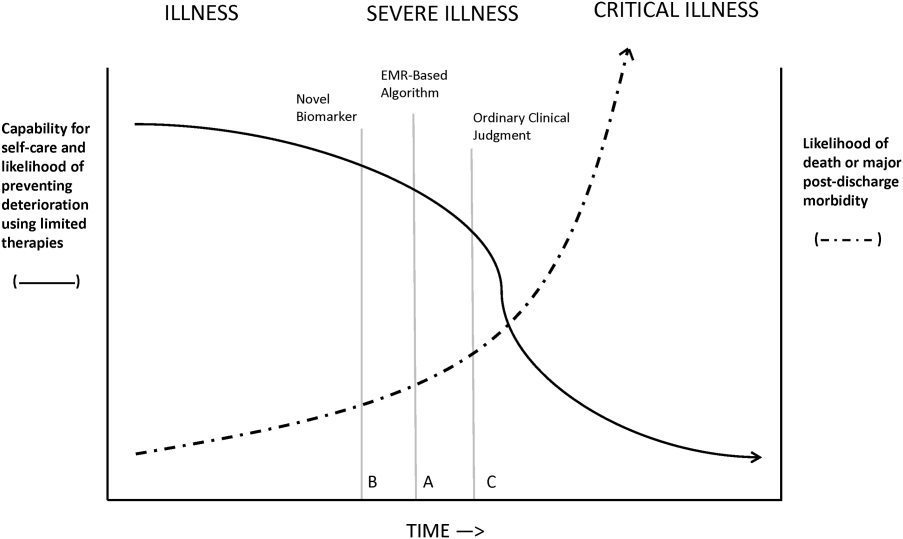
Whereas our ability to quantify many processes and short‐term outcomes is expanding rapidly, quantification of the possible benefit of early detection is complicated by the fact that, even in the best of circumstances, not all patients can be rescued. For some patients, rescue may be temporary, raising the prospect of repeated episodes of critical illness and prolonged intensive care without any hope of leaving the hospital. Figure 2 shows that, for these patients, the problem is no longer simply one of preventing death and preserving function but, rather, preserving autonomy and dignity. In this context, early detection means earlier specification of patient preferences.[5, 6]
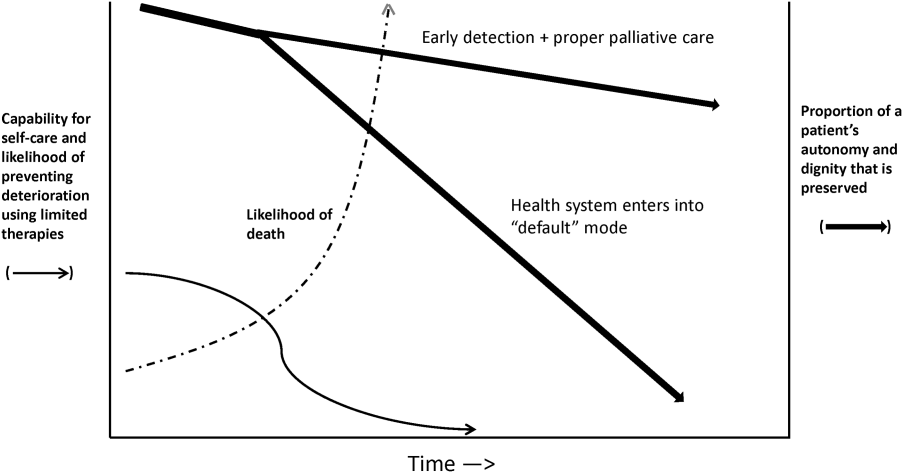
JUST WHAT CONSTITUTES EARLY DETECTION (AND HOW DO WE QUANTIFY IT)?
RRTs arose as the result of a number of studies showing thatin retrospectin‐hospital deteriorations should not have been unexpected. Given comprehensive inpatient EMRs, it is now possible to develop more rigorous definitions. A minimum set of parameters that one would need to specify for proper quantification of early detection is shown on Figure 3. The first is specifying a T0, that is, the moment when a prediction regarding event X (which needs to be defined) is issued. This is different from the (currently unmeasurable) biologic onset of illness as well as the first documented indication that critical illness was present. Further, it is important to be explicit about the event time frame (the time period during which a predicted event is expected to occur): we are predicting that X will occur within E hours of the T0. The time frame between the T0 and X, which we are referring to as lead time, is clinically very important, as it represents the time period during which the response arm (eg, RRT intervention) is to be instituted. Statistical approaches can be used to estimate it, but once an early detection system is in place, it can be quantified. Figure 3 is not restricted to electronic systems; all components shown can be and are used by unaided human cognition.
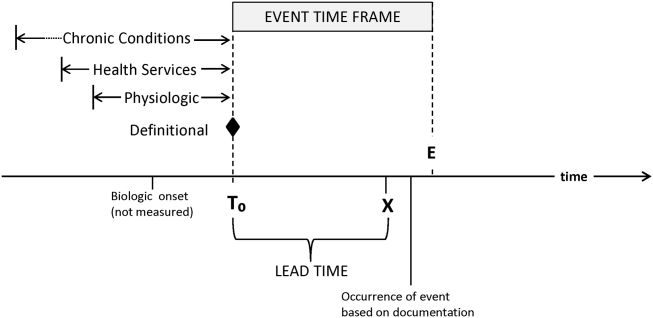
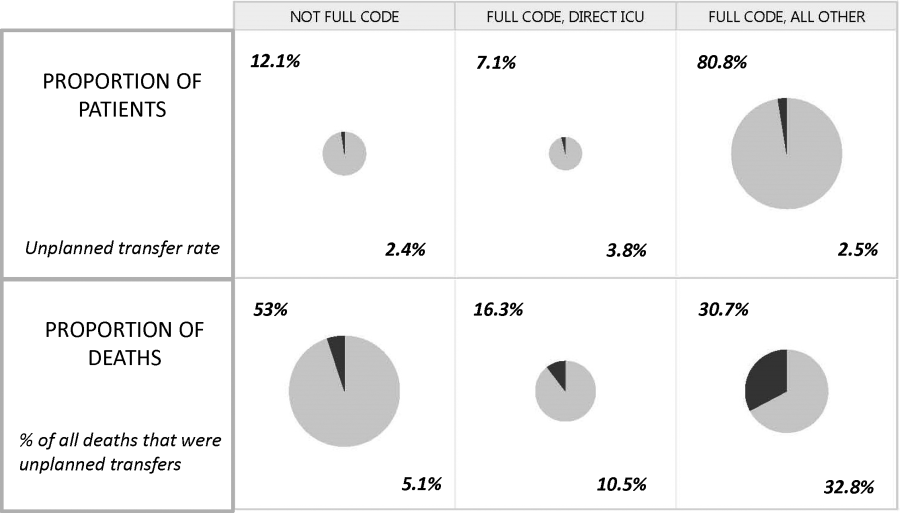
It is essential to specify what data are used to generate probability estimates as well as the time frames used, which we refer to as the look‐back time frames. Several types of data could be employed, with some data elements (eg, age or gender) being discrete data with a 1:1 fixed correspondence between the patient and the data. Other data have a many‐to‐1 relationship, and an exact look‐back time frame must be specified for each data type. For example, it seems reasonable to specify a short (1224 hours) look‐back period for some types of data (eg, vital signs, lactate, admission diagnosis or chief complaint), an intermediate time period (13 days) for information on the current encounter, and a longer (months to years) time period for preexisting illness or comorbidity burden.
Because many events are rare, traditional measures used to assess model performance, such as the area under the receiver operator characteristic curve (C statistic), are not as helpful.[7] Consequently, much more emphasis needs to be given to 2 key metrics: number needed to evaluate (or workup to detection ratio) and threshold‐specific sensitivity (ability of the alert to detect X at a given threshold). With these, one can answer 3 questions that will be asked by the physicians and nurses who are not likely to be researchers, and who will have little interest in the statistics: How many patients do I need to work up each day? How many patients will I need to work up for each possible outcome identified? For this amount of work, how many of the possible outcomes will we catch?
Data availability for the study of severe and critical illness continues to expand. Practically, this means that future research will require more nuanced ontologies for the classification of physiologic derangement. Current approaches to severity scoring (collapsing data into composite scores) need to be replaced by dynamic approaches that consider differential effects on organ systems as well as what can be measured. Severity scoring will also need to incorporate the rate of change of a score (or probability derived from a score) in predicting the occurrence of an event of interest as well as judging response to treatment. Thus, instead of at time of ICU admission, the patient had a severity score of 76, we may have although this patient's severity score at the time of admission was decreasing by 4 points per hour per 10 mL/kg fluid given, the probability for respiratory instability was increasing by 2.3% per hour given 3 L/min supplemental oxygen. This approach is concordant with work done in other clinical settings (eg, in addition to an absolute value of maximal negative inspiratory pressure or vital capacity, the rate of deterioration of neuromuscular weakness in Guillain‐Barr syndrome is also important in predicting respiratory failure[8]).
