User login
Increasing attention has been paid to the need for effective handoffs between healthcare providers since the Joint Commission identified standardized handoff protocols as a National Patient Safety Goal in 2006.1 Aside from adverse consequences for patients, poor handoffs produce provider uncertainty about care plans.[2, 3] Agreement on clinical information after a handoff is critical because a significant proportion of data is not documented in the medical record, leaving providers reliant on verbal communication.[4, 5, 6] Providers may enter the handoff with differing opinions; however, to mitigate the potential safety consequences of discontinuity of care,[7] the goal should be to achieve consensus about proposed courses of action.
Given the recent focus on improving handoffs, rigorous, outcome‐driven measures of handoff quality are clearly needed, but measuring shift‐to‐shift handoff quality has proved challenging.[8, 9] Previous studies of physician handoffs surveyed receivers for satisfaction,[10, 11] compared reported omissions to audio recordings,[3] and developed evaluation tools for receivers to rate handoffs.[12, 13, 14, 15] None directly assess the underlying goal of a handoff: the transfer of understanding from sender to receiver, enabling safe transfer of patient care responsibility.[16] We therefore chose to measure agreement on patient condition and treatment plans following handoff as an indicator of the quality of the shared clinical understanding formed. Advantages of piloting this approach in the pediatric cardiac intensive care unit (CICU) include the relatively homogenous patient population and small number of medical providers. If effective, the strategy of tool development and evaluation could be generalized to different clinical environments and provider groups.
Our aim was to develop and validate a tool to measure the level of shared clinical understanding regarding the condition and treatment plan of a CICU patient after handoff. The tool we designed was the pediatric cardiology Patient Knowledge Assessment Tool (PKAT), a brief, multiple‐item questionnaire focused on key data elements for individual CICU patients. Although variation in provider opinion helps detect diagnostic or treatment errors,[8] the PKAT is based on the assumption that achieving consensus on clinical status and the next steps of care is the goal of the handoff.
METHODS
Setting
The CICU is a 24‐bed medical and surgical unit in a 500‐bed free standing children's hospital. CICU attending physicians work 12‐ or 24‐hour shifts and supervise front line clinicians (including subspecialty fellows, nurse practitioners, and hospitalists, referred to as clinicians in this article) who work day or night shifts. Handoffs occur twice daily, with no significant differences in handoff practices between the 2 times. Attending physicians (referred to as attendings in this article) conduct parallel but separate handoffs from clinicians. All providers work exclusively in the CICU with the exception of fellows, who rotate monthly.
This study was approved by the institutional review board at The Children's Hospital of Philadelphia. All provider subjects provided informed consent. Consent for patient subjects was waived.
Development of the PKAT
We developed the PKAT content domains based on findings from previous studies,[2, 3] unpublished survey data about handoff omissions in our CICU, and CICU attending expert opinion. Pilot testing included 39 attendings and clinicians involved in 60 handoffs representing a wide variety of admissions. Participants were encouraged to share opinions on tool content and design with study staff. The PKAT (see Supporting Information, Appendix, in the online version of this article) was refined iteratively based on this feedback.
Video Simulation Testing
We used video simulation to test the PKAT for inter‐rater reliability. Nine patient handoff scenarios were written with varying levels of patient complexity and clarity of dialogue. The scenarios were filmed using the same actors and location to minimize variability aside from content. We recruited 10 experienced provider subjects (attendings and senior fellows) to minimize the effect of knowledge deficits. For each simulated handoff, subjects were encouraged to annotate a mock sign‐out sheet, which mimicked the content and format of the CICU sign‐out sheet. After watching all 9 scenarios, subjects completed a PKAT for each handoff from the perspective of the receiver based on the videotape. These standardized conditions allowed for assessment of inter‐rater reliability.
In Situ Testing
We then tested the PKAT in situ in the CICU to assess construct validity. We chose to study the morning handoff because the timing and location are more consistent. We planned to study 90 patient handoffs because the standard practice for testing a new psychometric instrument is to collect 10 observations per item.[17] On study days, 4 providers completed a PKAT for each selected handoff: the sending attending, receiving attending, sending clinician, and receiving clinician.
Study days were scheduled over 2 months to encompass a range of providers. Given the small number of attendings, we did not exclude those who had participated in video simulation testing. On study days, 6 patients were enrolled using stratified sampling to ensure adequate representation of new admissions (ie, admitted within 24 hours). The sending attending received the PKAT forms prior to the handoff. The receiving attending and clinicians received the PKAT after handoff. This difference in administration was due to logistic concerns: sending attendings requested to receive the PKATs earlier because they had to complete all 6 PKATs, whereas other providers completed 3 or fewer per day. Thus, sending attendings could complete the PKAT before or after the handoff, whereas all other participants completed the instrument after the handoff.
To test for construct validity, we gathered data on participating providers and patients, hypothesizing that PKAT agreement levels would decrease in response to less experienced providers or more complex patients. Provider characteristics included previous handoff education and amount of time worked in our CICU. Attending CICU experience was dichotomized into first year versus second or greater year. Clinician experience was dichotomized into first or second month versus third or greater month of CICU service. Each PKAT asked the handoff receiver whether he or she had recently cared for this patient or gathered information prior to handoff (eg, speaking to bedside nurse).
Recorded patient characteristics included age, length of stay, and admission type including neonatal/preoperative observation, postoperative (first 7 days after operation), prolonged postoperative (>7 days after operation), and medical (all others). In recognition of differences in handoffs during the first 24 hours of admission and the right‐skewed length of stay in the CICU, we analyzed length of stay based on the following categories: new admission (<24 hours), days 2 to 7, days 8 to 14, days 15 to 31, and >31 days. Because the number of active medications has been shown to correlate with treatment regimen complexity[18] and physician ratings of illness severity,[19] we recorded this number as a surrogate measure of patient complexity. For analytic purposes, we categorized the number of active medications into quartiles.
Provider subject characteristics and PKAT responses were collected using paper forms and entered into REDCap (Research Electronic Data Capture; REDCap Consortium,
Statistical Analysis
The primary outcome measure was the PKAT agreement level among providers evaluating the same handoff. For the reliability assessment, we calculated agreement across all providers analyzing the simulation videos, expecting that multiple providers should have high agreement for the same scenarios if the instrument has high inter‐rater reliability. For the validity assessment, we calculated agreement for each individual handoff by item and then calculated average levels of agreement for each item across provider and patient characteristics. We analyzed handoffs between attendings and clinicians separately. For items with mutually exclusive responses, simple yes/no agreement was calculated. For items requiring at least 1 response, agreement was coded when both respondents selected at least 1 response in common. For items that did not require a selection, credit was given if both subjects agreed that none of the conditions were present or if they agreed that at least 1 condition was present. In a secondary analysis, we repeated the analyses with unique sender‐receiver pair as the unit of analysis to account for correlation in the pair interaction.
Summary statistics were used to describe provider and patient characteristics. Mean rates of agreement with 95% confidence intervals were calculated for each item. The Wilcoxon rank sum test was used to compare mean results between groups (eg, attendings vs clinicians). A nonparametric test for trend, which is an extension of the Wilcoxon rank sum test,[21] was used to compare mean results across ordered categories (eg, length of stay). All tests of significance were at P<0.05 level and 2‐tailed. All statistical analysis was done using Stata 12 (StataCorp, College Station, TX).
RESULTS
Provider subject types are represented in Table 1. Handoffs between these 29 individuals resulted in 70 unique sender and receiver combinations with a median of 2 PKATs completed per unique sender‐receiver pair (range, 115). Attendings had lower rates of handoff education than clinicians (11% vs 85% for in situ testing participants, P=0.01). Attendings participating in in situ testing had worked in the CICU for a median of 3 years (range, 116 years). Clinicians participating in in situ testing had a median of 3 months of CICU experience (range, 195 months). Providers were 100% compliant with PKAT completion.
