User login
Patient-level exclusions from mHealth in a safety-net health system
Interest in mHealth—the use of mobile communication devices for clinical and public health—has exploded among clinicians and researchers for its potential to efficiently improve patient health. Recent studies have used mHealth’s asynchronous receptive and expressive communication functions in interventions targeted to managing care transitions and hospital readmissions.1-3 We also recently published on improved readmission risk assessments using postdischarge measures of patient reported outcomes, which could be collected through mobile devices. 4 But persistent disparities in access to5 and engagement with6 smartphones may threaten validity and equity when mHealth strategies do not fully address its own limitations.
Disparities introduced by uneven access to technology are well known, but the rapid, albeit belated, adoption of mobile devices by racial minority groups in the United States has allowed authors of recent thoughtful publications to recast mHealth as itself offering solutions to the disparities’ problem.7,8 Others have cautioned the emergence of disparities along domains other than race, such as low literacy and limited English proficiency (LEP).9 In this paper, we assessed the impact of inadequate reading health literacy (IRHL) and LEP on factors related to access and engagement with mHealth. We conducted our study among urban low-income adults in whom IRHL and LEP are common.
METHODS
We surveyed patients in a large public safety-net health system serving 132 municipalities, including the city of Chicago, in northeastern Illinois. In 2015, nearly 90% of patients were racial-ethnic minorities with more than one-third insured by Medicaid and another one-third uninsured. We sampled adult inpatients and outpatients separately by nonselectively approaching patients in November 2015 to complete an in-person questionnaire in a 464-bed hospital and in 2 primary-care clinics. All inpatients occupied a nonisolation room in a general medical-surgical ward that had been sampled for data collection for that day in 9-day cycles with 8 other similar units. All outpatients in the clinic waiting areas were approached on consecutive days until a predetermined recruitment target was met. Each participant was surveyed once in his/her preferred language (English or Spanish), was 18 years and older, consented verbally, and received no compensation. Sample size provided 80% power to detect a device ownership rate of 50% in an evenly allocated low literacy population compared to a reference rate of 66% assuming a 2-sided α of 0.05 using the Fisher exact test.
The 18-item questionnaire was informed by constructs addressed in the 2015 Pew Research Center smartphone survey.10 However, in addition to device ownership, we inquired about device capabilities, service-plan details, service interruptions due to difficulty paying bills in the previous year, home-Internet access, an active e-mail account, and self-assigned demographics. Self-reported reading health literacy,11 more directly measured than e-health literacy, was screened using a parsimonious instrument validated as a dichotomized measure.12 Instruments in English and Spanish were tested for appropriate and comprehensible word choices and syntax through pilot testing. We inferred LEP among patients preferring to complete the survey in Spanish based on our familiarity with the population. We defined any Internet access as having a mobile data-service plan or having home-Internet access. In addition, we inquired about primary insurance provider and offered Medicaid patients an informational brochure about the federal Lifeline Program (https://www.fcc.gov/lifeline) that subsidizes text-messaging-enabled cellular telephone service for low-income patients. Notably, we assessed engagement by asking about the extent of patients’ interest in “new ways of communicating with your doctor, clinic, or pharmacy using” text, e-mail, or mobile apps with a 5-level response scale ranging from “not at all interested” to “very interested”.
Participant characteristics were confirmed to be similar to the Cook County Health and Hospitals System patient population in 2015 with regards to age, gender, and race/ethnicity. We calculated unadjusted and adjusted odds ratios for IRHL and LEP’s association with each dependent measure of access (to smartphone, Internet, or e-mail) and engagement (using text messaging, e-mail, or mobile apps) controlling for age, gender, primary payer, recruitment location, IRHL, and LEP. Because we oversampled inpatients, we estimated sampling-weight-adjusted proportions and 95% confidence intervals (CI) of the entire CCHHS patient population with access to smartphone, data/text plan, non-prepaid plan, and service interruptions using STATA v13 (StataCorp LP, College Station Texas). The project received a waiver upon review by the local Institutional Review Board.
RESULTS
Participation rate was 65% (302/464). Differences in patients by site are shown in Table 1. IRHL was more frequent and LEP less frequent among hospitalized patients. As shown in Table 2, patients with IRHL were less likely to have any Internet access, to have an active e-mail account, and to be interested in using e-mail for healthcare communications. Patients with LEP were less likely than English speakers to be interested in using mobile apps. Inpatients were less likely than outpatients to be interested in text messaging for healthcare communications.
The estimated proportion (95% CI) of the health system’s patients owning a text-enabled mobile device was 87% (75%-94%) and an Internet-enabled mobile device was 64% (47%-78%). The proportion with no data service interruptions in the previous year was 40% (31%-50%).
DISCUSSION
In this cross-section of urban low-income adult patients, IRHL and LEP were factors associated with potential disparities introduced by mHealth. Even as access to smartphones becomes ubiquitous, lagging access to Internet and e-mail among low literacy patients, and low levels of technology engagement for healthcare communications among patients with IRHL or LEP, underscore concerns about equity in health systems’ adoption of mHealth strategies. Hospitalized patients were found to have diminished engagement with mHealth independent of IRHL and LEP.
Regarding engagement, significantly fewer patients with IRHL or LEP were interested in using technology for healthcare communications. Our finding suggests that health disparities already associated with these conditions13 may not be reduced by mobile device outreach alone and may even be worsened by it. Touch screens, audio-enabled questionnaires, and language translation engines are innovations that may be helpful to mitigate IRHL and LEP, but evidence is scarce. Privacy and security concerns, and lack of experience with technology, may also lower engagement. A contemporaneous study found lower apps’ usage among Latinos, also suggesting that language concordance between apps, their source, and targeted users is important.14 Low-tech solutions involving mobile telephone or even lower tech in-person communications targeted to the estimated 26% of the US population with low literacy15 and 20% with LEP16 may be practical stopgap measures. Even as disparities in access to technology across race-ethnicity are diminishing,10 equity across poverty levels, low levels of education, cultural norms, and disabilities may be more challenging to overcome. Our assessment indicates that large exclusions of a safety-net population in 2015 are a legitimate concern in communication strategies that rely too heavily on mHealth. These findings underscore the CONSORT-EHEALTH recommendation that investigators report web-based recruitment strategies and data-collection methods comprehensively.17
Regarding access, our estimates suggest that historical disparities in smartphone ownership are diminishing, but access to Internet capabilities may still be lower among the urban poor compared to the nation as a whole. The Pew Research Center found that 64% of Americans owned a smartphone in 2015 (respondents defined smartphone).10 In comparison, 87% (95% CI, 75%, 94%) of our study participants owned a text-enabled mobile device and 64% (47%, 78%) owned an Internet-enabled mobile device. However, the 40% (31%, 50%) of our safety-net population with an uninterrupted data plan over the previous year may be lower than the 50% of Americans reporting uninterrupted data plans over their lifetime.10 The impact of expense-related data plan interruptions is magnified by the 40% of our study population—compared to 15% of Americans—who are dependent on mobile devices for Internet access.10 The association between Internet connectivity and literacy evokes multiple bidirectional pathways yet to be elucidated. But if mHealth can reduce health disparities, closing the gap in device ownership is only a partial accomplishment, and future work also needs to expand Internet connectivity to allow literacy-enhancing and literacy-naïve technologies to flourish.
This study has limitations. Our study population was a consecutive sample and participation rate was less than 100%. However, we recruited participants into the study the way we may also have approached patients to introduce mHealth options in our clinical settings. Our sampling method proved adequate for our primary goal to explain differences in technology access and engagement using regression analysis. Although our patient population may not directly generalize to many healthcare systems, including other safety-net systems serving regions with variable technology uptake,18 our findings reflect the capacities and the preferences of the most disadvantaged segments of urban populations. We systematically excluded LEP non-Spanish speakers, but they consisted of less than 5% of inpatients and no outpatients. We did not assess current technology use. Finally, as discussed earlier, access and use of new technologies change rapidly and frequent updates are necessary.
mHealth is a promising tool because it may increase healthcare access, improve care quality, and promote research. All these potential benefits will be obtained with accompanying efforts to reduce healthcare disparities, especially where some technologies themselves are exclusionary.
Disclosures
The authors report no financial conflicts of interest.
1. Khosravi P, Ghapanchi AH. Investigating the effectiveness of technologies applied to assist seniors: a systematic literature review. Int J Med Inform. 2016;85:17-26. PubMed
2. Feltner C, Jones CD, Cené CW, et al. Transitional care interventions to prevent readmissions for persons with heart failure: a systematic review and meta-analysis. Ann Intern Med. 2014;160:774-784. PubMed
3. Prieto-Centurion V, Gussin HA, Rolle AJ, Krishnan JA. Chronic obstructive pulmonary disease readmissions at minority-serving institutions. Ann Am Thorac Soc. 2013;10:680-684. PubMed
4. Hinami K, Smith J, Deamant CD, BuBeshter K, Trick WE. When do patient-reported outcome measures inform readmission risk? J Hosp Med. 2015;10:294-300. PubMed
5. Gibbons MC. A historical overview of health disparities and the potential of eHealth solutions. J Med Internet Res. 2005;7:e50. PubMed
6. Nelson LA, Mulvaney SA, Gebretsadik T, Ho YX, Johnson KB, Osborn CY. Disparities in the use of a mHealth medication adherence promotion intervention for low-income adults with type2 diabetes. J Am Med Inform Assoc. 2016;23:12-18. PubMed
7. Martin T. Assessing mHealth: opportunities and barriers to patient engagement. J Health Care Poor Underserved. 2012;23:935-941. PubMed
8. Horn IB, Mendoza FS. Reframing the disparities agenda: a time to rethink, a time to focus. Acad Pediatr. 2013;14:115-116. PubMed
9. Viswanath K, Nagler RH, Bigman-Galimore CA, McCauley MP, Jung M, Ramanadhan S. The communications revolution and health inequalities in the 21st century: implications for cancer control. Cancer Epidemiol, BiomarkersPrev. 2012;21:1701-1708. PubMed
10. Pew Research Center. The Smartphone Difference. Pew Research Center; April 2015. Available at: http://www.pewinternet.org/2015/04/01/us-smartphone-use-in-2015/. Accessed January 12, 2017.
11. Baker DW. The meaning and measure of health literacy. J Gen Intern Med. 2011;21:878-883. PubMed
12. Morris NS, MacLean CD, Chew LD, Littenberg B. The single item literacy screener: evalution of a brief instrument to identify limited reading ability. BMC Fam Pract. 2006;7:21. PubMed
13. Sentell T, Braun K. Low health literacy, limited English proficiency, and health status in Asians, Latinos, and other racial/ethnic groups in California. J Health Commun. 2012;17:82-99. PubMed
14. Arora S, Ford K, Terp S, et al. Describing the evolution of mobile technology usage for Latino patients and comparing findings to national mHealth estimates. J Am Med Inform Assoc. 2016;23:979-983. PubMed
15. Paasche-Orlow MK, Parker RM, Gazmararian JA, Nielsen-Bohlman LT, Rudd RR. The prevalence of limited health literacy. J Gen Intern Med. 2005;20:175-184. PubMed
16. US Census Bureau. Detailed languages spoken at home and ability to speak English for the population 5 years and over: 2009-2013. Published 2015. Available at: http://www.census.gov/data/tables/2013/demo/2009-2013-lang-tables.html. Accessed March 31, 2016.
17. Eysenbach G, CONSORT-EHEALTH Group. CONSORT-EHEALTH: improving and standardizing evaluation reports of Web-based and mobile health intervention. J Med Internet Res. 2011;13:e126. PubMed
18. Schickedanz A, Huang D, Lopex A, et al. Access, interest, and attitudes toward electronic communication for health care among patients in the medical safety net. J Gen Intern Med. 2013;28:914-920. PubMed
Interest in mHealth—the use of mobile communication devices for clinical and public health—has exploded among clinicians and researchers for its potential to efficiently improve patient health. Recent studies have used mHealth’s asynchronous receptive and expressive communication functions in interventions targeted to managing care transitions and hospital readmissions.1-3 We also recently published on improved readmission risk assessments using postdischarge measures of patient reported outcomes, which could be collected through mobile devices. 4 But persistent disparities in access to5 and engagement with6 smartphones may threaten validity and equity when mHealth strategies do not fully address its own limitations.
Disparities introduced by uneven access to technology are well known, but the rapid, albeit belated, adoption of mobile devices by racial minority groups in the United States has allowed authors of recent thoughtful publications to recast mHealth as itself offering solutions to the disparities’ problem.7,8 Others have cautioned the emergence of disparities along domains other than race, such as low literacy and limited English proficiency (LEP).9 In this paper, we assessed the impact of inadequate reading health literacy (IRHL) and LEP on factors related to access and engagement with mHealth. We conducted our study among urban low-income adults in whom IRHL and LEP are common.
METHODS
We surveyed patients in a large public safety-net health system serving 132 municipalities, including the city of Chicago, in northeastern Illinois. In 2015, nearly 90% of patients were racial-ethnic minorities with more than one-third insured by Medicaid and another one-third uninsured. We sampled adult inpatients and outpatients separately by nonselectively approaching patients in November 2015 to complete an in-person questionnaire in a 464-bed hospital and in 2 primary-care clinics. All inpatients occupied a nonisolation room in a general medical-surgical ward that had been sampled for data collection for that day in 9-day cycles with 8 other similar units. All outpatients in the clinic waiting areas were approached on consecutive days until a predetermined recruitment target was met. Each participant was surveyed once in his/her preferred language (English or Spanish), was 18 years and older, consented verbally, and received no compensation. Sample size provided 80% power to detect a device ownership rate of 50% in an evenly allocated low literacy population compared to a reference rate of 66% assuming a 2-sided α of 0.05 using the Fisher exact test.
The 18-item questionnaire was informed by constructs addressed in the 2015 Pew Research Center smartphone survey.10 However, in addition to device ownership, we inquired about device capabilities, service-plan details, service interruptions due to difficulty paying bills in the previous year, home-Internet access, an active e-mail account, and self-assigned demographics. Self-reported reading health literacy,11 more directly measured than e-health literacy, was screened using a parsimonious instrument validated as a dichotomized measure.12 Instruments in English and Spanish were tested for appropriate and comprehensible word choices and syntax through pilot testing. We inferred LEP among patients preferring to complete the survey in Spanish based on our familiarity with the population. We defined any Internet access as having a mobile data-service plan or having home-Internet access. In addition, we inquired about primary insurance provider and offered Medicaid patients an informational brochure about the federal Lifeline Program (https://www.fcc.gov/lifeline) that subsidizes text-messaging-enabled cellular telephone service for low-income patients. Notably, we assessed engagement by asking about the extent of patients’ interest in “new ways of communicating with your doctor, clinic, or pharmacy using” text, e-mail, or mobile apps with a 5-level response scale ranging from “not at all interested” to “very interested”.
Participant characteristics were confirmed to be similar to the Cook County Health and Hospitals System patient population in 2015 with regards to age, gender, and race/ethnicity. We calculated unadjusted and adjusted odds ratios for IRHL and LEP’s association with each dependent measure of access (to smartphone, Internet, or e-mail) and engagement (using text messaging, e-mail, or mobile apps) controlling for age, gender, primary payer, recruitment location, IRHL, and LEP. Because we oversampled inpatients, we estimated sampling-weight-adjusted proportions and 95% confidence intervals (CI) of the entire CCHHS patient population with access to smartphone, data/text plan, non-prepaid plan, and service interruptions using STATA v13 (StataCorp LP, College Station Texas). The project received a waiver upon review by the local Institutional Review Board.