Electronic data also could permit better definition of patient preferences regarding escalation of care. At present, available electronic data are limited (primarily, orders such as do not resuscitate).[9] However, this EMR domain is gradually expanding.[10, 11] Entities such as the National Institutes of Health could develop sophisticated and rapid questionnaires around patient preferences that are similar to those developed for the Patient Reported Outcomes Measurement Information System.[12] Such tools could have a significant effect on our ability to quantify the benefits of early detection as it relates to a patient's preferences (including better delineation of what treatments they would and would not want).
ACTIVATING A RESPONSE ARM
Early identification, antibiotic administration, fluid resuscitation, and source control are now widely felt to constitute low‐hanging fruit for decreasing morbidity and mortality in severe sepsis. All these measures are included in quality improvement programs and sepsis bundles.[13, 14, 15] However, before early interventions can be instituted, sepsis must at least be suspected, hence the need for early detection. The situation with respect to patient deterioration (for reasons other than sepsis) in general medical surgical wards is less clear‐cut. Reasons for deterioration are much more heterogenous and, consequently, early detection is likely necessary but not sufficient for outcomes improvement.
The 2 projects described in this issue describe nonspecific (indicating elevated risk but not specifying what led to the elevation of risk) and sepsis‐specific alerting systems. In the case of the nonspecific system, detection may not lead to an immediate deployment of a response arm. Instead, a secondary evaluation process must be triggered first. Following this evaluation component, a response arm may or may not be required. In contrast, the sepsis‐specific project essentially transforms the general medicalsurgical ward into a screening system. This screening system then also triggers specific bundle components.
Neither of these systems relies on unaided human cognition. In the case of the nonspecific system, a complex equation generates a probability that is displayed in the EMR, with protocols specifying what actions are to be taken when that probability exceeds a prespecified threshold. With respect to the sepsis screening system, clinicians are supported by EMR alerts as well as protocols that increase nursing autonomy when sepsis is suspected.
The distinction between nonspecific (eg, acute respiratory failure or hemodynamic deterioration) and specific (eg, severe sepsis) alerting systems is likely to disappear as advances in the field occur. For example, incorporation of natural language processing would permit inclusion of semantic data, which could be processed so as to prebucket an alert into one that not just gave a probability, but also a likely cause for the elevated probability.
In addition, both types of systems suffer from the limitation of working off a limited database because, in general, current textbooks and training programs primary focus remains that of treatment of full‐blown clinical syndromes. For example, little is known about how one should manage patients with intermediate lactate values, despite evidence showing that a significant percentage of patients who die from sepsis will initially have such values, with 1 study showing 63% as many deaths with initial lactate of 2.5 to 4.0 mmol/L as occurred with an initial lactate of >4.0 mmol/L.[16] Lastly, as is discussed below, both systems will encounter similar problems when it comes to quantifying benefit.
QUANTIFYING BENEFIT
Whereas the notion of deploying RRTs has clearly been successful, success in demonstrating unequivocal benefit remains elusive.[17, 18, 19] Outcome measures vary dramatically across studies and have included the number of RRT calls, decreases in code blue events on the ward, and decreases in inpatient mortality.[20] We suspect that other reasons are behind this problem. First is the lack of adequate risk adjustment and ignoring the impact of patients near the end of life on the denominator. Figure 4 shows recent data from 21 Kaiser Permanente Northern California (KPNC) hospitals, which can now capture care directive orders electronically,[21] illustrates this problem. The majority (53%) of hospital deaths occur among a highly variable proportion (range across hospitals, 6.5%18.0%) of patients who arrive at the hospital with a restricted resuscitation preference (do not resuscitate, partial code, and comfort care only). These patients do not want to die or crash and burn but, were they to trigger an alert, they would not necessarily want to be rescued by being transferred to the ICU either; moreover, internal KPNC analyses show that large numbers of these patients have sepsis and refuse aggressive treatment. The second major confounder is that ICUs save lives. Consequently, although early detection could lead to fewer transfers to the ICU, using the end point of ICU admission is very problematic, because in many cases the goal of alerting systems should be to get patients to the ICU sooner, which would not affect the outcome of transfer to the ICU in a downward direction; in fact, such systems might increase transfer to the ICU.
The complexities summarized in Figure 4 mean that it is likely that formal quantification of benefit will require examination of multiple measures, including balancing measures as described below. It is also evident that, in this respectlack of agreement as to what constitutes a good outcomethe issues being faced here are a reflection of a broader area of disagreement within our profession and society at large that extends to medical conditions other than critical illness.
POTENTIAL HARMS OF EARLY DETECTION
Implementation of early detection and rapid response systems are not inherently free of harm. If these systems are not shown to have benefit, then the cost of operating them is moving resources away from other, possibly evidence‐based, interventions.[22] At the individual level, alerts could frighten patients and their families (for example, some people are very uncomfortable with the idea that one can predict events). Physicians and nurses who work in the hospital are already quite busy, so every time an alert is issued, it adds to the demand on their already limited time, hence, the critical importance of strategies to minimize false alarms and alert fatigue. Moreover, altering existing workflows can be disruptive and unpopular.
A potentially more quantifiable problem is the impact of early detection systems on ICU operations. For example, if an RRT decides to transfer a patient from the ward to the ICU as a preventive measure (soft landing) and this in turn ties up an ICU bed, that bed is then unavailable for a new patient in the emergency department. Similarly, early detection systems coupled with structured protocols for promoting soft landings could result in a change in ICU case mix, with greater patient flow due to increased numbers of patients with lower severity and lower ICU length of stay. These considerations suggest the need to couple early detection with other supportive data systems and workflows (eg, systems that monitor bed capacity proactively).
Lastly, if documentation protocols are not established and followed, early detection systems could expose both individual clinicians as well as healthcare institutions to medicallegal risk. This consideration could be particularly important in those instances where an alert is issued and, for whatever reasons, clinicians do not take action and do not document that decision. At present, early detection systems are relatively uncommon, but they may gradually become standard of care. This means that in‐house out of ICU deteriorations, which are generally considered to be bad luck or due to a specific error or oversight, may then be considered to be preventable. Another possible scenario that could arise is that of plaintiffs invoking enterprise liability, where a hospital's not having an early detection system becomes considered negligent.
ARTICLES IN THIS ISSUE
In this issue of the Journal of Hospital Medicine, we examine early detection from various perspectives but around a common theme that usually gets less attention in the academic literature: implementation. The article by Schorr et al.[23] describes a disease‐specific approach that can be instantiated using either electronic or paper tools. Escobar et al.[24] describe the quantitative as well as the electronic architecture of an early warning system (EWS) pilot at 2 hospitals that are part of an integrated healthcare delivery system. Dummett et al.[25] then show how a clinical rescue component was developed to take advantage of the EWS, whereas Granich et al.[26] describe the complementary component (integration of supportive care and ensuring that patient preferences are respected). The paper by Liu et al.[27] concludes by placing all of this work in a much broader context, that of the learning healthcare system.
FUTURE DIRECTIONS: KEY GAPS IN THE FIELD
Important gaps remain with respect to early detection and response systems. Future research will need to focus on a number of areas. First and foremost, better approaches to quantifying the costbenefit relationships of these systems are needed; somehow, we need to move beyond a purely intuitive sense that they are good things. Related to this is the need to establish metrics that would permit rigorous comparisons between different approaches; this work needs to go beyond simple comparisons of the statistical characteristics of different predictive models. Ideally, it should include comparisons of different approaches for the response arms as well. We also need to characterize clinician understanding about detection systems, what constitutes impending or incipient critical illness, and the optimum way to provide early detection. Finally, better approaches to integrating health services research with basic science work must be developed; for example, how should one test new biomarkers in settings with early detection and response systems?
The most important frontier, however, is how one can make early detection and response systems more patient centered and how one can enhance their ability to respect patient preferences. Developing systems to improve clinical management is laudable, but somehow we need to also find ways to have these systems make a better connection to what patients want most and what matters most to them, something that may need to include new ways that sometimes suspend use of these systems. At the end of the day, after early detection, patients must have a care experience that they see as an unequivocal improvement.
Acknowledgements
The authors thank our 2 foundation program officers, Dr. Marybeth Sharpe and Ms. Kate Weiland, for their administrative support and encouragement. The authors also thank Dr. Tracy Lieu, Dr. Michelle Caughey, Dr. Philip Madvig, and Ms. Barbara Crawford for their administrative assistance, Dr. Vincent Liu for comments on the manuscript, and Ms. Rachel Lesser for her help with formatting the manuscript and figures.
