User login
Incorporating an EWS Into Practice
Patients who deteriorate outside highly monitored settings and who require unplanned transfer to the intensive care unit (ICU) are known to have high mortality and morbidity.[1, 2, 3, 4, 5] The notion that early detection of a deteriorating patient improves outcomes has intuitive appeal and is discussed in a large number of publications.[6, 7, 8, 9, 10] However, much less information is available on what should be done after early detection is made.[11] Existing literature on early warning systems (EWSs) does not provide enough detail to serve as a map for implementation. This lack of transparency is complicated by the fact that, although the comprehensive inpatient electronic medical record (EMR) now constitutes the central locus for clinical practice, much of the existing literature comes from research institutions that may employ home‐grown EMRs, not community hospitals that employ commercially available systems.
In this issue of the Journal of Hospital Medicine, we describe our efforts to bridge that gap by implementing an EWS in a pair of community hospitals. The EWS's development and its basic statistical and electronic infrastructure are described in the articles by Escobar and Dellinger and Escobar et al.[2, 12, 13] In this report, we focus on how we addressed clinicians' primary concern: What do we do when we get an alert? Because it is described in detail by Granich et al.[14] elsewhere in this issue of the Journal of Hospital Medicine, a critical component of our implementation process (ensuring that patient preferences with respect to supportive care are honored) is not discussed.
Our article is divided into the following sections: rationale, preimplementation preparatory work, workflow development, response protocols, challenges and key learnings, and concluding reflections.
RATIONALE
Much of the previous work on the implementation of alarm systems has focused on the statistics behind detection or on the quantification of processes (eg, how many rapid response calls were triggered) or on outcomes such as mortality. The conceptual underpinnings and practical steps necessary for successful integration of an alarm system into the clinicians' workflow have not been articulated. Our theoretical framework was based on (1) improving situational awareness[15] (knowing what is going on around you and what is likely to happen next) and (2) mitigating cognitive errors.
An EWS enhances situational awareness most directly by earlier identification of a problem with a particular patient. As is detailed by Escobar et al.[16] in this issue of the Journal of Hospital Medicine, our EWS extracts EMR data every 6 hours, performs multiple calculations, and then displays 3 scores in real time in the inpatient dashboard (known as the Patient Lists activity in the Epic EMR). The first of these scores is the Laboratory‐Based Acute Physiologic Score, version 2 (LAPS2), an objective severity score whose retrospective version is already in use in Kaiser Permanente Northern California (KPNC) for internal benchmarking.[13] This score captures a patient's overall degree of physiologic instability within the preceding 72 hours. The second is the Comorbidity Point Score, version 2 (COPS2), a longitudinal comorbidity score based on the patient's diagnoses over the preceding 12 months.[13] This score captures a patient's overall comorbidity burden. Thus, it is possible for a patient to be very ill (high COPS2) while also being stable (low LAPS2) or vice versa. Both of these scores have other uses, including prediction of rehospitalization risk in real time,[17] which is also being piloted at KPNC. Finally, the Advanced Alert Monitoring (AAM) score, which integrates the LAPS2 and COPS2 with other variables, provides a 12‐hour deterioration risk, with a threshold value of 8% triggering response protocols. At or above this threshold, which was agreed to prior to implementation, the system achieves 25% sensitivity, 98% specificity, with a number needed to evaluate of 10 to 12, a level of workload that was felt to be acceptable by clinicians. Actions triggered by the EWS may be quite different from those one would take when being notified of a code blue, which is called at the time an event occurs. The EWS focuses attention on patients who might be missed because they do not yet appear critically ill. It also provides a shared, quantifiable measure of a patient's risk that can trigger a standardized plan of action to follow in evaluating and treating a patient.[15]
In addition to enhancing situational awareness, we intended the alarms to produce cognitive change in practitioners. Our goal was to replace medical intuition with analytic, evidence‐based judgment of future illness. We proceeded with the understanding that replacing quick intuition with slower analytic response is an essential skill in developing sound clinical reasoning.[18, 19, 20] The alert encourages physicians to reassess high‐risk patients facilitating a cognitive shift from automatic, error‐prone processing to slower, deliberate processing. Given the busy pace of ward work, slowing down permits clinicians to reassess previously overlooked details. Related to this process of inducing cognitive change is a secondary effect: we uncovered and discussed physician biases. Physicians are subject to potential biases that allow patients to deteriorate.[18, 19, 20] Therefore, we addressed bias through education. By reviewing particular cases of unanticipated deterioration at each hospital facility, we provided evidence for the problem of in‐hospital deterioration. This framed the new tool as an opportunity for improving treatment and encouraged physicians to act on the alert using a structured process.
INTERVENTIONS
Preimplementation Preparatory Work
Initial KPNC data provided strong support for the generally accepted notion that unplanned transfer patients have poor outcomes.[2, 4, 5] However, published reports failed to provide the granular detail clinicians need to implement a response arm at the unit and patient level. In preparation for going live, we conducted a retrospective chart review. This included data from patients hospitalized from January 1, 2011 through December 31, 2012 (additional detail is provided in the Supporting Information, Appendix, in the online version of this article). The key findings from our internal review of subjective documentation preceding deterioration are similar to those described in the literature and summarized in Figure 1, which displays the 5 most common clinical presentations associated with unplanned transfers.
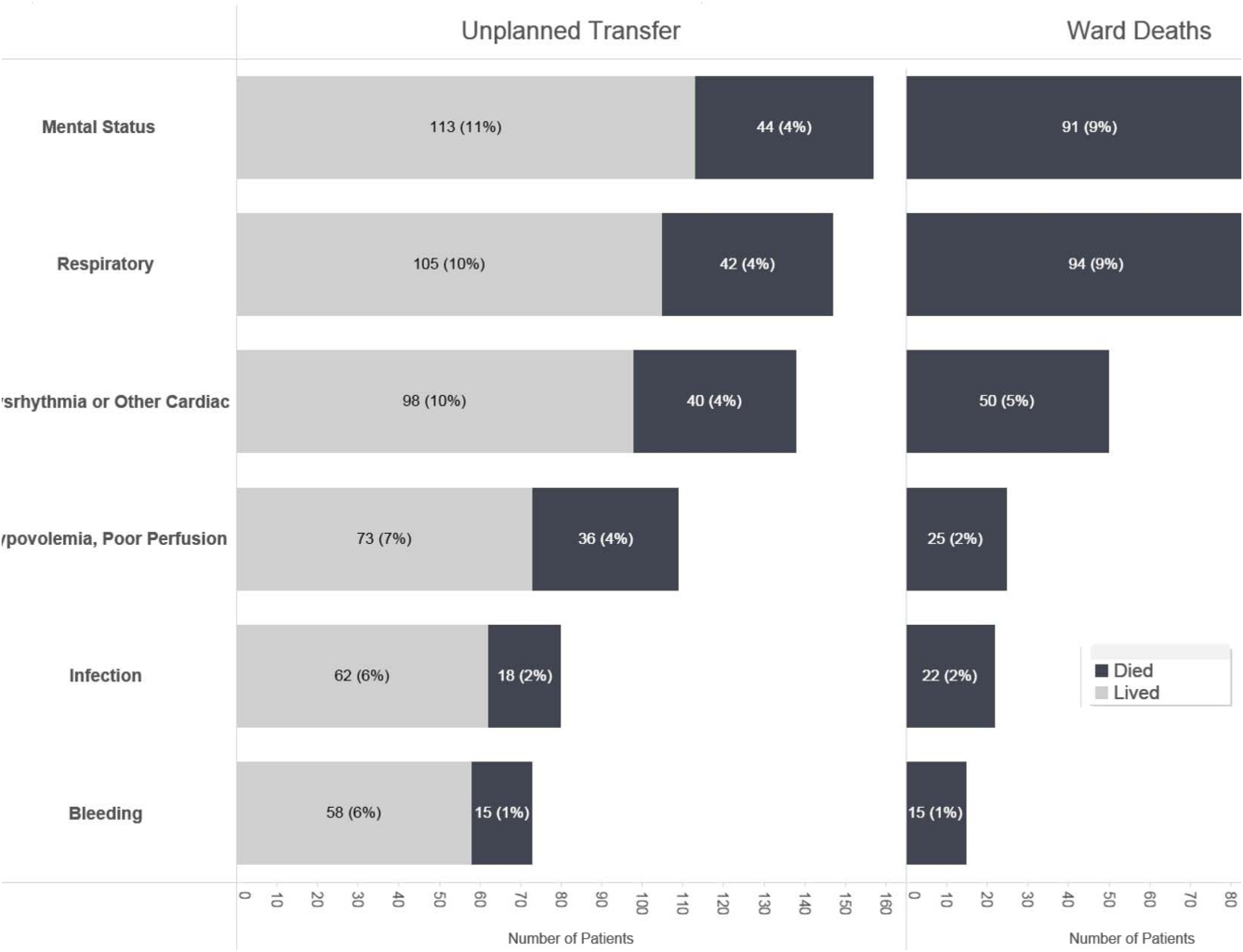
The chart review served several major roles. First, it facilitated cognitive change by eliminating the notion that it can't happen here. Second, it provided considerable guidance on key clinical components that had to be incorporated into the workflow. Third, it engaged the rapid response team (RRT) in reviewing our work retrospectively to identify future opportunities. Finally, the review provided considerable guidance with respect to structuring documentation requirements.
As a result of the above efforts, other processes detailed below, and knowledge described in several of the companion articles in this issue of the Journal of Hospital Medicine, 3 critical elements, which had been explicitly required by our leadership, were in place prior to the go‐live date: a general consensus among hospitalists and nurses that this would be worth testing, a basic clinical response workflow, and an automated checklist for documentation. We refined these in a 2‐week shadowing phase preceding the start date. In this phase, the alerts were not displayed in the EMR. Instead, programmers working on the project notified selected physician leaders by phone. This permitted them to understand exactly what sort of patients were reaching the physiologic threshold so that they could better prepare both RRT registered nurses (RNs) and hospitalists for the go‐live date. This also provided an opportunity to begin refining the documentation process using actual patients.
The original name for our project was Early Detection of Impending Physiologic Deterioration. However, during the preparatory phase, consultation with our public relations staff led to a concern that the name could be frightening to some patients. This highlights the need to consider patient perceptions and how words used in 1 way by physicians can have different connotations to nonclinicians. Consequently, the system was renamed, and it is now referred to as Advance Alert Monitoring (AAM).
Workflow Development
We carefully examined the space where electronic data, graphical user interfaces, and clinical practice blend, a nexus now commonly referred to as workflow or user experience.[21] To promote situational awareness and effect cognitive change, we utilized the Institute for Health Care Improvement's Plan‐Do‐Study‐Act model.[22, 23] We then facilitated the iterative development of a clinician‐endorsed workflow.[22, 23, 24, 25] By adjusting the workflow based on ongoing experience and giving clinicians multiple opportunities to revise (a process that continues to date), we ensured clinicians would approach and endorse the alarm system as a useful tool for decision support.
Table 1 summarizes the work groups assembled for our implementation, and Table 2 provides a system‐oriented checklist indicating key components that need to be in place prior to having an early warning system go live in a hospital. Figure 2 summarizes the alert response protocols we developed through an iterative process at the 2 pilot sites. The care path shown in Figure 2 is the result of considerable revision, mostly due to actual experience acquired following the go live date. The diagram also includes a component that is still work in progress. This is how an emergency department probability estimate (triage support) will be integrated into both the ward as well as the ICU workflows. Although this is beyond the scope of this article, other hospitals may be experimenting with triage support (eg, for sepsis patients), so it is important to consider how one would incorporate such support into workflows.