Simulation Testing, n=10 | In Situ Testing, n=29 | |
---|---|---|
| ||
Attending physicians | 40% (4) | 31% (9) |
Clinicians | 60% (6) | 69% (20) |
Clinician type | ||
Cardiology | 67% (4) | 35% (7) |
Critical care medicine | 33% (2) | 25% (5) |
CICU nurse practitioner | 25% (5) | |
Anesthesia | 5% (1) | |
Neonatology | 5% (1) | |
Hospitalist | 5% (1) |
Video Simulation Testing
Inter‐rater agreement is shown in Figure 1. Raters achieved perfect agreement for 8/9 questions on at least 1 scenario, supporting high inter‐rater reliability for these items. Some items had particularly high reliability. For example, on item 3, subjects achieved perfect agreement for 5/9 scenarios, making 1 both the median and maximum value. Because item 7 (barriers to transfer) did not demonstrate high inter‐rater agreement, we excluded it from the in situ analysis.
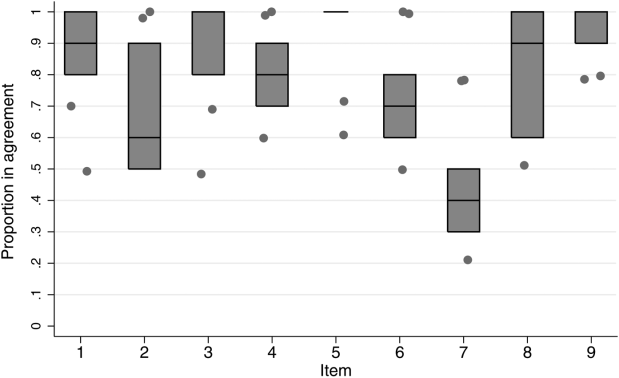
In Situ Testing
Characteristics of patients whose handoffs were selected for in situ testing are listed in Table 2. Because some patients were selected on multiple study days, these 90 handoffs represented 58 unique patients. These 58 patients are representative of the CICU population (data not shown). The number of handoffs studied per patient ranged from 1 to 7 (median 1). A total of 19 patients were included in the study more than once; 13 were included twice.
Characteristic | Categories | Percentage |
---|---|---|
| ||
Age | <1 month | 30 |
112 months | 34 | |
112 years | 28 | |
1318 years | 6 | |
>18 years | 2 | |
Type of admission | Postnatal observation/preoperative | 20 |
Postoperative | 29 | |
Prolonged postoperative (>7 days) | 33 | |
Other admission | 18 | |
CICU days | 1 | 31 |
27 | 22 | |
814 | 10 | |
1531 | 13 | |
>31 | 23 | |
Active medications | <8 | 26 |
811 | 26 | |
1218 | 26 | |
>18 | 23 |
Rates of agreement between handoff pairs, stratified by attending versus clinician, are shown in Table 3. Overall mean levels of agreement ranged from 0.41 to 0.87 (median 0.77). Except for the ratio of pulmonary to systemic blood flow question, there were no significant differences in agreement between attendings as compared to clinicians. When this analysis was repeated with unique sender‐receiver pair as the unit of analysis to account for within‐pair clustering, we obtained qualitatively similar results (data not shown).
PKAT Item | Agreement Level | ||||
---|---|---|---|---|---|
Attending Physician Pair | Clinician Pair | Pa | |||
Mean | 95% CI | Mean | 95% CI | ||
| |||||
Clinical condition | 0.71 | 0.620.81 | 0.78 | 0.690.87 | 0.31 |
Cardiovascular plan | 0.76 | 0.670.85 | 0.68 | 0.580.78 | 0.25 |
Respiratory plan | 0.67 | 0.580.78 | 0.76 | 0.670.85 | 0.26 |
Source of pulmonary blood flow | 0.83 | 0.750.91 | 0.87 | 0.800.94 | 0.53 |
Ratio of pulmonary to systemic flow | 0.67 | 0.570.77 | 0.41 | 0.310.51 | <0.01 |
Anticoagulation indication | 0.79 | 0.700.87 | 0.77 | 0.680.86 | 0.72 |
Active cardiovascular issues | 0.87 | 0.800.94 | 0.76 | 0.670.85 | 0.06 |
Active noncardiovascular issues | 0.80 | 0.720.88 | 0.78 | 0.690.87 | 0.72 |
Both length of stay and increasing number of medications affected agreement levels for PKAT items (Table 4). Increasing length of stay correlated directly with agreement on cardiovascular plan and ratio of pulmonary to systemic flow and inversely with indication for anticoagulation. Increasing number of medications had an inverse correlation with agreement on indication for anticoagulation, active cardiovascular issues, and active noncardiovascular issues.
Item | CICU LOS | No. of Active Medications | |||||||||
---|---|---|---|---|---|---|---|---|---|---|---|
1 Day (n=56) | 27 Days (n=40) | 814 Days (n=18) | 1531 Days (n=24) | >31 Days (n=42) | Pa | 8 (n=46) | 811 (n=46) | 1218 (n=46) | >18 (n=42) | Pa | |
| |||||||||||
Clinical condition | 0.75 | 0.63 | 0.78 | 0.83 | 0.79 | 0.29 | 0.71 | 0.70 | 0.78 | 0.79 | 0.32 |
Cardiovascular plan | 0.59 | 0.73 | 0.67 | 0.79 | 0.86 | <0.01 | 0.63 | 0.72 | 0.63 | 0.81 | 0.16 |
Respiratory plan | 0.68 | 0.78 | 0.61 | 0.83 | 0.69 | 0.79 | 0.67 | 0.72 | 0.78 | 0.69 | 0.68 |
Source of pulmonary blood flow | 0.93 | 0.75 | 0.72 | 0.96 | 0.83 | 0.63 | 0.72 | 0.91 | 0.98 | 0.79 | 0.22 |
Ratio of pulmonary to systemic flow | 0.45 | 0.40 | 0.67 | 0.75 | 0.62 | 0.01 | 0.46 | 0.52 | 0.52 | 0.67 | 0.06 |
Anticoagulation indication | 0.89 | 0.83 | 0.89 | 0.67 | 0.60 | <0.01 | 0.93 | 0.78 | 0.76 | 0.62 | <0.01 |
Active cardiovascular issues | 0.86 | 0.78 | 0.72 | 0.92 | 0.76 | 0.52 | 0.87 | 0.76 | 0.54 | 0.55 | <0.01 |
Active noncardiovascular issues | 0.86 | 0.80 | 0.72 | 0.75 | 0.74 | 0.12 | 0.83 | 0.83 | 0.76 | 0.52 | <0.01 |
In contrast, there were no significant differences in item agreement levels based on provider characteristics, including experience, handoff education, prehandoff preparation, or continuity (data not shown).
CONCLUSIONS
Our results provide initial evidence of reliability and validity of scores for a novel tool, the PKAT, designed to assess providers' shared clinical understanding of a pediatric CICU patient's condition and treatment plan. Because this information should be mutually understood following any handoff, we believe this tool or similar agreement assessments could be used to measure handoff quality across a range of clinical settings. Under the standardized conditions of video simulation, experienced CICU providers achieved high levels of agreement on the PKAT, demonstrating inter‐rater reliability. In situ testing results suggest that the PKAT can validly identify differences in understanding between providers for both routine and complex patients.
The achievement of 100% compliance with in situ testing demonstrates that this type of tool can feasibly be used in a real‐time clinical environment. As expected, mean agreement levels in situ were lower than levels achieved in video simulation. By item, mean levels of agreement for attending and clinician pairs were similar.
Our assessment of PKAT validity demonstrated mixed results. On the one hand, PKAT agreement did not vary significantly by any measured provider characteristics. Consistent with the lack of difference between attendings and clinicians, more experienced providers in both groups did not achieve higher levels of agreement. This finding is surprising, and may illustrate that unmeasured provider characteristics, such as content knowledge, obscure the effects of experience or other measured variables on agreement levels. Alternatively, providing the PKAT to the sending attending prior to the handoff, rather than afterward as for the receiving attendings and clinicians, might have artificially lowered attending agreement levels, concealing a difference due to experience.
On the other hand, construct validity of several items was supported by the difference in agreement levels based on patient characteristics. Agreement levels varied on 5/8 questions as patients became more complex, either defined by length of stay or number of medications. These differences show that agreement on PKAT items responds to changes in handoff complexity, a form of construct validity. Furthermore, these findings suggest that handoffs of more chronic or complex patients may require more attention for components prone to disagreement in these settings. Although complexity and longer length of stay are nonmodifiable risk factors, identifying these handoffs as more susceptible to disagreement provides potential targets for intervention.