Disclosures
This work was supported by the Gordon and Betty Moore Foundation, The Permanente Medical Group, Inc., and Kaiser Foundation Hospitals, Inc. As part of our agreement with the Moore Foundation, we made a commitment to disseminate our findings in articles such as this one. However, the Gordon and Betty Moore Foundation and its staff played no role in how we actually structured our articles, nor did they review or preapprove any of the manuscripts submitted as part of the dissemination component. None of the authors has any conflicts of interest to declare of relevance to this work.
- Inpatient care for septicemia or sepsis: a challenge for patients and hospitals. NCHS Data Brief. 2011(62):1–8. , , ,
- Surviving sepsis campaign: association between performance metrics and outcomes in a 7.5‐year study. Crit Care Med. 2015;43(1):3–12. , , , et al.
- Early detection of impending physiologic deterioration among patients who are not in intensive care: development of predictive models using data from an automated electronic medical record. J Hosp Med. 2012;7(5):388–395. , , , , ,
- A randomized trial of real‐time automated clinical deterioration alerts sent to a rapid response team. J Hosp Med. 2014;9(7):424–429. , , , et al.
- Enhanced end‐of‐life care associated with deploying a rapid response team: a pilot study. J Hosp Med. 2009;4(7):449–452. , , , , ,
- The medical emergency team call: a sentinel event that triggers goals of care discussion. Crit Care Med. 2014;42(2):322–327. , , , ,
- Why the C‐statistic is not informative to evaluate early warning scores and what metrics to use. Crit Care. 2015;19:285. , , ,
- Anticipating mechanical ventilation in Guillain‐Barre syndrome. Arch Neurol. 2001;58(6):893–898. , , , ,
- The natural history of changes in preferences for life‐sustaining treatments and implications for inpatient mortality in younger and older hospitalized adults. J Am Geriatr Soc. 2016;64(5):981–989. , , , , ,
- Remote collection of questionnaires. Clin Exp Rheumatol. 2014;32(5 suppl 85):S168–S172. ,
- Be prepared to make your health care wishes known. Health care directives. Allina Health website. Available at: http://www.allinahealth.org/Customer-Service/Be-prepared/Be-prepared-to-make-your-health-care-wishes-known. Accessed January 1, 2015.
- Patient Reported Outcomes Measurement Information System. Dynamic tools to measure health outcomes from the patient perspective. Available at: http://www.nihpromis.org. Accessed January 15, 2015.
- Methodology of the surviving sepsis campaign global initiative for improving care of the patient with severe sepsis. Minerva Anestesiol. 2009;75(suppl 1):23–27. , , , , ,
- The Surviving Sepsis Campaign: a history and a perspective. Surg Infect (Larchmt). 2010;11(3):275–281. , ,
- The Surviving Sepsis Campaign: past, present and future. Trends Mol Med. 2014;20(4):192–194. ,
- Serum lactate as a predictor of mortality in emergency department patients with infection. Ann Emerg Med. 2005;45(5):524–528. , , , et al.
- Effect of a rapid response team on hospital‐wide mortality and code rates outside the ICU in a Children's Hospital. JAMA. 2007;298(19):2267–2274. , , , et al.
- Effects of a medical emergency team on reduction of incidence of and mortality from unexpected cardiac arrests in hospital: preliminary study. BMJ. 2002;324(7334):387–390. , , , , ,
- Rapid response teams: qualitative analysis of their effectiveness. Am J Crit Care. 2013;22(3):198–210. ,
- Hospital‐wide code rates and mortality before and after implementation of a rapid response team. JAMA. 2008;300(21):2506–2513. , , , , ,
- Risk‐adjusting hospital mortality using a comprehensive electronic record in an integrated healthcare delivery system. Med Care. 2013;51(5):446–453. , , , ,
- Rapid response teams—walk, don't run. JAMA. 2006;296(13):1645–1647. , ,
- Schorr et al. J Hosp Med. 2016;11:000–000.
- Piloting electronic medical record–based early detection of inpatient deterioration in community hospitals. J Hosp Med. 2016;11:000–000. , , , et al.
- Dummett et al. J Hosp Med. 2016;11:000–000.
- Granich et al. J Hosp Med. 2016;11:000–000.
- Liu et al. , , , , , Data that drive: closing the loop in the learning hospital system. J Hosp Med. 2016;11:000–000.
This issue of the Journal of Hospital Medicine describes 2 research and quality improvement demonstration projects funded by the Gordon and Betty Moore Foundation. Early detection is central to both projects. This introductory article does not provide a global review of the now voluminous literature on rapid response teams (RRTs), sepsis detection systems, or treatment protocols. Rather, it takes a step back and reassesses just what early detection and quantification of critical illness are. It then examines the implications of early detection and its quantification.
CONCEPTUAL FRAMEWORK
We define severe illness as the presence of acute disease such that a person can no longer expect to improve without dedicated hospital treatment but which is not inevitably associated with mortality, postdischarge morbidity, or major loss of autonomy. In contrast, we define critical illness as acute disease with high a priori risk of mortality, postdischarge morbidity, and major (possibly total) loss of autonomy. We accept that the boundaries between ordinary illness, severe illness, and critical illness are blurred. The basic assumption behind all efforts at early detection is that these edges can be made sharp, and that the knowledge base required to do so can also lead to improvements in treatment protocols and patient outcomes. Further, it is assumed that at least some forms of critical illness can be prevented or mitigated by earlier detection, identification, and treatment.
Research over the last 2 decades has provided important support for this intuitive view as well as making it more nuanced. With respect to epidemiology, the big news is that sepsis is the biggest culprit, and that it accounts for a substantial proportion of all hospital deaths, including many previously considered unexpected hospital deaths due to in‐hospital deterioration.[1] With respect to treatment, a number of studies have demonstrated that crucial therapies previously considered to be intensive care unit (ICU) therapies can be initiated in the emergency department or general medicalsurgical ward.[2]
Figure 1 shows an idealized framework for illness presenting in the emergency department or general medicalsurgical wards. It illustrates the notion that a transition period exists when patients may be rescued with less intense therapy than will be required when condition progression occurs. Once a certain threshold is crossed, the risk of death or major postdischarge morbidity rises exponentially. Unaided human cognition's ability to determine where a given patient is in this continuum is dangerously variable and is highly dependent on the individuals training and experience. Consequently, as described in several of the articles in this issue as well as multiple other publications, health systems are employing comprehensive electronic medical records (EMRs) and are migrating to algorithmic approaches that combine multiple types of patient data.[3, 4] Although we are still some distance from being able to define exact boundaries between illness, severe illness, and critical illness, current EMRs permit much better definition of patient states, care processes, and short‐term outcomes.
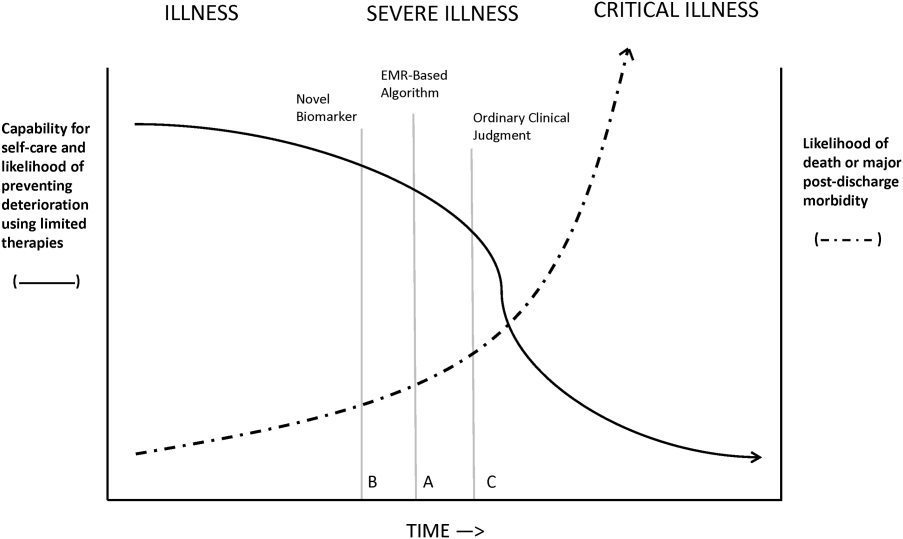
Whereas our ability to quantify many processes and short‐term outcomes is expanding rapidly, quantification of the possible benefit of early detection is complicated by the fact that, even in the best of circumstances, not all patients can be rescued. For some patients, rescue may be temporary, raising the prospect of repeated episodes of critical illness and prolonged intensive care without any hope of leaving the hospital. Figure 2 shows that, for these patients, the problem is no longer simply one of preventing death and preserving function but, rather, preserving autonomy and dignity. In this context, early detection means earlier specification of patient preferences.[5, 6]
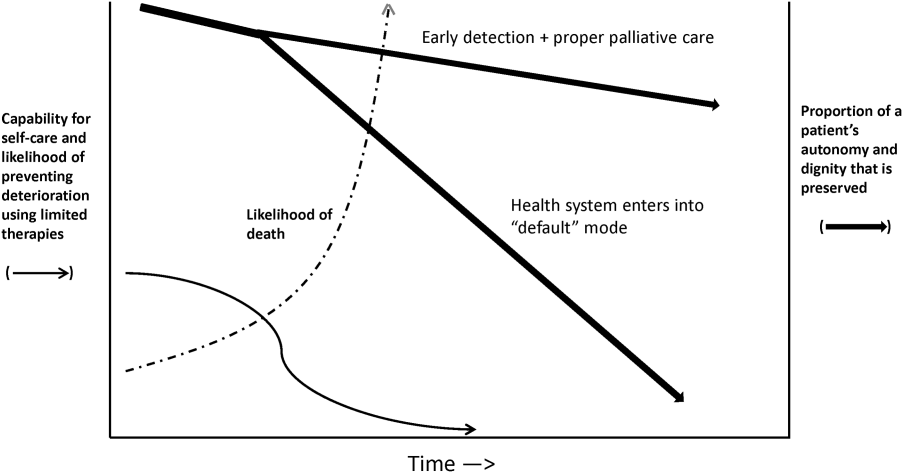
JUST WHAT CONSTITUTES EARLY DETECTION (AND HOW DO WE QUANTIFY IT)?