Workgroup | Goals |
---|---|
| |
Clinical checklist | Perform structured chart review of selected unplanned transfer patients and near misses |
Develop a checklist for mitigation strategies given an alert | |
Develop documentation standards given an alert | |
Develop escalation protocol given an alert | |
Workload and threshold | Determine threshold for sensitivity of alerts and resulting impact on clinician workload |
Patient preferences | Prepare background information to be presented to providers regarding end‐of‐life care and POLST orders |
Coordinate with clinical checklist workgroup to generate documentation templates that provide guidance for appropriate management of patients regarding preferences on escalation of care and end‐of‐life care | |
Electronic medical record coordination | Review proposed electronic medical record changes |
Make recommendation for further changes as needed | |
Develop plan for rollout of new and/or revised electronic record tools | |
Designate contact list for questions/emssues that may arise regarding electronic record changes during the pilot | |
Determine alert display choices and mode of alert notification | |
Nursing committee | Review staffing needs in anticipation of alert |
Coordinate with workload and threshold group | |
Develop training calendar to ensure skills necessary for successful implementation of alerts | |
Make recommendations for potential modification of rapid response team's role in development of a clinical checklist for nurses responding to an alert | |
Design educational materials for clinicians | |
Local communication strategy | Develop internal communication plan (for clinical staff not directly involved with pilot) |
Develop external communication plan (for nonclinicians who may hear about the project) |
Level | Tasks |
---|---|
Administration | Obtain executive committee approval |
Establish communication protocols with quality assurance and quality improvement committees | |
Review protocols with medicallegal department | |
Communication | Write media material for patients and families |
Develop and disseminate scripts for front‐line staff | |
Develop communication and meet with all relevant front‐line staff on merits of project | |
Educate all staff on workflow changes and impacts | |
Clinical preparation | Conduct internal review of unplanned transfers and present results to all clinicians |
Determine service level agreements, ownership of at‐risk patients, who will access alerts | |
Conduct staff meetings to educate staff | |
Perform debriefs on relevant cases | |
Determine desired outcomes, process measures, balancing measures | |
Determine acceptable clinician burden (alerts/day) | |
Technology | Establish documentation templates |
Ensure access to new data fields (electronic medical record security process must be followed for access rights) | |
Workflows | Workflows (clinical response, patient preferences, supportive care, communication, documentation) must be in place prior to actual go live |
Shadowing | Testing period (alerts communicated to selected clinicians prior to going live) should occur |
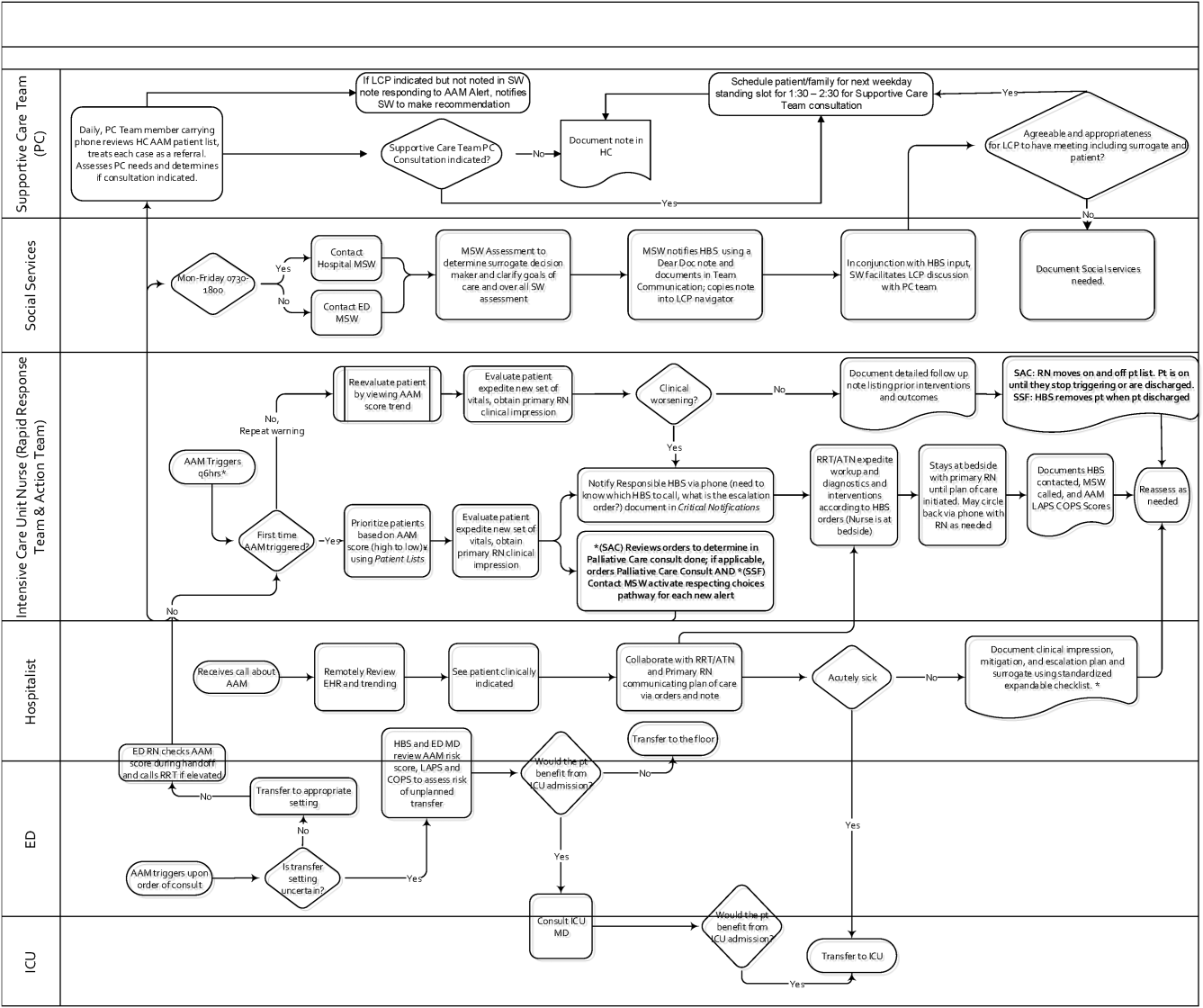
RESPONSE PROTOCOLS
At South San Francisco, the RRT consists of an ICU nurse, a respiratory care therapist, and a designated hospitalist; at Sacramento, the team is also augmented by an additional nurse (the house supervisor). In addition to responding to the AAM alerts, RRT nurses respond to other emergency calls such as code blues, stroke alerts, and patient or patient‐familyinitiated rapid response calls. They also expedite time sensitive workups and treatments. They check up on recent transfers from the ICU to ensure continued improvement justifying staying on the ward. Serving as peer educators, they assist with processes such as chest tube or central line insertions, troubleshoot high‐risk medication administration, and ensure that treatment bundles (eg, for sepsis) occur expeditiously.
The RRT reviews EWS scores every 6 hours. The AAM score is seen as soon as providers open the chart, which helps triage patients for evaluation. Because patients can still be at risk even without an elevated AAM score, all normal escalation pathways remain in place. Once an alert is noted in the inpatient dashboard, the RRT nurse obtains a fresh set of vital signs, assesses the patient's clinical status, and informs the physician, social worker, and primary nurse (Figure 2). Team members work with the bedside nurse, providing support with assessment, interventions, plans, and follow‐up. Once advised of the alert, the hospitalist performs a second chart review and evaluates the patient at the bedside to identify factors that could underlie potential deterioration. After this evaluation, the hospitalist documents concerns, orders appropriate interventions (which can include escalation), and determines appropriate follow‐up. We made sure the team knew that respiratory distress, arrhythmias, mental status changes, or worsening infection were responsible for over 80% of in‐hospital deterioration cases. We also involved palliative care earlier in patient care, streamlining the process so the RRT makes just 1 phone call to the social worker, who contacts the palliative care physician and nurse to ensure patients have a designated surrogate in the event of further deterioration.
Our initial documentation template consisted of a comprehensive organ system‐based physician checklist. However, although this was of use to covering physicians unfamiliar with a given patient, it was redundant and annoying to attending providers already familiar with the patient. After more than 30 iterations, we settled on a succinct note that only documented the clinicians' clinical judgment as to what constituted the major risk for deterioration and what the mitigation strategies would be. Both of these judgments are in a checklist format (see Supporting Information, Appendix, in the online version of this article for the components of the physician and nurse notes).
Prior to the implementation of the system, RRT nurses performed proactive rounding by manually checking patient labs and vital signs, an inefficient process due to the poor sensitivity and specificity of individual values. Following implementation of the system, RRT RNs and clinicians switched to sorting patients by the 3 scores (COPS2, LAPS2, AAM). For example, patients may be stable at admission (as evidenced by their AAM score) but be at high risk due to their comorbidities. One approach that has been employed is to proactively check such patients to ensure they have a care directive in place, as is described in the article by Granich et al.[14] The Supportive Care Team (detailed in Granich et al.) assesses needs for palliative care and provides in‐hospital consultation as needed. Social services staff perform chart reviews to ensure a patient surrogate has been defined and also works with patients and their families to clarify goals of care.
CHALLENGES AND KEY LEARNINGS
One challenge that arose was reconciling the periodic nature of the alert (every 6 hours) with physicians' availability, which varied due to different rounding workflows at the 2 sites. Consequently, the alert cycle was changed; at the first site, the cycle was set to 1000‐1600‐2200‐0400, whereas the second site chose 0800‐1400‐2000‐0200.
One essential but problematic component of the clinical response is the issue of documentation. Inadequate documentation could lead to adverse outcomes, clinician malpractice exposure, and placing the entire hospital at risk for enterprise liability when clinical responses are not documented. This issue is complicated by the fact that overzealous efforts could lead to less or no documentation by making it too onerous for busy clinicians. We found that the ease with which data can populate progress notes in the EMR can lead to note bloat. Clearly, no documentation is not enough, and a complete history and physical is too much. Paradoxically, 1 of the issues underlying our problems with documentation was the proactive nature of the alerts themselves; because they are based on an outcome prediction in the next 12 hours, documenting the response to them may lack (perceived) urgency.
Shortly after the system went live, a patient who had been recently transferred out to the ward from the ICU triggered an alert. As a response was mounted, the team realized that existing ward protocols did not specify which physician service (intensivist or hospitalist) was responsible for patients who were transitioning from 1 unit to another. We also had to perform multiple revisions of the protocols specifying how alerts were handled when they occurred at times of change of shift. Eventually, we settled on having the combination of a hospitalist and an RRT nurse as the cornerstone of the response, with the hospitalist service as the primary owner of the entire process, but this arrangement might need to be varied in different settings. As a result of the experience with the pilot, the business case for deployment in the remaining 19 hospitals includes a formal budget request so that all have properly staffed RRTs, although the issue of primary ownership of the alert process for different patient types (eg, surgical patients) will be decided on a hospital‐by‐hospital basis. These experiences raise the intriguing possibility that implementation of alert systems can lead to the identification of systemic gaps in existing protocols. These gaps can include specific components of the hospital service agreements between multiple departments (emergency, hospital medicine, ICU, palliative care, surgery) as well as problems with existing workflows.
In addition to ongoing tweaking of care protocols, 3 issues remain unresolved. First is the issue of documentation. The current documentation notes are not completely satisfactory, and we are working with the KPNC EMR administrators to refine the tool. Desirable refinements include (1) having the system scores populate in more accessible sectors of the EMR where their retrieval will facilitate increased automation of the note writing process, (2) changing the note type to a note that will facilitate process audits, and (3) linking the note to other EMR tools so that the response arm can be tracked more formally. The second issue is the need to develop strategies to address staff turnover; for example, newer staff may not have received the same degree of exposure to the system as those who were there when it was started. Finally, due to limited resources, we have done very limited work on more mechanistic analyses of the clinical response itself. For example, it would be desirable to perform a formal quantitative, risk‐adjusted process‐outcome analysis of why some patients' outcomes are better than others following an alert.
Finally, it is also the case that we have had some unexpected occurrences that hint at new uses and benefits of alert systems. One of these is the phenomenon of chasing the alert. Some clinicians, on their own, have taken a more proactive stance in the care of patients in whom the AAM score is rising or near the alert threshold. This has 2 potential consequences. Some patients are stabilized and thus do not reach threshold instability levels. In other cases, patients reach threshold but the response team is informed that things are already under control. A second unexpected result is increased requests for COPS2 scores by clinicians who have heard about the system, particularly surgeons who would like to use the comorbidity scores as a screening tool in the outpatient setting. Because KPNC is an integrated system, it is not likely that such alternatives will be implemented immediately without considerable analysis, but it is clear that the system's deployment has captured the clinicians' imagination.