It is important to move beyond he said/she said evaluations to assess shared understanding after a handoff, because high fidelity transfer of information is necessary for safe transfer of responsibility. The PKAT addresses this key component of handoff quality in a novel fashion. Although high‐fidelity information transfer may correlate with receiving provider satisfaction, this relationship has not yet been explored. Future studies will evaluate the association between receiver evaluations of handoffs and PKAT agreement, as well as the relationship between PKAT performance and subsequent patient outcomes.
Limitations of this approach include the challenges inherent in reducing a complex understanding of a patient to a multiple‐item instrument. Furthermore, PKAT use may influence handoff content due to the Hawthorne effect. Although our analysis rests on the argument that agreement is the goal of a handoff, some differences of opinion within the care team enrich resilience. Regardless, to maintain continuity of care, providers need to reach agreement on the next steps in a patient's care during the handoff. Because we focused only on agreement, this approach does not compare respondents' answers to a verifiable source of truth, if it exists. Therefore, 2 respondents who agree on the wrong answer receive the same score as 2 who agree on the right answer. Other limitations include using the number of medications as a marker of handoff complexity. Finally, conducting this study in a single CICU limits generalizability. However, we believe that all PKAT items are generalizable to other pediatric CICUs, and that several are generalizable to other pediatric intensive care settings. The approach of measuring shared understanding could be generalized more widely with development of items specific to different clinical settings.
Because the PKAT can be completed and scored quickly, it could be used as a real‐time measure of quality improvement interventions such as the introduction of a standardized handoff protocol. Alternatively, provider pairs could use the PKAT as a final handoff safety check to confirm consensus before transfer of responsibility. The concept of measuring shared clinical understanding could be extended to develop similar instruments for different clinical settings.
Acknowledgements
The authors thank the CICU providers for their enthusiasm for and participation in this study. The authors also thank Margaret Wolff, MD, Newton Buchanan, and the Center for Simulation, Advanced Education and Innovation at The Children's Hospital of Philadelphia for assistance in filming the video scenarios.
Disclosures: Dr. Bates was supported in part by NICHD/T32 HD060550 and NHLBI/T32 HL07915 grant funding. Dr. Metlay was supported by a Mid‐Career Investigator Award in Patient Oriented Research (K24‐AI073957). The authors report no conflicts of interest.
- The published literature on handoffs in hospitals: deficiencies identified in an extensive review. Quality and Safety in Health Care. 2010;19(6):493–497. doi: 10.1136/qshc.2009.033480. , .
- Communication failures in patient sign‐out and suggestions for improvement: a critical incident analysis. Qual Saf Health Care. 2005;14(6):401–407. .
- Consequences of inadequate sign‐out for patient care. Arch Intern Med. 2008;168(16):1755–1760. , , , , .
- Nursing handovers: do we really need them? J Nurs Manag. 2004;12(1):37–42. , , , , , .
- Assessing clinical handover between paramedics and the trauma team. Injury. 2010;41(5):460–464. , , , et al.
- Answering questions on call: Pediatric resident physicians' use of handoffs and other resources. J Hosp Med. 2013;8(6):328–333. , , , , .
- Managing discontinuity in academic medical centers: strategies for a safe and effective resident sign‐out. J Hosp Med. 2006;1(4):257–266. , , , , .
- Patient handoffs: standardized and reliable measurement tools remain elusive. Jt Comm J Qual Patient Saf. 2010;36(2):52–61. , .
- Improving measurement in clinical handover. Qual Saf Health Care. 2009;18(4):272–276. , , , , .
- Adequacy of information transferred at resident sign‐out (inhospital handover of care): a prospective survey. Qual Saf Health Care. 2008;17(1):6–10. , , , .
- Standardized Sign‐out reduces intern perception of medical errors on the general internal medicine ward. Teach Learn Med. 2009;21(2):121–126. , , .
- Hand‐off education and evaluation: piloting the observed simulated hand‐off experience (OSHE). J Gen Intern Med. 2009;25(2):129–134. , , , et al.
- Assessing the quality of patient handoffs at care transitions. Qual Saf Health Care. 2010;19(6):1–5. , , , , .
- Implementing peer evaluation of handoffs: associations with experience and workload. J Hosp Med. 2013;8(3):132–136. , , , , .
- Development of a handoff evaluation tool for shift‐to‐shift physician handoffs: the handoff CEX. J Hosp Med. 2013;8(4):191–200. , , , et al.
- The effects of patient handoff characteristics on subsequent care: a systematic review and areas for future research. Acad Med. 2012;87(8):1105–1124. , .
- Construct validity in organizational behavior. In: Cummings LL, Stawe BM, eds. Research in Organizational Behavior. Vol 2. Greenwich, CT: JAI Press; 1980:3–43. .
- Development and validation of the medication regimen complexity index. Ann Pharmacother. 2004;38(9):1369–1376. , , , , .
- A chronic disease score from automated pharmacy data. J Clin Epidemiol. 1992;45(2):197–203. , , .
- Research electronic data capture (REDCap)—a metadata‐driven methodology and workflow process for providing translational research informatics support. J Biomed Inform. 2009;42(2):377–381. , , , , , .
- A Wilcoxon‐type test for trend. Stat Med. 1985;4(1):87–90. .
Increasing attention has been paid to the need for effective handoffs between healthcare providers since the Joint Commission identified standardized handoff protocols as a National Patient Safety Goal in 2006.1 Aside from adverse consequences for patients, poor handoffs produce provider uncertainty about care plans.[2, 3] Agreement on clinical information after a handoff is critical because a significant proportion of data is not documented in the medical record, leaving providers reliant on verbal communication.[4, 5, 6] Providers may enter the handoff with differing opinions; however, to mitigate the potential safety consequences of discontinuity of care,[7] the goal should be to achieve consensus about proposed courses of action.
Given the recent focus on improving handoffs, rigorous, outcome‐driven measures of handoff quality are clearly needed, but measuring shift‐to‐shift handoff quality has proved challenging.[8, 9] Previous studies of physician handoffs surveyed receivers for satisfaction,[10, 11] compared reported omissions to audio recordings,[3] and developed evaluation tools for receivers to rate handoffs.[12, 13, 14, 15] None directly assess the underlying goal of a handoff: the transfer of understanding from sender to receiver, enabling safe transfer of patient care responsibility.[16] We therefore chose to measure agreement on patient condition and treatment plans following handoff as an indicator of the quality of the shared clinical understanding formed. Advantages of piloting this approach in the pediatric cardiac intensive care unit (CICU) include the relatively homogenous patient population and small number of medical providers. If effective, the strategy of tool development and evaluation could be generalized to different clinical environments and provider groups.
Our aim was to develop and validate a tool to measure the level of shared clinical understanding regarding the condition and treatment plan of a CICU patient after handoff. The tool we designed was the pediatric cardiology Patient Knowledge Assessment Tool (PKAT), a brief, multiple‐item questionnaire focused on key data elements for individual CICU patients. Although variation in provider opinion helps detect diagnostic or treatment errors,[8] the PKAT is based on the assumption that achieving consensus on clinical status and the next steps of care is the goal of the handoff.
METHODS
Setting
The CICU is a 24‐bed medical and surgical unit in a 500‐bed free standing children's hospital. CICU attending physicians work 12‐ or 24‐hour shifts and supervise front line clinicians (including subspecialty fellows, nurse practitioners, and hospitalists, referred to as clinicians in this article) who work day or night shifts. Handoffs occur twice daily, with no significant differences in handoff practices between the 2 times. Attending physicians (referred to as attendings in this article) conduct parallel but separate handoffs from clinicians. All providers work exclusively in the CICU with the exception of fellows, who rotate monthly.
This study was approved by the institutional review board at The Children's Hospital of Philadelphia. All provider subjects provided informed consent. Consent for patient subjects was waived.