RRTs arose as the result of a number of studies showing thatin retrospectin‐hospital deteriorations should not have been unexpected. Given comprehensive inpatient EMRs, it is now possible to develop more rigorous definitions. A minimum set of parameters that one would need to specify for proper quantification of early detection is shown on Figure 3. The first is specifying a T0, that is, the moment when a prediction regarding event X (which needs to be defined) is issued. This is different from the (currently unmeasurable) biologic onset of illness as well as the first documented indication that critical illness was present. Further, it is important to be explicit about the event time frame (the time period during which a predicted event is expected to occur): we are predicting that X will occur within E hours of the T0. The time frame between the T0 and X, which we are referring to as lead time, is clinically very important, as it represents the time period during which the response arm (eg, RRT intervention) is to be instituted. Statistical approaches can be used to estimate it, but once an early detection system is in place, it can be quantified. Figure 3 is not restricted to electronic systems; all components shown can be and are used by unaided human cognition.
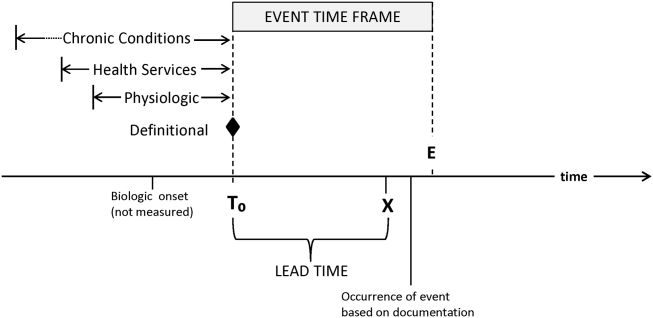
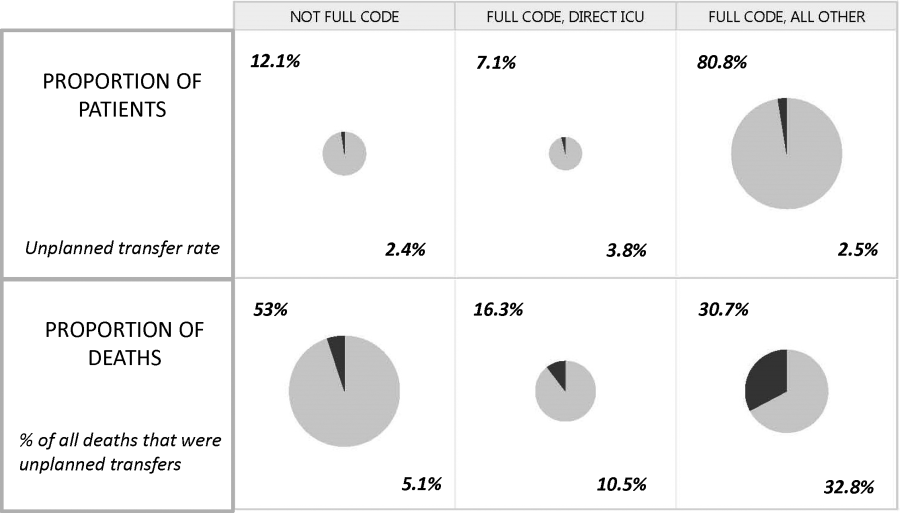
It is essential to specify what data are used to generate probability estimates as well as the time frames used, which we refer to as the look‐back time frames. Several types of data could be employed, with some data elements (eg, age or gender) being discrete data with a 1:1 fixed correspondence between the patient and the data. Other data have a many‐to‐1 relationship, and an exact look‐back time frame must be specified for each data type. For example, it seems reasonable to specify a short (1224 hours) look‐back period for some types of data (eg, vital signs, lactate, admission diagnosis or chief complaint), an intermediate time period (13 days) for information on the current encounter, and a longer (months to years) time period for preexisting illness or comorbidity burden.
Because many events are rare, traditional measures used to assess model performance, such as the area under the receiver operator characteristic curve (C statistic), are not as helpful.[7] Consequently, much more emphasis needs to be given to 2 key metrics: number needed to evaluate (or workup to detection ratio) and threshold‐specific sensitivity (ability of the alert to detect X at a given threshold). With these, one can answer 3 questions that will be asked by the physicians and nurses who are not likely to be researchers, and who will have little interest in the statistics: How many patients do I need to work up each day? How many patients will I need to work up for each possible outcome identified? For this amount of work, how many of the possible outcomes will we catch?
Data availability for the study of severe and critical illness continues to expand. Practically, this means that future research will require more nuanced ontologies for the classification of physiologic derangement. Current approaches to severity scoring (collapsing data into composite scores) need to be replaced by dynamic approaches that consider differential effects on organ systems as well as what can be measured. Severity scoring will also need to incorporate the rate of change of a score (or probability derived from a score) in predicting the occurrence of an event of interest as well as judging response to treatment. Thus, instead of at time of ICU admission, the patient had a severity score of 76, we may have although this patient's severity score at the time of admission was decreasing by 4 points per hour per 10 mL/kg fluid given, the probability for respiratory instability was increasing by 2.3% per hour given 3 L/min supplemental oxygen. This approach is concordant with work done in other clinical settings (eg, in addition to an absolute value of maximal negative inspiratory pressure or vital capacity, the rate of deterioration of neuromuscular weakness in Guillain‐Barr syndrome is also important in predicting respiratory failure[8]).
Electronic data also could permit better definition of patient preferences regarding escalation of care. At present, available electronic data are limited (primarily, orders such as do not resuscitate).[9] However, this EMR domain is gradually expanding.[10, 11] Entities such as the National Institutes of Health could develop sophisticated and rapid questionnaires around patient preferences that are similar to those developed for the Patient Reported Outcomes Measurement Information System.[12] Such tools could have a significant effect on our ability to quantify the benefits of early detection as it relates to a patient's preferences (including better delineation of what treatments they would and would not want).
ACTIVATING A RESPONSE ARM
Early identification, antibiotic administration, fluid resuscitation, and source control are now widely felt to constitute low‐hanging fruit for decreasing morbidity and mortality in severe sepsis. All these measures are included in quality improvement programs and sepsis bundles.[13, 14, 15] However, before early interventions can be instituted, sepsis must at least be suspected, hence the need for early detection. The situation with respect to patient deterioration (for reasons other than sepsis) in general medical surgical wards is less clear‐cut. Reasons for deterioration are much more heterogenous and, consequently, early detection is likely necessary but not sufficient for outcomes improvement.
The 2 projects described in this issue describe nonspecific (indicating elevated risk but not specifying what led to the elevation of risk) and sepsis‐specific alerting systems. In the case of the nonspecific system, detection may not lead to an immediate deployment of a response arm. Instead, a secondary evaluation process must be triggered first. Following this evaluation component, a response arm may or may not be required. In contrast, the sepsis‐specific project essentially transforms the general medicalsurgical ward into a screening system. This screening system then also triggers specific bundle components.
Neither of these systems relies on unaided human cognition. In the case of the nonspecific system, a complex equation generates a probability that is displayed in the EMR, with protocols specifying what actions are to be taken when that probability exceeds a prespecified threshold. With respect to the sepsis screening system, clinicians are supported by EMR alerts as well as protocols that increase nursing autonomy when sepsis is suspected.