CONCLUSIONS AND FUTURE DIRECTIONS
Our preparatory efforts have been successful. We have found that embedding an EWS in a commercially available EMR is acceptable to hospital physicians and nurses. We have developed a coordinated workflow for mitigation and escalation that is tightly linked to the availability of probabilistic alerts in real time. Although resource limitations have precluded us from conducting formal clinician surveys, the EWS has been discussed at multiple hospital‐wide as well as department‐specific meetings. Although there have been requests for clarification, refinements, and modifications in workflows, no one has suggested that the system be discontinued. Further, many of the other KPNC hospitals have requested that the EWS be deployed at their site. We have examined KPNC databases that track patient complaints and have not found any complaints that could be linked to the EWS. Most importantly, the existence of the workflows we have developed has played a major role in KPNC's decision to deploy the system in its remaining hospitals.
Although alert fatigue is the number 1 reason that clinicians do not utilize embedded clinical decision support,[26] simply calibrating statistical models is insufficient. Careful consideration of clinicians' needs and responsibilities, particularly around ownership of patients and documentation, is essential. Such consideration needs to include planning time and socializing the system (providing multiple venues for clinicians to learn about the system as well as participate in the process for using it).
We anticipate that, as the system leaves the pilot stage and becomes a routine component of hospital care, additional enhancements (eg, sending notifications to smart phones, providing an alert response tracking system) will be added. Our organization is also implementing real‐time concurrent review of inpatient EMRs (eg, for proactive detection of an expanded range of potential process failures), and work is underway on how to link the workflows we describe here with this effort. As has been the case with other systems,[27] it is likely that we will eventually move to continuous scanning of patient data rather than only every 6 hours. Given that the basic workflow is quite robust and amenable to local modifications, we are confident that our clinicians and hospitals will adapt to future system enhancements.
Lastly, we intend to conduct additional research on the clinical response itself. In particular, we consider it extremely important to conduct formal quantitative analyses on why some patients' outcomes are better than others following an alert. A key component of this effort will be to develop tools that can permit an automatedor nearly automatedassessment of the clinical response. For example, we are considering automated approaches that would scan the EMR for the presence of specific orders, notes, vital signs patterns, and laboratory tests following an alert. Whereas it may not be possible to dispense with manual chart review, even partial automation of a feedback process could lead to significant enhancement of our quality improvement efforts.
Acknowledgements
The authors thank Dr. Michelle Caughey, Dr. Philip Madvig, Dr. Brian Hoberman, Dr. Patricia Conolly, and Ms. Barbara Crawford for their administrative support; Dr. Tracy Lieu for reviewing the manuscript; and Ms. Rachel Lesser for formatting the manuscript. The authors also thank Drs. Jason Anderson, John Fitzgibbon, Elena M. Nishimura, and Najm Haq for their support of the project. We are particularly grateful to our nurses, Theresa A. Villorente, Zoe Sutton, Doanh Ly, Catherine Burger, and Hillary R. Mitchell, for their critical assistance. Last but not least, we also thank all the hospitalists and nurses at the Kaiser Permanente Sacramento and South San Francisco hospitals.
Disclosures: This work was supported by a grant from the Gordon and Betty Moore Foundation (Early Detection, Prevention, and Mitigation of Impending Physiologic Deterioration in Hospitalized Patients Outside Intensive Care: Phase 3, pilot), The Permanente Medical Group, Inc., and Kaiser Foundation Hospitals, Inc. Dr. Liu was supported by the National Institute for General Medical Sciences award K23GM112018. As part of our agreement with the Moore Foundation, we made a commitment to disseminate our findings in articles such as this one. However, the Gordon and Betty Moore Foundation played no role in how we actually structured our articles, nor did they review or preapprove any of the manuscripts submitted as part of the dissemination component; the same was the case with the other sponsors. None of the authors has any conflicts of interest to declare of relevance to this work
- Location of patients before transfer to a tertiary care intensive care unit: impact on outcome. J Crit Care. 2009;24(1):108–113. , , , , .
- Intra‐hospital transfers to a higher level of care: contribution to total hospital and intensive care unit (ICU) mortality and length of stay (LOS). J Hosp Med. 2011;6(2):74–80. , , , , , .
- Unplanned transfers to a medical intensive care unit: causes and relationship to preventable errors in care. J Hosp Med. 2011;6(2):68–72. , , , .
- Adverse outcomes associated with delayed intensive care unit transfers in an integrated healthcare system. J Hosp Med. 2012;7(3):224–230. , , , .
- Risk factors for unplanned transfer to intensive care within 24 hours of admission from the emergency department in an integrated healthcare system. J Hosp Med. 2013;8(1):13–19. , , , , , .
- Reducing hospital standardized mortality rate with early interventions. J Trauma Nursing. 2006;13(4):178–182. , , , , , .
- Centile‐based early warning scores derived from statistical distributions of vital signs. Resuscitation. 2011;82(8):1013–1018. , , , , , .
- Randomized trial of automated, electronic monitoring to facilitate early detection of sepsis in the intensive care unit. Crit Care Med. 2012;40(7):2096–2101. , , , et al.
- Early recognition of acutely deteriorating patients in non‐intensive care units: assessment of an innovative monitoring technology. J Hosp Med. 2012;7(8):628–633. , , , et al.
- A randomized trial of real‐time automated clinical deterioration alerts sent to a rapid response team. J Hosp Med. 2014;9(7):424–429. , , , et al.
- Improving situation awareness to reduce unrecognized clinical deterioration and serious safety events. Pediatrics. 2013;131(1):e298–e308. , , , et al.
- Early detection of impending physiologic deterioration among patients who are not in intensive care: development of predictive models using data from an automated electronic medical record. J Hosp Med. 2012;7(5):388–395. , , , , , .
- Risk‐adjusting hospital mortality using a comprehensive electronic record in an integrated healthcare delivery system. Med Care. 2013;51(5):446–453. , , , , .
- Early detection of critical illness outside the intensive care unit: clarifying treatment plans and honoring goals of care using a supportive care team. J Hosp Med. 2016;11:000–000. , , . et al.
- A qualitative study examining the influences on situation awareness and the identification, mitigation and escalation of recognised patient risk. BMJ Qual Saf. 2014;23(2):153–161. , .
- Piloting electronic medical record‐based early detection of inpatient deterioration in community hospitals. J Hosp Med. 2016;11:000–000. , , , et al.
- Nonelective rehospitalizations and postdischarge mortality: predictive models suitable for use in real time. Med Care. 2015;53(11):916–923. , , , , , .
- The importance of cognitive errors in diagnosis and strategies to minimize them. Acad Med. 2003;78(8):775–780. .
- Cognitive debiasing 1: origins of bias and theory of debiasing. BMJ Qual Saf. 2013;22(suppl 2):ii58–ii64. , , .
- Cognitive debiasing 2: impediments to and strategies for change. BMJ Qual Saf. 2013;22(suppl 2):ii65–ii72. , , .
- Use of health information technology to reduce diagnostic errors. BMJ Qual Saf. 2013;22(suppl 2):ii40–ii51. , , .
- The Improvement Guide: A Practical Approach to Enhancing Organizational Performance. 2nd ed. San Francisco, CA: Jossey‐Bass; 2009. , , , , , .
- Understanding the components of quality improvement collaboratives: a systematic literature review. Milbank Q. 2013;91(2):354–394. , , , , .
- Reducing diagnostic errors in medicine: what's the goal? Acad Med. 2002;77(10):981–992. , , .
- Huddling for high reliability and situation awareness. BMJ Qual Saf. 2013;22(11):899–906. , , , .
- Top 10 patient safety concerns for healthcare organizations. ECRI Institute website. Available at: https://www.ecri.org/Pages/Top‐10‐Patient‐Safety‐Concerns.aspx. Accessed February 18, 2016.
- Automated detection of physiologic deterioration in hospitalized patients. J Am Med Inform Assoc. 2015;22(2):350–360. , , , et al.
Patients who deteriorate outside highly monitored settings and who require unplanned transfer to the intensive care unit (ICU) are known to have high mortality and morbidity.[1, 2, 3, 4, 5] The notion that early detection of a deteriorating patient improves outcomes has intuitive appeal and is discussed in a large number of publications.[6, 7, 8, 9, 10] However, much less information is available on what should be done after early detection is made.[11] Existing literature on early warning systems (EWSs) does not provide enough detail to serve as a map for implementation. This lack of transparency is complicated by the fact that, although the comprehensive inpatient electronic medical record (EMR) now constitutes the central locus for clinical practice, much of the existing literature comes from research institutions that may employ home‐grown EMRs, not community hospitals that employ commercially available systems.
In this issue of the Journal of Hospital Medicine, we describe our efforts to bridge that gap by implementing an EWS in a pair of community hospitals. The EWS's development and its basic statistical and electronic infrastructure are described in the articles by Escobar and Dellinger and Escobar et al.[2, 12, 13] In this report, we focus on how we addressed clinicians' primary concern: What do we do when we get an alert? Because it is described in detail by Granich et al.[14] elsewhere in this issue of the Journal of Hospital Medicine, a critical component of our implementation process (ensuring that patient preferences with respect to supportive care are honored) is not discussed.
Our article is divided into the following sections: rationale, preimplementation preparatory work, workflow development, response protocols, challenges and key learnings, and concluding reflections.
RATIONALE
Much of the previous work on the implementation of alarm systems has focused on the statistics behind detection or on the quantification of processes (eg, how many rapid response calls were triggered) or on outcomes such as mortality. The conceptual underpinnings and practical steps necessary for successful integration of an alarm system into the clinicians' workflow have not been articulated. Our theoretical framework was based on (1) improving situational awareness[15] (knowing what is going on around you and what is likely to happen next) and (2) mitigating cognitive errors.
An EWS enhances situational awareness most directly by earlier identification of a problem with a particular patient. As is detailed by Escobar et al.[16] in this issue of the Journal of Hospital Medicine, our EWS extracts EMR data every 6 hours, performs multiple calculations, and then displays 3 scores in real time in the inpatient dashboard (known as the Patient Lists activity in the Epic EMR). The first of these scores is the Laboratory‐Based Acute Physiologic Score, version 2 (LAPS2), an objective severity score whose retrospective version is already in use in Kaiser Permanente Northern California (KPNC) for internal benchmarking.[13] This score captures a patient's overall degree of physiologic instability within the preceding 72 hours. The second is the Comorbidity Point Score, version 2 (COPS2), a longitudinal comorbidity score based on the patient's diagnoses over the preceding 12 months.[13] This score captures a patient's overall comorbidity burden. Thus, it is possible for a patient to be very ill (high COPS2) while also being stable (low LAPS2) or vice versa. Both of these scores have other uses, including prediction of rehospitalization risk in real time,[17] which is also being piloted at KPNC. Finally, the Advanced Alert Monitoring (AAM) score, which integrates the LAPS2 and COPS2 with other variables, provides a 12‐hour deterioration risk, with a threshold value of 8% triggering response protocols. At or above this threshold, which was agreed to prior to implementation, the system achieves 25% sensitivity, 98% specificity, with a number needed to evaluate of 10 to 12, a level of workload that was felt to be acceptable by clinicians. Actions triggered by the EWS may be quite different from those one would take when being notified of a code blue, which is called at the time an event occurs. The EWS focuses attention on patients who might be missed because they do not yet appear critically ill. It also provides a shared, quantifiable measure of a patient's risk that can trigger a standardized plan of action to follow in evaluating and treating a patient.[15]
In addition to enhancing situational awareness, we intended the alarms to produce cognitive change in practitioners. Our goal was to replace medical intuition with analytic, evidence‐based judgment of future illness. We proceeded with the understanding that replacing quick intuition with slower analytic response is an essential skill in developing sound clinical reasoning.[18, 19, 20] The alert encourages physicians to reassess high‐risk patients facilitating a cognitive shift from automatic, error‐prone processing to slower, deliberate processing. Given the busy pace of ward work, slowing down permits clinicians to reassess previously overlooked details. Related to this process of inducing cognitive change is a secondary effect: we uncovered and discussed physician biases. Physicians are subject to potential biases that allow patients to deteriorate.[18, 19, 20] Therefore, we addressed bias through education. By reviewing particular cases of unanticipated deterioration at each hospital facility, we provided evidence for the problem of in‐hospital deterioration. This framed the new tool as an opportunity for improving treatment and encouraged physicians to act on the alert using a structured process.