RESULTS
Participation rate was 65% (302/464). Differences in patients by site are shown in Table 1. IRHL was more frequent and LEP less frequent among hospitalized patients. As shown in Table 2, patients with IRHL were less likely to have any Internet access, to have an active e-mail account, and to be interested in using e-mail for healthcare communications. Patients with LEP were less likely than English speakers to be interested in using mobile apps. Inpatients were less likely than outpatients to be interested in text messaging for healthcare communications.
The estimated proportion (95% CI) of the health system’s patients owning a text-enabled mobile device was 87% (75%-94%) and an Internet-enabled mobile device was 64% (47%-78%). The proportion with no data service interruptions in the previous year was 40% (31%-50%).
DISCUSSION
In this cross-section of urban low-income adult patients, IRHL and LEP were factors associated with potential disparities introduced by mHealth. Even as access to smartphones becomes ubiquitous, lagging access to Internet and e-mail among low literacy patients, and low levels of technology engagement for healthcare communications among patients with IRHL or LEP, underscore concerns about equity in health systems’ adoption of mHealth strategies. Hospitalized patients were found to have diminished engagement with mHealth independent of IRHL and LEP.
Regarding engagement, significantly fewer patients with IRHL or LEP were interested in using technology for healthcare communications. Our finding suggests that health disparities already associated with these conditions13 may not be reduced by mobile device outreach alone and may even be worsened by it. Touch screens, audio-enabled questionnaires, and language translation engines are innovations that may be helpful to mitigate IRHL and LEP, but evidence is scarce. Privacy and security concerns, and lack of experience with technology, may also lower engagement. A contemporaneous study found lower apps’ usage among Latinos, also suggesting that language concordance between apps, their source, and targeted users is important.14 Low-tech solutions involving mobile telephone or even lower tech in-person communications targeted to the estimated 26% of the US population with low literacy15 and 20% with LEP16 may be practical stopgap measures. Even as disparities in access to technology across race-ethnicity are diminishing,10 equity across poverty levels, low levels of education, cultural norms, and disabilities may be more challenging to overcome. Our assessment indicates that large exclusions of a safety-net population in 2015 are a legitimate concern in communication strategies that rely too heavily on mHealth. These findings underscore the CONSORT-EHEALTH recommendation that investigators report web-based recruitment strategies and data-collection methods comprehensively.17
Regarding access, our estimates suggest that historical disparities in smartphone ownership are diminishing, but access to Internet capabilities may still be lower among the urban poor compared to the nation as a whole. The Pew Research Center found that 64% of Americans owned a smartphone in 2015 (respondents defined smartphone).10 In comparison, 87% (95% CI, 75%, 94%) of our study participants owned a text-enabled mobile device and 64% (47%, 78%) owned an Internet-enabled mobile device. However, the 40% (31%, 50%) of our safety-net population with an uninterrupted data plan over the previous year may be lower than the 50% of Americans reporting uninterrupted data plans over their lifetime.10 The impact of expense-related data plan interruptions is magnified by the 40% of our study population—compared to 15% of Americans—who are dependent on mobile devices for Internet access.10 The association between Internet connectivity and literacy evokes multiple bidirectional pathways yet to be elucidated. But if mHealth can reduce health disparities, closing the gap in device ownership is only a partial accomplishment, and future work also needs to expand Internet connectivity to allow literacy-enhancing and literacy-naïve technologies to flourish.
This study has limitations. Our study population was a consecutive sample and participation rate was less than 100%. However, we recruited participants into the study the way we may also have approached patients to introduce mHealth options in our clinical settings. Our sampling method proved adequate for our primary goal to explain differences in technology access and engagement using regression analysis. Although our patient population may not directly generalize to many healthcare systems, including other safety-net systems serving regions with variable technology uptake,18 our findings reflect the capacities and the preferences of the most disadvantaged segments of urban populations. We systematically excluded LEP non-Spanish speakers, but they consisted of less than 5% of inpatients and no outpatients. We did not assess current technology use. Finally, as discussed earlier, access and use of new technologies change rapidly and frequent updates are necessary.
mHealth is a promising tool because it may increase healthcare access, improve care quality, and promote research. All these potential benefits will be obtained with accompanying efforts to reduce healthcare disparities, especially where some technologies themselves are exclusionary.
Disclosures
The authors report no financial conflicts of interest.
Interest in mHealth—the use of mobile communication devices for clinical and public health—has exploded among clinicians and researchers for its potential to efficiently improve patient health. Recent studies have used mHealth’s asynchronous receptive and expressive communication functions in interventions targeted to managing care transitions and hospital readmissions.1-3 We also recently published on improved readmission risk assessments using postdischarge measures of patient reported outcomes, which could be collected through mobile devices. 4 But persistent disparities in access to5 and engagement with6 smartphones may threaten validity and equity when mHealth strategies do not fully address its own limitations.
Disparities introduced by uneven access to technology are well known, but the rapid, albeit belated, adoption of mobile devices by racial minority groups in the United States has allowed authors of recent thoughtful publications to recast mHealth as itself offering solutions to the disparities’ problem.7,8 Others have cautioned the emergence of disparities along domains other than race, such as low literacy and limited English proficiency (LEP).9 In this paper, we assessed the impact of inadequate reading health literacy (IRHL) and LEP on factors related to access and engagement with mHealth. We conducted our study among urban low-income adults in whom IRHL and LEP are common.
METHODS
We surveyed patients in a large public safety-net health system serving 132 municipalities, including the city of Chicago, in northeastern Illinois. In 2015, nearly 90% of patients were racial-ethnic minorities with more than one-third insured by Medicaid and another one-third uninsured. We sampled adult inpatients and outpatients separately by nonselectively approaching patients in November 2015 to complete an in-person questionnaire in a 464-bed hospital and in 2 primary-care clinics. All inpatients occupied a nonisolation room in a general medical-surgical ward that had been sampled for data collection for that day in 9-day cycles with 8 other similar units. All outpatients in the clinic waiting areas were approached on consecutive days until a predetermined recruitment target was met. Each participant was surveyed once in his/her preferred language (English or Spanish), was 18 years and older, consented verbally, and received no compensation. Sample size provided 80% power to detect a device ownership rate of 50% in an evenly allocated low literacy population compared to a reference rate of 66% assuming a 2-sided α of 0.05 using the Fisher exact test.
The 18-item questionnaire was informed by constructs addressed in the 2015 Pew Research Center smartphone survey.10 However, in addition to device ownership, we inquired about device capabilities, service-plan details, service interruptions due to difficulty paying bills in the previous year, home-Internet access, an active e-mail account, and self-assigned demographics. Self-reported reading health literacy,11 more directly measured than e-health literacy, was screened using a parsimonious instrument validated as a dichotomized measure.12 Instruments in English and Spanish were tested for appropriate and comprehensible word choices and syntax through pilot testing. We inferred LEP among patients preferring to complete the survey in Spanish based on our familiarity with the population. We defined any Internet access as having a mobile data-service plan or having home-Internet access. In addition, we inquired about primary insurance provider and offered Medicaid patients an informational brochure about the federal Lifeline Program (https://www.fcc.gov/lifeline) that subsidizes text-messaging-enabled cellular telephone service for low-income patients. Notably, we assessed engagement by asking about the extent of patients’ interest in “new ways of communicating with your doctor, clinic, or pharmacy using” text, e-mail, or mobile apps with a 5-level response scale ranging from “not at all interested” to “very interested”.
Participant characteristics were confirmed to be similar to the Cook County Health and Hospitals System patient population in 2015 with regards to age, gender, and race/ethnicity. We calculated unadjusted and adjusted odds ratios for IRHL and LEP’s association with each dependent measure of access (to smartphone, Internet, or e-mail) and engagement (using text messaging, e-mail, or mobile apps) controlling for age, gender, primary payer, recruitment location, IRHL, and LEP. Because we oversampled inpatients, we estimated sampling-weight-adjusted proportions and 95% confidence intervals (CI) of the entire CCHHS patient population with access to smartphone, data/text plan, non-prepaid plan, and service interruptions using STATA v13 (StataCorp LP, College Station Texas). The project received a waiver upon review by the local Institutional Review Board.
RESULTS
Participation rate was 65% (302/464). Differences in patients by site are shown in Table 1. IRHL was more frequent and LEP less frequent among hospitalized patients. As shown in Table 2, patients with IRHL were less likely to have any Internet access, to have an active e-mail account, and to be interested in using e-mail for healthcare communications. Patients with LEP were less likely than English speakers to be interested in using mobile apps. Inpatients were less likely than outpatients to be interested in text messaging for healthcare communications.
The estimated proportion (95% CI) of the health system’s patients owning a text-enabled mobile device was 87% (75%-94%) and an Internet-enabled mobile device was 64% (47%-78%). The proportion with no data service interruptions in the previous year was 40% (31%-50%).
DISCUSSION
In this cross-section of urban low-income adult patients, IRHL and LEP were factors associated with potential disparities introduced by mHealth. Even as access to smartphones becomes ubiquitous, lagging access to Internet and e-mail among low literacy patients, and low levels of technology engagement for healthcare communications among patients with IRHL or LEP, underscore concerns about equity in health systems’ adoption of mHealth strategies. Hospitalized patients were found to have diminished engagement with mHealth independent of IRHL and LEP.
Regarding engagement, significantly fewer patients with IRHL or LEP were interested in using technology for healthcare communications. Our finding suggests that health disparities already associated with these conditions13 may not be reduced by mobile device outreach alone and may even be worsened by it. Touch screens, audio-enabled questionnaires, and language translation engines are innovations that may be helpful to mitigate IRHL and LEP, but evidence is scarce. Privacy and security concerns, and lack of experience with technology, may also lower engagement. A contemporaneous study found lower apps’ usage among Latinos, also suggesting that language concordance between apps, their source, and targeted users is important.14 Low-tech solutions involving mobile telephone or even lower tech in-person communications targeted to the estimated 26% of the US population with low literacy15 and 20% with LEP16 may be practical stopgap measures. Even as disparities in access to technology across race-ethnicity are diminishing,10 equity across poverty levels, low levels of education, cultural norms, and disabilities may be more challenging to overcome. Our assessment indicates that large exclusions of a safety-net population in 2015 are a legitimate concern in communication strategies that rely too heavily on mHealth. These findings underscore the CONSORT-EHEALTH recommendation that investigators report web-based recruitment strategies and data-collection methods comprehensively.17
Regarding access, our estimates suggest that historical disparities in smartphone ownership are diminishing, but access to Internet capabilities may still be lower among the urban poor compared to the nation as a whole. The Pew Research Center found that 64% of Americans owned a smartphone in 2015 (respondents defined smartphone).10 In comparison, 87% (95% CI, 75%, 94%) of our study participants owned a text-enabled mobile device and 64% (47%, 78%) owned an Internet-enabled mobile device. However, the 40% (31%, 50%) of our safety-net population with an uninterrupted data plan over the previous year may be lower than the 50% of Americans reporting uninterrupted data plans over their lifetime.10 The impact of expense-related data plan interruptions is magnified by the 40% of our study population—compared to 15% of Americans—who are dependent on mobile devices for Internet access.10 The association between Internet connectivity and literacy evokes multiple bidirectional pathways yet to be elucidated. But if mHealth can reduce health disparities, closing the gap in device ownership is only a partial accomplishment, and future work also needs to expand Internet connectivity to allow literacy-enhancing and literacy-naïve technologies to flourish.
This study has limitations. Our study population was a consecutive sample and participation rate was less than 100%. However, we recruited participants into the study the way we may also have approached patients to introduce mHealth options in our clinical settings. Our sampling method proved adequate for our primary goal to explain differences in technology access and engagement using regression analysis. Although our patient population may not directly generalize to many healthcare systems, including other safety-net systems serving regions with variable technology uptake,18 our findings reflect the capacities and the preferences of the most disadvantaged segments of urban populations. We systematically excluded LEP non-Spanish speakers, but they consisted of less than 5% of inpatients and no outpatients. We did not assess current technology use. Finally, as discussed earlier, access and use of new technologies change rapidly and frequent updates are necessary.
mHealth is a promising tool because it may increase healthcare access, improve care quality, and promote research. All these potential benefits will be obtained with accompanying efforts to reduce healthcare disparities, especially where some technologies themselves are exclusionary.
Disclosures
The authors report no financial conflicts of interest.
1. Khosravi P, Ghapanchi AH. Investigating the effectiveness of technologies applied to assist seniors: a systematic literature review. Int J Med Inform. 2016;85:17-26. PubMed
2. Feltner C, Jones CD, Cené CW, et al. Transitional care interventions to prevent readmissions for persons with heart failure: a systematic review and meta-analysis. Ann Intern Med. 2014;160:774-784. PubMed
3. Prieto-Centurion V, Gussin HA, Rolle AJ, Krishnan JA. Chronic obstructive pulmonary disease readmissions at minority-serving institutions. Ann Am Thorac Soc. 2013;10:680-684. PubMed
4. Hinami K, Smith J, Deamant CD, BuBeshter K, Trick WE. When do patient-reported outcome measures inform readmission risk? J Hosp Med. 2015;10:294-300. PubMed
5. Gibbons MC. A historical overview of health disparities and the potential of eHealth solutions. J Med Internet Res. 2005;7:e50. PubMed
6. Nelson LA, Mulvaney SA, Gebretsadik T, Ho YX, Johnson KB, Osborn CY. Disparities in the use of a mHealth medication adherence promotion intervention for low-income adults with type2 diabetes. J Am Med Inform Assoc. 2016;23:12-18. PubMed
7. Martin T. Assessing mHealth: opportunities and barriers to patient engagement. J Health Care Poor Underserved. 2012;23:935-941. PubMed
8. Horn IB, Mendoza FS. Reframing the disparities agenda: a time to rethink, a time to focus. Acad Pediatr. 2013;14:115-116. PubMed
9. Viswanath K, Nagler RH, Bigman-Galimore CA, McCauley MP, Jung M, Ramanadhan S. The communications revolution and health inequalities in the 21st century: implications for cancer control. Cancer Epidemiol, BiomarkersPrev. 2012;21:1701-1708. PubMed
10. Pew Research Center. The Smartphone Difference. Pew Research Center; April 2015. Available at: http://www.pewinternet.org/2015/04/01/us-smartphone-use-in-2015/. Accessed January 12, 2017.
11. Baker DW. The meaning and measure of health literacy. J Gen Intern Med. 2011;21:878-883. PubMed
12. Morris NS, MacLean CD, Chew LD, Littenberg B. The single item literacy screener: evalution of a brief instrument to identify limited reading ability. BMC Fam Pract. 2006;7:21. PubMed
13. Sentell T, Braun K. Low health literacy, limited English proficiency, and health status in Asians, Latinos, and other racial/ethnic groups in California. J Health Commun. 2012;17:82-99. PubMed
14. Arora S, Ford K, Terp S, et al. Describing the evolution of mobile technology usage for Latino patients and comparing findings to national mHealth estimates. J Am Med Inform Assoc. 2016;23:979-983. PubMed
15. Paasche-Orlow MK, Parker RM, Gazmararian JA, Nielsen-Bohlman LT, Rudd RR. The prevalence of limited health literacy. J Gen Intern Med. 2005;20:175-184. PubMed
16. US Census Bureau. Detailed languages spoken at home and ability to speak English for the population 5 years and over: 2009-2013. Published 2015. Available at: http://www.census.gov/data/tables/2013/demo/2009-2013-lang-tables.html. Accessed March 31, 2016.