The distinction between nonspecific (eg, acute respiratory failure or hemodynamic deterioration) and specific (eg, severe sepsis) alerting systems is likely to disappear as advances in the field occur. For example, incorporation of natural language processing would permit inclusion of semantic data, which could be processed so as to prebucket an alert into one that not just gave a probability, but also a likely cause for the elevated probability.
In addition, both types of systems suffer from the limitation of working off a limited database because, in general, current textbooks and training programs primary focus remains that of treatment of full‐blown clinical syndromes. For example, little is known about how one should manage patients with intermediate lactate values, despite evidence showing that a significant percentage of patients who die from sepsis will initially have such values, with 1 study showing 63% as many deaths with initial lactate of 2.5 to 4.0 mmol/L as occurred with an initial lactate of >4.0 mmol/L.[16] Lastly, as is discussed below, both systems will encounter similar problems when it comes to quantifying benefit.
QUANTIFYING BENEFIT
Whereas the notion of deploying RRTs has clearly been successful, success in demonstrating unequivocal benefit remains elusive.[17, 18, 19] Outcome measures vary dramatically across studies and have included the number of RRT calls, decreases in code blue events on the ward, and decreases in inpatient mortality.[20] We suspect that other reasons are behind this problem. First is the lack of adequate risk adjustment and ignoring the impact of patients near the end of life on the denominator. Figure 4 shows recent data from 21 Kaiser Permanente Northern California (KPNC) hospitals, which can now capture care directive orders electronically,[21] illustrates this problem. The majority (53%) of hospital deaths occur among a highly variable proportion (range across hospitals, 6.5%18.0%) of patients who arrive at the hospital with a restricted resuscitation preference (do not resuscitate, partial code, and comfort care only). These patients do not want to die or crash and burn but, were they to trigger an alert, they would not necessarily want to be rescued by being transferred to the ICU either; moreover, internal KPNC analyses show that large numbers of these patients have sepsis and refuse aggressive treatment. The second major confounder is that ICUs save lives. Consequently, although early detection could lead to fewer transfers to the ICU, using the end point of ICU admission is very problematic, because in many cases the goal of alerting systems should be to get patients to the ICU sooner, which would not affect the outcome of transfer to the ICU in a downward direction; in fact, such systems might increase transfer to the ICU.
The complexities summarized in Figure 4 mean that it is likely that formal quantification of benefit will require examination of multiple measures, including balancing measures as described below. It is also evident that, in this respectlack of agreement as to what constitutes a good outcomethe issues being faced here are a reflection of a broader area of disagreement within our profession and society at large that extends to medical conditions other than critical illness.
POTENTIAL HARMS OF EARLY DETECTION
Implementation of early detection and rapid response systems are not inherently free of harm. If these systems are not shown to have benefit, then the cost of operating them is moving resources away from other, possibly evidence‐based, interventions.[22] At the individual level, alerts could frighten patients and their families (for example, some people are very uncomfortable with the idea that one can predict events). Physicians and nurses who work in the hospital are already quite busy, so every time an alert is issued, it adds to the demand on their already limited time, hence, the critical importance of strategies to minimize false alarms and alert fatigue. Moreover, altering existing workflows can be disruptive and unpopular.
A potentially more quantifiable problem is the impact of early detection systems on ICU operations. For example, if an RRT decides to transfer a patient from the ward to the ICU as a preventive measure (soft landing) and this in turn ties up an ICU bed, that bed is then unavailable for a new patient in the emergency department. Similarly, early detection systems coupled with structured protocols for promoting soft landings could result in a change in ICU case mix, with greater patient flow due to increased numbers of patients with lower severity and lower ICU length of stay. These considerations suggest the need to couple early detection with other supportive data systems and workflows (eg, systems that monitor bed capacity proactively).
Lastly, if documentation protocols are not established and followed, early detection systems could expose both individual clinicians as well as healthcare institutions to medicallegal risk. This consideration could be particularly important in those instances where an alert is issued and, for whatever reasons, clinicians do not take action and do not document that decision. At present, early detection systems are relatively uncommon, but they may gradually become standard of care. This means that in‐house out of ICU deteriorations, which are generally considered to be bad luck or due to a specific error or oversight, may then be considered to be preventable. Another possible scenario that could arise is that of plaintiffs invoking enterprise liability, where a hospital's not having an early detection system becomes considered negligent.
ARTICLES IN THIS ISSUE
In this issue of the Journal of Hospital Medicine, we examine early detection from various perspectives but around a common theme that usually gets less attention in the academic literature: implementation. The article by Schorr et al.[23] describes a disease‐specific approach that can be instantiated using either electronic or paper tools. Escobar et al.[24] describe the quantitative as well as the electronic architecture of an early warning system (EWS) pilot at 2 hospitals that are part of an integrated healthcare delivery system. Dummett et al.[25] then show how a clinical rescue component was developed to take advantage of the EWS, whereas Granich et al.[26] describe the complementary component (integration of supportive care and ensuring that patient preferences are respected). The paper by Liu et al.[27] concludes by placing all of this work in a much broader context, that of the learning healthcare system.
FUTURE DIRECTIONS: KEY GAPS IN THE FIELD
Important gaps remain with respect to early detection and response systems. Future research will need to focus on a number of areas. First and foremost, better approaches to quantifying the costbenefit relationships of these systems are needed; somehow, we need to move beyond a purely intuitive sense that they are good things. Related to this is the need to establish metrics that would permit rigorous comparisons between different approaches; this work needs to go beyond simple comparisons of the statistical characteristics of different predictive models. Ideally, it should include comparisons of different approaches for the response arms as well. We also need to characterize clinician understanding about detection systems, what constitutes impending or incipient critical illness, and the optimum way to provide early detection. Finally, better approaches to integrating health services research with basic science work must be developed; for example, how should one test new biomarkers in settings with early detection and response systems?
The most important frontier, however, is how one can make early detection and response systems more patient centered and how one can enhance their ability to respect patient preferences. Developing systems to improve clinical management is laudable, but somehow we need to also find ways to have these systems make a better connection to what patients want most and what matters most to them, something that may need to include new ways that sometimes suspend use of these systems. At the end of the day, after early detection, patients must have a care experience that they see as an unequivocal improvement.
Acknowledgements
The authors thank our 2 foundation program officers, Dr. Marybeth Sharpe and Ms. Kate Weiland, for their administrative support and encouragement. The authors also thank Dr. Tracy Lieu, Dr. Michelle Caughey, Dr. Philip Madvig, and Ms. Barbara Crawford for their administrative assistance, Dr. Vincent Liu for comments on the manuscript, and Ms. Rachel Lesser for her help with formatting the manuscript and figures.
Disclosures
This work was supported by the Gordon and Betty Moore Foundation, The Permanente Medical Group, Inc., and Kaiser Foundation Hospitals, Inc. As part of our agreement with the Moore Foundation, we made a commitment to disseminate our findings in articles such as this one. However, the Gordon and Betty Moore Foundation and its staff played no role in how we actually structured our articles, nor did they review or preapprove any of the manuscripts submitted as part of the dissemination component. None of the authors has any conflicts of interest to declare of relevance to this work.
This issue of the Journal of Hospital Medicine describes 2 research and quality improvement demonstration projects funded by the Gordon and Betty Moore Foundation. Early detection is central to both projects. This introductory article does not provide a global review of the now voluminous literature on rapid response teams (RRTs), sepsis detection systems, or treatment protocols. Rather, it takes a step back and reassesses just what early detection and quantification of critical illness are. It then examines the implications of early detection and its quantification.
CONCEPTUAL FRAMEWORK
We define severe illness as the presence of acute disease such that a person can no longer expect to improve without dedicated hospital treatment but which is not inevitably associated with mortality, postdischarge morbidity, or major loss of autonomy. In contrast, we define critical illness as acute disease with high a priori risk of mortality, postdischarge morbidity, and major (possibly total) loss of autonomy. We accept that the boundaries between ordinary illness, severe illness, and critical illness are blurred. The basic assumption behind all efforts at early detection is that these edges can be made sharp, and that the knowledge base required to do so can also lead to improvements in treatment protocols and patient outcomes. Further, it is assumed that at least some forms of critical illness can be prevented or mitigated by earlier detection, identification, and treatment.