Development of the PKAT
We developed the PKAT content domains based on findings from previous studies,[2, 3] unpublished survey data about handoff omissions in our CICU, and CICU attending expert opinion. Pilot testing included 39 attendings and clinicians involved in 60 handoffs representing a wide variety of admissions. Participants were encouraged to share opinions on tool content and design with study staff. The PKAT (see Supporting Information, Appendix, in the online version of this article) was refined iteratively based on this feedback.
Video Simulation Testing
We used video simulation to test the PKAT for inter‐rater reliability. Nine patient handoff scenarios were written with varying levels of patient complexity and clarity of dialogue. The scenarios were filmed using the same actors and location to minimize variability aside from content. We recruited 10 experienced provider subjects (attendings and senior fellows) to minimize the effect of knowledge deficits. For each simulated handoff, subjects were encouraged to annotate a mock sign‐out sheet, which mimicked the content and format of the CICU sign‐out sheet. After watching all 9 scenarios, subjects completed a PKAT for each handoff from the perspective of the receiver based on the videotape. These standardized conditions allowed for assessment of inter‐rater reliability.
In Situ Testing
We then tested the PKAT in situ in the CICU to assess construct validity. We chose to study the morning handoff because the timing and location are more consistent. We planned to study 90 patient handoffs because the standard practice for testing a new psychometric instrument is to collect 10 observations per item.[17] On study days, 4 providers completed a PKAT for each selected handoff: the sending attending, receiving attending, sending clinician, and receiving clinician.
Study days were scheduled over 2 months to encompass a range of providers. Given the small number of attendings, we did not exclude those who had participated in video simulation testing. On study days, 6 patients were enrolled using stratified sampling to ensure adequate representation of new admissions (ie, admitted within 24 hours). The sending attending received the PKAT forms prior to the handoff. The receiving attending and clinicians received the PKAT after handoff. This difference in administration was due to logistic concerns: sending attendings requested to receive the PKATs earlier because they had to complete all 6 PKATs, whereas other providers completed 3 or fewer per day. Thus, sending attendings could complete the PKAT before or after the handoff, whereas all other participants completed the instrument after the handoff.
To test for construct validity, we gathered data on participating providers and patients, hypothesizing that PKAT agreement levels would decrease in response to less experienced providers or more complex patients. Provider characteristics included previous handoff education and amount of time worked in our CICU. Attending CICU experience was dichotomized into first year versus second or greater year. Clinician experience was dichotomized into first or second month versus third or greater month of CICU service. Each PKAT asked the handoff receiver whether he or she had recently cared for this patient or gathered information prior to handoff (eg, speaking to bedside nurse).
Recorded patient characteristics included age, length of stay, and admission type including neonatal/preoperative observation, postoperative (first 7 days after operation), prolonged postoperative (>7 days after operation), and medical (all others). In recognition of differences in handoffs during the first 24 hours of admission and the right‐skewed length of stay in the CICU, we analyzed length of stay based on the following categories: new admission (<24 hours), days 2 to 7, days 8 to 14, days 15 to 31, and >31 days. Because the number of active medications has been shown to correlate with treatment regimen complexity[18] and physician ratings of illness severity,[19] we recorded this number as a surrogate measure of patient complexity. For analytic purposes, we categorized the number of active medications into quartiles.
Provider subject characteristics and PKAT responses were collected using paper forms and entered into REDCap (Research Electronic Data Capture; REDCap Consortium,
Statistical Analysis
The primary outcome measure was the PKAT agreement level among providers evaluating the same handoff. For the reliability assessment, we calculated agreement across all providers analyzing the simulation videos, expecting that multiple providers should have high agreement for the same scenarios if the instrument has high inter‐rater reliability. For the validity assessment, we calculated agreement for each individual handoff by item and then calculated average levels of agreement for each item across provider and patient characteristics. We analyzed handoffs between attendings and clinicians separately. For items with mutually exclusive responses, simple yes/no agreement was calculated. For items requiring at least 1 response, agreement was coded when both respondents selected at least 1 response in common. For items that did not require a selection, credit was given if both subjects agreed that none of the conditions were present or if they agreed that at least 1 condition was present. In a secondary analysis, we repeated the analyses with unique sender‐receiver pair as the unit of analysis to account for correlation in the pair interaction.
Summary statistics were used to describe provider and patient characteristics. Mean rates of agreement with 95% confidence intervals were calculated for each item. The Wilcoxon rank sum test was used to compare mean results between groups (eg, attendings vs clinicians). A nonparametric test for trend, which is an extension of the Wilcoxon rank sum test,[21] was used to compare mean results across ordered categories (eg, length of stay). All tests of significance were at P<0.05 level and 2‐tailed. All statistical analysis was done using Stata 12 (StataCorp, College Station, TX).
RESULTS
Provider subject types are represented in Table 1. Handoffs between these 29 individuals resulted in 70 unique sender and receiver combinations with a median of 2 PKATs completed per unique sender‐receiver pair (range, 115). Attendings had lower rates of handoff education than clinicians (11% vs 85% for in situ testing participants, P=0.01). Attendings participating in in situ testing had worked in the CICU for a median of 3 years (range, 116 years). Clinicians participating in in situ testing had a median of 3 months of CICU experience (range, 195 months). Providers were 100% compliant with PKAT completion.
Simulation Testing, n=10 | In Situ Testing, n=29 | |
---|---|---|
| ||
Attending physicians | 40% (4) | 31% (9) |
Clinicians | 60% (6) | 69% (20) |
Clinician type | ||
Cardiology | 67% (4) | 35% (7) |
Critical care medicine | 33% (2) | 25% (5) |
CICU nurse practitioner | 25% (5) | |
Anesthesia | 5% (1) | |
Neonatology | 5% (1) | |
Hospitalist | 5% (1) |
Video Simulation Testing
Inter‐rater agreement is shown in Figure 1. Raters achieved perfect agreement for 8/9 questions on at least 1 scenario, supporting high inter‐rater reliability for these items. Some items had particularly high reliability. For example, on item 3, subjects achieved perfect agreement for 5/9 scenarios, making 1 both the median and maximum value. Because item 7 (barriers to transfer) did not demonstrate high inter‐rater agreement, we excluded it from the in situ analysis.
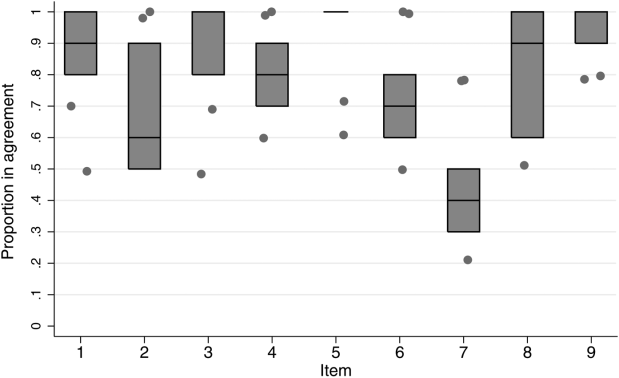
In Situ Testing
Characteristics of patients whose handoffs were selected for in situ testing are listed in Table 2. Because some patients were selected on multiple study days, these 90 handoffs represented 58 unique patients. These 58 patients are representative of the CICU population (data not shown). The number of handoffs studied per patient ranged from 1 to 7 (median 1). A total of 19 patients were included in the study more than once; 13 were included twice.
Characteristic | Categories | Percentage |
---|---|---|
| ||
Age | <1 month | 30 |
112 months | 34 | |
112 years | 28 | |
1318 years | 6 | |
>18 years | 2 | |
Type of admission | Postnatal observation/preoperative | 20 |
Postoperative | 29 | |
Prolonged postoperative (>7 days) | 33 | |
Other admission | 18 | |
CICU days | 1 | 31 |
27 | 22 | |
814 | 10 | |
1531 | 13 | |
>31 | 23 | |
Active medications | <8 | 26 |
811 | 26 | |
1218 | 26 | |
>18 | 23 |
Rates of agreement between handoff pairs, stratified by attending versus clinician, are shown in Table 3. Overall mean levels of agreement ranged from 0.41 to 0.87 (median 0.77). Except for the ratio of pulmonary to systemic blood flow question, there were no significant differences in agreement between attendings as compared to clinicians. When this analysis was repeated with unique sender‐receiver pair as the unit of analysis to account for within‐pair clustering, we obtained qualitatively similar results (data not shown).