17. Eysenbach G, CONSORT-EHEALTH Group. CONSORT-EHEALTH: improving and standardizing evaluation reports of Web-based and mobile health intervention. J Med Internet Res. 2011;13:e126. PubMed
18. Schickedanz A, Huang D, Lopex A, et al. Access, interest, and attitudes toward electronic communication for health care among patients in the medical safety net. J Gen Intern Med. 2013;28:914-920. PubMed
1. Khosravi P, Ghapanchi AH. Investigating the effectiveness of technologies applied to assist seniors: a systematic literature review. Int J Med Inform. 2016;85:17-26. PubMed
2. Feltner C, Jones CD, Cené CW, et al. Transitional care interventions to prevent readmissions for persons with heart failure: a systematic review and meta-analysis. Ann Intern Med. 2014;160:774-784. PubMed
3. Prieto-Centurion V, Gussin HA, Rolle AJ, Krishnan JA. Chronic obstructive pulmonary disease readmissions at minority-serving institutions. Ann Am Thorac Soc. 2013;10:680-684. PubMed
4. Hinami K, Smith J, Deamant CD, BuBeshter K, Trick WE. When do patient-reported outcome measures inform readmission risk? J Hosp Med. 2015;10:294-300. PubMed
5. Gibbons MC. A historical overview of health disparities and the potential of eHealth solutions. J Med Internet Res. 2005;7:e50. PubMed
6. Nelson LA, Mulvaney SA, Gebretsadik T, Ho YX, Johnson KB, Osborn CY. Disparities in the use of a mHealth medication adherence promotion intervention for low-income adults with type2 diabetes. J Am Med Inform Assoc. 2016;23:12-18. PubMed
7. Martin T. Assessing mHealth: opportunities and barriers to patient engagement. J Health Care Poor Underserved. 2012;23:935-941. PubMed
8. Horn IB, Mendoza FS. Reframing the disparities agenda: a time to rethink, a time to focus. Acad Pediatr. 2013;14:115-116. PubMed
9. Viswanath K, Nagler RH, Bigman-Galimore CA, McCauley MP, Jung M, Ramanadhan S. The communications revolution and health inequalities in the 21st century: implications for cancer control. Cancer Epidemiol, BiomarkersPrev. 2012;21:1701-1708. PubMed
10. Pew Research Center. The Smartphone Difference. Pew Research Center; April 2015. Available at: http://www.pewinternet.org/2015/04/01/us-smartphone-use-in-2015/. Accessed January 12, 2017.
11. Baker DW. The meaning and measure of health literacy. J Gen Intern Med. 2011;21:878-883. PubMed
12. Morris NS, MacLean CD, Chew LD, Littenberg B. The single item literacy screener: evalution of a brief instrument to identify limited reading ability. BMC Fam Pract. 2006;7:21. PubMed
13. Sentell T, Braun K. Low health literacy, limited English proficiency, and health status in Asians, Latinos, and other racial/ethnic groups in California. J Health Commun. 2012;17:82-99. PubMed
14. Arora S, Ford K, Terp S, et al. Describing the evolution of mobile technology usage for Latino patients and comparing findings to national mHealth estimates. J Am Med Inform Assoc. 2016;23:979-983. PubMed
15. Paasche-Orlow MK, Parker RM, Gazmararian JA, Nielsen-Bohlman LT, Rudd RR. The prevalence of limited health literacy. J Gen Intern Med. 2005;20:175-184. PubMed
16. US Census Bureau. Detailed languages spoken at home and ability to speak English for the population 5 years and over: 2009-2013. Published 2015. Available at: http://www.census.gov/data/tables/2013/demo/2009-2013-lang-tables.html. Accessed March 31, 2016.
17. Eysenbach G, CONSORT-EHEALTH Group. CONSORT-EHEALTH: improving and standardizing evaluation reports of Web-based and mobile health intervention. J Med Internet Res. 2011;13:e126. PubMed
18. Schickedanz A, Huang D, Lopex A, et al. Access, interest, and attitudes toward electronic communication for health care among patients in the medical safety net. J Gen Intern Med. 2013;28:914-920. PubMed
© 2017 Society of Hospital Medicine
Priorities and Gender Pay Gap
Hospitalists are a growing workforce numbering over 40,000 physicians, one‐third of whom are women.[1] Flexibility of work schedules and control over personal time have been the traditional selling points of the specialty.[2] Multiple studies of physician work life reveal growing physician dissatisfaction and a high prevalence of burnout.[3] To mitigate burnout risk, leaders in hospital medicine recognize the importance of creating a sustainable profession that offers both job and career satisfaction as well as work‐life balance and, importantly, fairness within the work environment.[4] Although success in some of these endeavors has been realized sporadically, sustaining work‐life balance and fairness in the specialty remains a work in progress, whereas evidence of high job attrition and pay inequities remain.[5, 6]
Pay inequity for women relative to men continues to be pervasive in medicine, including among early‐career physicians, researchers, and various specialists.[6, 7, 8, 9, 10, 11, 12, 13] The earnings gap seems to persist for physicians, even as federal efforts such as the Fair Pay Act of 2013 and the Paycheck Fairness Act of 2014 aim to end wage discrimination.[11, 14] Differences in specialty, part‐time status, and practice type do not mitigate the disparity.[8, 10, 15] Additional explanations have been proposed to explain the variability, including gender differences in negotiating skills, lack of opportunities to join networks of influence within organizations, and implicit or explicit bias and discrimination.[12, 16, 17, 18, 19, 20]
The earnings gap is also a consequence of what is commonly called the glass ceiling.[18, 19] Most agree that obstacles to fair advancement of women include absence of collaborative environments and role models who have successfully achieved work‐life balance.[17, 20, 21, 22] Somewhat surprisingly, women leaders in medicine seem to suffer greater income disparity than nonleaders; this income gap is prevalent among leaders in other elite professions as well.[7, 23] It is unknown whether women physicians' emphasis on work‐life balance, seen repeatedly in surveys, explains any of the pay disparity.[24] Little research to date has examined whether work‐life priorities of women in hospital medicine differ from men.
In this study, we sought to examine differences in job priorities between men and women hospitalists. In particular, we examine the relative prioritization of substantial pay to job satisfaction. We also examined gender differences in work patterns and earnings to explore potential sources of the persistent gender earnings gap.
METHODS
We analyzed data from the 20092010 Hospital Medicine Physician Worklife Survey, the design of which is detailed elsewhere.[4] Briefly, a 118‐item survey was administered by mail to a stratified sample of hospitalists from the Society of Hospital Medicine database and 3 large multisite hospitalist groups. A single survey item asked respondents to identify up to 4 out of 12 most important domains to their satisfaction with a hospitalist job. The domains were distilled from focus groups of nationally representative hospitalists as described previously,[4] and the survey item allowed up to one‐third of these domains to be identified as respondents' personal priorities. The list included: optimal variety of tasks, optimal workload, substantial pay, collegiality with other physicians, recognition by leaders, rewarding relationships with patients, satisfaction with nurses, optimal autonomy, control over personal time, fairness within organization, ample availability of resources to do job, and organizational climate of trust and belonging. We tabulated and ranked the frequency with which respondents selected each satisfaction domain by gender. Due to the nonstandard format of the survey item, we a priori decided to analyze only responses that were completed as instructed.
We also used demographic data including detailed work characteristics, clinical and nonclinical workload, total pretax earnings in 2009 as a hospitalist, and self‐identification as leader of their hospital medicine group. Respondent characteristics were tabulated and gender differences were tested using the t test, rank sum test, and the Fischer exact test as appropriate. We also listed the number of nonrespondents for each item. In estimating gender differences in earnings, we opted to use multiple‐imputation techniques to more conservatively account for greater variance inherent in the presence of missing data. Consistent with existing guidelines,[25] we demonstrated that item responses were not missing monotonically by visually inspecting patterns of nonresponse. We further demonstrated that data were missing at random by showing that response patterns of completed survey items did not predict whether or not a given variable response was missing using logistic regression models. We found no significant differences between respondents with complete and missing data. We verified that appropriate regression models for each variable on every other variable converged. We used Stata 13.1 (StataCorp, College Station, TX) to perform multiple imputations using chain equations (mi impute chain) to create 10 imputed tables for 7 normally distributed continuous variables using the ordinary least squares method, 3 non‐normally distributed variables using the predictive mean matching method, 2 nonordinal categorical variables using the multinomial logit method, and 1 binary variable using the logit method.[26, 27] Gender, pediatric specialty status, region of practice, and whether or not respondents prioritized substantial pay for job satisfaction were used as regular variables without missing data points.
Differences in earnings were assessed using a multivariate ordinary linear regression model applied to the imputed datasets fitted by forward selection of explanatory variables using P < 0.20 in bivariate analysis for inclusion and manual backward elimination of all statistically nonsignificant variables. We tested the significance of the women leader interaction term in the final parsimonious model. We used the usual significance threshold of P < 0.05 for inferences. Our analysis of publicly available anonymous data was exempt from IRB review.
RESULTS
Of the 816 survey respondents (response rate 25.6%), 40 either omitted the item soliciting work priorities or completed it incorrectly. Data from the remaining 776 respondents were used for the present analysis. Respondent characteristics are tabulated in Table 1. The characteristics of hospitalists by age, gender, specialty, practice model, and practice region were representative of US hospitalists from other surveys.[28]
Women | Men | P Value | No. of Missing Responses | |
---|---|---|---|---|
| ||||
No. | 263 | 513 | 0 | |
Role, n (%) | <0.01 | 0 | ||
Frontline hospitalist | 201 (76) | 337 (66) | ||
Hospitalist leader | 53 (24) | 176 (34) | ||
Age, y, mean (SD) | 42 (8) | 45 (9) | <0.01 | 67 |
Years in current job, mean (SD) | 5 (4) | 6 (5) | 0.07 | 14 |
Specialty, n (%) | <0.01 | 0 | ||
Internal medicine | 160 (61) | 369 (72) | ||
Pediatrics | 56 (21) | 57 (11) | ||
Other | 39 (15) | 47 (9) | ||
Family medicine | 8 (3) | 40 (8) | ||
Practice model, n (%) | 0.02 | 19 | ||
Hospital employed | 110 (43) | 227 (46) | ||
Multispecialty group | 44 (17) | 68 (14) | ||
University/medical school | 47 (18) | 58 (12) | ||
Multistate group | 27 (11) | 73 (15) | ||
Local hospitalist group | 22 (8) | 65 (13) | ||
Other | 7 (3) | 9 (2) | ||
Practice region, n (%) | 0.14 | 0 | ||
Southeast | 56 (21) | 151 (29) | ||
Midwest | 58 (22) | 106 (21) | ||
Northeast | 54 (21) | 96 (19) | ||
Southwest | 44 (17) | 83 (16) | ||
West | 51 (19) | 77 (15) | ||
Full‐time equivalents, n (%) | <0.01 | 42 | ||
<100% | 46 (18) | 60 (12) | ||
100% | 202 (81) | 402 (83) | ||
>100% | 2 (1) | 22 (5) | ||
Days per month doing clinical work if FTE 100%, median (IQR) | 15 (1418) | 16 (1420) | 0.12 | 11 |
Hours per day doing clinical work, median (IQR) | 11 (912) | 11 (912) | 0.67 | 30 |
Consecutive days doing clinical work, median (IQR) | 7 (57) | 7 (57) | 0.94 | 17 |
Percentage of work at night, median (IQR) | 15 (530) | 15 (525) | 0.45 | 16 |
Percentage of night work in hospital if working nights, median (IQR) | 100 (5100) | 100 (10100) | 0.12 | 8 |
Hours per month doing nonclinical work, median (IQR) | 12 (540) | 15 (540) | 0.77 | 26 |
Estimated daily billable encounters, mean (IQR) | 14 (1116) | 15 (1218) | 0.01 | 54 |
Total earnings in fiscal year 2009, median US$1,000 (IQR) | 185 (150210) | 202 (180240) | <0.01 | 56 |
Marriage/domestic partnership status, n (%) | 0.15 | 43 | ||
Married/currently in DP | 197 (80) | 421 (86) | ||
Never married/never in DP | 26 (11) | 42 (9) | ||
Divorced or separated | 18 (7) | 20 (4) | ||
Other | 4 (2) | 5 (1) | ||
Dependent children under 7 years old living in home, n (%) | 0.22 | 42 | ||
0 | 136 (55) | 265 (54) | ||
1 | 47 (19) | 92 (19) | ||
2 | 52 (21) | 87 (18) | ||
3 | 12 (5) | 43 (9) |
Several gender differences were seen in the characteristics of hospitalists and their work (Table 1). Women compared to men hospitalists were less likely to be leaders, more likely to be pediatricians, work in university settings, and practice in Western states. Women compared to men, on average, were younger by 3 years, worked fewer full‐time equivalents (FTEs), worked a greater percentage of nights, and reported fewer billable encounters per shift. They were also more likely to be divorced or separated.
Job satisfaction priorities differed for women and men hospitalists. Table 2 lists job satisfaction domains in descending order of the frequency prioritized by men. The largest proportion of women and men prioritized optimal workload. However, although substantial pay was prioritized next most frequently by men, more women prioritized collegiality and control over personal time than substantial pay.
Women, % | Rank | Men, % | Rank | |
---|---|---|---|---|
Optimal workload | 59 | 1 | 59 | 1 |
Substantial pay | 41 | 4 | 50 | 2 |
Control over personal time | 44 | 3 | 41 | 3 |
Collegiality with physicians | 47 | 2 | 38 | 4 |
Rewarding relationships with patients | 35 | 5 | 34 | 5 |
Organizational climate of trust and belonging | 27 | 7 | 33 | 6 |
Ample availability of resources to do job | 24 | 9 | 27 | 7 |
Optimal autonomy | 26 | 8 | 24 | 8 |
Fairness within organization | 15 | 10 | 23 | 9 |
Optimal variety of tasks | 29 | 6 | 22 | 10 |
Recognition by leaders | 11 | 12 | 10 | 11 |
Satisfaction with nurses | 12 | 11 | 7 | 12 |
Key differences in individual characteristics, work patterns, and indicating substantial pay as a priority were associated with self‐reported total earnings in 2009 from respondents' work as a hospitalist. As shown in Table 3, the inclusion of detailed productivity measures such as FTE, days of monthly clinical work, and estimated number of daily billable encounters yielded a model that explained 33% of variance in earnings. After adjusting for significant covariates including pediatric specialty, practice model, geography, and amount and type of clinical work, the estimated underpayment of women compared to men was $14,581. Hospitalists who prioritized substantial pay earned $10,771 more than those who did not. The female x leader interaction term testing the hypothesis that gender disparity is greater among leaders than frontline hospitalists was not statistically significant ($16,720, P = 0.087) and excluded from the final model.
Differences in Salary, 2009 US$ (95% CI) | P Value | |
---|---|---|
| ||
Women | 14,581 (23,074 to 6,089) | <0.01 |
Leader | 21,997 (13,313 to 30,682) | <0.01 |
Prioritized substantial pay | 10,771 (2,651 to 18,891) | <0.01 |
Pediatric specialty | 31,126 (43,007 to 19,244) | <0.01 |
Practice model | ||
Hospital employed | REF | |
Multispecialty group | 1,922 (13,891 to 10,047) | 0.75 |
University/medical school | 33,503 (46,336 to 20,671) | <0.01 |
Multistate group | 6,505 (72,69 to 20,279) | 0.35 |
Local hospitalist group | 9,330 (4,352 to 23,012) | 0.18 |
Other | 17,364 (45,741 to 11,012) | 0.23 |
Practice region | ||
Southeast | REF | |
Midwest | 1,225 (10,595 to 13,044) | 0.84 |
Northeast | 15,712 (28,182 to 3,242) | 0.01 |
Southwest | 722 (13,545 to 12,101) | 0.91 |
West | 5,251 (7,383 to 17,885) | 0.41 |
FTE | 1,021 (762 to 1,279) | <0.01 |
Days per month doing clinical work | 1,209 (443 to 1,975) | <0.01 |
Estimated daily billable encounters | 608 (20 to 1,196) | 0.04 |
DISCUSSION
In a national stratified sample of US hospitalists, we found gender differences in job satisfaction priorities and hospitalist work characteristics. We also confirmed the persistence of a substantial gender earnings disparity. Lower earnings among women compared to men hospitalists were present in our data after controlling for age, pediatric specialty, practice model, geography, type of clinical work, and productivity measures. The gender earnings disparity noted in 1999[6] persists, although it appears to have decreased, possibly indicating progress toward equity. We showed that women hospitalists' relative tendency not to prioritize pay explains a significant portion of the residual income gap.