Research over the last 2 decades has provided important support for this intuitive view as well as making it more nuanced. With respect to epidemiology, the big news is that sepsis is the biggest culprit, and that it accounts for a substantial proportion of all hospital deaths, including many previously considered unexpected hospital deaths due to in‐hospital deterioration.[1] With respect to treatment, a number of studies have demonstrated that crucial therapies previously considered to be intensive care unit (ICU) therapies can be initiated in the emergency department or general medicalsurgical ward.[2]
Figure 1 shows an idealized framework for illness presenting in the emergency department or general medicalsurgical wards. It illustrates the notion that a transition period exists when patients may be rescued with less intense therapy than will be required when condition progression occurs. Once a certain threshold is crossed, the risk of death or major postdischarge morbidity rises exponentially. Unaided human cognition's ability to determine where a given patient is in this continuum is dangerously variable and is highly dependent on the individuals training and experience. Consequently, as described in several of the articles in this issue as well as multiple other publications, health systems are employing comprehensive electronic medical records (EMRs) and are migrating to algorithmic approaches that combine multiple types of patient data.[3, 4] Although we are still some distance from being able to define exact boundaries between illness, severe illness, and critical illness, current EMRs permit much better definition of patient states, care processes, and short‐term outcomes.
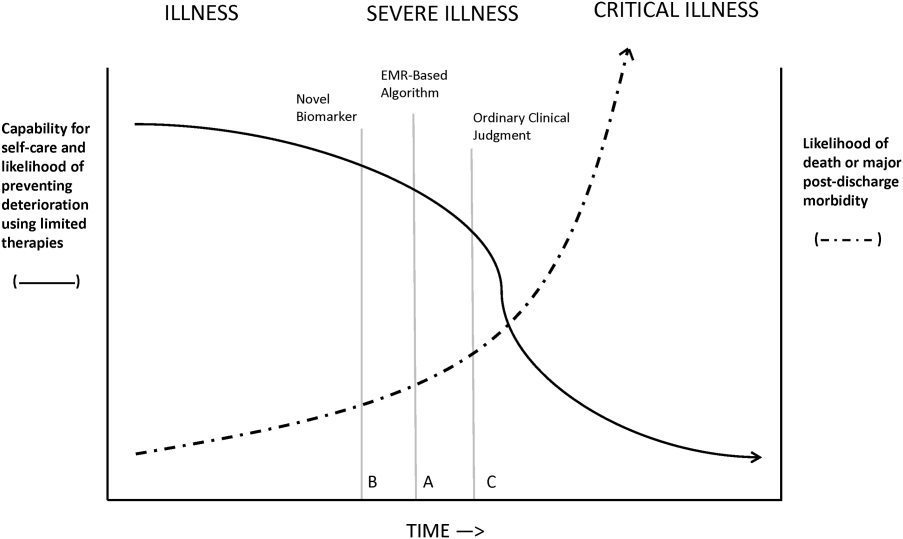
Whereas our ability to quantify many processes and short‐term outcomes is expanding rapidly, quantification of the possible benefit of early detection is complicated by the fact that, even in the best of circumstances, not all patients can be rescued. For some patients, rescue may be temporary, raising the prospect of repeated episodes of critical illness and prolonged intensive care without any hope of leaving the hospital. Figure 2 shows that, for these patients, the problem is no longer simply one of preventing death and preserving function but, rather, preserving autonomy and dignity. In this context, early detection means earlier specification of patient preferences.[5, 6]
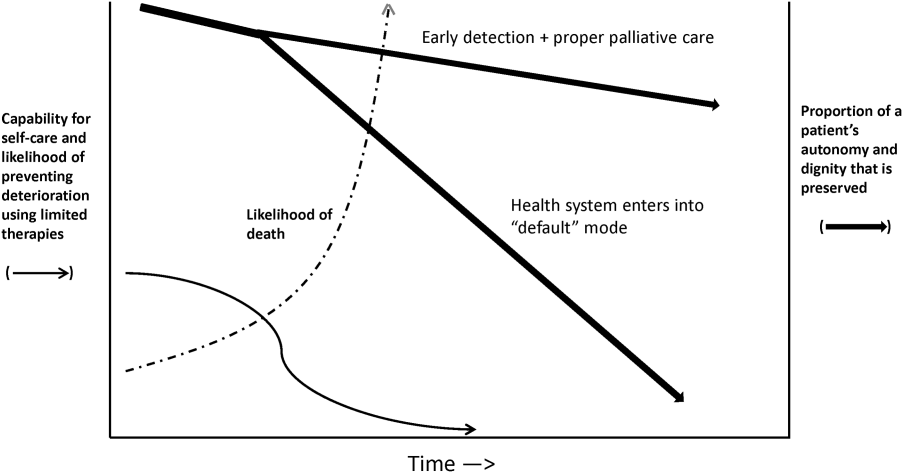
JUST WHAT CONSTITUTES EARLY DETECTION (AND HOW DO WE QUANTIFY IT)?
RRTs arose as the result of a number of studies showing thatin retrospectin‐hospital deteriorations should not have been unexpected. Given comprehensive inpatient EMRs, it is now possible to develop more rigorous definitions. A minimum set of parameters that one would need to specify for proper quantification of early detection is shown on Figure 3. The first is specifying a T0, that is, the moment when a prediction regarding event X (which needs to be defined) is issued. This is different from the (currently unmeasurable) biologic onset of illness as well as the first documented indication that critical illness was present. Further, it is important to be explicit about the event time frame (the time period during which a predicted event is expected to occur): we are predicting that X will occur within E hours of the T0. The time frame between the T0 and X, which we are referring to as lead time, is clinically very important, as it represents the time period during which the response arm (eg, RRT intervention) is to be instituted. Statistical approaches can be used to estimate it, but once an early detection system is in place, it can be quantified. Figure 3 is not restricted to electronic systems; all components shown can be and are used by unaided human cognition.
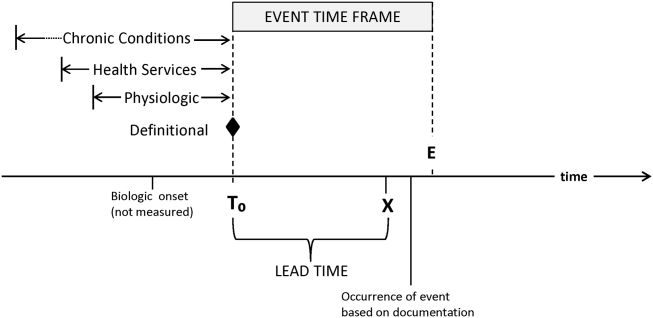
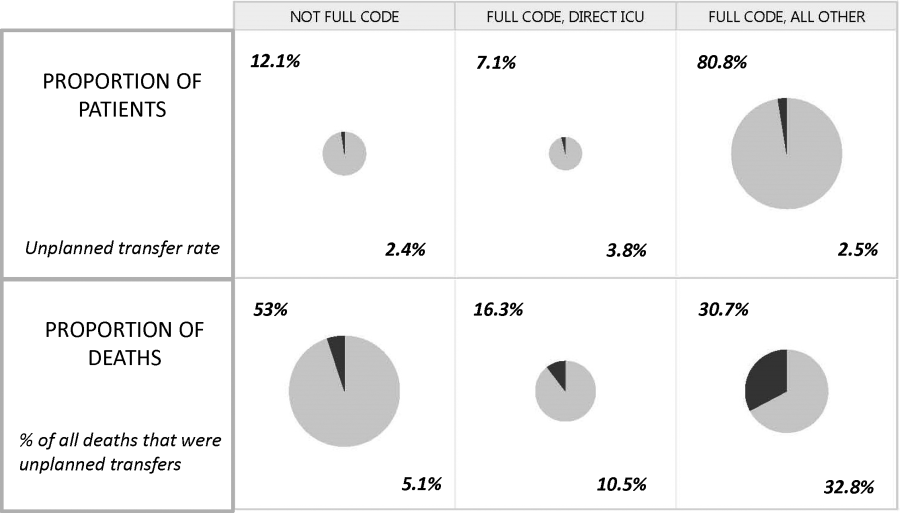
It is essential to specify what data are used to generate probability estimates as well as the time frames used, which we refer to as the look‐back time frames. Several types of data could be employed, with some data elements (eg, age or gender) being discrete data with a 1:1 fixed correspondence between the patient and the data. Other data have a many‐to‐1 relationship, and an exact look‐back time frame must be specified for each data type. For example, it seems reasonable to specify a short (1224 hours) look‐back period for some types of data (eg, vital signs, lactate, admission diagnosis or chief complaint), an intermediate time period (13 days) for information on the current encounter, and a longer (months to years) time period for preexisting illness or comorbidity burden.
Because many events are rare, traditional measures used to assess model performance, such as the area under the receiver operator characteristic curve (C statistic), are not as helpful.[7] Consequently, much more emphasis needs to be given to 2 key metrics: number needed to evaluate (or workup to detection ratio) and threshold‐specific sensitivity (ability of the alert to detect X at a given threshold). With these, one can answer 3 questions that will be asked by the physicians and nurses who are not likely to be researchers, and who will have little interest in the statistics: How many patients do I need to work up each day? How many patients will I need to work up for each possible outcome identified? For this amount of work, how many of the possible outcomes will we catch?