PKAT Item | Agreement Level | ||||
---|---|---|---|---|---|
Attending Physician Pair | Clinician Pair | Pa | |||
Mean | 95% CI | Mean | 95% CI | ||
| |||||
Clinical condition | 0.71 | 0.620.81 | 0.78 | 0.690.87 | 0.31 |
Cardiovascular plan | 0.76 | 0.670.85 | 0.68 | 0.580.78 | 0.25 |
Respiratory plan | 0.67 | 0.580.78 | 0.76 | 0.670.85 | 0.26 |
Source of pulmonary blood flow | 0.83 | 0.750.91 | 0.87 | 0.800.94 | 0.53 |
Ratio of pulmonary to systemic flow | 0.67 | 0.570.77 | 0.41 | 0.310.51 | <0.01 |
Anticoagulation indication | 0.79 | 0.700.87 | 0.77 | 0.680.86 | 0.72 |
Active cardiovascular issues | 0.87 | 0.800.94 | 0.76 | 0.670.85 | 0.06 |
Active noncardiovascular issues | 0.80 | 0.720.88 | 0.78 | 0.690.87 | 0.72 |
Both length of stay and increasing number of medications affected agreement levels for PKAT items (Table 4). Increasing length of stay correlated directly with agreement on cardiovascular plan and ratio of pulmonary to systemic flow and inversely with indication for anticoagulation. Increasing number of medications had an inverse correlation with agreement on indication for anticoagulation, active cardiovascular issues, and active noncardiovascular issues.
Item | CICU LOS | No. of Active Medications | |||||||||
---|---|---|---|---|---|---|---|---|---|---|---|
1 Day (n=56) | 27 Days (n=40) | 814 Days (n=18) | 1531 Days (n=24) | >31 Days (n=42) | Pa | 8 (n=46) | 811 (n=46) | 1218 (n=46) | >18 (n=42) | Pa | |
| |||||||||||
Clinical condition | 0.75 | 0.63 | 0.78 | 0.83 | 0.79 | 0.29 | 0.71 | 0.70 | 0.78 | 0.79 | 0.32 |
Cardiovascular plan | 0.59 | 0.73 | 0.67 | 0.79 | 0.86 | <0.01 | 0.63 | 0.72 | 0.63 | 0.81 | 0.16 |
Respiratory plan | 0.68 | 0.78 | 0.61 | 0.83 | 0.69 | 0.79 | 0.67 | 0.72 | 0.78 | 0.69 | 0.68 |
Source of pulmonary blood flow | 0.93 | 0.75 | 0.72 | 0.96 | 0.83 | 0.63 | 0.72 | 0.91 | 0.98 | 0.79 | 0.22 |
Ratio of pulmonary to systemic flow | 0.45 | 0.40 | 0.67 | 0.75 | 0.62 | 0.01 | 0.46 | 0.52 | 0.52 | 0.67 | 0.06 |
Anticoagulation indication | 0.89 | 0.83 | 0.89 | 0.67 | 0.60 | <0.01 | 0.93 | 0.78 | 0.76 | 0.62 | <0.01 |
Active cardiovascular issues | 0.86 | 0.78 | 0.72 | 0.92 | 0.76 | 0.52 | 0.87 | 0.76 | 0.54 | 0.55 | <0.01 |
Active noncardiovascular issues | 0.86 | 0.80 | 0.72 | 0.75 | 0.74 | 0.12 | 0.83 | 0.83 | 0.76 | 0.52 | <0.01 |
In contrast, there were no significant differences in item agreement levels based on provider characteristics, including experience, handoff education, prehandoff preparation, or continuity (data not shown).
CONCLUSIONS
Our results provide initial evidence of reliability and validity of scores for a novel tool, the PKAT, designed to assess providers' shared clinical understanding of a pediatric CICU patient's condition and treatment plan. Because this information should be mutually understood following any handoff, we believe this tool or similar agreement assessments could be used to measure handoff quality across a range of clinical settings. Under the standardized conditions of video simulation, experienced CICU providers achieved high levels of agreement on the PKAT, demonstrating inter‐rater reliability. In situ testing results suggest that the PKAT can validly identify differences in understanding between providers for both routine and complex patients.
The achievement of 100% compliance with in situ testing demonstrates that this type of tool can feasibly be used in a real‐time clinical environment. As expected, mean agreement levels in situ were lower than levels achieved in video simulation. By item, mean levels of agreement for attending and clinician pairs were similar.
Our assessment of PKAT validity demonstrated mixed results. On the one hand, PKAT agreement did not vary significantly by any measured provider characteristics. Consistent with the lack of difference between attendings and clinicians, more experienced providers in both groups did not achieve higher levels of agreement. This finding is surprising, and may illustrate that unmeasured provider characteristics, such as content knowledge, obscure the effects of experience or other measured variables on agreement levels. Alternatively, providing the PKAT to the sending attending prior to the handoff, rather than afterward as for the receiving attendings and clinicians, might have artificially lowered attending agreement levels, concealing a difference due to experience.
On the other hand, construct validity of several items was supported by the difference in agreement levels based on patient characteristics. Agreement levels varied on 5/8 questions as patients became more complex, either defined by length of stay or number of medications. These differences show that agreement on PKAT items responds to changes in handoff complexity, a form of construct validity. Furthermore, these findings suggest that handoffs of more chronic or complex patients may require more attention for components prone to disagreement in these settings. Although complexity and longer length of stay are nonmodifiable risk factors, identifying these handoffs as more susceptible to disagreement provides potential targets for intervention.
It is important to move beyond he said/she said evaluations to assess shared understanding after a handoff, because high fidelity transfer of information is necessary for safe transfer of responsibility. The PKAT addresses this key component of handoff quality in a novel fashion. Although high‐fidelity information transfer may correlate with receiving provider satisfaction, this relationship has not yet been explored. Future studies will evaluate the association between receiver evaluations of handoffs and PKAT agreement, as well as the relationship between PKAT performance and subsequent patient outcomes.
Limitations of this approach include the challenges inherent in reducing a complex understanding of a patient to a multiple‐item instrument. Furthermore, PKAT use may influence handoff content due to the Hawthorne effect. Although our analysis rests on the argument that agreement is the goal of a handoff, some differences of opinion within the care team enrich resilience. Regardless, to maintain continuity of care, providers need to reach agreement on the next steps in a patient's care during the handoff. Because we focused only on agreement, this approach does not compare respondents' answers to a verifiable source of truth, if it exists. Therefore, 2 respondents who agree on the wrong answer receive the same score as 2 who agree on the right answer. Other limitations include using the number of medications as a marker of handoff complexity. Finally, conducting this study in a single CICU limits generalizability. However, we believe that all PKAT items are generalizable to other pediatric CICUs, and that several are generalizable to other pediatric intensive care settings. The approach of measuring shared understanding could be generalized more widely with development of items specific to different clinical settings.
Because the PKAT can be completed and scored quickly, it could be used as a real‐time measure of quality improvement interventions such as the introduction of a standardized handoff protocol. Alternatively, provider pairs could use the PKAT as a final handoff safety check to confirm consensus before transfer of responsibility. The concept of measuring shared clinical understanding could be extended to develop similar instruments for different clinical settings.
Acknowledgements
The authors thank the CICU providers for their enthusiasm for and participation in this study. The authors also thank Margaret Wolff, MD, Newton Buchanan, and the Center for Simulation, Advanced Education and Innovation at The Children's Hospital of Philadelphia for assistance in filming the video scenarios.
Disclosures: Dr. Bates was supported in part by NICHD/T32 HD060550 and NHLBI/T32 HL07915 grant funding. Dr. Metlay was supported by a Mid‐Career Investigator Award in Patient Oriented Research (K24‐AI073957). The authors report no conflicts of interest.