Hoff examined hospitalist earnings in a large national survey of hospitalists in 1999. Our cohorts differed in age and experience (both lower in the Hoff study). An estimated $24,000 ($124,266 vs. $148,132) earnings gap between women and men was greater than our estimate of $14,581 following an interval of 10 years. Although survey items differed, both studies found that women were less interested in pay than men when considering a hospitalist job. Hoff also found that work setting and attitudes about pay and lifestyle were significantly related to earnings. We extended the previous analyses of gender differences in job satisfaction priorities, work, and demographic characteristics to explain the earnings gap and understand how it may be remedied.
When considering job satisfaction, we found that more men than women prioritized substantial pay and that prioritization of substantial pay was directly related to higher earnings. Therefore, fewer women prioritizing pay partly explains women's lower earnings. Reasons for why fewer women prioritize pay were not assessed in this study but may include factors like being part of 2‐income households and competing commitments.[29] Priorities may even be influenced by empirically observed gender differences in discussions of financial matters, governed by cultural norms. Such norms may implicitly sanction employers to offer women less pay than men for the same or similar work. Women may disadvantage themselves by negotiating less or less well than men for higher starting and promotion salaries. They may be perceived more negatively than men when they do negotiate pay, leading to unintended negative consequences such as loss of social networks, decreased likability, and even loss of job offers.[29, 30, 31, 32]
More women prioritized optimal variety of tasks (6th most prevalent among women and 10th among men). Women who highly rate optimal variety of tasks as a job satisfier may choose positions in which they teach, perform research, and participate in hospital committees and quality‐improvement work, but offer lower pay. Yet hours per month doing nonclinical work was not significantly different between men and women, nor associated with earnings differences in our earnings models. Understanding whether women self‐select into hospitalist jobs with like‐minded colleagues to achieve complementary fit or end up supplementing their skills with hospitalists with different priorities may inform strategies to reduce the gender income disparity.[5] Unlike disparities between various hospital medicine groups, systematic disparities within practices risk generating low levels of organizational fairness and burnout among employees.
Not surprisingly, productivity was positively associated with earnings but did not fully account for the gender earnings gap. Our data demonstrated that women, on average, were associated with work characteristics that expectedly generate less compensation. For example, women were younger, more often part time, academic, pediatric, less often leaders, and reported fewer billable encounters compared to men. These differences account for some of the earnings gap between men and women, but these factors were controlled for in the earnings model. In addition, our analysis may have underestimated the gap by not incorporating loss of fringe benefits from part‐time status and not comprehensively counting incentive pay associated with high productivity. Other work patterns more commonly associated with women suggest an imbalance in reimbursement. More women than men work nights that are often compensated at higher rates than daytime work, yet their average pay was less, suggesting that compensation for night work may need to be adjusted to reflect its unique burdens and responsibilities.[33]
Although the gender pay gap was not more extreme among leaders compared to frontline hospitalists in our data, the trend, nonetheless, underscores an important consideration. Whereas clinical work is paid for in mostly measurable ways, pay for leadership may be influenced by intangible factors such as reputation, negotiation, and confidence that may disadvantage women relative to men.[7, 19, 23, 34, 35, 36] Efforts to overcome implicit gender bias should be most effective when we consciously couple fair promotion of women to leadership with fair compensation commensurate with their male peers.[21]
Our data are vulnerable to nonresponse bias.[1, 4, 5] Post hoc analyses demonstrated that distributions of age, gender, practice model and region of our respondents were similar to other nationally representative cohorts of hospitalists. Consequently, we believe our data can make valid estimates about a nationally representative sample of hospitalists. However, we acknowledge several additional weaknesses of self‐reported data, including recall bias and accuracy of productivity figures, which were rounded to variable significant digits by respondents. Earnings analysis using this data was intended to be exploratory, but the findings echoed analyses using more authoritative data sources.[11] Still, we made inferences conservatively by adopting multiple imputation techniques for dealing with nonresponse surveys in adherence to established reporting guidelines.[25] We also note several limitations relevant to multiple imputations. The greater prevalence of missing data for survey items soliciting earnings and the number of billable encounters suggest they were not truly missing at random as assumed. However, we showed that missingness is unrelated to the variables under study, justifying use of the technique. The wider measures of variance derived from multiple imputations make us vulnerable to not detecting associations that may exist.
The gender earnings gap found in hospital medicine echoes the gap found in multiple medical specialties, including but not limited to pediatrics, academic medicine, gastroenterology, and plastic surgery.[7, 8, 9, 11, 12, 13, 37] Hospital medicine employment models and practice patterns have important structural differences compared to previously studied populations that could mitigate factors contributing to women physicians' lower earnings. However, despite well‐defined working hours, lack of control over the number of patient encounters per day and high prevalence of hospital‐employed practice models, the gender earnings gap persists. We showed that lower prioritization for pay may reflect the self‐selection of women into lower paying jobs. Unmeasured factors, including implicit bias and differences in negotiations, social networks and mentoring opportunities[38, 39] may also contribute to pay differences between men and women hospitalists. As hospital medicine tackles gender inequities and other disparities, strategies to assess and address fair physician compensation must be on the table.
- Society of Hospital Medicine Career Satisfaction Task F. Job characteristics, satisfaction, and burnout across hospitalist practice models. J Hosp Med. 2012;7(5):402–410. , , , , ;
- The emerging role of “hospitalists” in the American health care system. N Engl J Med. 1996;335(7):514–517. , .
- Organizational climate, stress, and error in primary care: The MEMO study. In: Henriksen K, Battles JB, Marks ES, Lewin DI, eds. Advances in Patient Safety: From Research to Implementation. Vol. 1. Research Findings. Rockville, MD; Agency for Healthcare Research and Quality; 2005. , , , et al.
- Worklife and satisfaction of hospitalists: toward flourishing careers. J Gen Intern Med. 2012;27(1):28–36. , , , , .
- Person‐job fit: an exploratory cross‐sectional analysis of hospitalists. J Hosp Med. 2013;8(2):96–101. , , , , .
- Doing the same and earning less: male and female physicians in a new medical specialty. Inquiry. 2004;41(3):301–315. .
- Compensation and advancement of women in academic medicine: Is there equity? Ann Intern Med. 2004;141(3):205–212. , , , .
- Differences in earnings between male and female physicians. N Engl J Med. 1996;334(15):960–964. .
- Gender differences in the salaries of physician researchers. JAMA. 2012;307(22):2410–2417. , , , , , .
- The work lives of women physicians results from the physician work life study. The SGIM Career Satisfaction Study Group. J Gen Intern Med. 2000;15(6):372–380. , , , , , .
- The $16,819 pay gap for newly trained physicians: the unexplained trend of men earning more than women. Health Aff (Millwood). 2011;30(2):193–201. , , , .
- Assessing gender equity in a large academic department of pediatrics. Acad Med. 2012;87(1):98–104. , , , .
- Gender disparity in the practice of gastroenterology: the first 5 years of a career. Am J Gastroenterol. 2005;100(2):259–264. , , , , , .
- H.R. 438, Fair Pay Act of 2013. 113th Congress (2013‐2014).
- Topics to ponder: part‐time practice and pay parity. Gend Med. 2010;7(4):350–356. , , , , .
- Understanding and finding mentorship: a review for junior faculty. J Palliat Med. 2010;13(11):1373–1379. , .
- Career development for women in academic medicine: Multiple interventions in a department of medicine. JAMA. 1996;276(11):898–905. , , , et al.
- Sex differences in academic advancement. Results of a national study of pediatricians. N Engl J Med. 1996;335(17):1282–1289. , , , , , .
- Promotion of women physicians in academic medicine. Glass ceiling or sticky floor? JAMA. 1995;273(13):1022–1025. , , , .
- Stories from early‐career women physicians who have left academic medicine: a qualitative study at a single institution. Acad Med. 2011;86(6):752–758. , , , , .
- Experiencing the culture of academic medicine: gender matters, a national study. J Gen Intern Med. 2013;28(2):201–207. , , , , .
- Why aren't there more women leaders in academic medicine? tHe views of clinical department chairs. Acad Med. 2001;76(5):453–465. , .
- The gender gap in executive compensation: the role of female directors and chief executive officers. Ann Am Acad Pol Soc Sci. 2012(639):258–278. .
- Keys to career satisfaction: insights from a survey of women pediatric surgeons. J Pediatr Surg. 2004;39(6):984–990. , , .
- Multiple imputation for missing data in epidemiological and clinical research: potential and pitfalls. BMJ. 2009;338:b2393. , , , et al.
- Imputation and Variance Estimation Software [computer program]. Ann Arbor, MI: Universtiy of Michigan; 2007.
- Multiple Imputation using chained equations: issues and guidance for practice. Stat Med. 2010;30(4):377–399. , , .
- State of Hospital Medicine: 2010 Report Based on 2009 Data. Englewood, CO and Philadelphia, PA: Medical Group Management Association and Society of Hospital Medicine; 2010.
- The Changing Face of Medicine: Women Doctors and the Evolution Of Health Care in America. Ithaca, NY: ILR Press/Cornell University Press; 2008. , .
- Women Don't Ask: Negotiation and the Gender Divide. Princeton, NJ: Princeton University Press; 2003. , .
- Negotiation in academic medicine: a necessary career skill. J Womens Health (Larchmt). 2007;16(2):235–244. , , , et al.
- Women and salary negotiation: the costs of self‐advocacy. Psychol Women Q. 2001;25:65–76. .
- State of Hospital Medicine: 2014 Report Based on 2013 Data. Englewood, CO and Philadelphia, PA: Medical Group Management Association and Society of Hospital Medicine; 2014.
- Interventions that affect gender bias in hiring: a systematic review. Acad Med. 2009;84(10):1440–1446. , , .
- Sociology. The gender gap in NIH grant applications. Science. 2008;322(5907):1472–1474. , .
- Organizational climate and family life: how these factors affect the status of women faculty at one medical school. Acad Med. 2009;84(1):87–94. , , , .
- Gender differences in the professional and private lives of plastic surgeons. Ann Plast Surg. 2010;64(6):775–779. , , .
- Gender and supportive co‐worker relations in the medical profession. Gend Work Organ. 2014;21(1):1–17. .
- Stuck in the out‐group: Jennifer can't grow up, Jane's invisible, and Janet's over the hill. J Womens Health (Larchmt). 2014;23(6):481–484. , .
Hospitalists are a growing workforce numbering over 40,000 physicians, one‐third of whom are women.[1] Flexibility of work schedules and control over personal time have been the traditional selling points of the specialty.[2] Multiple studies of physician work life reveal growing physician dissatisfaction and a high prevalence of burnout.[3] To mitigate burnout risk, leaders in hospital medicine recognize the importance of creating a sustainable profession that offers both job and career satisfaction as well as work‐life balance and, importantly, fairness within the work environment.[4] Although success in some of these endeavors has been realized sporadically, sustaining work‐life balance and fairness in the specialty remains a work in progress, whereas evidence of high job attrition and pay inequities remain.[5, 6]
Pay inequity for women relative to men continues to be pervasive in medicine, including among early‐career physicians, researchers, and various specialists.[6, 7, 8, 9, 10, 11, 12, 13] The earnings gap seems to persist for physicians, even as federal efforts such as the Fair Pay Act of 2013 and the Paycheck Fairness Act of 2014 aim to end wage discrimination.[11, 14] Differences in specialty, part‐time status, and practice type do not mitigate the disparity.[8, 10, 15] Additional explanations have been proposed to explain the variability, including gender differences in negotiating skills, lack of opportunities to join networks of influence within organizations, and implicit or explicit bias and discrimination.[12, 16, 17, 18, 19, 20]
The earnings gap is also a consequence of what is commonly called the glass ceiling.[18, 19] Most agree that obstacles to fair advancement of women include absence of collaborative environments and role models who have successfully achieved work‐life balance.[17, 20, 21, 22] Somewhat surprisingly, women leaders in medicine seem to suffer greater income disparity than nonleaders; this income gap is prevalent among leaders in other elite professions as well.[7, 23] It is unknown whether women physicians' emphasis on work‐life balance, seen repeatedly in surveys, explains any of the pay disparity.[24] Little research to date has examined whether work‐life priorities of women in hospital medicine differ from men.
In this study, we sought to examine differences in job priorities between men and women hospitalists. In particular, we examine the relative prioritization of substantial pay to job satisfaction. We also examined gender differences in work patterns and earnings to explore potential sources of the persistent gender earnings gap.
METHODS
We analyzed data from the 20092010 Hospital Medicine Physician Worklife Survey, the design of which is detailed elsewhere.[4] Briefly, a 118‐item survey was administered by mail to a stratified sample of hospitalists from the Society of Hospital Medicine database and 3 large multisite hospitalist groups. A single survey item asked respondents to identify up to 4 out of 12 most important domains to their satisfaction with a hospitalist job. The domains were distilled from focus groups of nationally representative hospitalists as described previously,[4] and the survey item allowed up to one‐third of these domains to be identified as respondents' personal priorities. The list included: optimal variety of tasks, optimal workload, substantial pay, collegiality with other physicians, recognition by leaders, rewarding relationships with patients, satisfaction with nurses, optimal autonomy, control over personal time, fairness within organization, ample availability of resources to do job, and organizational climate of trust and belonging. We tabulated and ranked the frequency with which respondents selected each satisfaction domain by gender. Due to the nonstandard format of the survey item, we a priori decided to analyze only responses that were completed as instructed.
We also used demographic data including detailed work characteristics, clinical and nonclinical workload, total pretax earnings in 2009 as a hospitalist, and self‐identification as leader of their hospital medicine group. Respondent characteristics were tabulated and gender differences were tested using the t test, rank sum test, and the Fischer exact test as appropriate. We also listed the number of nonrespondents for each item. In estimating gender differences in earnings, we opted to use multiple‐imputation techniques to more conservatively account for greater variance inherent in the presence of missing data. Consistent with existing guidelines,[25] we demonstrated that item responses were not missing monotonically by visually inspecting patterns of nonresponse. We further demonstrated that data were missing at random by showing that response patterns of completed survey items did not predict whether or not a given variable response was missing using logistic regression models. We found no significant differences between respondents with complete and missing data. We verified that appropriate regression models for each variable on every other variable converged. We used Stata 13.1 (StataCorp, College Station, TX) to perform multiple imputations using chain equations (mi impute chain) to create 10 imputed tables for 7 normally distributed continuous variables using the ordinary least squares method, 3 non‐normally distributed variables using the predictive mean matching method, 2 nonordinal categorical variables using the multinomial logit method, and 1 binary variable using the logit method.[26, 27] Gender, pediatric specialty status, region of practice, and whether or not respondents prioritized substantial pay for job satisfaction were used as regular variables without missing data points.
Differences in earnings were assessed using a multivariate ordinary linear regression model applied to the imputed datasets fitted by forward selection of explanatory variables using P < 0.20 in bivariate analysis for inclusion and manual backward elimination of all statistically nonsignificant variables. We tested the significance of the women leader interaction term in the final parsimonious model. We used the usual significance threshold of P < 0.05 for inferences. Our analysis of publicly available anonymous data was exempt from IRB review.