Data availability for the study of severe and critical illness continues to expand. Practically, this means that future research will require more nuanced ontologies for the classification of physiologic derangement. Current approaches to severity scoring (collapsing data into composite scores) need to be replaced by dynamic approaches that consider differential effects on organ systems as well as what can be measured. Severity scoring will also need to incorporate the rate of change of a score (or probability derived from a score) in predicting the occurrence of an event of interest as well as judging response to treatment. Thus, instead of at time of ICU admission, the patient had a severity score of 76, we may have although this patient's severity score at the time of admission was decreasing by 4 points per hour per 10 mL/kg fluid given, the probability for respiratory instability was increasing by 2.3% per hour given 3 L/min supplemental oxygen. This approach is concordant with work done in other clinical settings (eg, in addition to an absolute value of maximal negative inspiratory pressure or vital capacity, the rate of deterioration of neuromuscular weakness in Guillain‐Barr syndrome is also important in predicting respiratory failure[8]).
Electronic data also could permit better definition of patient preferences regarding escalation of care. At present, available electronic data are limited (primarily, orders such as do not resuscitate).[9] However, this EMR domain is gradually expanding.[10, 11] Entities such as the National Institutes of Health could develop sophisticated and rapid questionnaires around patient preferences that are similar to those developed for the Patient Reported Outcomes Measurement Information System.[12] Such tools could have a significant effect on our ability to quantify the benefits of early detection as it relates to a patient's preferences (including better delineation of what treatments they would and would not want).
ACTIVATING A RESPONSE ARM
Early identification, antibiotic administration, fluid resuscitation, and source control are now widely felt to constitute low‐hanging fruit for decreasing morbidity and mortality in severe sepsis. All these measures are included in quality improvement programs and sepsis bundles.[13, 14, 15] However, before early interventions can be instituted, sepsis must at least be suspected, hence the need for early detection. The situation with respect to patient deterioration (for reasons other than sepsis) in general medical surgical wards is less clear‐cut. Reasons for deterioration are much more heterogenous and, consequently, early detection is likely necessary but not sufficient for outcomes improvement.
The 2 projects described in this issue describe nonspecific (indicating elevated risk but not specifying what led to the elevation of risk) and sepsis‐specific alerting systems. In the case of the nonspecific system, detection may not lead to an immediate deployment of a response arm. Instead, a secondary evaluation process must be triggered first. Following this evaluation component, a response arm may or may not be required. In contrast, the sepsis‐specific project essentially transforms the general medicalsurgical ward into a screening system. This screening system then also triggers specific bundle components.
Neither of these systems relies on unaided human cognition. In the case of the nonspecific system, a complex equation generates a probability that is displayed in the EMR, with protocols specifying what actions are to be taken when that probability exceeds a prespecified threshold. With respect to the sepsis screening system, clinicians are supported by EMR alerts as well as protocols that increase nursing autonomy when sepsis is suspected.
The distinction between nonspecific (eg, acute respiratory failure or hemodynamic deterioration) and specific (eg, severe sepsis) alerting systems is likely to disappear as advances in the field occur. For example, incorporation of natural language processing would permit inclusion of semantic data, which could be processed so as to prebucket an alert into one that not just gave a probability, but also a likely cause for the elevated probability.
In addition, both types of systems suffer from the limitation of working off a limited database because, in general, current textbooks and training programs primary focus remains that of treatment of full‐blown clinical syndromes. For example, little is known about how one should manage patients with intermediate lactate values, despite evidence showing that a significant percentage of patients who die from sepsis will initially have such values, with 1 study showing 63% as many deaths with initial lactate of 2.5 to 4.0 mmol/L as occurred with an initial lactate of >4.0 mmol/L.[16] Lastly, as is discussed below, both systems will encounter similar problems when it comes to quantifying benefit.
QUANTIFYING BENEFIT
Whereas the notion of deploying RRTs has clearly been successful, success in demonstrating unequivocal benefit remains elusive.[17, 18, 19] Outcome measures vary dramatically across studies and have included the number of RRT calls, decreases in code blue events on the ward, and decreases in inpatient mortality.[20] We suspect that other reasons are behind this problem. First is the lack of adequate risk adjustment and ignoring the impact of patients near the end of life on the denominator. Figure 4 shows recent data from 21 Kaiser Permanente Northern California (KPNC) hospitals, which can now capture care directive orders electronically,[21] illustrates this problem. The majority (53%) of hospital deaths occur among a highly variable proportion (range across hospitals, 6.5%18.0%) of patients who arrive at the hospital with a restricted resuscitation preference (do not resuscitate, partial code, and comfort care only). These patients do not want to die or crash and burn but, were they to trigger an alert, they would not necessarily want to be rescued by being transferred to the ICU either; moreover, internal KPNC analyses show that large numbers of these patients have sepsis and refuse aggressive treatment. The second major confounder is that ICUs save lives. Consequently, although early detection could lead to fewer transfers to the ICU, using the end point of ICU admission is very problematic, because in many cases the goal of alerting systems should be to get patients to the ICU sooner, which would not affect the outcome of transfer to the ICU in a downward direction; in fact, such systems might increase transfer to the ICU.
The complexities summarized in Figure 4 mean that it is likely that formal quantification of benefit will require examination of multiple measures, including balancing measures as described below. It is also evident that, in this respectlack of agreement as to what constitutes a good outcomethe issues being faced here are a reflection of a broader area of disagreement within our profession and society at large that extends to medical conditions other than critical illness.
POTENTIAL HARMS OF EARLY DETECTION
Implementation of early detection and rapid response systems are not inherently free of harm. If these systems are not shown to have benefit, then the cost of operating them is moving resources away from other, possibly evidence‐based, interventions.[22] At the individual level, alerts could frighten patients and their families (for example, some people are very uncomfortable with the idea that one can predict events). Physicians and nurses who work in the hospital are already quite busy, so every time an alert is issued, it adds to the demand on their already limited time, hence, the critical importance of strategies to minimize false alarms and alert fatigue. Moreover, altering existing workflows can be disruptive and unpopular.
A potentially more quantifiable problem is the impact of early detection systems on ICU operations. For example, if an RRT decides to transfer a patient from the ward to the ICU as a preventive measure (soft landing) and this in turn ties up an ICU bed, that bed is then unavailable for a new patient in the emergency department. Similarly, early detection systems coupled with structured protocols for promoting soft landings could result in a change in ICU case mix, with greater patient flow due to increased numbers of patients with lower severity and lower ICU length of stay. These considerations suggest the need to couple early detection with other supportive data systems and workflows (eg, systems that monitor bed capacity proactively).
Lastly, if documentation protocols are not established and followed, early detection systems could expose both individual clinicians as well as healthcare institutions to medicallegal risk. This consideration could be particularly important in those instances where an alert is issued and, for whatever reasons, clinicians do not take action and do not document that decision. At present, early detection systems are relatively uncommon, but they may gradually become standard of care. This means that in‐house out of ICU deteriorations, which are generally considered to be bad luck or due to a specific error or oversight, may then be considered to be preventable. Another possible scenario that could arise is that of plaintiffs invoking enterprise liability, where a hospital's not having an early detection system becomes considered negligent.
ARTICLES IN THIS ISSUE
In this issue of the Journal of Hospital Medicine, we examine early detection from various perspectives but around a common theme that usually gets less attention in the academic literature: implementation. The article by Schorr et al.[23] describes a disease‐specific approach that can be instantiated using either electronic or paper tools. Escobar et al.[24] describe the quantitative as well as the electronic architecture of an early warning system (EWS) pilot at 2 hospitals that are part of an integrated healthcare delivery system. Dummett et al.[25] then show how a clinical rescue component was developed to take advantage of the EWS, whereas Granich et al.[26] describe the complementary component (integration of supportive care and ensuring that patient preferences are respected). The paper by Liu et al.[27] concludes by placing all of this work in a much broader context, that of the learning healthcare system.
FUTURE DIRECTIONS: KEY GAPS IN THE FIELD
Important gaps remain with respect to early detection and response systems. Future research will need to focus on a number of areas. First and foremost, better approaches to quantifying the costbenefit relationships of these systems are needed; somehow, we need to move beyond a purely intuitive sense that they are good things. Related to this is the need to establish metrics that would permit rigorous comparisons between different approaches; this work needs to go beyond simple comparisons of the statistical characteristics of different predictive models. Ideally, it should include comparisons of different approaches for the response arms as well. We also need to characterize clinician understanding about detection systems, what constitutes impending or incipient critical illness, and the optimum way to provide early detection. Finally, better approaches to integrating health services research with basic science work must be developed; for example, how should one test new biomarkers in settings with early detection and response systems?