INTERVENTIONS
Preimplementation Preparatory Work
Initial KPNC data provided strong support for the generally accepted notion that unplanned transfer patients have poor outcomes.[2, 4, 5] However, published reports failed to provide the granular detail clinicians need to implement a response arm at the unit and patient level. In preparation for going live, we conducted a retrospective chart review. This included data from patients hospitalized from January 1, 2011 through December 31, 2012 (additional detail is provided in the Supporting Information, Appendix, in the online version of this article). The key findings from our internal review of subjective documentation preceding deterioration are similar to those described in the literature and summarized in Figure 1, which displays the 5 most common clinical presentations associated with unplanned transfers.
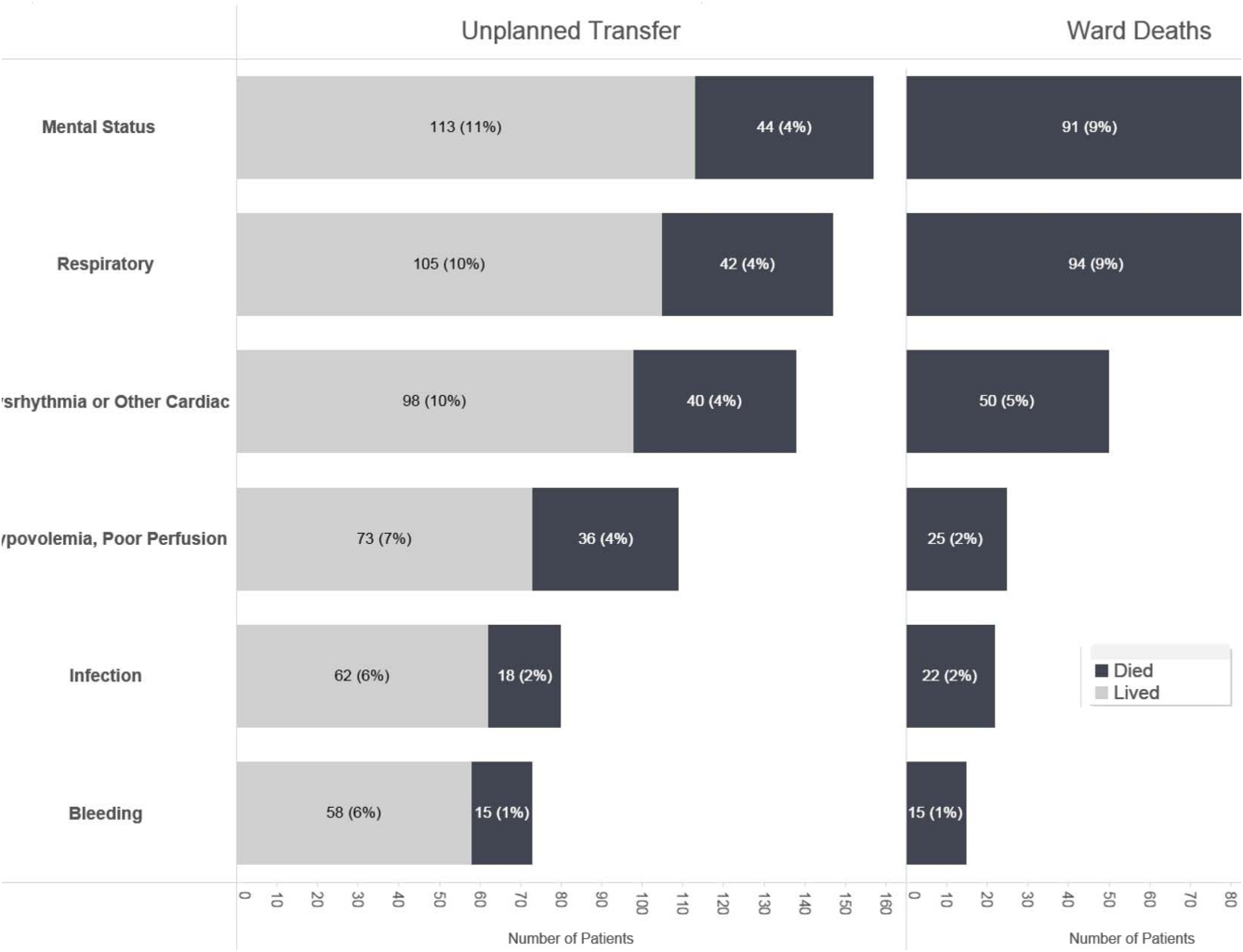
The chart review served several major roles. First, it facilitated cognitive change by eliminating the notion that it can't happen here. Second, it provided considerable guidance on key clinical components that had to be incorporated into the workflow. Third, it engaged the rapid response team (RRT) in reviewing our work retrospectively to identify future opportunities. Finally, the review provided considerable guidance with respect to structuring documentation requirements.
As a result of the above efforts, other processes detailed below, and knowledge described in several of the companion articles in this issue of the Journal of Hospital Medicine, 3 critical elements, which had been explicitly required by our leadership, were in place prior to the go‐live date: a general consensus among hospitalists and nurses that this would be worth testing, a basic clinical response workflow, and an automated checklist for documentation. We refined these in a 2‐week shadowing phase preceding the start date. In this phase, the alerts were not displayed in the EMR. Instead, programmers working on the project notified selected physician leaders by phone. This permitted them to understand exactly what sort of patients were reaching the physiologic threshold so that they could better prepare both RRT registered nurses (RNs) and hospitalists for the go‐live date. This also provided an opportunity to begin refining the documentation process using actual patients.
The original name for our project was Early Detection of Impending Physiologic Deterioration. However, during the preparatory phase, consultation with our public relations staff led to a concern that the name could be frightening to some patients. This highlights the need to consider patient perceptions and how words used in 1 way by physicians can have different connotations to nonclinicians. Consequently, the system was renamed, and it is now referred to as Advance Alert Monitoring (AAM).
Workflow Development
We carefully examined the space where electronic data, graphical user interfaces, and clinical practice blend, a nexus now commonly referred to as workflow or user experience.[21] To promote situational awareness and effect cognitive change, we utilized the Institute for Health Care Improvement's Plan‐Do‐Study‐Act model.[22, 23] We then facilitated the iterative development of a clinician‐endorsed workflow.[22, 23, 24, 25] By adjusting the workflow based on ongoing experience and giving clinicians multiple opportunities to revise (a process that continues to date), we ensured clinicians would approach and endorse the alarm system as a useful tool for decision support.
Table 1 summarizes the work groups assembled for our implementation, and Table 2 provides a system‐oriented checklist indicating key components that need to be in place prior to having an early warning system go live in a hospital. Figure 2 summarizes the alert response protocols we developed through an iterative process at the 2 pilot sites. The care path shown in Figure 2 is the result of considerable revision, mostly due to actual experience acquired following the go live date. The diagram also includes a component that is still work in progress. This is how an emergency department probability estimate (triage support) will be integrated into both the ward as well as the ICU workflows. Although this is beyond the scope of this article, other hospitals may be experimenting with triage support (eg, for sepsis patients), so it is important to consider how one would incorporate such support into workflows.
Workgroup | Goals |
---|---|
| |
Clinical checklist | Perform structured chart review of selected unplanned transfer patients and near misses |
Develop a checklist for mitigation strategies given an alert | |
Develop documentation standards given an alert | |
Develop escalation protocol given an alert | |
Workload and threshold | Determine threshold for sensitivity of alerts and resulting impact on clinician workload |
Patient preferences | Prepare background information to be presented to providers regarding end‐of‐life care and POLST orders |
Coordinate with clinical checklist workgroup to generate documentation templates that provide guidance for appropriate management of patients regarding preferences on escalation of care and end‐of‐life care | |
Electronic medical record coordination | Review proposed electronic medical record changes |
Make recommendation for further changes as needed | |
Develop plan for rollout of new and/or revised electronic record tools | |
Designate contact list for questions/emssues that may arise regarding electronic record changes during the pilot | |
Determine alert display choices and mode of alert notification | |
Nursing committee | Review staffing needs in anticipation of alert |
Coordinate with workload and threshold group | |
Develop training calendar to ensure skills necessary for successful implementation of alerts | |
Make recommendations for potential modification of rapid response team's role in development of a clinical checklist for nurses responding to an alert | |
Design educational materials for clinicians | |
Local communication strategy | Develop internal communication plan (for clinical staff not directly involved with pilot) |
Develop external communication plan (for nonclinicians who may hear about the project) |
Level | Tasks |
---|---|
Administration | Obtain executive committee approval |
Establish communication protocols with quality assurance and quality improvement committees | |
Review protocols with medicallegal department | |
Communication | Write media material for patients and families |
Develop and disseminate scripts for front‐line staff | |
Develop communication and meet with all relevant front‐line staff on merits of project | |
Educate all staff on workflow changes and impacts | |
Clinical preparation | Conduct internal review of unplanned transfers and present results to all clinicians |
Determine service level agreements, ownership of at‐risk patients, who will access alerts | |
Conduct staff meetings to educate staff | |
Perform debriefs on relevant cases | |
Determine desired outcomes, process measures, balancing measures | |
Determine acceptable clinician burden (alerts/day) | |
Technology | Establish documentation templates |
Ensure access to new data fields (electronic medical record security process must be followed for access rights) | |
Workflows | Workflows (clinical response, patient preferences, supportive care, communication, documentation) must be in place prior to actual go live |
Shadowing | Testing period (alerts communicated to selected clinicians prior to going live) should occur |
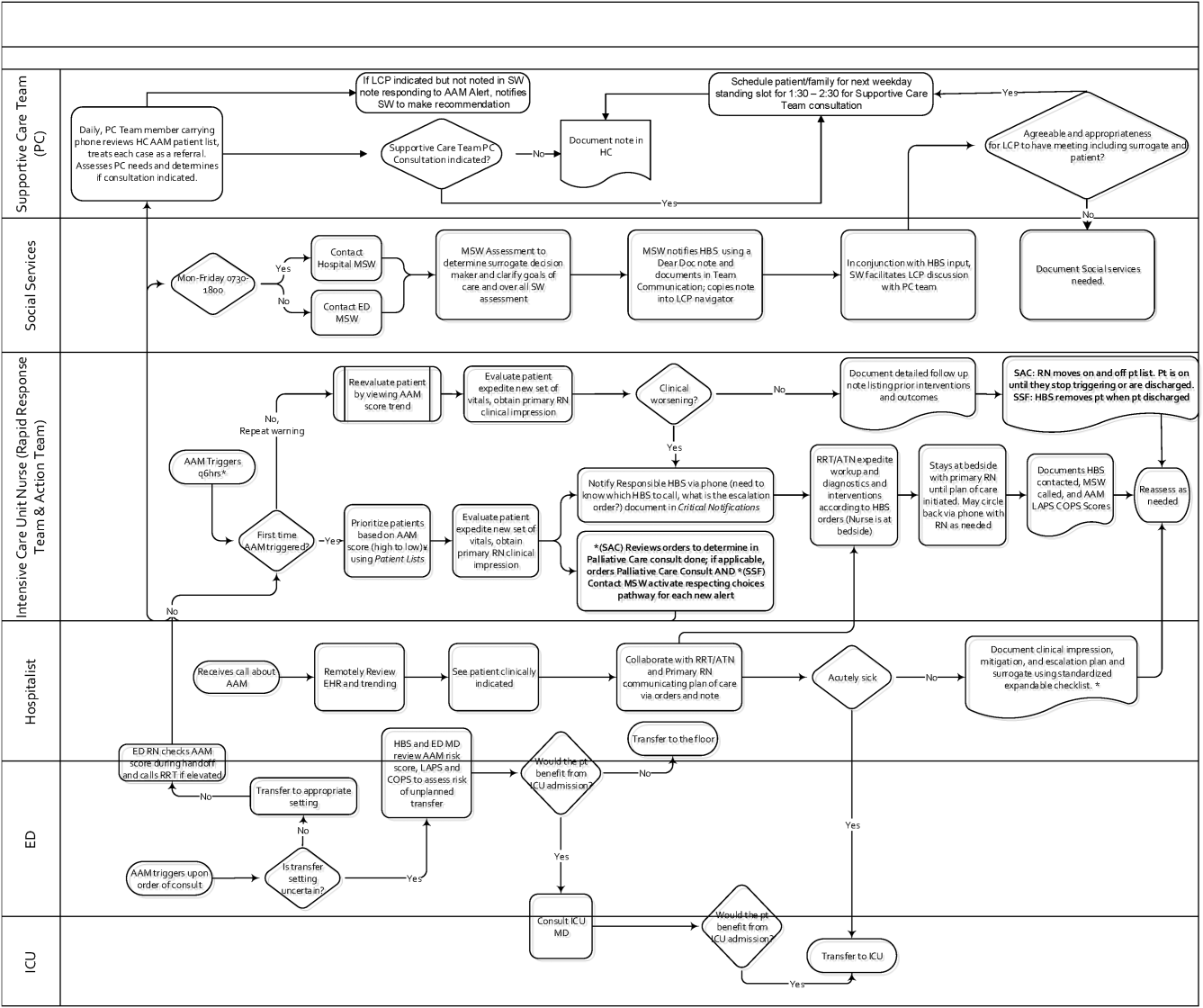
RESPONSE PROTOCOLS
At South San Francisco, the RRT consists of an ICU nurse, a respiratory care therapist, and a designated hospitalist; at Sacramento, the team is also augmented by an additional nurse (the house supervisor). In addition to responding to the AAM alerts, RRT nurses respond to other emergency calls such as code blues, stroke alerts, and patient or patient‐familyinitiated rapid response calls. They also expedite time sensitive workups and treatments. They check up on recent transfers from the ICU to ensure continued improvement justifying staying on the ward. Serving as peer educators, they assist with processes such as chest tube or central line insertions, troubleshoot high‐risk medication administration, and ensure that treatment bundles (eg, for sepsis) occur expeditiously.