Increasing attention has been paid to the need for effective handoffs between healthcare providers since the Joint Commission identified standardized handoff protocols as a National Patient Safety Goal in 2006.1 Aside from adverse consequences for patients, poor handoffs produce provider uncertainty about care plans.[2, 3] Agreement on clinical information after a handoff is critical because a significant proportion of data is not documented in the medical record, leaving providers reliant on verbal communication.[4, 5, 6] Providers may enter the handoff with differing opinions; however, to mitigate the potential safety consequences of discontinuity of care,[7] the goal should be to achieve consensus about proposed courses of action.
Given the recent focus on improving handoffs, rigorous, outcome‐driven measures of handoff quality are clearly needed, but measuring shift‐to‐shift handoff quality has proved challenging.[8, 9] Previous studies of physician handoffs surveyed receivers for satisfaction,[10, 11] compared reported omissions to audio recordings,[3] and developed evaluation tools for receivers to rate handoffs.[12, 13, 14, 15] None directly assess the underlying goal of a handoff: the transfer of understanding from sender to receiver, enabling safe transfer of patient care responsibility.[16] We therefore chose to measure agreement on patient condition and treatment plans following handoff as an indicator of the quality of the shared clinical understanding formed. Advantages of piloting this approach in the pediatric cardiac intensive care unit (CICU) include the relatively homogenous patient population and small number of medical providers. If effective, the strategy of tool development and evaluation could be generalized to different clinical environments and provider groups.
Our aim was to develop and validate a tool to measure the level of shared clinical understanding regarding the condition and treatment plan of a CICU patient after handoff. The tool we designed was the pediatric cardiology Patient Knowledge Assessment Tool (PKAT), a brief, multiple‐item questionnaire focused on key data elements for individual CICU patients. Although variation in provider opinion helps detect diagnostic or treatment errors,[8] the PKAT is based on the assumption that achieving consensus on clinical status and the next steps of care is the goal of the handoff.
METHODS
Setting
The CICU is a 24‐bed medical and surgical unit in a 500‐bed free standing children's hospital. CICU attending physicians work 12‐ or 24‐hour shifts and supervise front line clinicians (including subspecialty fellows, nurse practitioners, and hospitalists, referred to as clinicians in this article) who work day or night shifts. Handoffs occur twice daily, with no significant differences in handoff practices between the 2 times. Attending physicians (referred to as attendings in this article) conduct parallel but separate handoffs from clinicians. All providers work exclusively in the CICU with the exception of fellows, who rotate monthly.
This study was approved by the institutional review board at The Children's Hospital of Philadelphia. All provider subjects provided informed consent. Consent for patient subjects was waived.
Development of the PKAT
We developed the PKAT content domains based on findings from previous studies,[2, 3] unpublished survey data about handoff omissions in our CICU, and CICU attending expert opinion. Pilot testing included 39 attendings and clinicians involved in 60 handoffs representing a wide variety of admissions. Participants were encouraged to share opinions on tool content and design with study staff. The PKAT (see Supporting Information, Appendix, in the online version of this article) was refined iteratively based on this feedback.
Video Simulation Testing
We used video simulation to test the PKAT for inter‐rater reliability. Nine patient handoff scenarios were written with varying levels of patient complexity and clarity of dialogue. The scenarios were filmed using the same actors and location to minimize variability aside from content. We recruited 10 experienced provider subjects (attendings and senior fellows) to minimize the effect of knowledge deficits. For each simulated handoff, subjects were encouraged to annotate a mock sign‐out sheet, which mimicked the content and format of the CICU sign‐out sheet. After watching all 9 scenarios, subjects completed a PKAT for each handoff from the perspective of the receiver based on the videotape. These standardized conditions allowed for assessment of inter‐rater reliability.
In Situ Testing
We then tested the PKAT in situ in the CICU to assess construct validity. We chose to study the morning handoff because the timing and location are more consistent. We planned to study 90 patient handoffs because the standard practice for testing a new psychometric instrument is to collect 10 observations per item.[17] On study days, 4 providers completed a PKAT for each selected handoff: the sending attending, receiving attending, sending clinician, and receiving clinician.
Study days were scheduled over 2 months to encompass a range of providers. Given the small number of attendings, we did not exclude those who had participated in video simulation testing. On study days, 6 patients were enrolled using stratified sampling to ensure adequate representation of new admissions (ie, admitted within 24 hours). The sending attending received the PKAT forms prior to the handoff. The receiving attending and clinicians received the PKAT after handoff. This difference in administration was due to logistic concerns: sending attendings requested to receive the PKATs earlier because they had to complete all 6 PKATs, whereas other providers completed 3 or fewer per day. Thus, sending attendings could complete the PKAT before or after the handoff, whereas all other participants completed the instrument after the handoff.
To test for construct validity, we gathered data on participating providers and patients, hypothesizing that PKAT agreement levels would decrease in response to less experienced providers or more complex patients. Provider characteristics included previous handoff education and amount of time worked in our CICU. Attending CICU experience was dichotomized into first year versus second or greater year. Clinician experience was dichotomized into first or second month versus third or greater month of CICU service. Each PKAT asked the handoff receiver whether he or she had recently cared for this patient or gathered information prior to handoff (eg, speaking to bedside nurse).
Recorded patient characteristics included age, length of stay, and admission type including neonatal/preoperative observation, postoperative (first 7 days after operation), prolonged postoperative (>7 days after operation), and medical (all others). In recognition of differences in handoffs during the first 24 hours of admission and the right‐skewed length of stay in the CICU, we analyzed length of stay based on the following categories: new admission (<24 hours), days 2 to 7, days 8 to 14, days 15 to 31, and >31 days. Because the number of active medications has been shown to correlate with treatment regimen complexity[18] and physician ratings of illness severity,[19] we recorded this number as a surrogate measure of patient complexity. For analytic purposes, we categorized the number of active medications into quartiles.
Provider subject characteristics and PKAT responses were collected using paper forms and entered into REDCap (Research Electronic Data Capture; REDCap Consortium,
Statistical Analysis
The primary outcome measure was the PKAT agreement level among providers evaluating the same handoff. For the reliability assessment, we calculated agreement across all providers analyzing the simulation videos, expecting that multiple providers should have high agreement for the same scenarios if the instrument has high inter‐rater reliability. For the validity assessment, we calculated agreement for each individual handoff by item and then calculated average levels of agreement for each item across provider and patient characteristics. We analyzed handoffs between attendings and clinicians separately. For items with mutually exclusive responses, simple yes/no agreement was calculated. For items requiring at least 1 response, agreement was coded when both respondents selected at least 1 response in common. For items that did not require a selection, credit was given if both subjects agreed that none of the conditions were present or if they agreed that at least 1 condition was present. In a secondary analysis, we repeated the analyses with unique sender‐receiver pair as the unit of analysis to account for correlation in the pair interaction.
Summary statistics were used to describe provider and patient characteristics. Mean rates of agreement with 95% confidence intervals were calculated for each item. The Wilcoxon rank sum test was used to compare mean results between groups (eg, attendings vs clinicians). A nonparametric test for trend, which is an extension of the Wilcoxon rank sum test,[21] was used to compare mean results across ordered categories (eg, length of stay). All tests of significance were at P<0.05 level and 2‐tailed. All statistical analysis was done using Stata 12 (StataCorp, College Station, TX).
RESULTS
Provider subject types are represented in Table 1. Handoffs between these 29 individuals resulted in 70 unique sender and receiver combinations with a median of 2 PKATs completed per unique sender‐receiver pair (range, 115). Attendings had lower rates of handoff education than clinicians (11% vs 85% for in situ testing participants, P=0.01). Attendings participating in in situ testing had worked in the CICU for a median of 3 years (range, 116 years). Clinicians participating in in situ testing had a median of 3 months of CICU experience (range, 195 months). Providers were 100% compliant with PKAT completion.
Simulation Testing, n=10 | In Situ Testing, n=29 | |
---|---|---|
| ||
Attending physicians | 40% (4) | 31% (9) |
Clinicians | 60% (6) | 69% (20) |
Clinician type | ||
Cardiology | 67% (4) | 35% (7) |
Critical care medicine | 33% (2) | 25% (5) |
CICU nurse practitioner | 25% (5) | |
Anesthesia | 5% (1) | |
Neonatology | 5% (1) | |
Hospitalist | 5% (1) |
Video Simulation Testing
Inter‐rater agreement is shown in Figure 1. Raters achieved perfect agreement for 8/9 questions on at least 1 scenario, supporting high inter‐rater reliability for these items. Some items had particularly high reliability. For example, on item 3, subjects achieved perfect agreement for 5/9 scenarios, making 1 both the median and maximum value. Because item 7 (barriers to transfer) did not demonstrate high inter‐rater agreement, we excluded it from the in situ analysis.