The most important frontier, however, is how one can make early detection and response systems more patient centered and how one can enhance their ability to respect patient preferences. Developing systems to improve clinical management is laudable, but somehow we need to also find ways to have these systems make a better connection to what patients want most and what matters most to them, something that may need to include new ways that sometimes suspend use of these systems. At the end of the day, after early detection, patients must have a care experience that they see as an unequivocal improvement.
Acknowledgements
The authors thank our 2 foundation program officers, Dr. Marybeth Sharpe and Ms. Kate Weiland, for their administrative support and encouragement. The authors also thank Dr. Tracy Lieu, Dr. Michelle Caughey, Dr. Philip Madvig, and Ms. Barbara Crawford for their administrative assistance, Dr. Vincent Liu for comments on the manuscript, and Ms. Rachel Lesser for her help with formatting the manuscript and figures.
Disclosures
This work was supported by the Gordon and Betty Moore Foundation, The Permanente Medical Group, Inc., and Kaiser Foundation Hospitals, Inc. As part of our agreement with the Moore Foundation, we made a commitment to disseminate our findings in articles such as this one. However, the Gordon and Betty Moore Foundation and its staff played no role in how we actually structured our articles, nor did they review or preapprove any of the manuscripts submitted as part of the dissemination component. None of the authors has any conflicts of interest to declare of relevance to this work.
- Inpatient care for septicemia or sepsis: a challenge for patients and hospitals. NCHS Data Brief. 2011(62):1–8. , , ,
- Surviving sepsis campaign: association between performance metrics and outcomes in a 7.5‐year study. Crit Care Med. 2015;43(1):3–12. , , , et al.
- Early detection of impending physiologic deterioration among patients who are not in intensive care: development of predictive models using data from an automated electronic medical record. J Hosp Med. 2012;7(5):388–395. , , , , ,
- A randomized trial of real‐time automated clinical deterioration alerts sent to a rapid response team. J Hosp Med. 2014;9(7):424–429. , , , et al.
- Enhanced end‐of‐life care associated with deploying a rapid response team: a pilot study. J Hosp Med. 2009;4(7):449–452. , , , , ,
- The medical emergency team call: a sentinel event that triggers goals of care discussion. Crit Care Med. 2014;42(2):322–327. , , , ,
- Why the C‐statistic is not informative to evaluate early warning scores and what metrics to use. Crit Care. 2015;19:285. , , ,
- Anticipating mechanical ventilation in Guillain‐Barre syndrome. Arch Neurol. 2001;58(6):893–898. , , , ,
- The natural history of changes in preferences for life‐sustaining treatments and implications for inpatient mortality in younger and older hospitalized adults. J Am Geriatr Soc. 2016;64(5):981–989. , , , , ,
- Remote collection of questionnaires. Clin Exp Rheumatol. 2014;32(5 suppl 85):S168–S172. ,
- Be prepared to make your health care wishes known. Health care directives. Allina Health website. Available at: http://www.allinahealth.org/Customer-Service/Be-prepared/Be-prepared-to-make-your-health-care-wishes-known. Accessed January 1, 2015.
- Patient Reported Outcomes Measurement Information System. Dynamic tools to measure health outcomes from the patient perspective. Available at: http://www.nihpromis.org. Accessed January 15, 2015.
- Methodology of the surviving sepsis campaign global initiative for improving care of the patient with severe sepsis. Minerva Anestesiol. 2009;75(suppl 1):23–27. , , , , ,
- The Surviving Sepsis Campaign: a history and a perspective. Surg Infect (Larchmt). 2010;11(3):275–281. , ,
- The Surviving Sepsis Campaign: past, present and future. Trends Mol Med. 2014;20(4):192–194. ,
- Serum lactate as a predictor of mortality in emergency department patients with infection. Ann Emerg Med. 2005;45(5):524–528. , , , et al.
- Effect of a rapid response team on hospital‐wide mortality and code rates outside the ICU in a Children's Hospital. JAMA. 2007;298(19):2267–2274. , , , et al.
- Effects of a medical emergency team on reduction of incidence of and mortality from unexpected cardiac arrests in hospital: preliminary study. BMJ. 2002;324(7334):387–390. , , , , ,
- Rapid response teams: qualitative analysis of their effectiveness. Am J Crit Care. 2013;22(3):198–210. ,
- Hospital‐wide code rates and mortality before and after implementation of a rapid response team. JAMA. 2008;300(21):2506–2513. , , , , ,
- Risk‐adjusting hospital mortality using a comprehensive electronic record in an integrated healthcare delivery system. Med Care. 2013;51(5):446–453. , , , ,
- Rapid response teams—walk, don't run. JAMA. 2006;296(13):1645–1647. , ,
- Schorr et al. J Hosp Med. 2016;11:000–000.
- Piloting electronic medical record–based early detection of inpatient deterioration in community hospitals. J Hosp Med. 2016;11:000–000. , , , et al.
- Dummett et al. J Hosp Med. 2016;11:000–000.
- Granich et al. J Hosp Med. 2016;11:000–000.
- Liu et al. , , , , , Data that drive: closing the loop in the learning hospital system. J Hosp Med. 2016;11:000–000.
- Inpatient care for septicemia or sepsis: a challenge for patients and hospitals. NCHS Data Brief. 2011(62):1–8. , , ,
- Surviving sepsis campaign: association between performance metrics and outcomes in a 7.5‐year study. Crit Care Med. 2015;43(1):3–12. , , , et al.
- Early detection of impending physiologic deterioration among patients who are not in intensive care: development of predictive models using data from an automated electronic medical record. J Hosp Med. 2012;7(5):388–395. , , , , ,
- A randomized trial of real‐time automated clinical deterioration alerts sent to a rapid response team. J Hosp Med. 2014;9(7):424–429. , , , et al.
- Enhanced end‐of‐life care associated with deploying a rapid response team: a pilot study. J Hosp Med. 2009;4(7):449–452. , , , , ,
- The medical emergency team call: a sentinel event that triggers goals of care discussion. Crit Care Med. 2014;42(2):322–327. , , , ,
- Why the C‐statistic is not informative to evaluate early warning scores and what metrics to use. Crit Care. 2015;19:285. , , ,
- Anticipating mechanical ventilation in Guillain‐Barre syndrome. Arch Neurol. 2001;58(6):893–898. , , , ,
- The natural history of changes in preferences for life‐sustaining treatments and implications for inpatient mortality in younger and older hospitalized adults. J Am Geriatr Soc. 2016;64(5):981–989. , , , , ,
- Remote collection of questionnaires. Clin Exp Rheumatol. 2014;32(5 suppl 85):S168–S172. ,
- Be prepared to make your health care wishes known. Health care directives. Allina Health website. Available at: http://www.allinahealth.org/Customer-Service/Be-prepared/Be-prepared-to-make-your-health-care-wishes-known. Accessed January 1, 2015.
- Patient Reported Outcomes Measurement Information System. Dynamic tools to measure health outcomes from the patient perspective. Available at: http://www.nihpromis.org. Accessed January 15, 2015.
- Methodology of the surviving sepsis campaign global initiative for improving care of the patient with severe sepsis. Minerva Anestesiol. 2009;75(suppl 1):23–27. , , , , ,
- The Surviving Sepsis Campaign: a history and a perspective. Surg Infect (Larchmt). 2010;11(3):275–281. , ,
- The Surviving Sepsis Campaign: past, present and future. Trends Mol Med. 2014;20(4):192–194. ,
- Serum lactate as a predictor of mortality in emergency department patients with infection. Ann Emerg Med. 2005;45(5):524–528. , , , et al.
- Effect of a rapid response team on hospital‐wide mortality and code rates outside the ICU in a Children's Hospital. JAMA. 2007;298(19):2267–2274. , , , et al.
- Effects of a medical emergency team on reduction of incidence of and mortality from unexpected cardiac arrests in hospital: preliminary study. BMJ. 2002;324(7334):387–390. , , , , ,
- Rapid response teams: qualitative analysis of their effectiveness. Am J Crit Care. 2013;22(3):198–210. ,
- Hospital‐wide code rates and mortality before and after implementation of a rapid response team. JAMA. 2008;300(21):2506–2513. , , , , ,
- Risk‐adjusting hospital mortality using a comprehensive electronic record in an integrated healthcare delivery system. Med Care. 2013;51(5):446–453. , , , ,
- Rapid response teams—walk, don't run. JAMA. 2006;296(13):1645–1647. , ,
- Schorr et al. J Hosp Med. 2016;11:000–000.
- Piloting electronic medical record–based early detection of inpatient deterioration in community hospitals. J Hosp Med. 2016;11:000–000. , , , et al.
- Dummett et al. J Hosp Med. 2016;11:000–000.
- Granich et al. J Hosp Med. 2016;11:000–000.
- Liu et al. , , , , , Data that drive: closing the loop in the learning hospital system. J Hosp Med. 2016;11:000–000.