RESULTS
Of the 816 survey respondents (response rate 25.6%), 40 either omitted the item soliciting work priorities or completed it incorrectly. Data from the remaining 776 respondents were used for the present analysis. Respondent characteristics are tabulated in Table 1. The characteristics of hospitalists by age, gender, specialty, practice model, and practice region were representative of US hospitalists from other surveys.[28]
Women | Men | P Value | No. of Missing Responses | |
---|---|---|---|---|
| ||||
No. | 263 | 513 | 0 | |
Role, n (%) | <0.01 | 0 | ||
Frontline hospitalist | 201 (76) | 337 (66) | ||
Hospitalist leader | 53 (24) | 176 (34) | ||
Age, y, mean (SD) | 42 (8) | 45 (9) | <0.01 | 67 |
Years in current job, mean (SD) | 5 (4) | 6 (5) | 0.07 | 14 |
Specialty, n (%) | <0.01 | 0 | ||
Internal medicine | 160 (61) | 369 (72) | ||
Pediatrics | 56 (21) | 57 (11) | ||
Other | 39 (15) | 47 (9) | ||
Family medicine | 8 (3) | 40 (8) | ||
Practice model, n (%) | 0.02 | 19 | ||
Hospital employed | 110 (43) | 227 (46) | ||
Multispecialty group | 44 (17) | 68 (14) | ||
University/medical school | 47 (18) | 58 (12) | ||
Multistate group | 27 (11) | 73 (15) | ||
Local hospitalist group | 22 (8) | 65 (13) | ||
Other | 7 (3) | 9 (2) | ||
Practice region, n (%) | 0.14 | 0 | ||
Southeast | 56 (21) | 151 (29) | ||
Midwest | 58 (22) | 106 (21) | ||
Northeast | 54 (21) | 96 (19) | ||
Southwest | 44 (17) | 83 (16) | ||
West | 51 (19) | 77 (15) | ||
Full‐time equivalents, n (%) | <0.01 | 42 | ||
<100% | 46 (18) | 60 (12) | ||
100% | 202 (81) | 402 (83) | ||
>100% | 2 (1) | 22 (5) | ||
Days per month doing clinical work if FTE 100%, median (IQR) | 15 (1418) | 16 (1420) | 0.12 | 11 |
Hours per day doing clinical work, median (IQR) | 11 (912) | 11 (912) | 0.67 | 30 |
Consecutive days doing clinical work, median (IQR) | 7 (57) | 7 (57) | 0.94 | 17 |
Percentage of work at night, median (IQR) | 15 (530) | 15 (525) | 0.45 | 16 |
Percentage of night work in hospital if working nights, median (IQR) | 100 (5100) | 100 (10100) | 0.12 | 8 |
Hours per month doing nonclinical work, median (IQR) | 12 (540) | 15 (540) | 0.77 | 26 |
Estimated daily billable encounters, mean (IQR) | 14 (1116) | 15 (1218) | 0.01 | 54 |
Total earnings in fiscal year 2009, median US$1,000 (IQR) | 185 (150210) | 202 (180240) | <0.01 | 56 |
Marriage/domestic partnership status, n (%) | 0.15 | 43 | ||
Married/currently in DP | 197 (80) | 421 (86) | ||
Never married/never in DP | 26 (11) | 42 (9) | ||
Divorced or separated | 18 (7) | 20 (4) | ||
Other | 4 (2) | 5 (1) | ||
Dependent children under 7 years old living in home, n (%) | 0.22 | 42 | ||
0 | 136 (55) | 265 (54) | ||
1 | 47 (19) | 92 (19) | ||
2 | 52 (21) | 87 (18) | ||
3 | 12 (5) | 43 (9) |
Several gender differences were seen in the characteristics of hospitalists and their work (Table 1). Women compared to men hospitalists were less likely to be leaders, more likely to be pediatricians, work in university settings, and practice in Western states. Women compared to men, on average, were younger by 3 years, worked fewer full‐time equivalents (FTEs), worked a greater percentage of nights, and reported fewer billable encounters per shift. They were also more likely to be divorced or separated.
Job satisfaction priorities differed for women and men hospitalists. Table 2 lists job satisfaction domains in descending order of the frequency prioritized by men. The largest proportion of women and men prioritized optimal workload. However, although substantial pay was prioritized next most frequently by men, more women prioritized collegiality and control over personal time than substantial pay.
Women, % | Rank | Men, % | Rank | |
---|---|---|---|---|
Optimal workload | 59 | 1 | 59 | 1 |
Substantial pay | 41 | 4 | 50 | 2 |
Control over personal time | 44 | 3 | 41 | 3 |
Collegiality with physicians | 47 | 2 | 38 | 4 |
Rewarding relationships with patients | 35 | 5 | 34 | 5 |
Organizational climate of trust and belonging | 27 | 7 | 33 | 6 |
Ample availability of resources to do job | 24 | 9 | 27 | 7 |
Optimal autonomy | 26 | 8 | 24 | 8 |
Fairness within organization | 15 | 10 | 23 | 9 |
Optimal variety of tasks | 29 | 6 | 22 | 10 |
Recognition by leaders | 11 | 12 | 10 | 11 |
Satisfaction with nurses | 12 | 11 | 7 | 12 |
Key differences in individual characteristics, work patterns, and indicating substantial pay as a priority were associated with self‐reported total earnings in 2009 from respondents' work as a hospitalist. As shown in Table 3, the inclusion of detailed productivity measures such as FTE, days of monthly clinical work, and estimated number of daily billable encounters yielded a model that explained 33% of variance in earnings. After adjusting for significant covariates including pediatric specialty, practice model, geography, and amount and type of clinical work, the estimated underpayment of women compared to men was $14,581. Hospitalists who prioritized substantial pay earned $10,771 more than those who did not. The female x leader interaction term testing the hypothesis that gender disparity is greater among leaders than frontline hospitalists was not statistically significant ($16,720, P = 0.087) and excluded from the final model.
Differences in Salary, 2009 US$ (95% CI) | P Value | |
---|---|---|
| ||
Women | 14,581 (23,074 to 6,089) | <0.01 |
Leader | 21,997 (13,313 to 30,682) | <0.01 |
Prioritized substantial pay | 10,771 (2,651 to 18,891) | <0.01 |
Pediatric specialty | 31,126 (43,007 to 19,244) | <0.01 |
Practice model | ||
Hospital employed | REF | |
Multispecialty group | 1,922 (13,891 to 10,047) | 0.75 |
University/medical school | 33,503 (46,336 to 20,671) | <0.01 |
Multistate group | 6,505 (72,69 to 20,279) | 0.35 |
Local hospitalist group | 9,330 (4,352 to 23,012) | 0.18 |
Other | 17,364 (45,741 to 11,012) | 0.23 |
Practice region | ||
Southeast | REF | |
Midwest | 1,225 (10,595 to 13,044) | 0.84 |
Northeast | 15,712 (28,182 to 3,242) | 0.01 |
Southwest | 722 (13,545 to 12,101) | 0.91 |
West | 5,251 (7,383 to 17,885) | 0.41 |
FTE | 1,021 (762 to 1,279) | <0.01 |
Days per month doing clinical work | 1,209 (443 to 1,975) | <0.01 |
Estimated daily billable encounters | 608 (20 to 1,196) | 0.04 |
DISCUSSION
In a national stratified sample of US hospitalists, we found gender differences in job satisfaction priorities and hospitalist work characteristics. We also confirmed the persistence of a substantial gender earnings disparity. Lower earnings among women compared to men hospitalists were present in our data after controlling for age, pediatric specialty, practice model, geography, type of clinical work, and productivity measures. The gender earnings disparity noted in 1999[6] persists, although it appears to have decreased, possibly indicating progress toward equity. We showed that women hospitalists' relative tendency not to prioritize pay explains a significant portion of the residual income gap.
Hoff examined hospitalist earnings in a large national survey of hospitalists in 1999. Our cohorts differed in age and experience (both lower in the Hoff study). An estimated $24,000 ($124,266 vs. $148,132) earnings gap between women and men was greater than our estimate of $14,581 following an interval of 10 years. Although survey items differed, both studies found that women were less interested in pay than men when considering a hospitalist job. Hoff also found that work setting and attitudes about pay and lifestyle were significantly related to earnings. We extended the previous analyses of gender differences in job satisfaction priorities, work, and demographic characteristics to explain the earnings gap and understand how it may be remedied.
When considering job satisfaction, we found that more men than women prioritized substantial pay and that prioritization of substantial pay was directly related to higher earnings. Therefore, fewer women prioritizing pay partly explains women's lower earnings. Reasons for why fewer women prioritize pay were not assessed in this study but may include factors like being part of 2‐income households and competing commitments.[29] Priorities may even be influenced by empirically observed gender differences in discussions of financial matters, governed by cultural norms. Such norms may implicitly sanction employers to offer women less pay than men for the same or similar work. Women may disadvantage themselves by negotiating less or less well than men for higher starting and promotion salaries. They may be perceived more negatively than men when they do negotiate pay, leading to unintended negative consequences such as loss of social networks, decreased likability, and even loss of job offers.[29, 30, 31, 32]
More women prioritized optimal variety of tasks (6th most prevalent among women and 10th among men). Women who highly rate optimal variety of tasks as a job satisfier may choose positions in which they teach, perform research, and participate in hospital committees and quality‐improvement work, but offer lower pay. Yet hours per month doing nonclinical work was not significantly different between men and women, nor associated with earnings differences in our earnings models. Understanding whether women self‐select into hospitalist jobs with like‐minded colleagues to achieve complementary fit or end up supplementing their skills with hospitalists with different priorities may inform strategies to reduce the gender income disparity.[5] Unlike disparities between various hospital medicine groups, systematic disparities within practices risk generating low levels of organizational fairness and burnout among employees.
Not surprisingly, productivity was positively associated with earnings but did not fully account for the gender earnings gap. Our data demonstrated that women, on average, were associated with work characteristics that expectedly generate less compensation. For example, women were younger, more often part time, academic, pediatric, less often leaders, and reported fewer billable encounters compared to men. These differences account for some of the earnings gap between men and women, but these factors were controlled for in the earnings model. In addition, our analysis may have underestimated the gap by not incorporating loss of fringe benefits from part‐time status and not comprehensively counting incentive pay associated with high productivity. Other work patterns more commonly associated with women suggest an imbalance in reimbursement. More women than men work nights that are often compensated at higher rates than daytime work, yet their average pay was less, suggesting that compensation for night work may need to be adjusted to reflect its unique burdens and responsibilities.[33]
Although the gender pay gap was not more extreme among leaders compared to frontline hospitalists in our data, the trend, nonetheless, underscores an important consideration. Whereas clinical work is paid for in mostly measurable ways, pay for leadership may be influenced by intangible factors such as reputation, negotiation, and confidence that may disadvantage women relative to men.[7, 19, 23, 34, 35, 36] Efforts to overcome implicit gender bias should be most effective when we consciously couple fair promotion of women to leadership with fair compensation commensurate with their male peers.[21]
Our data are vulnerable to nonresponse bias.[1, 4, 5] Post hoc analyses demonstrated that distributions of age, gender, practice model and region of our respondents were similar to other nationally representative cohorts of hospitalists. Consequently, we believe our data can make valid estimates about a nationally representative sample of hospitalists. However, we acknowledge several additional weaknesses of self‐reported data, including recall bias and accuracy of productivity figures, which were rounded to variable significant digits by respondents. Earnings analysis using this data was intended to be exploratory, but the findings echoed analyses using more authoritative data sources.[11] Still, we made inferences conservatively by adopting multiple imputation techniques for dealing with nonresponse surveys in adherence to established reporting guidelines.[25] We also note several limitations relevant to multiple imputations. The greater prevalence of missing data for survey items soliciting earnings and the number of billable encounters suggest they were not truly missing at random as assumed. However, we showed that missingness is unrelated to the variables under study, justifying use of the technique. The wider measures of variance derived from multiple imputations make us vulnerable to not detecting associations that may exist.
The gender earnings gap found in hospital medicine echoes the gap found in multiple medical specialties, including but not limited to pediatrics, academic medicine, gastroenterology, and plastic surgery.[7, 8, 9, 11, 12, 13, 37] Hospital medicine employment models and practice patterns have important structural differences compared to previously studied populations that could mitigate factors contributing to women physicians' lower earnings. However, despite well‐defined working hours, lack of control over the number of patient encounters per day and high prevalence of hospital‐employed practice models, the gender earnings gap persists. We showed that lower prioritization for pay may reflect the self‐selection of women into lower paying jobs. Unmeasured factors, including implicit bias and differences in negotiations, social networks and mentoring opportunities[38, 39] may also contribute to pay differences between men and women hospitalists. As hospital medicine tackles gender inequities and other disparities, strategies to assess and address fair physician compensation must be on the table.
Hospitalists are a growing workforce numbering over 40,000 physicians, one‐third of whom are women.[1] Flexibility of work schedules and control over personal time have been the traditional selling points of the specialty.[2] Multiple studies of physician work life reveal growing physician dissatisfaction and a high prevalence of burnout.[3] To mitigate burnout risk, leaders in hospital medicine recognize the importance of creating a sustainable profession that offers both job and career satisfaction as well as work‐life balance and, importantly, fairness within the work environment.[4] Although success in some of these endeavors has been realized sporadically, sustaining work‐life balance and fairness in the specialty remains a work in progress, whereas evidence of high job attrition and pay inequities remain.[5, 6]
Pay inequity for women relative to men continues to be pervasive in medicine, including among early‐career physicians, researchers, and various specialists.[6, 7, 8, 9, 10, 11, 12, 13] The earnings gap seems to persist for physicians, even as federal efforts such as the Fair Pay Act of 2013 and the Paycheck Fairness Act of 2014 aim to end wage discrimination.[11, 14] Differences in specialty, part‐time status, and practice type do not mitigate the disparity.[8, 10, 15] Additional explanations have been proposed to explain the variability, including gender differences in negotiating skills, lack of opportunities to join networks of influence within organizations, and implicit or explicit bias and discrimination.[12, 16, 17, 18, 19, 20]
The earnings gap is also a consequence of what is commonly called the glass ceiling.[18, 19] Most agree that obstacles to fair advancement of women include absence of collaborative environments and role models who have successfully achieved work‐life balance.[17, 20, 21, 22] Somewhat surprisingly, women leaders in medicine seem to suffer greater income disparity than nonleaders; this income gap is prevalent among leaders in other elite professions as well.[7, 23] It is unknown whether women physicians' emphasis on work‐life balance, seen repeatedly in surveys, explains any of the pay disparity.[24] Little research to date has examined whether work‐life priorities of women in hospital medicine differ from men.
In this study, we sought to examine differences in job priorities between men and women hospitalists. In particular, we examine the relative prioritization of substantial pay to job satisfaction. We also examined gender differences in work patterns and earnings to explore potential sources of the persistent gender earnings gap.
METHODS
We analyzed data from the 20092010 Hospital Medicine Physician Worklife Survey, the design of which is detailed elsewhere.[4] Briefly, a 118‐item survey was administered by mail to a stratified sample of hospitalists from the Society of Hospital Medicine database and 3 large multisite hospitalist groups. A single survey item asked respondents to identify up to 4 out of 12 most important domains to their satisfaction with a hospitalist job. The domains were distilled from focus groups of nationally representative hospitalists as described previously,[4] and the survey item allowed up to one‐third of these domains to be identified as respondents' personal priorities. The list included: optimal variety of tasks, optimal workload, substantial pay, collegiality with other physicians, recognition by leaders, rewarding relationships with patients, satisfaction with nurses, optimal autonomy, control over personal time, fairness within organization, ample availability of resources to do job, and organizational climate of trust and belonging. We tabulated and ranked the frequency with which respondents selected each satisfaction domain by gender. Due to the nonstandard format of the survey item, we a priori decided to analyze only responses that were completed as instructed.