The RRT reviews EWS scores every 6 hours. The AAM score is seen as soon as providers open the chart, which helps triage patients for evaluation. Because patients can still be at risk even without an elevated AAM score, all normal escalation pathways remain in place. Once an alert is noted in the inpatient dashboard, the RRT nurse obtains a fresh set of vital signs, assesses the patient's clinical status, and informs the physician, social worker, and primary nurse (Figure 2). Team members work with the bedside nurse, providing support with assessment, interventions, plans, and follow‐up. Once advised of the alert, the hospitalist performs a second chart review and evaluates the patient at the bedside to identify factors that could underlie potential deterioration. After this evaluation, the hospitalist documents concerns, orders appropriate interventions (which can include escalation), and determines appropriate follow‐up. We made sure the team knew that respiratory distress, arrhythmias, mental status changes, or worsening infection were responsible for over 80% of in‐hospital deterioration cases. We also involved palliative care earlier in patient care, streamlining the process so the RRT makes just 1 phone call to the social worker, who contacts the palliative care physician and nurse to ensure patients have a designated surrogate in the event of further deterioration.
Our initial documentation template consisted of a comprehensive organ system‐based physician checklist. However, although this was of use to covering physicians unfamiliar with a given patient, it was redundant and annoying to attending providers already familiar with the patient. After more than 30 iterations, we settled on a succinct note that only documented the clinicians' clinical judgment as to what constituted the major risk for deterioration and what the mitigation strategies would be. Both of these judgments are in a checklist format (see Supporting Information, Appendix, in the online version of this article for the components of the physician and nurse notes).
Prior to the implementation of the system, RRT nurses performed proactive rounding by manually checking patient labs and vital signs, an inefficient process due to the poor sensitivity and specificity of individual values. Following implementation of the system, RRT RNs and clinicians switched to sorting patients by the 3 scores (COPS2, LAPS2, AAM). For example, patients may be stable at admission (as evidenced by their AAM score) but be at high risk due to their comorbidities. One approach that has been employed is to proactively check such patients to ensure they have a care directive in place, as is described in the article by Granich et al.[14] The Supportive Care Team (detailed in Granich et al.) assesses needs for palliative care and provides in‐hospital consultation as needed. Social services staff perform chart reviews to ensure a patient surrogate has been defined and also works with patients and their families to clarify goals of care.
CHALLENGES AND KEY LEARNINGS
One challenge that arose was reconciling the periodic nature of the alert (every 6 hours) with physicians' availability, which varied due to different rounding workflows at the 2 sites. Consequently, the alert cycle was changed; at the first site, the cycle was set to 1000‐1600‐2200‐0400, whereas the second site chose 0800‐1400‐2000‐0200.
One essential but problematic component of the clinical response is the issue of documentation. Inadequate documentation could lead to adverse outcomes, clinician malpractice exposure, and placing the entire hospital at risk for enterprise liability when clinical responses are not documented. This issue is complicated by the fact that overzealous efforts could lead to less or no documentation by making it too onerous for busy clinicians. We found that the ease with which data can populate progress notes in the EMR can lead to note bloat. Clearly, no documentation is not enough, and a complete history and physical is too much. Paradoxically, 1 of the issues underlying our problems with documentation was the proactive nature of the alerts themselves; because they are based on an outcome prediction in the next 12 hours, documenting the response to them may lack (perceived) urgency.
Shortly after the system went live, a patient who had been recently transferred out to the ward from the ICU triggered an alert. As a response was mounted, the team realized that existing ward protocols did not specify which physician service (intensivist or hospitalist) was responsible for patients who were transitioning from 1 unit to another. We also had to perform multiple revisions of the protocols specifying how alerts were handled when they occurred at times of change of shift. Eventually, we settled on having the combination of a hospitalist and an RRT nurse as the cornerstone of the response, with the hospitalist service as the primary owner of the entire process, but this arrangement might need to be varied in different settings. As a result of the experience with the pilot, the business case for deployment in the remaining 19 hospitals includes a formal budget request so that all have properly staffed RRTs, although the issue of primary ownership of the alert process for different patient types (eg, surgical patients) will be decided on a hospital‐by‐hospital basis. These experiences raise the intriguing possibility that implementation of alert systems can lead to the identification of systemic gaps in existing protocols. These gaps can include specific components of the hospital service agreements between multiple departments (emergency, hospital medicine, ICU, palliative care, surgery) as well as problems with existing workflows.
In addition to ongoing tweaking of care protocols, 3 issues remain unresolved. First is the issue of documentation. The current documentation notes are not completely satisfactory, and we are working with the KPNC EMR administrators to refine the tool. Desirable refinements include (1) having the system scores populate in more accessible sectors of the EMR where their retrieval will facilitate increased automation of the note writing process, (2) changing the note type to a note that will facilitate process audits, and (3) linking the note to other EMR tools so that the response arm can be tracked more formally. The second issue is the need to develop strategies to address staff turnover; for example, newer staff may not have received the same degree of exposure to the system as those who were there when it was started. Finally, due to limited resources, we have done very limited work on more mechanistic analyses of the clinical response itself. For example, it would be desirable to perform a formal quantitative, risk‐adjusted process‐outcome analysis of why some patients' outcomes are better than others following an alert.
Finally, it is also the case that we have had some unexpected occurrences that hint at new uses and benefits of alert systems. One of these is the phenomenon of chasing the alert. Some clinicians, on their own, have taken a more proactive stance in the care of patients in whom the AAM score is rising or near the alert threshold. This has 2 potential consequences. Some patients are stabilized and thus do not reach threshold instability levels. In other cases, patients reach threshold but the response team is informed that things are already under control. A second unexpected result is increased requests for COPS2 scores by clinicians who have heard about the system, particularly surgeons who would like to use the comorbidity scores as a screening tool in the outpatient setting. Because KPNC is an integrated system, it is not likely that such alternatives will be implemented immediately without considerable analysis, but it is clear that the system's deployment has captured the clinicians' imagination.
CONCLUSIONS AND FUTURE DIRECTIONS
Our preparatory efforts have been successful. We have found that embedding an EWS in a commercially available EMR is acceptable to hospital physicians and nurses. We have developed a coordinated workflow for mitigation and escalation that is tightly linked to the availability of probabilistic alerts in real time. Although resource limitations have precluded us from conducting formal clinician surveys, the EWS has been discussed at multiple hospital‐wide as well as department‐specific meetings. Although there have been requests for clarification, refinements, and modifications in workflows, no one has suggested that the system be discontinued. Further, many of the other KPNC hospitals have requested that the EWS be deployed at their site. We have examined KPNC databases that track patient complaints and have not found any complaints that could be linked to the EWS. Most importantly, the existence of the workflows we have developed has played a major role in KPNC's decision to deploy the system in its remaining hospitals.
Although alert fatigue is the number 1 reason that clinicians do not utilize embedded clinical decision support,[26] simply calibrating statistical models is insufficient. Careful consideration of clinicians' needs and responsibilities, particularly around ownership of patients and documentation, is essential. Such consideration needs to include planning time and socializing the system (providing multiple venues for clinicians to learn about the system as well as participate in the process for using it).
We anticipate that, as the system leaves the pilot stage and becomes a routine component of hospital care, additional enhancements (eg, sending notifications to smart phones, providing an alert response tracking system) will be added. Our organization is also implementing real‐time concurrent review of inpatient EMRs (eg, for proactive detection of an expanded range of potential process failures), and work is underway on how to link the workflows we describe here with this effort. As has been the case with other systems,[27] it is likely that we will eventually move to continuous scanning of patient data rather than only every 6 hours. Given that the basic workflow is quite robust and amenable to local modifications, we are confident that our clinicians and hospitals will adapt to future system enhancements.
Lastly, we intend to conduct additional research on the clinical response itself. In particular, we consider it extremely important to conduct formal quantitative analyses on why some patients' outcomes are better than others following an alert. A key component of this effort will be to develop tools that can permit an automatedor nearly automatedassessment of the clinical response. For example, we are considering automated approaches that would scan the EMR for the presence of specific orders, notes, vital signs patterns, and laboratory tests following an alert. Whereas it may not be possible to dispense with manual chart review, even partial automation of a feedback process could lead to significant enhancement of our quality improvement efforts.
Acknowledgements
The authors thank Dr. Michelle Caughey, Dr. Philip Madvig, Dr. Brian Hoberman, Dr. Patricia Conolly, and Ms. Barbara Crawford for their administrative support; Dr. Tracy Lieu for reviewing the manuscript; and Ms. Rachel Lesser for formatting the manuscript. The authors also thank Drs. Jason Anderson, John Fitzgibbon, Elena M. Nishimura, and Najm Haq for their support of the project. We are particularly grateful to our nurses, Theresa A. Villorente, Zoe Sutton, Doanh Ly, Catherine Burger, and Hillary R. Mitchell, for their critical assistance. Last but not least, we also thank all the hospitalists and nurses at the Kaiser Permanente Sacramento and South San Francisco hospitals.
Disclosures: This work was supported by a grant from the Gordon and Betty Moore Foundation (Early Detection, Prevention, and Mitigation of Impending Physiologic Deterioration in Hospitalized Patients Outside Intensive Care: Phase 3, pilot), The Permanente Medical Group, Inc., and Kaiser Foundation Hospitals, Inc. Dr. Liu was supported by the National Institute for General Medical Sciences award K23GM112018. As part of our agreement with the Moore Foundation, we made a commitment to disseminate our findings in articles such as this one. However, the Gordon and Betty Moore Foundation played no role in how we actually structured our articles, nor did they review or preapprove any of the manuscripts submitted as part of the dissemination component; the same was the case with the other sponsors. None of the authors has any conflicts of interest to declare of relevance to this work
Patients who deteriorate outside highly monitored settings and who require unplanned transfer to the intensive care unit (ICU) are known to have high mortality and morbidity.[1, 2, 3, 4, 5] The notion that early detection of a deteriorating patient improves outcomes has intuitive appeal and is discussed in a large number of publications.[6, 7, 8, 9, 10] However, much less information is available on what should be done after early detection is made.[11] Existing literature on early warning systems (EWSs) does not provide enough detail to serve as a map for implementation. This lack of transparency is complicated by the fact that, although the comprehensive inpatient electronic medical record (EMR) now constitutes the central locus for clinical practice, much of the existing literature comes from research institutions that may employ home‐grown EMRs, not community hospitals that employ commercially available systems.