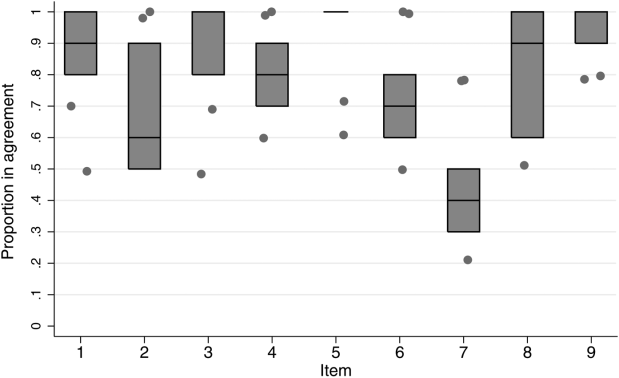
In Situ Testing
Characteristics of patients whose handoffs were selected for in situ testing are listed in Table 2. Because some patients were selected on multiple study days, these 90 handoffs represented 58 unique patients. These 58 patients are representative of the CICU population (data not shown). The number of handoffs studied per patient ranged from 1 to 7 (median 1). A total of 19 patients were included in the study more than once; 13 were included twice.
Characteristic | Categories | Percentage |
---|---|---|
| ||
Age | <1 month | 30 |
112 months | 34 | |
112 years | 28 | |
1318 years | 6 | |
>18 years | 2 | |
Type of admission | Postnatal observation/preoperative | 20 |
Postoperative | 29 | |
Prolonged postoperative (>7 days) | 33 | |
Other admission | 18 | |
CICU days | 1 | 31 |
27 | 22 | |
814 | 10 | |
1531 | 13 | |
>31 | 23 | |
Active medications | <8 | 26 |
811 | 26 | |
1218 | 26 | |
>18 | 23 |
Rates of agreement between handoff pairs, stratified by attending versus clinician, are shown in Table 3. Overall mean levels of agreement ranged from 0.41 to 0.87 (median 0.77). Except for the ratio of pulmonary to systemic blood flow question, there were no significant differences in agreement between attendings as compared to clinicians. When this analysis was repeated with unique sender‐receiver pair as the unit of analysis to account for within‐pair clustering, we obtained qualitatively similar results (data not shown).
PKAT Item | Agreement Level | ||||
---|---|---|---|---|---|
Attending Physician Pair | Clinician Pair | Pa | |||
Mean | 95% CI | Mean | 95% CI | ||
| |||||
Clinical condition | 0.71 | 0.620.81 | 0.78 | 0.690.87 | 0.31 |
Cardiovascular plan | 0.76 | 0.670.85 | 0.68 | 0.580.78 | 0.25 |
Respiratory plan | 0.67 | 0.580.78 | 0.76 | 0.670.85 | 0.26 |
Source of pulmonary blood flow | 0.83 | 0.750.91 | 0.87 | 0.800.94 | 0.53 |
Ratio of pulmonary to systemic flow | 0.67 | 0.570.77 | 0.41 | 0.310.51 | <0.01 |
Anticoagulation indication | 0.79 | 0.700.87 | 0.77 | 0.680.86 | 0.72 |
Active cardiovascular issues | 0.87 | 0.800.94 | 0.76 | 0.670.85 | 0.06 |
Active noncardiovascular issues | 0.80 | 0.720.88 | 0.78 | 0.690.87 | 0.72 |
Both length of stay and increasing number of medications affected agreement levels for PKAT items (Table 4). Increasing length of stay correlated directly with agreement on cardiovascular plan and ratio of pulmonary to systemic flow and inversely with indication for anticoagulation. Increasing number of medications had an inverse correlation with agreement on indication for anticoagulation, active cardiovascular issues, and active noncardiovascular issues.
Item | CICU LOS | No. of Active Medications | |||||||||
---|---|---|---|---|---|---|---|---|---|---|---|
1 Day (n=56) | 27 Days (n=40) | 814 Days (n=18) | 1531 Days (n=24) | >31 Days (n=42) | Pa | 8 (n=46) | 811 (n=46) | 1218 (n=46) | >18 (n=42) | Pa | |
| |||||||||||
Clinical condition | 0.75 | 0.63 | 0.78 | 0.83 | 0.79 | 0.29 | 0.71 | 0.70 | 0.78 | 0.79 | 0.32 |
Cardiovascular plan | 0.59 | 0.73 | 0.67 | 0.79 | 0.86 | <0.01 | 0.63 | 0.72 | 0.63 | 0.81 | 0.16 |
Respiratory plan | 0.68 | 0.78 | 0.61 | 0.83 | 0.69 | 0.79 | 0.67 | 0.72 | 0.78 | 0.69 | 0.68 |
Source of pulmonary blood flow | 0.93 | 0.75 | 0.72 | 0.96 | 0.83 | 0.63 | 0.72 | 0.91 | 0.98 | 0.79 | 0.22 |
Ratio of pulmonary to systemic flow | 0.45 | 0.40 | 0.67 | 0.75 | 0.62 | 0.01 | 0.46 | 0.52 | 0.52 | 0.67 | 0.06 |
Anticoagulation indication | 0.89 | 0.83 | 0.89 | 0.67 | 0.60 | <0.01 | 0.93 | 0.78 | 0.76 | 0.62 | <0.01 |
Active cardiovascular issues | 0.86 | 0.78 | 0.72 | 0.92 | 0.76 | 0.52 | 0.87 | 0.76 | 0.54 | 0.55 | <0.01 |
Active noncardiovascular issues | 0.86 | 0.80 | 0.72 | 0.75 | 0.74 | 0.12 | 0.83 | 0.83 | 0.76 | 0.52 | <0.01 |
In contrast, there were no significant differences in item agreement levels based on provider characteristics, including experience, handoff education, prehandoff preparation, or continuity (data not shown).
CONCLUSIONS
Our results provide initial evidence of reliability and validity of scores for a novel tool, the PKAT, designed to assess providers' shared clinical understanding of a pediatric CICU patient's condition and treatment plan. Because this information should be mutually understood following any handoff, we believe this tool or similar agreement assessments could be used to measure handoff quality across a range of clinical settings. Under the standardized conditions of video simulation, experienced CICU providers achieved high levels of agreement on the PKAT, demonstrating inter‐rater reliability. In situ testing results suggest that the PKAT can validly identify differences in understanding between providers for both routine and complex patients.
The achievement of 100% compliance with in situ testing demonstrates that this type of tool can feasibly be used in a real‐time clinical environment. As expected, mean agreement levels in situ were lower than levels achieved in video simulation. By item, mean levels of agreement for attending and clinician pairs were similar.
Our assessment of PKAT validity demonstrated mixed results. On the one hand, PKAT agreement did not vary significantly by any measured provider characteristics. Consistent with the lack of difference between attendings and clinicians, more experienced providers in both groups did not achieve higher levels of agreement. This finding is surprising, and may illustrate that unmeasured provider characteristics, such as content knowledge, obscure the effects of experience or other measured variables on agreement levels. Alternatively, providing the PKAT to the sending attending prior to the handoff, rather than afterward as for the receiving attendings and clinicians, might have artificially lowered attending agreement levels, concealing a difference due to experience.
On the other hand, construct validity of several items was supported by the difference in agreement levels based on patient characteristics. Agreement levels varied on 5/8 questions as patients became more complex, either defined by length of stay or number of medications. These differences show that agreement on PKAT items responds to changes in handoff complexity, a form of construct validity. Furthermore, these findings suggest that handoffs of more chronic or complex patients may require more attention for components prone to disagreement in these settings. Although complexity and longer length of stay are nonmodifiable risk factors, identifying these handoffs as more susceptible to disagreement provides potential targets for intervention.