We also used demographic data including detailed work characteristics, clinical and nonclinical workload, total pretax earnings in 2009 as a hospitalist, and self‐identification as leader of their hospital medicine group. Respondent characteristics were tabulated and gender differences were tested using the t test, rank sum test, and the Fischer exact test as appropriate. We also listed the number of nonrespondents for each item. In estimating gender differences in earnings, we opted to use multiple‐imputation techniques to more conservatively account for greater variance inherent in the presence of missing data. Consistent with existing guidelines,[25] we demonstrated that item responses were not missing monotonically by visually inspecting patterns of nonresponse. We further demonstrated that data were missing at random by showing that response patterns of completed survey items did not predict whether or not a given variable response was missing using logistic regression models. We found no significant differences between respondents with complete and missing data. We verified that appropriate regression models for each variable on every other variable converged. We used Stata 13.1 (StataCorp, College Station, TX) to perform multiple imputations using chain equations (mi impute chain) to create 10 imputed tables for 7 normally distributed continuous variables using the ordinary least squares method, 3 non‐normally distributed variables using the predictive mean matching method, 2 nonordinal categorical variables using the multinomial logit method, and 1 binary variable using the logit method.[26, 27] Gender, pediatric specialty status, region of practice, and whether or not respondents prioritized substantial pay for job satisfaction were used as regular variables without missing data points.
Differences in earnings were assessed using a multivariate ordinary linear regression model applied to the imputed datasets fitted by forward selection of explanatory variables using P < 0.20 in bivariate analysis for inclusion and manual backward elimination of all statistically nonsignificant variables. We tested the significance of the women leader interaction term in the final parsimonious model. We used the usual significance threshold of P < 0.05 for inferences. Our analysis of publicly available anonymous data was exempt from IRB review.
RESULTS
Of the 816 survey respondents (response rate 25.6%), 40 either omitted the item soliciting work priorities or completed it incorrectly. Data from the remaining 776 respondents were used for the present analysis. Respondent characteristics are tabulated in Table 1. The characteristics of hospitalists by age, gender, specialty, practice model, and practice region were representative of US hospitalists from other surveys.[28]
Women | Men | P Value | No. of Missing Responses | |
---|---|---|---|---|
| ||||
No. | 263 | 513 | 0 | |
Role, n (%) | <0.01 | 0 | ||
Frontline hospitalist | 201 (76) | 337 (66) | ||
Hospitalist leader | 53 (24) | 176 (34) | ||
Age, y, mean (SD) | 42 (8) | 45 (9) | <0.01 | 67 |
Years in current job, mean (SD) | 5 (4) | 6 (5) | 0.07 | 14 |
Specialty, n (%) | <0.01 | 0 | ||
Internal medicine | 160 (61) | 369 (72) | ||
Pediatrics | 56 (21) | 57 (11) | ||
Other | 39 (15) | 47 (9) | ||
Family medicine | 8 (3) | 40 (8) | ||
Practice model, n (%) | 0.02 | 19 | ||
Hospital employed | 110 (43) | 227 (46) | ||
Multispecialty group | 44 (17) | 68 (14) | ||
University/medical school | 47 (18) | 58 (12) | ||
Multistate group | 27 (11) | 73 (15) | ||
Local hospitalist group | 22 (8) | 65 (13) | ||
Other | 7 (3) | 9 (2) | ||
Practice region, n (%) | 0.14 | 0 | ||
Southeast | 56 (21) | 151 (29) | ||
Midwest | 58 (22) | 106 (21) | ||
Northeast | 54 (21) | 96 (19) | ||
Southwest | 44 (17) | 83 (16) | ||
West | 51 (19) | 77 (15) | ||
Full‐time equivalents, n (%) | <0.01 | 42 | ||
<100% | 46 (18) | 60 (12) | ||
100% | 202 (81) | 402 (83) | ||
>100% | 2 (1) | 22 (5) | ||
Days per month doing clinical work if FTE 100%, median (IQR) | 15 (1418) | 16 (1420) | 0.12 | 11 |
Hours per day doing clinical work, median (IQR) | 11 (912) | 11 (912) | 0.67 | 30 |
Consecutive days doing clinical work, median (IQR) | 7 (57) | 7 (57) | 0.94 | 17 |
Percentage of work at night, median (IQR) | 15 (530) | 15 (525) | 0.45 | 16 |
Percentage of night work in hospital if working nights, median (IQR) | 100 (5100) | 100 (10100) | 0.12 | 8 |
Hours per month doing nonclinical work, median (IQR) | 12 (540) | 15 (540) | 0.77 | 26 |
Estimated daily billable encounters, mean (IQR) | 14 (1116) | 15 (1218) | 0.01 | 54 |
Total earnings in fiscal year 2009, median US$1,000 (IQR) | 185 (150210) | 202 (180240) | <0.01 | 56 |
Marriage/domestic partnership status, n (%) | 0.15 | 43 | ||
Married/currently in DP | 197 (80) | 421 (86) | ||
Never married/never in DP | 26 (11) | 42 (9) | ||
Divorced or separated | 18 (7) | 20 (4) | ||
Other | 4 (2) | 5 (1) | ||
Dependent children under 7 years old living in home, n (%) | 0.22 | 42 | ||
0 | 136 (55) | 265 (54) | ||
1 | 47 (19) | 92 (19) | ||
2 | 52 (21) | 87 (18) | ||
3 | 12 (5) | 43 (9) |
Several gender differences were seen in the characteristics of hospitalists and their work (Table 1). Women compared to men hospitalists were less likely to be leaders, more likely to be pediatricians, work in university settings, and practice in Western states. Women compared to men, on average, were younger by 3 years, worked fewer full‐time equivalents (FTEs), worked a greater percentage of nights, and reported fewer billable encounters per shift. They were also more likely to be divorced or separated.
Job satisfaction priorities differed for women and men hospitalists. Table 2 lists job satisfaction domains in descending order of the frequency prioritized by men. The largest proportion of women and men prioritized optimal workload. However, although substantial pay was prioritized next most frequently by men, more women prioritized collegiality and control over personal time than substantial pay.
Women, % | Rank | Men, % | Rank | |
---|---|---|---|---|
Optimal workload | 59 | 1 | 59 | 1 |
Substantial pay | 41 | 4 | 50 | 2 |
Control over personal time | 44 | 3 | 41 | 3 |
Collegiality with physicians | 47 | 2 | 38 | 4 |
Rewarding relationships with patients | 35 | 5 | 34 | 5 |
Organizational climate of trust and belonging | 27 | 7 | 33 | 6 |
Ample availability of resources to do job | 24 | 9 | 27 | 7 |
Optimal autonomy | 26 | 8 | 24 | 8 |
Fairness within organization | 15 | 10 | 23 | 9 |
Optimal variety of tasks | 29 | 6 | 22 | 10 |
Recognition by leaders | 11 | 12 | 10 | 11 |
Satisfaction with nurses | 12 | 11 | 7 | 12 |
Key differences in individual characteristics, work patterns, and indicating substantial pay as a priority were associated with self‐reported total earnings in 2009 from respondents' work as a hospitalist. As shown in Table 3, the inclusion of detailed productivity measures such as FTE, days of monthly clinical work, and estimated number of daily billable encounters yielded a model that explained 33% of variance in earnings. After adjusting for significant covariates including pediatric specialty, practice model, geography, and amount and type of clinical work, the estimated underpayment of women compared to men was $14,581. Hospitalists who prioritized substantial pay earned $10,771 more than those who did not. The female x leader interaction term testing the hypothesis that gender disparity is greater among leaders than frontline hospitalists was not statistically significant ($16,720, P = 0.087) and excluded from the final model.
Differences in Salary, 2009 US$ (95% CI) | P Value | |
---|---|---|
| ||
Women | 14,581 (23,074 to 6,089) | <0.01 |
Leader | 21,997 (13,313 to 30,682) | <0.01 |
Prioritized substantial pay | 10,771 (2,651 to 18,891) | <0.01 |
Pediatric specialty | 31,126 (43,007 to 19,244) | <0.01 |
Practice model | ||
Hospital employed | REF | |
Multispecialty group | 1,922 (13,891 to 10,047) | 0.75 |
University/medical school | 33,503 (46,336 to 20,671) | <0.01 |
Multistate group | 6,505 (72,69 to 20,279) | 0.35 |
Local hospitalist group | 9,330 (4,352 to 23,012) | 0.18 |
Other | 17,364 (45,741 to 11,012) | 0.23 |
Practice region | ||
Southeast | REF | |
Midwest | 1,225 (10,595 to 13,044) | 0.84 |
Northeast | 15,712 (28,182 to 3,242) | 0.01 |
Southwest | 722 (13,545 to 12,101) | 0.91 |
West | 5,251 (7,383 to 17,885) | 0.41 |
FTE | 1,021 (762 to 1,279) | <0.01 |
Days per month doing clinical work | 1,209 (443 to 1,975) | <0.01 |
Estimated daily billable encounters | 608 (20 to 1,196) | 0.04 |
DISCUSSION
In a national stratified sample of US hospitalists, we found gender differences in job satisfaction priorities and hospitalist work characteristics. We also confirmed the persistence of a substantial gender earnings disparity. Lower earnings among women compared to men hospitalists were present in our data after controlling for age, pediatric specialty, practice model, geography, type of clinical work, and productivity measures. The gender earnings disparity noted in 1999[6] persists, although it appears to have decreased, possibly indicating progress toward equity. We showed that women hospitalists' relative tendency not to prioritize pay explains a significant portion of the residual income gap.
Hoff examined hospitalist earnings in a large national survey of hospitalists in 1999. Our cohorts differed in age and experience (both lower in the Hoff study). An estimated $24,000 ($124,266 vs. $148,132) earnings gap between women and men was greater than our estimate of $14,581 following an interval of 10 years. Although survey items differed, both studies found that women were less interested in pay than men when considering a hospitalist job. Hoff also found that work setting and attitudes about pay and lifestyle were significantly related to earnings. We extended the previous analyses of gender differences in job satisfaction priorities, work, and demographic characteristics to explain the earnings gap and understand how it may be remedied.
When considering job satisfaction, we found that more men than women prioritized substantial pay and that prioritization of substantial pay was directly related to higher earnings. Therefore, fewer women prioritizing pay partly explains women's lower earnings. Reasons for why fewer women prioritize pay were not assessed in this study but may include factors like being part of 2‐income households and competing commitments.[29] Priorities may even be influenced by empirically observed gender differences in discussions of financial matters, governed by cultural norms. Such norms may implicitly sanction employers to offer women less pay than men for the same or similar work. Women may disadvantage themselves by negotiating less or less well than men for higher starting and promotion salaries. They may be perceived more negatively than men when they do negotiate pay, leading to unintended negative consequences such as loss of social networks, decreased likability, and even loss of job offers.[29, 30, 31, 32]
More women prioritized optimal variety of tasks (6th most prevalent among women and 10th among men). Women who highly rate optimal variety of tasks as a job satisfier may choose positions in which they teach, perform research, and participate in hospital committees and quality‐improvement work, but offer lower pay. Yet hours per month doing nonclinical work was not significantly different between men and women, nor associated with earnings differences in our earnings models. Understanding whether women self‐select into hospitalist jobs with like‐minded colleagues to achieve complementary fit or end up supplementing their skills with hospitalists with different priorities may inform strategies to reduce the gender income disparity.[5] Unlike disparities between various hospital medicine groups, systematic disparities within practices risk generating low levels of organizational fairness and burnout among employees.
Not surprisingly, productivity was positively associated with earnings but did not fully account for the gender earnings gap. Our data demonstrated that women, on average, were associated with work characteristics that expectedly generate less compensation. For example, women were younger, more often part time, academic, pediatric, less often leaders, and reported fewer billable encounters compared to men. These differences account for some of the earnings gap between men and women, but these factors were controlled for in the earnings model. In addition, our analysis may have underestimated the gap by not incorporating loss of fringe benefits from part‐time status and not comprehensively counting incentive pay associated with high productivity. Other work patterns more commonly associated with women suggest an imbalance in reimbursement. More women than men work nights that are often compensated at higher rates than daytime work, yet their average pay was less, suggesting that compensation for night work may need to be adjusted to reflect its unique burdens and responsibilities.[33]
Although the gender pay gap was not more extreme among leaders compared to frontline hospitalists in our data, the trend, nonetheless, underscores an important consideration. Whereas clinical work is paid for in mostly measurable ways, pay for leadership may be influenced by intangible factors such as reputation, negotiation, and confidence that may disadvantage women relative to men.[7, 19, 23, 34, 35, 36] Efforts to overcome implicit gender bias should be most effective when we consciously couple fair promotion of women to leadership with fair compensation commensurate with their male peers.[21]
Our data are vulnerable to nonresponse bias.[1, 4, 5] Post hoc analyses demonstrated that distributions of age, gender, practice model and region of our respondents were similar to other nationally representative cohorts of hospitalists. Consequently, we believe our data can make valid estimates about a nationally representative sample of hospitalists. However, we acknowledge several additional weaknesses of self‐reported data, including recall bias and accuracy of productivity figures, which were rounded to variable significant digits by respondents. Earnings analysis using this data was intended to be exploratory, but the findings echoed analyses using more authoritative data sources.[11] Still, we made inferences conservatively by adopting multiple imputation techniques for dealing with nonresponse surveys in adherence to established reporting guidelines.[25] We also note several limitations relevant to multiple imputations. The greater prevalence of missing data for survey items soliciting earnings and the number of billable encounters suggest they were not truly missing at random as assumed. However, we showed that missingness is unrelated to the variables under study, justifying use of the technique. The wider measures of variance derived from multiple imputations make us vulnerable to not detecting associations that may exist.
The gender earnings gap found in hospital medicine echoes the gap found in multiple medical specialties, including but not limited to pediatrics, academic medicine, gastroenterology, and plastic surgery.[7, 8, 9, 11, 12, 13, 37] Hospital medicine employment models and practice patterns have important structural differences compared to previously studied populations that could mitigate factors contributing to women physicians' lower earnings. However, despite well‐defined working hours, lack of control over the number of patient encounters per day and high prevalence of hospital‐employed practice models, the gender earnings gap persists. We showed that lower prioritization for pay may reflect the self‐selection of women into lower paying jobs. Unmeasured factors, including implicit bias and differences in negotiations, social networks and mentoring opportunities[38, 39] may also contribute to pay differences between men and women hospitalists. As hospital medicine tackles gender inequities and other disparities, strategies to assess and address fair physician compensation must be on the table.
- Society of Hospital Medicine Career Satisfaction Task F. Job characteristics, satisfaction, and burnout across hospitalist practice models. J Hosp Med. 2012;7(5):402–410. , , , , ;
- The emerging role of “hospitalists” in the American health care system. N Engl J Med. 1996;335(7):514–517. , .
- Organizational climate, stress, and error in primary care: The MEMO study. In: Henriksen K, Battles JB, Marks ES, Lewin DI, eds. Advances in Patient Safety: From Research to Implementation. Vol. 1. Research Findings. Rockville, MD; Agency for Healthcare Research and Quality; 2005. , , , et al.
- Worklife and satisfaction of hospitalists: toward flourishing careers. J Gen Intern Med. 2012;27(1):28–36. , , , , .
- Person‐job fit: an exploratory cross‐sectional analysis of hospitalists. J Hosp Med. 2013;8(2):96–101. , , , , .
- Doing the same and earning less: male and female physicians in a new medical specialty. Inquiry. 2004;41(3):301–315. .
- Compensation and advancement of women in academic medicine: Is there equity? Ann Intern Med. 2004;141(3):205–212. , , , .
- Differences in earnings between male and female physicians. N Engl J Med. 1996;334(15):960–964. .
- Gender differences in the salaries of physician researchers. JAMA. 2012;307(22):2410–2417. , , , , , .
- The work lives of women physicians results from the physician work life study. The SGIM Career Satisfaction Study Group. J Gen Intern Med. 2000;15(6):372–380. , , , , , .