In this issue of the Journal of Hospital Medicine, we describe our efforts to bridge that gap by implementing an EWS in a pair of community hospitals. The EWS's development and its basic statistical and electronic infrastructure are described in the articles by Escobar and Dellinger and Escobar et al.[2, 12, 13] In this report, we focus on how we addressed clinicians' primary concern: What do we do when we get an alert? Because it is described in detail by Granich et al.[14] elsewhere in this issue of the Journal of Hospital Medicine, a critical component of our implementation process (ensuring that patient preferences with respect to supportive care are honored) is not discussed.
Our article is divided into the following sections: rationale, preimplementation preparatory work, workflow development, response protocols, challenges and key learnings, and concluding reflections.
RATIONALE
Much of the previous work on the implementation of alarm systems has focused on the statistics behind detection or on the quantification of processes (eg, how many rapid response calls were triggered) or on outcomes such as mortality. The conceptual underpinnings and practical steps necessary for successful integration of an alarm system into the clinicians' workflow have not been articulated. Our theoretical framework was based on (1) improving situational awareness[15] (knowing what is going on around you and what is likely to happen next) and (2) mitigating cognitive errors.
An EWS enhances situational awareness most directly by earlier identification of a problem with a particular patient. As is detailed by Escobar et al.[16] in this issue of the Journal of Hospital Medicine, our EWS extracts EMR data every 6 hours, performs multiple calculations, and then displays 3 scores in real time in the inpatient dashboard (known as the Patient Lists activity in the Epic EMR). The first of these scores is the Laboratory‐Based Acute Physiologic Score, version 2 (LAPS2), an objective severity score whose retrospective version is already in use in Kaiser Permanente Northern California (KPNC) for internal benchmarking.[13] This score captures a patient's overall degree of physiologic instability within the preceding 72 hours. The second is the Comorbidity Point Score, version 2 (COPS2), a longitudinal comorbidity score based on the patient's diagnoses over the preceding 12 months.[13] This score captures a patient's overall comorbidity burden. Thus, it is possible for a patient to be very ill (high COPS2) while also being stable (low LAPS2) or vice versa. Both of these scores have other uses, including prediction of rehospitalization risk in real time,[17] which is also being piloted at KPNC. Finally, the Advanced Alert Monitoring (AAM) score, which integrates the LAPS2 and COPS2 with other variables, provides a 12‐hour deterioration risk, with a threshold value of 8% triggering response protocols. At or above this threshold, which was agreed to prior to implementation, the system achieves 25% sensitivity, 98% specificity, with a number needed to evaluate of 10 to 12, a level of workload that was felt to be acceptable by clinicians. Actions triggered by the EWS may be quite different from those one would take when being notified of a code blue, which is called at the time an event occurs. The EWS focuses attention on patients who might be missed because they do not yet appear critically ill. It also provides a shared, quantifiable measure of a patient's risk that can trigger a standardized plan of action to follow in evaluating and treating a patient.[15]
In addition to enhancing situational awareness, we intended the alarms to produce cognitive change in practitioners. Our goal was to replace medical intuition with analytic, evidence‐based judgment of future illness. We proceeded with the understanding that replacing quick intuition with slower analytic response is an essential skill in developing sound clinical reasoning.[18, 19, 20] The alert encourages physicians to reassess high‐risk patients facilitating a cognitive shift from automatic, error‐prone processing to slower, deliberate processing. Given the busy pace of ward work, slowing down permits clinicians to reassess previously overlooked details. Related to this process of inducing cognitive change is a secondary effect: we uncovered and discussed physician biases. Physicians are subject to potential biases that allow patients to deteriorate.[18, 19, 20] Therefore, we addressed bias through education. By reviewing particular cases of unanticipated deterioration at each hospital facility, we provided evidence for the problem of in‐hospital deterioration. This framed the new tool as an opportunity for improving treatment and encouraged physicians to act on the alert using a structured process.
INTERVENTIONS
Preimplementation Preparatory Work
Initial KPNC data provided strong support for the generally accepted notion that unplanned transfer patients have poor outcomes.[2, 4, 5] However, published reports failed to provide the granular detail clinicians need to implement a response arm at the unit and patient level. In preparation for going live, we conducted a retrospective chart review. This included data from patients hospitalized from January 1, 2011 through December 31, 2012 (additional detail is provided in the Supporting Information, Appendix, in the online version of this article). The key findings from our internal review of subjective documentation preceding deterioration are similar to those described in the literature and summarized in Figure 1, which displays the 5 most common clinical presentations associated with unplanned transfers.
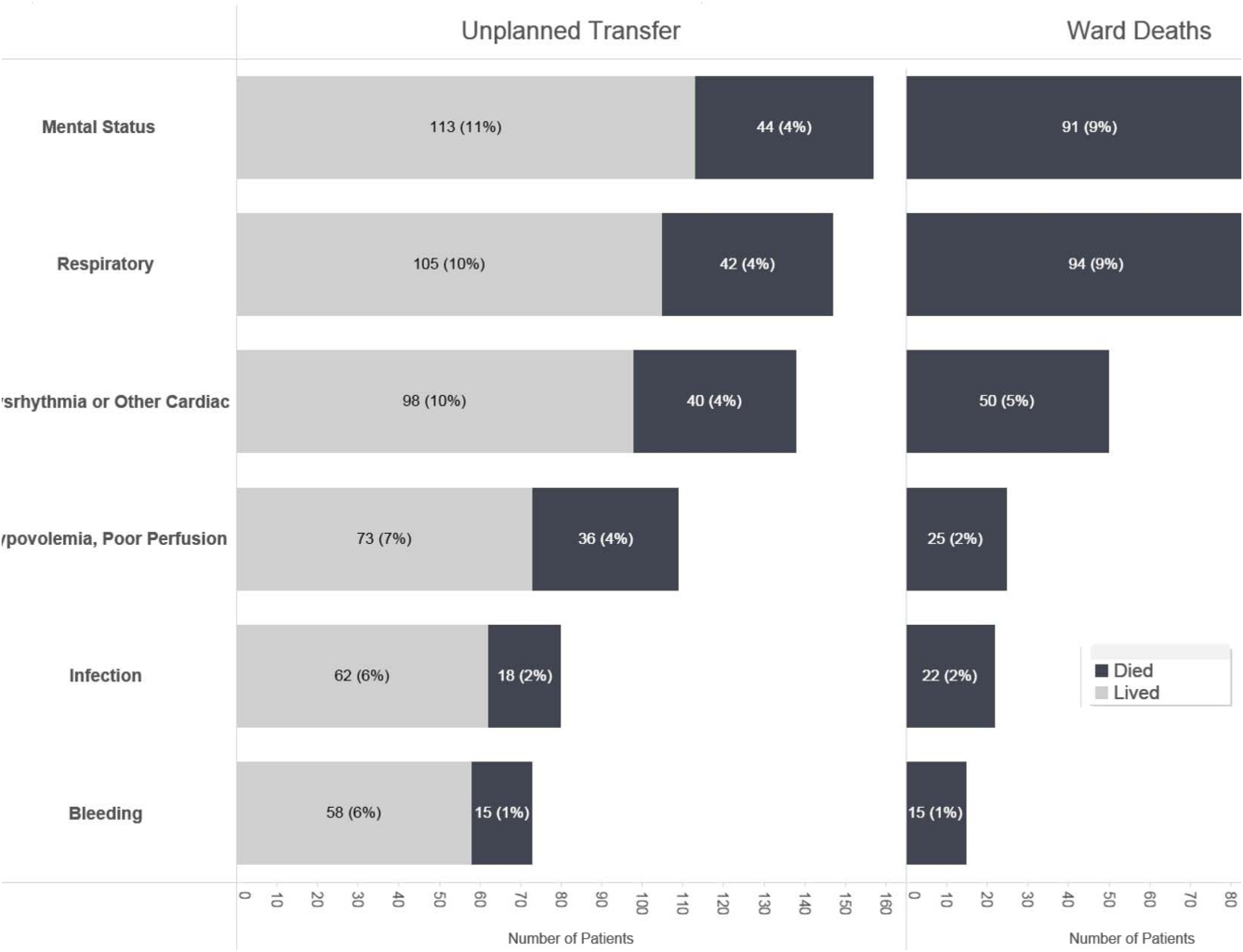
The chart review served several major roles. First, it facilitated cognitive change by eliminating the notion that it can't happen here. Second, it provided considerable guidance on key clinical components that had to be incorporated into the workflow. Third, it engaged the rapid response team (RRT) in reviewing our work retrospectively to identify future opportunities. Finally, the review provided considerable guidance with respect to structuring documentation requirements.
As a result of the above efforts, other processes detailed below, and knowledge described in several of the companion articles in this issue of the Journal of Hospital Medicine, 3 critical elements, which had been explicitly required by our leadership, were in place prior to the go‐live date: a general consensus among hospitalists and nurses that this would be worth testing, a basic clinical response workflow, and an automated checklist for documentation. We refined these in a 2‐week shadowing phase preceding the start date. In this phase, the alerts were not displayed in the EMR. Instead, programmers working on the project notified selected physician leaders by phone. This permitted them to understand exactly what sort of patients were reaching the physiologic threshold so that they could better prepare both RRT registered nurses (RNs) and hospitalists for the go‐live date. This also provided an opportunity to begin refining the documentation process using actual patients.
The original name for our project was Early Detection of Impending Physiologic Deterioration. However, during the preparatory phase, consultation with our public relations staff led to a concern that the name could be frightening to some patients. This highlights the need to consider patient perceptions and how words used in 1 way by physicians can have different connotations to nonclinicians. Consequently, the system was renamed, and it is now referred to as Advance Alert Monitoring (AAM).
Workflow Development
We carefully examined the space where electronic data, graphical user interfaces, and clinical practice blend, a nexus now commonly referred to as workflow or user experience.[21] To promote situational awareness and effect cognitive change, we utilized the Institute for Health Care Improvement's Plan‐Do‐Study‐Act model.[22, 23] We then facilitated the iterative development of a clinician‐endorsed workflow.[22, 23, 24, 25] By adjusting the workflow based on ongoing experience and giving clinicians multiple opportunities to revise (a process that continues to date), we ensured clinicians would approach and endorse the alarm system as a useful tool for decision support.
Table 1 summarizes the work groups assembled for our implementation, and Table 2 provides a system‐oriented checklist indicating key components that need to be in place prior to having an early warning system go live in a hospital. Figure 2 summarizes the alert response protocols we developed through an iterative process at the 2 pilot sites. The care path shown in Figure 2 is the result of considerable revision, mostly due to actual experience acquired following the go live date. The diagram also includes a component that is still work in progress. This is how an emergency department probability estimate (triage support) will be integrated into both the ward as well as the ICU workflows. Although this is beyond the scope of this article, other hospitals may be experimenting with triage support (eg, for sepsis patients), so it is important to consider how one would incorporate such support into workflows.