It is important to move beyond he said/she said evaluations to assess shared understanding after a handoff, because high fidelity transfer of information is necessary for safe transfer of responsibility. The PKAT addresses this key component of handoff quality in a novel fashion. Although high‐fidelity information transfer may correlate with receiving provider satisfaction, this relationship has not yet been explored. Future studies will evaluate the association between receiver evaluations of handoffs and PKAT agreement, as well as the relationship between PKAT performance and subsequent patient outcomes.
Limitations of this approach include the challenges inherent in reducing a complex understanding of a patient to a multiple‐item instrument. Furthermore, PKAT use may influence handoff content due to the Hawthorne effect. Although our analysis rests on the argument that agreement is the goal of a handoff, some differences of opinion within the care team enrich resilience. Regardless, to maintain continuity of care, providers need to reach agreement on the next steps in a patient's care during the handoff. Because we focused only on agreement, this approach does not compare respondents' answers to a verifiable source of truth, if it exists. Therefore, 2 respondents who agree on the wrong answer receive the same score as 2 who agree on the right answer. Other limitations include using the number of medications as a marker of handoff complexity. Finally, conducting this study in a single CICU limits generalizability. However, we believe that all PKAT items are generalizable to other pediatric CICUs, and that several are generalizable to other pediatric intensive care settings. The approach of measuring shared understanding could be generalized more widely with development of items specific to different clinical settings.
Because the PKAT can be completed and scored quickly, it could be used as a real‐time measure of quality improvement interventions such as the introduction of a standardized handoff protocol. Alternatively, provider pairs could use the PKAT as a final handoff safety check to confirm consensus before transfer of responsibility. The concept of measuring shared clinical understanding could be extended to develop similar instruments for different clinical settings.
Acknowledgements
The authors thank the CICU providers for their enthusiasm for and participation in this study. The authors also thank Margaret Wolff, MD, Newton Buchanan, and the Center for Simulation, Advanced Education and Innovation at The Children's Hospital of Philadelphia for assistance in filming the video scenarios.
Disclosures: Dr. Bates was supported in part by NICHD/T32 HD060550 and NHLBI/T32 HL07915 grant funding. Dr. Metlay was supported by a Mid‐Career Investigator Award in Patient Oriented Research (K24‐AI073957). The authors report no conflicts of interest.
- The published literature on handoffs in hospitals: deficiencies identified in an extensive review. Quality and Safety in Health Care. 2010;19(6):493–497. doi: 10.1136/qshc.2009.033480. , .
- Communication failures in patient sign‐out and suggestions for improvement: a critical incident analysis. Qual Saf Health Care. 2005;14(6):401–407. .
- Consequences of inadequate sign‐out for patient care. Arch Intern Med. 2008;168(16):1755–1760. , , , , .
- Nursing handovers: do we really need them? J Nurs Manag. 2004;12(1):37–42. , , , , , .
- Assessing clinical handover between paramedics and the trauma team. Injury. 2010;41(5):460–464. , , , et al.
- Answering questions on call: Pediatric resident physicians' use of handoffs and other resources. J Hosp Med. 2013;8(6):328–333. , , , , .
- Managing discontinuity in academic medical centers: strategies for a safe and effective resident sign‐out. J Hosp Med. 2006;1(4):257–266. , , , , .
- Patient handoffs: standardized and reliable measurement tools remain elusive. Jt Comm J Qual Patient Saf. 2010;36(2):52–61. , .
- Improving measurement in clinical handover. Qual Saf Health Care. 2009;18(4):272–276. , , , , .
- Adequacy of information transferred at resident sign‐out (inhospital handover of care): a prospective survey. Qual Saf Health Care. 2008;17(1):6–10. , , , .
- Standardized Sign‐out reduces intern perception of medical errors on the general internal medicine ward. Teach Learn Med. 2009;21(2):121–126. , , .
- Hand‐off education and evaluation: piloting the observed simulated hand‐off experience (OSHE). J Gen Intern Med. 2009;25(2):129–134. , , , et al.
- Assessing the quality of patient handoffs at care transitions. Qual Saf Health Care. 2010;19(6):1–5. , , , , .
- Implementing peer evaluation of handoffs: associations with experience and workload. J Hosp Med. 2013;8(3):132–136. , , , , .
- Development of a handoff evaluation tool for shift‐to‐shift physician handoffs: the handoff CEX. J Hosp Med. 2013;8(4):191–200. , , , et al.
- The effects of patient handoff characteristics on subsequent care: a systematic review and areas for future research. Acad Med. 2012;87(8):1105–1124. , .
- Construct validity in organizational behavior. In: Cummings LL, Stawe BM, eds. Research in Organizational Behavior. Vol 2. Greenwich, CT: JAI Press; 1980:3–43. .
- Development and validation of the medication regimen complexity index. Ann Pharmacother. 2004;38(9):1369–1376. , , , , .
- A chronic disease score from automated pharmacy data. J Clin Epidemiol. 1992;45(2):197–203. , , .
- Research electronic data capture (REDCap)—a metadata‐driven methodology and workflow process for providing translational research informatics support. J Biomed Inform. 2009;42(2):377–381. , , , , , .
- A Wilcoxon‐type test for trend. Stat Med. 1985;4(1):87–90. .
- The published literature on handoffs in hospitals: deficiencies identified in an extensive review. Quality and Safety in Health Care. 2010;19(6):493–497. doi: 10.1136/qshc.2009.033480. , .
- Communication failures in patient sign‐out and suggestions for improvement: a critical incident analysis. Qual Saf Health Care. 2005;14(6):401–407. .
- Consequences of inadequate sign‐out for patient care. Arch Intern Med. 2008;168(16):1755–1760. , , , , .
- Nursing handovers: do we really need them? J Nurs Manag. 2004;12(1):37–42. , , , , , .
- Assessing clinical handover between paramedics and the trauma team. Injury. 2010;41(5):460–464. , , , et al.
- Answering questions on call: Pediatric resident physicians' use of handoffs and other resources. J Hosp Med. 2013;8(6):328–333. , , , , .
- Managing discontinuity in academic medical centers: strategies for a safe and effective resident sign‐out. J Hosp Med. 2006;1(4):257–266. , , , , .
- Patient handoffs: standardized and reliable measurement tools remain elusive. Jt Comm J Qual Patient Saf. 2010;36(2):52–61. , .
- Improving measurement in clinical handover. Qual Saf Health Care. 2009;18(4):272–276. , , , , .
- Adequacy of information transferred at resident sign‐out (inhospital handover of care): a prospective survey. Qual Saf Health Care. 2008;17(1):6–10. , , , .
- Standardized Sign‐out reduces intern perception of medical errors on the general internal medicine ward. Teach Learn Med. 2009;21(2):121–126. , , .
- Hand‐off education and evaluation: piloting the observed simulated hand‐off experience (OSHE). J Gen Intern Med. 2009;25(2):129–134. , , , et al.
- Assessing the quality of patient handoffs at care transitions. Qual Saf Health Care. 2010;19(6):1–5. , , , , .
- Implementing peer evaluation of handoffs: associations with experience and workload. J Hosp Med. 2013;8(3):132–136. , , , , .
- Development of a handoff evaluation tool for shift‐to‐shift physician handoffs: the handoff CEX. J Hosp Med. 2013;8(4):191–200. , , , et al.
- The effects of patient handoff characteristics on subsequent care: a systematic review and areas for future research. Acad Med. 2012;87(8):1105–1124. , .
- Construct validity in organizational behavior. In: Cummings LL, Stawe BM, eds. Research in Organizational Behavior. Vol 2. Greenwich, CT: JAI Press; 1980:3–43. .
- Development and validation of the medication regimen complexity index. Ann Pharmacother. 2004;38(9):1369–1376. , , , , .
- A chronic disease score from automated pharmacy data. J Clin Epidemiol. 1992;45(2):197–203. , , .
- Research electronic data capture (REDCap)—a metadata‐driven methodology and workflow process for providing translational research informatics support. J Biomed Inform. 2009;42(2):377–381. , , , , , .
- A Wilcoxon‐type test for trend. Stat Med. 1985;4(1):87–90. .
© 2014 Society of Hospital Medicine