- The $16,819 pay gap for newly trained physicians: the unexplained trend of men earning more than women. Health Aff (Millwood). 2011;30(2):193–201. , , , .
- Assessing gender equity in a large academic department of pediatrics. Acad Med. 2012;87(1):98–104. , , , .
- Gender disparity in the practice of gastroenterology: the first 5 years of a career. Am J Gastroenterol. 2005;100(2):259–264. , , , , , .
- H.R. 438, Fair Pay Act of 2013. 113th Congress (2013‐2014).
- Topics to ponder: part‐time practice and pay parity. Gend Med. 2010;7(4):350–356. , , , , .
- Understanding and finding mentorship: a review for junior faculty. J Palliat Med. 2010;13(11):1373–1379. , .
- Career development for women in academic medicine: Multiple interventions in a department of medicine. JAMA. 1996;276(11):898–905. , , , et al.
- Sex differences in academic advancement. Results of a national study of pediatricians. N Engl J Med. 1996;335(17):1282–1289. , , , , , .
- Promotion of women physicians in academic medicine. Glass ceiling or sticky floor? JAMA. 1995;273(13):1022–1025. , , , .
- Stories from early‐career women physicians who have left academic medicine: a qualitative study at a single institution. Acad Med. 2011;86(6):752–758. , , , , .
- Experiencing the culture of academic medicine: gender matters, a national study. J Gen Intern Med. 2013;28(2):201–207. , , , , .
- Why aren't there more women leaders in academic medicine? tHe views of clinical department chairs. Acad Med. 2001;76(5):453–465. , .
- The gender gap in executive compensation: the role of female directors and chief executive officers. Ann Am Acad Pol Soc Sci. 2012(639):258–278. .
- Keys to career satisfaction: insights from a survey of women pediatric surgeons. J Pediatr Surg. 2004;39(6):984–990. , , .
- Multiple imputation for missing data in epidemiological and clinical research: potential and pitfalls. BMJ. 2009;338:b2393. , , , et al.
- Imputation and Variance Estimation Software [computer program]. Ann Arbor, MI: Universtiy of Michigan; 2007.
- Multiple Imputation using chained equations: issues and guidance for practice. Stat Med. 2010;30(4):377–399. , , .
- State of Hospital Medicine: 2010 Report Based on 2009 Data. Englewood, CO and Philadelphia, PA: Medical Group Management Association and Society of Hospital Medicine; 2010.
- The Changing Face of Medicine: Women Doctors and the Evolution Of Health Care in America. Ithaca, NY: ILR Press/Cornell University Press; 2008. , .
- Women Don't Ask: Negotiation and the Gender Divide. Princeton, NJ: Princeton University Press; 2003. , .
- Negotiation in academic medicine: a necessary career skill. J Womens Health (Larchmt). 2007;16(2):235–244. , , , et al.
- Women and salary negotiation: the costs of self‐advocacy. Psychol Women Q. 2001;25:65–76. .
- State of Hospital Medicine: 2014 Report Based on 2013 Data. Englewood, CO and Philadelphia, PA: Medical Group Management Association and Society of Hospital Medicine; 2014.
- Interventions that affect gender bias in hiring: a systematic review. Acad Med. 2009;84(10):1440–1446. , , .
- Sociology. The gender gap in NIH grant applications. Science. 2008;322(5907):1472–1474. , .
- Organizational climate and family life: how these factors affect the status of women faculty at one medical school. Acad Med. 2009;84(1):87–94. , , , .
- Gender differences in the professional and private lives of plastic surgeons. Ann Plast Surg. 2010;64(6):775–779. , , .
- Gender and supportive co‐worker relations in the medical profession. Gend Work Organ. 2014;21(1):1–17. .
- Stuck in the out‐group: Jennifer can't grow up, Jane's invisible, and Janet's over the hill. J Womens Health (Larchmt). 2014;23(6):481–484. , .
- Society of Hospital Medicine Career Satisfaction Task F. Job characteristics, satisfaction, and burnout across hospitalist practice models. J Hosp Med. 2012;7(5):402–410. , , , , ;
- The emerging role of “hospitalists” in the American health care system. N Engl J Med. 1996;335(7):514–517. , .
- Organizational climate, stress, and error in primary care: The MEMO study. In: Henriksen K, Battles JB, Marks ES, Lewin DI, eds. Advances in Patient Safety: From Research to Implementation. Vol. 1. Research Findings. Rockville, MD; Agency for Healthcare Research and Quality; 2005. , , , et al.
- Worklife and satisfaction of hospitalists: toward flourishing careers. J Gen Intern Med. 2012;27(1):28–36. , , , , .
- Person‐job fit: an exploratory cross‐sectional analysis of hospitalists. J Hosp Med. 2013;8(2):96–101. , , , , .
- Doing the same and earning less: male and female physicians in a new medical specialty. Inquiry. 2004;41(3):301–315. .
- Compensation and advancement of women in academic medicine: Is there equity? Ann Intern Med. 2004;141(3):205–212. , , , .
- Differences in earnings between male and female physicians. N Engl J Med. 1996;334(15):960–964. .
- Gender differences in the salaries of physician researchers. JAMA. 2012;307(22):2410–2417. , , , , , .
- The work lives of women physicians results from the physician work life study. The SGIM Career Satisfaction Study Group. J Gen Intern Med. 2000;15(6):372–380. , , , , , .
- The $16,819 pay gap for newly trained physicians: the unexplained trend of men earning more than women. Health Aff (Millwood). 2011;30(2):193–201. , , , .
- Assessing gender equity in a large academic department of pediatrics. Acad Med. 2012;87(1):98–104. , , , .
- Gender disparity in the practice of gastroenterology: the first 5 years of a career. Am J Gastroenterol. 2005;100(2):259–264. , , , , , .
- H.R. 438, Fair Pay Act of 2013. 113th Congress (2013‐2014).
- Topics to ponder: part‐time practice and pay parity. Gend Med. 2010;7(4):350–356. , , , , .
- Understanding and finding mentorship: a review for junior faculty. J Palliat Med. 2010;13(11):1373–1379. , .
- Career development for women in academic medicine: Multiple interventions in a department of medicine. JAMA. 1996;276(11):898–905. , , , et al.
- Sex differences in academic advancement. Results of a national study of pediatricians. N Engl J Med. 1996;335(17):1282–1289. , , , , , .
- Promotion of women physicians in academic medicine. Glass ceiling or sticky floor? JAMA. 1995;273(13):1022–1025. , , , .
- Stories from early‐career women physicians who have left academic medicine: a qualitative study at a single institution. Acad Med. 2011;86(6):752–758. , , , , .
- Experiencing the culture of academic medicine: gender matters, a national study. J Gen Intern Med. 2013;28(2):201–207. , , , , .
- Why aren't there more women leaders in academic medicine? tHe views of clinical department chairs. Acad Med. 2001;76(5):453–465. , .
- The gender gap in executive compensation: the role of female directors and chief executive officers. Ann Am Acad Pol Soc Sci. 2012(639):258–278. .
- Keys to career satisfaction: insights from a survey of women pediatric surgeons. J Pediatr Surg. 2004;39(6):984–990. , , .
- Multiple imputation for missing data in epidemiological and clinical research: potential and pitfalls. BMJ. 2009;338:b2393. , , , et al.
- Imputation and Variance Estimation Software [computer program]. Ann Arbor, MI: Universtiy of Michigan; 2007.
- Multiple Imputation using chained equations: issues and guidance for practice. Stat Med. 2010;30(4):377–399. , , .
- State of Hospital Medicine: 2010 Report Based on 2009 Data. Englewood, CO and Philadelphia, PA: Medical Group Management Association and Society of Hospital Medicine; 2010.
- The Changing Face of Medicine: Women Doctors and the Evolution Of Health Care in America. Ithaca, NY: ILR Press/Cornell University Press; 2008. , .
- Women Don't Ask: Negotiation and the Gender Divide. Princeton, NJ: Princeton University Press; 2003. , .
- Negotiation in academic medicine: a necessary career skill. J Womens Health (Larchmt). 2007;16(2):235–244. , , , et al.
- Women and salary negotiation: the costs of self‐advocacy. Psychol Women Q. 2001;25:65–76. .
- State of Hospital Medicine: 2014 Report Based on 2013 Data. Englewood, CO and Philadelphia, PA: Medical Group Management Association and Society of Hospital Medicine; 2014.
- Interventions that affect gender bias in hiring: a systematic review. Acad Med. 2009;84(10):1440–1446. , , .
- Sociology. The gender gap in NIH grant applications. Science. 2008;322(5907):1472–1474. , .
- Organizational climate and family life: how these factors affect the status of women faculty at one medical school. Acad Med. 2009;84(1):87–94. , , , .
- Gender differences in the professional and private lives of plastic surgeons. Ann Plast Surg. 2010;64(6):775–779. , , .
- Gender and supportive co‐worker relations in the medical profession. Gend Work Organ. 2014;21(1):1–17. .
- Stuck in the out‐group: Jennifer can't grow up, Jane's invisible, and Janet's over the hill. J Womens Health (Larchmt). 2014;23(6):481–484. , .
© 2015 Society of Hospital Medicine
Patient‐Reported Outcome Measures
Despite widespread efforts to predict 30‐day rehospitalizations among discharged general medical patients,[1, 2, 3] not many strategies have incorporated patient‐reported outcome (PRO) measures in predictive models.[4] This despite the many longitudinal studies of the ambulatory population that demonstrate the higher likelihood of hospitalizations among those who score poorly on General Self‐Rated Health (GSRH),[5, 6, 7] baseline or declining Health‐Related Quality of Life,[8, 9, 10, 11, 12] psychological symptoms,[13, 14] and physical symptoms assessments.[15] One of the few existing studies that included PRO measures in 30‐day readmission models showed the predictive value of the 12‐item short form (SF12) Physical Component Score.[16] Others showed that persistent symptoms were associated with readmissions in patients with heart disease.[17, 18]
The paucity of efforts to connect PRO measures to utilization may be due to the limited availability of these measures in routine clinical records and the incomplete knowledge about how various PRO measures may fluctuate during episodes of acute illnesses and their treatments during hospitalizations. Health perception measures reflect both enduring features like self‐concept as well as dynamic features like a person's immediate health status.[19] As such, GSRH reflects the presence of chronic illnesses but is also responsive to acute events.[20, 21] Similarly, Health‐Related Quality of Life measures are dynamic as they decline around episodes of acute illness but are stable over a longer time window in their tendency to recover.[22] We do not know how fluctuations in measures of symptom burden, perceived health, and quality of life around the hospital‐to‐home transition may differentially inform readmission risk. Using a longitudinal cohort study, we addressed 2 questions: (1) How do PRO measures change when measured serially during the hospital‐to‐home transition? (2) How does the relative timing of each PRO measure variably inform the risk of subsequent utilization events including hospital readmissions?
METHODS
We conducted a longitudinal cohort study using data originally collected for a trial (
The following instruments were administered at each interview. The physical symptom severity portion of the Memorial Symptom Assessment Scale (MSAS) solicited the severity rank (none/a little bit/somewhat/quite a bit/very much) of 17 physical symptoms in the last week; the score was calculated by averaging the severity rank of the 12 most common symptom in the sample.[25, 26] The Patient Reported Outcomes Measurement Information System (PROMIS) Global Health Short Form is an instrument assessing GSRH (1 item), Social Activities (1 item), Global Physical Health (4 items), and Global Mental Health (4 items including a single‐item quality‐of‐life measure). Fatigue and pain for Global Physical Health, and emotional health for Global Mental Health were assessed over the past 7 days. Each of the 2 Global Health scores was standardized to a national mean of 50 and standard deviation of 10.[27]
The rate of survey completion at each follow‐up was calculated. Characteristics of participants were tabulated. Characteristics of patients censored prior to study completion were compared with patients with complete data. Box plots for MSAS physical symptom severity, and Global Physical and Mental Health scores were constructed to illustrate the comparisons of the mean scores between each consecutive survey period using t tests assuming unequal variance. A similar box plot of GSRH illustrated the comparison of the median score between consecutive surveys using the rank sum test. Hospital‐based utilization events were defined as either an emergency department visit or hospitalization at 1 of the 2 hospitals of the Cook County Health & Hospitals System (CCHHS). After accounting for patient data censored due to death (date reported by family) or withdrawal from study, Kaplan‐Meier curves showing time to first hospital‐based utilization event during each interval between surveys were drawn separately for above‐ and below‐median MSAS, Global Physical and Mental Health scores, and for poor or fair versus good, very good, or excellent GSRH assessment. The null hypothesis that the survivor functions were equal between the better and worse median quantiles or GSRH categories was tested using the log‐rank test at 14 and 30 days from survey completion. Hazard ratios for time to first utilization event within 14 days of each survey were calculated for the MSAS score, Global Physical and Mental Health as continuous variables, and GSRH response categories relative to poor using bivariate and multivariate Cox proportional hazard equations. Multivariate models incorporated the following 5 covariates: at least 1 utilization event during the study period prior to the survey, Charlson score, age, gender, and race/ethnicity category. Likelihood ratio statistics were calculated to test the hypothesis that the model including the PRO measure and covariates predicted the outcome equally well compared to the nested model with only covariates. We used the traditional threshold of .05 when reporting significance. All analyses were performed in Stata 13 (StataCorp, College Station, TX). The methods for patient consent, data collection, analyses, and reporting were reviewed and approved by the CCHHS institutional review board.
RESULTS
A total of 196 patients completed the initial survey. The completion rates were 98%, 90%, and 88% for the 30‐, 90‐, and 180‐day follow‐up surveys, respectively. As shown in Table 1, participants average age was 52 years, and about half were women. The majority was non‐Hispanic black, and 21% preferred to complete the survey in Spanish. Diabetes, congestive heart failure, cancer, and chronic pulmonary disease were each prevalent in at least one‐fifth of our patient cohort. Demographic characteristics were similar between the 160 patients who completed all 3 follow‐up surveys and the 36 who missed at least 1 follow‐up survey. Among the latter group, 1 withdrew at 30 days, 1 withdrew and 4 had died at 90 days, and 1 withdrew and 9 had died at 180 days.
| |
Age, y, mean (SD) | 52 (10) |
Female, n (%) | 100 (51) |
Race/ethnicity category, n (%) | |
Non‐Hispanic black | 117 (60) |
Hispanic | 52 (27) |
Non‐Hispanic white | 20 (10) |
Other | 6 (3) |
Language, n (%) | |
English | 155 (79) |
Spanish | 41 (21) |
Charlson Comorbidity Index, median (range) | 1 (09) |
Charlson comorbidities, n (%) | |
Diabetes | 71 (36) |
Congestive heart failure | 52 (27) |
Cancer (with and without metastases) | 43 (22) |
Chronic pulmonary disease | 40 (20) |
Myocardial infarction | 17 (9) |
Renal disease | 11 (6) |
Figure 1 shows a timeline of the follow‐up surveys and utilization events in the form of overlapping histograms. The majority of 30‐day follow‐up questionnaires were completed earlier than targeted, at a median of 17 (interquartile range [IQR] 16, 20) days after discharge. Similarly, questionnaires targeted for 90 and 180 days were completed at medians of 78 (IQR 7684) and 167 (IQR 166169) days from discharge. Fifty‐four (28%) patients experienced a first utilization event in the first 30 days following discharge. During the 60‐, 90‐, and 30‐day intervals after the first, second, and third follow‐up surveys, respectively, 63 (33%), 54 (31%), and 16 (9%) patients experienced a first utilization event.