Workgroup | Goals |
---|---|
| |
Clinical checklist | Perform structured chart review of selected unplanned transfer patients and near misses |
Develop a checklist for mitigation strategies given an alert | |
Develop documentation standards given an alert | |
Develop escalation protocol given an alert | |
Workload and threshold | Determine threshold for sensitivity of alerts and resulting impact on clinician workload |
Patient preferences | Prepare background information to be presented to providers regarding end‐of‐life care and POLST orders |
Coordinate with clinical checklist workgroup to generate documentation templates that provide guidance for appropriate management of patients regarding preferences on escalation of care and end‐of‐life care | |
Electronic medical record coordination | Review proposed electronic medical record changes |
Make recommendation for further changes as needed | |
Develop plan for rollout of new and/or revised electronic record tools | |
Designate contact list for questions/emssues that may arise regarding electronic record changes during the pilot | |
Determine alert display choices and mode of alert notification | |
Nursing committee | Review staffing needs in anticipation of alert |
Coordinate with workload and threshold group | |
Develop training calendar to ensure skills necessary for successful implementation of alerts | |
Make recommendations for potential modification of rapid response team's role in development of a clinical checklist for nurses responding to an alert | |
Design educational materials for clinicians | |
Local communication strategy | Develop internal communication plan (for clinical staff not directly involved with pilot) |
Develop external communication plan (for nonclinicians who may hear about the project) |
Level | Tasks |
---|---|
Administration | Obtain executive committee approval |
Establish communication protocols with quality assurance and quality improvement committees | |
Review protocols with medicallegal department | |
Communication | Write media material for patients and families |
Develop and disseminate scripts for front‐line staff | |
Develop communication and meet with all relevant front‐line staff on merits of project | |
Educate all staff on workflow changes and impacts | |
Clinical preparation | Conduct internal review of unplanned transfers and present results to all clinicians |
Determine service level agreements, ownership of at‐risk patients, who will access alerts | |
Conduct staff meetings to educate staff | |
Perform debriefs on relevant cases | |
Determine desired outcomes, process measures, balancing measures | |
Determine acceptable clinician burden (alerts/day) | |
Technology | Establish documentation templates |
Ensure access to new data fields (electronic medical record security process must be followed for access rights) | |
Workflows | Workflows (clinical response, patient preferences, supportive care, communication, documentation) must be in place prior to actual go live |
Shadowing | Testing period (alerts communicated to selected clinicians prior to going live) should occur |
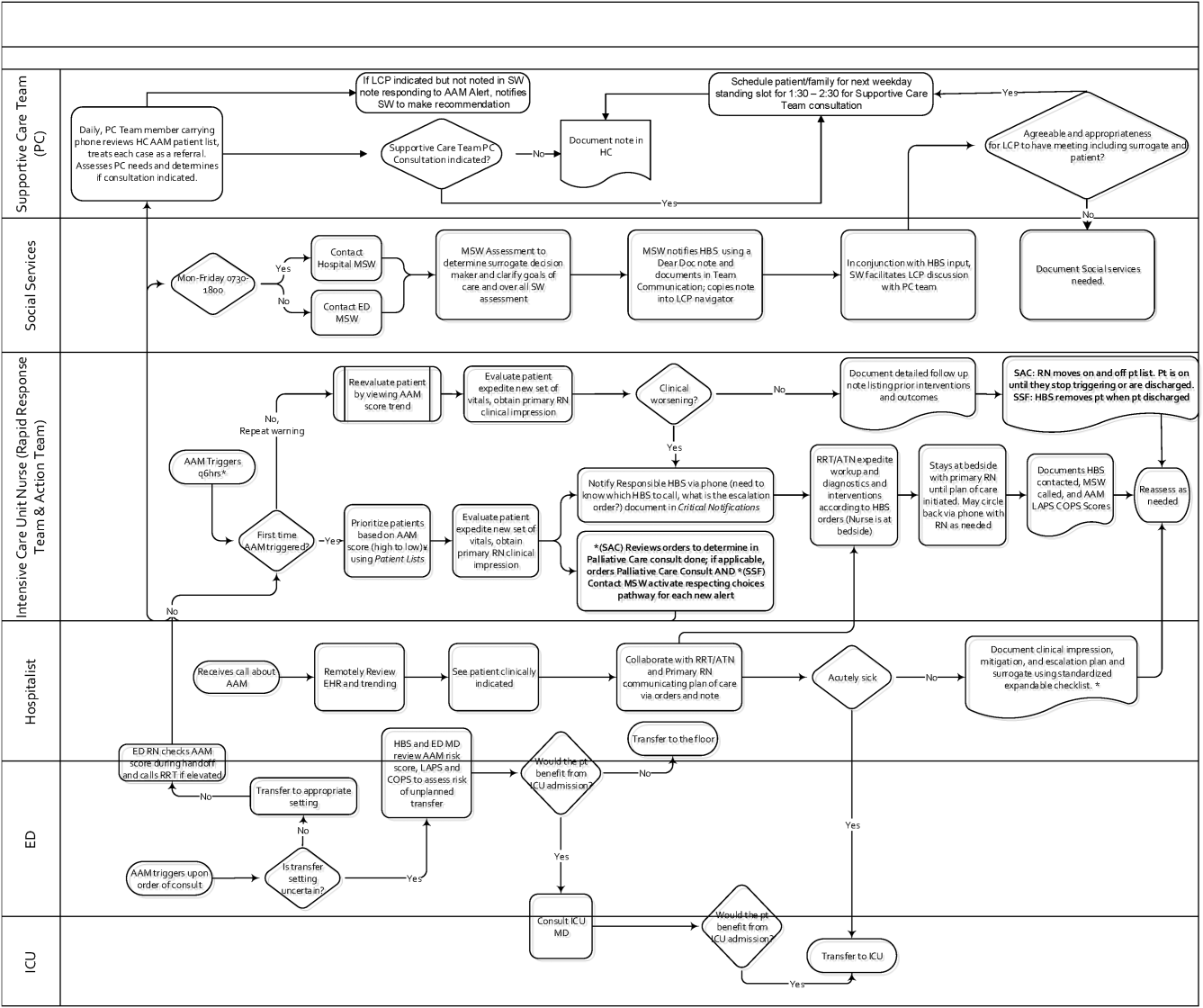
RESPONSE PROTOCOLS
At South San Francisco, the RRT consists of an ICU nurse, a respiratory care therapist, and a designated hospitalist; at Sacramento, the team is also augmented by an additional nurse (the house supervisor). In addition to responding to the AAM alerts, RRT nurses respond to other emergency calls such as code blues, stroke alerts, and patient or patient‐familyinitiated rapid response calls. They also expedite time sensitive workups and treatments. They check up on recent transfers from the ICU to ensure continued improvement justifying staying on the ward. Serving as peer educators, they assist with processes such as chest tube or central line insertions, troubleshoot high‐risk medication administration, and ensure that treatment bundles (eg, for sepsis) occur expeditiously.
The RRT reviews EWS scores every 6 hours. The AAM score is seen as soon as providers open the chart, which helps triage patients for evaluation. Because patients can still be at risk even without an elevated AAM score, all normal escalation pathways remain in place. Once an alert is noted in the inpatient dashboard, the RRT nurse obtains a fresh set of vital signs, assesses the patient's clinical status, and informs the physician, social worker, and primary nurse (Figure 2). Team members work with the bedside nurse, providing support with assessment, interventions, plans, and follow‐up. Once advised of the alert, the hospitalist performs a second chart review and evaluates the patient at the bedside to identify factors that could underlie potential deterioration. After this evaluation, the hospitalist documents concerns, orders appropriate interventions (which can include escalation), and determines appropriate follow‐up. We made sure the team knew that respiratory distress, arrhythmias, mental status changes, or worsening infection were responsible for over 80% of in‐hospital deterioration cases. We also involved palliative care earlier in patient care, streamlining the process so the RRT makes just 1 phone call to the social worker, who contacts the palliative care physician and nurse to ensure patients have a designated surrogate in the event of further deterioration.
Our initial documentation template consisted of a comprehensive organ system‐based physician checklist. However, although this was of use to covering physicians unfamiliar with a given patient, it was redundant and annoying to attending providers already familiar with the patient. After more than 30 iterations, we settled on a succinct note that only documented the clinicians' clinical judgment as to what constituted the major risk for deterioration and what the mitigation strategies would be. Both of these judgments are in a checklist format (see Supporting Information, Appendix, in the online version of this article for the components of the physician and nurse notes).
Prior to the implementation of the system, RRT nurses performed proactive rounding by manually checking patient labs and vital signs, an inefficient process due to the poor sensitivity and specificity of individual values. Following implementation of the system, RRT RNs and clinicians switched to sorting patients by the 3 scores (COPS2, LAPS2, AAM). For example, patients may be stable at admission (as evidenced by their AAM score) but be at high risk due to their comorbidities. One approach that has been employed is to proactively check such patients to ensure they have a care directive in place, as is described in the article by Granich et al.[14] The Supportive Care Team (detailed in Granich et al.) assesses needs for palliative care and provides in‐hospital consultation as needed. Social services staff perform chart reviews to ensure a patient surrogate has been defined and also works with patients and their families to clarify goals of care.
CHALLENGES AND KEY LEARNINGS
One challenge that arose was reconciling the periodic nature of the alert (every 6 hours) with physicians' availability, which varied due to different rounding workflows at the 2 sites. Consequently, the alert cycle was changed; at the first site, the cycle was set to 1000‐1600‐2200‐0400, whereas the second site chose 0800‐1400‐2000‐0200.
One essential but problematic component of the clinical response is the issue of documentation. Inadequate documentation could lead to adverse outcomes, clinician malpractice exposure, and placing the entire hospital at risk for enterprise liability when clinical responses are not documented. This issue is complicated by the fact that overzealous efforts could lead to less or no documentation by making it too onerous for busy clinicians. We found that the ease with which data can populate progress notes in the EMR can lead to note bloat. Clearly, no documentation is not enough, and a complete history and physical is too much. Paradoxically, 1 of the issues underlying our problems with documentation was the proactive nature of the alerts themselves; because they are based on an outcome prediction in the next 12 hours, documenting the response to them may lack (perceived) urgency.
Shortly after the system went live, a patient who had been recently transferred out to the ward from the ICU triggered an alert. As a response was mounted, the team realized that existing ward protocols did not specify which physician service (intensivist or hospitalist) was responsible for patients who were transitioning from 1 unit to another. We also had to perform multiple revisions of the protocols specifying how alerts were handled when they occurred at times of change of shift. Eventually, we settled on having the combination of a hospitalist and an RRT nurse as the cornerstone of the response, with the hospitalist service as the primary owner of the entire process, but this arrangement might need to be varied in different settings. As a result of the experience with the pilot, the business case for deployment in the remaining 19 hospitals includes a formal budget request so that all have properly staffed RRTs, although the issue of primary ownership of the alert process for different patient types (eg, surgical patients) will be decided on a hospital‐by‐hospital basis. These experiences raise the intriguing possibility that implementation of alert systems can lead to the identification of systemic gaps in existing protocols. These gaps can include specific components of the hospital service agreements between multiple departments (emergency, hospital medicine, ICU, palliative care, surgery) as well as problems with existing workflows.
In addition to ongoing tweaking of care protocols, 3 issues remain unresolved. First is the issue of documentation. The current documentation notes are not completely satisfactory, and we are working with the KPNC EMR administrators to refine the tool. Desirable refinements include (1) having the system scores populate in more accessible sectors of the EMR where their retrieval will facilitate increased automation of the note writing process, (2) changing the note type to a note that will facilitate process audits, and (3) linking the note to other EMR tools so that the response arm can be tracked more formally. The second issue is the need to develop strategies to address staff turnover; for example, newer staff may not have received the same degree of exposure to the system as those who were there when it was started. Finally, due to limited resources, we have done very limited work on more mechanistic analyses of the clinical response itself. For example, it would be desirable to perform a formal quantitative, risk‐adjusted process‐outcome analysis of why some patients' outcomes are better than others following an alert.
Finally, it is also the case that we have had some unexpected occurrences that hint at new uses and benefits of alert systems. One of these is the phenomenon of chasing the alert. Some clinicians, on their own, have taken a more proactive stance in the care of patients in whom the AAM score is rising or near the alert threshold. This has 2 potential consequences. Some patients are stabilized and thus do not reach threshold instability levels. In other cases, patients reach threshold but the response team is informed that things are already under control. A second unexpected result is increased requests for COPS2 scores by clinicians who have heard about the system, particularly surgeons who would like to use the comorbidity scores as a screening tool in the outpatient setting. Because KPNC is an integrated system, it is not likely that such alternatives will be implemented immediately without considerable analysis, but it is clear that the system's deployment has captured the clinicians' imagination.
CONCLUSIONS AND FUTURE DIRECTIONS
Our preparatory efforts have been successful. We have found that embedding an EWS in a commercially available EMR is acceptable to hospital physicians and nurses. We have developed a coordinated workflow for mitigation and escalation that is tightly linked to the availability of probabilistic alerts in real time. Although resource limitations have precluded us from conducting formal clinician surveys, the EWS has been discussed at multiple hospital‐wide as well as department‐specific meetings. Although there have been requests for clarification, refinements, and modifications in workflows, no one has suggested that the system be discontinued. Further, many of the other KPNC hospitals have requested that the EWS be deployed at their site. We have examined KPNC databases that track patient complaints and have not found any complaints that could be linked to the EWS. Most importantly, the existence of the workflows we have developed has played a major role in KPNC's decision to deploy the system in its remaining hospitals.
Although alert fatigue is the number 1 reason that clinicians do not utilize embedded clinical decision support,[26] simply calibrating statistical models is insufficient. Careful consideration of clinicians' needs and responsibilities, particularly around ownership of patients and documentation, is essential. Such consideration needs to include planning time and socializing the system (providing multiple venues for clinicians to learn about the system as well as participate in the process for using it).
We anticipate that, as the system leaves the pilot stage and becomes a routine component of hospital care, additional enhancements (eg, sending notifications to smart phones, providing an alert response tracking system) will be added. Our organization is also implementing real‐time concurrent review of inpatient EMRs (eg, for proactive detection of an expanded range of potential process failures), and work is underway on how to link the workflows we describe here with this effort. As has been the case with other systems,[27] it is likely that we will eventually move to continuous scanning of patient data rather than only every 6 hours. Given that the basic workflow is quite robust and amenable to local modifications, we are confident that our clinicians and hospitals will adapt to future system enhancements.
Lastly, we intend to conduct additional research on the clinical response itself. In particular, we consider it extremely important to conduct formal quantitative analyses on why some patients' outcomes are better than others following an alert. A key component of this effort will be to develop tools that can permit an automatedor nearly automatedassessment of the clinical response. For example, we are considering automated approaches that would scan the EMR for the presence of specific orders, notes, vital signs patterns, and laboratory tests following an alert. Whereas it may not be possible to dispense with manual chart review, even partial automation of a feedback process could lead to significant enhancement of our quality improvement efforts.
Acknowledgements
The authors thank Dr. Michelle Caughey, Dr. Philip Madvig, Dr. Brian Hoberman, Dr. Patricia Conolly, and Ms. Barbara Crawford for their administrative support; Dr. Tracy Lieu for reviewing the manuscript; and Ms. Rachel Lesser for formatting the manuscript. The authors also thank Drs. Jason Anderson, John Fitzgibbon, Elena M. Nishimura, and Najm Haq for their support of the project. We are particularly grateful to our nurses, Theresa A. Villorente, Zoe Sutton, Doanh Ly, Catherine Burger, and Hillary R. Mitchell, for their critical assistance. Last but not least, we also thank all the hospitalists and nurses at the Kaiser Permanente Sacramento and South San Francisco hospitals.
Disclosures: This work was supported by a grant from the Gordon and Betty Moore Foundation (Early Detection, Prevention, and Mitigation of Impending Physiologic Deterioration in Hospitalized Patients Outside Intensive Care: Phase 3, pilot), The Permanente Medical Group, Inc., and Kaiser Foundation Hospitals, Inc. Dr. Liu was supported by the National Institute for General Medical Sciences award K23GM112018. As part of our agreement with the Moore Foundation, we made a commitment to disseminate our findings in articles such as this one. However, the Gordon and Betty Moore Foundation played no role in how we actually structured our articles, nor did they review or preapprove any of the manuscripts submitted as part of the dissemination component; the same was the case with the other sponsors. None of the authors has any conflicts of interest to declare of relevance to this work
- Location of patients before transfer to a tertiary care intensive care unit: impact on outcome. J Crit Care. 2009;24(1):108–113. , , , , .
- Intra‐hospital transfers to a higher level of care: contribution to total hospital and intensive care unit (ICU) mortality and length of stay (LOS). J Hosp Med. 2011;6(2):74–80. , , , , , .
- Unplanned transfers to a medical intensive care unit: causes and relationship to preventable errors in care. J Hosp Med. 2011;6(2):68–72. , , , .
- Adverse outcomes associated with delayed intensive care unit transfers in an integrated healthcare system. J Hosp Med. 2012;7(3):224–230. , , , .
- Risk factors for unplanned transfer to intensive care within 24 hours of admission from the emergency department in an integrated healthcare system. J Hosp Med. 2013;8(1):13–19. , , , , , .
- Reducing hospital standardized mortality rate with early interventions. J Trauma Nursing. 2006;13(4):178–182. , , , , , .
- Centile‐based early warning scores derived from statistical distributions of vital signs. Resuscitation. 2011;82(8):1013–1018. , , , , , .
- Randomized trial of automated, electronic monitoring to facilitate early detection of sepsis in the intensive care unit. Crit Care Med. 2012;40(7):2096–2101. , , , et al.
- Early recognition of acutely deteriorating patients in non‐intensive care units: assessment of an innovative monitoring technology. J Hosp Med. 2012;7(8):628–633. , , , et al.
- A randomized trial of real‐time automated clinical deterioration alerts sent to a rapid response team. J Hosp Med. 2014;9(7):424–429. , , , et al.
- Improving situation awareness to reduce unrecognized clinical deterioration and serious safety events. Pediatrics. 2013;131(1):e298–e308. , , , et al.
- Early detection of impending physiologic deterioration among patients who are not in intensive care: development of predictive models using data from an automated electronic medical record. J Hosp Med. 2012;7(5):388–395. , , , , , .
- Risk‐adjusting hospital mortality using a comprehensive electronic record in an integrated healthcare delivery system. Med Care. 2013;51(5):446–453. , , , , .
- Early detection of critical illness outside the intensive care unit: clarifying treatment plans and honoring goals of care using a supportive care team. J Hosp Med. 2016;11:000–000. , , . et al.
- A qualitative study examining the influences on situation awareness and the identification, mitigation and escalation of recognised patient risk. BMJ Qual Saf. 2014;23(2):153–161. , .
- Piloting electronic medical record‐based early detection of inpatient deterioration in community hospitals. J Hosp Med. 2016;11:000–000. , , , et al.
- Nonelective rehospitalizations and postdischarge mortality: predictive models suitable for use in real time. Med Care. 2015;53(11):916–923. , , , , , .
- The importance of cognitive errors in diagnosis and strategies to minimize them. Acad Med. 2003;78(8):775–780. .
- Cognitive debiasing 1: origins of bias and theory of debiasing. BMJ Qual Saf. 2013;22(suppl 2):ii58–ii64. , , .
- Cognitive debiasing 2: impediments to and strategies for change. BMJ Qual Saf. 2013;22(suppl 2):ii65–ii72. , , .
- Use of health information technology to reduce diagnostic errors. BMJ Qual Saf. 2013;22(suppl 2):ii40–ii51. , , .
- The Improvement Guide: A Practical Approach to Enhancing Organizational Performance. 2nd ed. San Francisco, CA: Jossey‐Bass; 2009. , , , , , .
- Understanding the components of quality improvement collaboratives: a systematic literature review. Milbank Q. 2013;91(2):354–394. , , , , .
- Reducing diagnostic errors in medicine: what's the goal? Acad Med. 2002;77(10):981–992. , , .
- Huddling for high reliability and situation awareness. BMJ Qual Saf. 2013;22(11):899–906. , , , .
- Top 10 patient safety concerns for healthcare organizations. ECRI Institute website. Available at: https://www.ecri.org/Pages/Top‐10‐Patient‐Safety‐Concerns.aspx. Accessed February 18, 2016.
- Automated detection of physiologic deterioration in hospitalized patients. J Am Med Inform Assoc. 2015;22(2):350–360. , , , et al.
- Location of patients before transfer to a tertiary care intensive care unit: impact on outcome. J Crit Care. 2009;24(1):108–113. , , , , .
- Intra‐hospital transfers to a higher level of care: contribution to total hospital and intensive care unit (ICU) mortality and length of stay (LOS). J Hosp Med. 2011;6(2):74–80. , , , , , .
- Unplanned transfers to a medical intensive care unit: causes and relationship to preventable errors in care. J Hosp Med. 2011;6(2):68–72. , , , .
- Adverse outcomes associated with delayed intensive care unit transfers in an integrated healthcare system. J Hosp Med. 2012;7(3):224–230. , , , .
- Risk factors for unplanned transfer to intensive care within 24 hours of admission from the emergency department in an integrated healthcare system. J Hosp Med. 2013;8(1):13–19. , , , , , .
- Reducing hospital standardized mortality rate with early interventions. J Trauma Nursing. 2006;13(4):178–182. , , , , , .
- Centile‐based early warning scores derived from statistical distributions of vital signs. Resuscitation. 2011;82(8):1013–1018. , , , , , .
- Randomized trial of automated, electronic monitoring to facilitate early detection of sepsis in the intensive care unit. Crit Care Med. 2012;40(7):2096–2101. , , , et al.
- Early recognition of acutely deteriorating patients in non‐intensive care units: assessment of an innovative monitoring technology. J Hosp Med. 2012;7(8):628–633. , , , et al.
- A randomized trial of real‐time automated clinical deterioration alerts sent to a rapid response team. J Hosp Med. 2014;9(7):424–429. , , , et al.
- Improving situation awareness to reduce unrecognized clinical deterioration and serious safety events. Pediatrics. 2013;131(1):e298–e308. , , , et al.
- Early detection of impending physiologic deterioration among patients who are not in intensive care: development of predictive models using data from an automated electronic medical record. J Hosp Med. 2012;7(5):388–395. , , , , , .
- Risk‐adjusting hospital mortality using a comprehensive electronic record in an integrated healthcare delivery system. Med Care. 2013;51(5):446–453. , , , , .
- Early detection of critical illness outside the intensive care unit: clarifying treatment plans and honoring goals of care using a supportive care team. J Hosp Med. 2016;11:000–000. , , . et al.
- A qualitative study examining the influences on situation awareness and the identification, mitigation and escalation of recognised patient risk. BMJ Qual Saf. 2014;23(2):153–161. , .
- Piloting electronic medical record‐based early detection of inpatient deterioration in community hospitals. J Hosp Med. 2016;11:000–000. , , , et al.
- Nonelective rehospitalizations and postdischarge mortality: predictive models suitable for use in real time. Med Care. 2015;53(11):916–923. , , , , , .
- The importance of cognitive errors in diagnosis and strategies to minimize them. Acad Med. 2003;78(8):775–780. .
- Cognitive debiasing 1: origins of bias and theory of debiasing. BMJ Qual Saf. 2013;22(suppl 2):ii58–ii64. , , .
- Cognitive debiasing 2: impediments to and strategies for change. BMJ Qual Saf. 2013;22(suppl 2):ii65–ii72. , , .
- Use of health information technology to reduce diagnostic errors. BMJ Qual Saf. 2013;22(suppl 2):ii40–ii51. , , .
- The Improvement Guide: A Practical Approach to Enhancing Organizational Performance. 2nd ed. San Francisco, CA: Jossey‐Bass; 2009. , , , , , .
- Understanding the components of quality improvement collaboratives: a systematic literature review. Milbank Q. 2013;91(2):354–394. , , , , .
- Reducing diagnostic errors in medicine: what's the goal? Acad Med. 2002;77(10):981–992. , , .
- Huddling for high reliability and situation awareness. BMJ Qual Saf. 2013;22(11):899–906. , , , .
- Top 10 patient safety concerns for healthcare organizations. ECRI Institute website. Available at: https://www.ecri.org/Pages/Top‐10‐Patient‐Safety‐Concerns.aspx. Accessed February 18, 2016.
- Automated detection of physiologic deterioration in hospitalized patients. J Am Med Inform Assoc. 2015;22(2):350–360. , , , et al.