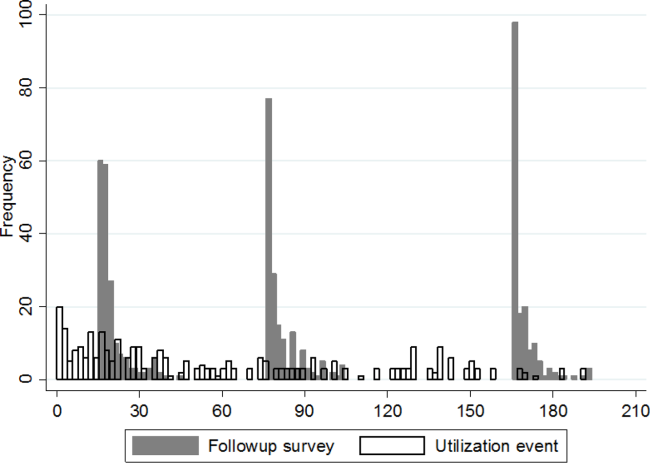
A significant improvement in MSAS physical symptom severity was detected between the hospitalization and the 30‐day follow‐up (Figure 2A). Although the mean Global Physical Health score was below the national mean of 50 at every survey period, a similar improvement in the measure was noted between the hospitalization and the 30‐day follow‐up (Figure 2B). The mean Global Mental Health score was also below the national mean but remained stable throughout the study (Figure 2C). The median GSRH was stable at 2 (IQR 23) at every survey wave (Figure 2D). Of note, compared to patients who completed all 3 follow‐up surveys, patients who missed at least 1 follow‐up reported higher MSAS score (1.5 vs 1.8, P=0.03), lower Global Physical Health (36.1 vs 33.5, P=0.09), and lower Global Mental Health (44.7 vs 41.0, P=0.03) during their hospitalization. In addition, patients with complete data experienced an average of 1.2 utilization events during the study, whereas those with missing data experienced an average of 2.1 utilization events (P=0.03).
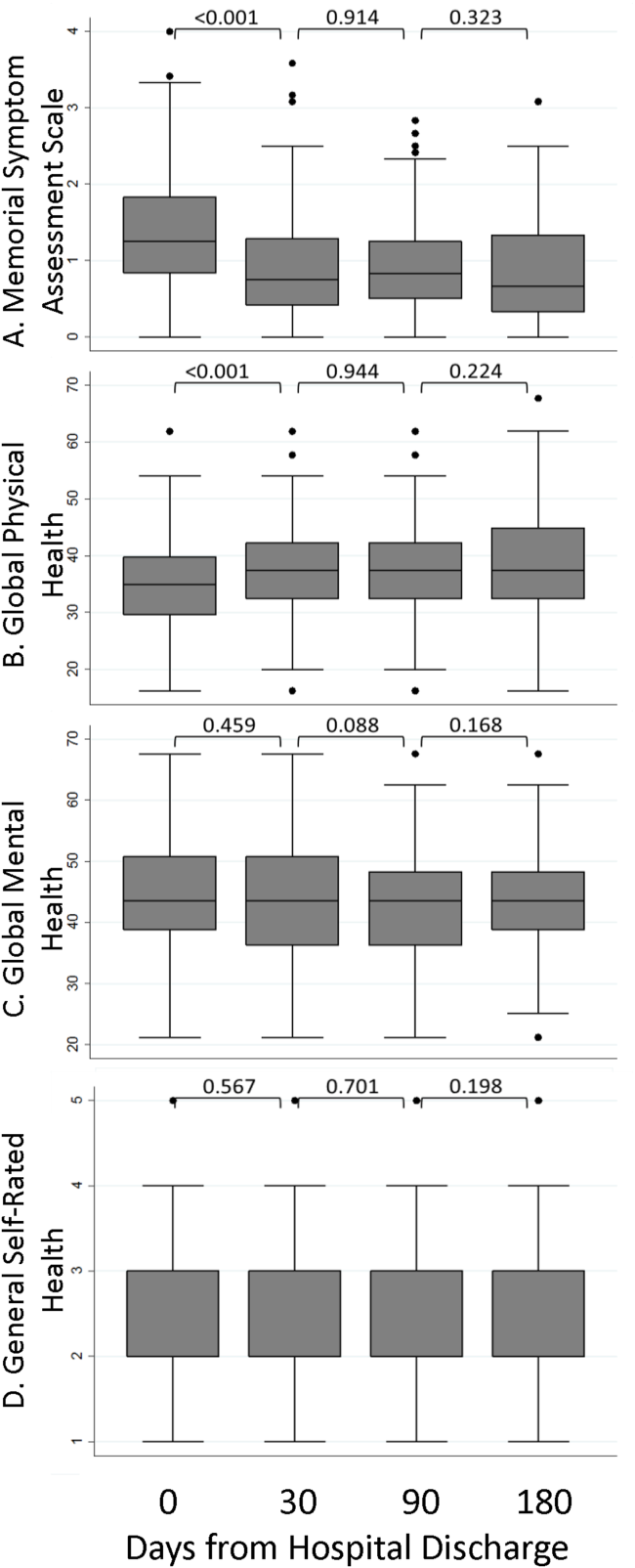
The MSAS physical symptom severity and Global Physical Health scores from the index hospitalizations did not identify patients with a first utilization event within 30 days. However, patients with poor Global Mental Health and GSRH in the hospital were more likely to experience a utilization event within 14 days of discharge (Figure 3). During the postdischarge period, patients scoring poorly on each of the PRO measures trended toward a greater risk of an early utilization event, but the association between utilization and MSAS was most consistently significant (Figure 3A). In general, the associations with MSAS, Global Physical Health, and GSRH were stronger with the risk of utilization events within 14 days than within 30 days (Figure 3A,B,D). The Global Mental Health score was not associated with a subsequent utilization when measured during the 180‐day postdischarge period.
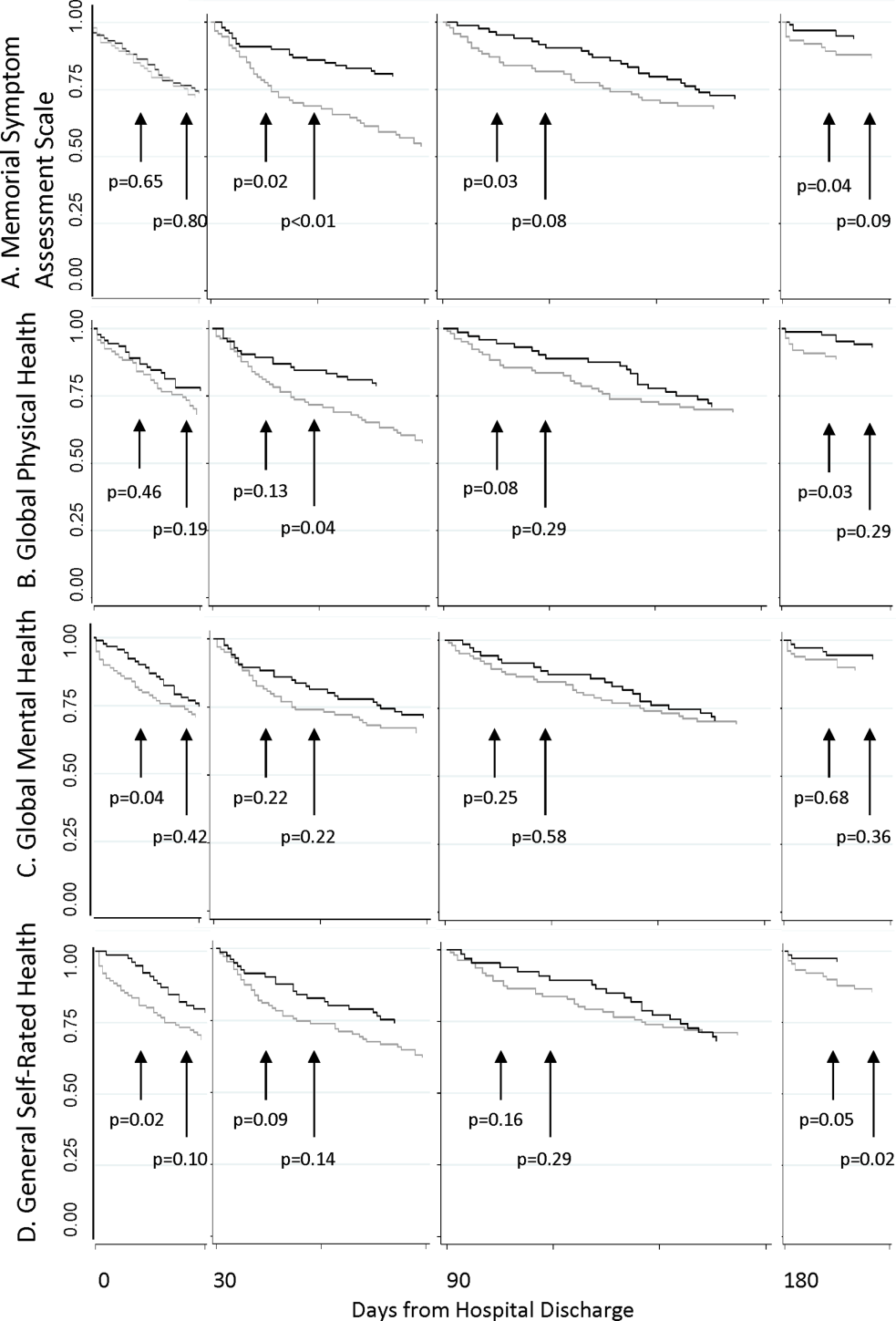
As shown in Table 2, Cox proportional hazard models incorporating covariates preserved most of the significant associations seen in the unadjusted analyses. Global Mental Health and good relative to poor GSRH obtained during the hospitalization remained significant. MSAS obtained at each postdischarge follow‐up trended positively with utilization and was statistically significant at 90 and 180 days. Global Physical Health obtained at each postdischarge follow‐up similarly trended negatively with utilization and was significant at 180 days. Each multivariate model incorporating a PRO measure with a significant coefficient contributed to better fit of the predictive model compared to the nested model without the PRO measure.
Unadjusted Hazard Ratio | P | Adjusted Hazard Ratio* | P | Likelihood Ratio | P | |
---|---|---|---|---|---|---|
| ||||||
Hospital discharge | ||||||
MSAS | 1.47 | 0.11 | 1.38 | 0.19 | 1.65 | 0.20 |
Global Physical Health | 0.96 | 0.10 | 0.96 | 0.13 | 2.29 | 0.13 |
Global Mental Health | 0.96 | 0.05 | 0.96 | 0.05 | 4.05 | 0.04 |
GSRH | ||||||
Fair | 1.09 | 0.85 | 1.26 | 0.61 | 12.27 | 0.02 |
Good | 0.24 | 0.04 | 0.23 | 0.03 | ||
Very good | 1.09 | 0.90 | 1.40 | 0.63 | ||
Excellent | NC | NS | NC | NS | ||
30 days | ||||||
MSAS | 1.54 | 0.07 | 1.40 | 0.20 | 1.57 | 0.21 |
Global Physical Health | 0.96 | 0.08 | 0.97 | 0.24 | 1.42 | 0.23 |
Global Mental Health | 0.98 | 0.42 | 0.99 | 0.62 | 0.25 | 0.62 |
GSRH | ||||||
Fair | 0.92 | 0.86 | 1.19 | 0.72 | 8.85 | 0.07 |
Good | 0.85 | 0.31 | 0.94 | 0.91 | ||
Very good | NC | NS | NC | NS | ||
Excellent | 2.69 | 0.36 | 6.28 | 0.11 | ||
90 days | ||||||
MSAS | 2.23 | 0.03 | 2.20 | 0.05 | 3.79 | 0.05 |
Global Physical Health | 0.94 | 0.07 | 0.95 | 0.11 | 2.75 | 0.10 |
Global Mental Health | 0.96 | 0.20 | 0.95 | 0.15 | 2.11 | 0.15 |
GSRH | ||||||
Fair | 0.75 | 0.63 | 0.67 | 0.53 | 6.67 | 0.15 |
Good | 0.32 | 0.19 | 0.28 | 0.15 | ||
Very good | NC | NS | NC | NS | ||
Excellent | 2.12 | 0.50 | 2.20 | 0.49 | ||
180 days | ||||||
MSAS | 2.39 | 0.03 | 3.51 | 0.01 | 7.04 | 0.01 |
Global Physical Health | 0.93 | 0.06 | 0.93 | 0.03 | 4.61 | 0.03 |
Global Mental Health | 0.97 | 0.38 | 0.96 | 0.33 | 0.95 | 0.33 |
GSRH | ||||||
Fair | 0.98 | 0.98 | 0.64 | 0.55 | 7.13 | 0.13 |
Good | 0.33 | 0.23 | 0.20 | 0.09 | ||
Very good | NC | NS | NC | NS | ||
Excellent | NC | NS | NC | NS |
DISCUSSION
In this longitudinal cohort study, patients, on average, reported relatively severe symptoms, low PROMIS Global Physical and Mental Health scores, and poor GSRH during the inpatient stay in an urban safety‐net hospital. Symptom severity and Global Physical Health improved, on average, by 30 days before stabilizing, but their poor levels in the hospital did not predict 30‐day hospital‐based utilization events. On the other hand, Global Mental Health and GSRH were stable through hospitalizations, and patients scoring poorly on these measures were at greater risk of utilization events within 14 days of discharge. PRO measures obtained during the 180‐day postdischarge period trended toward distinguishing populations with greater baseline risk of proximate utilization events. However, MSAS physical symptom severity and Global Physical Health were more consistently predictive of these events at statistically significant levels compared to Global Mental Health and GSRH in our relatively small sample of patients. Each of these measures selectively improved the fit‐of‐risk prediction models for hospital‐based utilization.
Some of the heterogeneity in readmission risk is explained by differences in PRO measures. Although the MSAS score and Global Physical Health assessment were reliable predictors of utilization when measured in ambulatory settings, they were less discriminating during acute hospitalizations when everyone, on average, reported severe symptoms and poor function. Our results were consistent with other studies that demonstrated the fairly rapid recovery in symptoms that follow hospitalizations,[28, 29] and these measures may become informative of utilization risk as early as 2 weeks postdischarge. GSRH and Global Mental Health (a measure of health‐related quality of life) only predicted utilizations immediately at hospital discharge. As multidimensional measures that reflect physical, social, and emotional capacity, these measures may indicate vulnerabilities in patients least able to handle the stresses of the early postdischarge period.
There is growing momentum around collecting PRO measures in routine clinical care as quality indicators that capture patient‐centered concerns.[30] Our study explored a novel application of these measures whose routine collection will likely proliferate, not solely for the purpose of helping healthcare systems identify patients at risk of unplanned resource utilization. Although multidimensional PRO measures seldom reflect conditions directly modifiable by simple interventions, we believe that the association between physical symptom burden and utilization in our data reveals a possible target for practice improvement. Hospitalists have contributed enormously to shorter lengths of stay that risk sicker and quicker discharges.[31] To mitigate its potential side effects on symptom management, a discharge plan that acknowledges physical symptoms that sometimes persist or recur beyond the hospitalization may be appropriate. This may be accomplished by ensuring that acute symptoms are resolving, giving clear instructions for symptom management at home, as now the standard of care for conditions like asthma,[32] and explicitly communicating the presence of residual symptoms to providers entrusted with continuity care. As an effective feedback measure that can drive continuous quality improvement, we believe that a technology‐based surveillance strategy that spans both the inpatient and outpatient domains is necessary.[23]
There are some notable similarities and differences between the results of our study and a recent hospital‐based study of PRO measures that used data from the Multi‐Center Hospitalist Project.[16] The Physical Component Score of the SF12 is similar to the PROMIS Global Physical Health score in that both incorporate measures of physical function, perceived health, pain, and energy level. Curiously, the SF12 Physical Component Score, but not the PROMIS Global Physical Health score, was associated with 30‐day rehospitalizations. An important difference between the measures is where the SF12 asks about limitations during the past 4 weeks the PROMIS instrument inquires about physical function in general and levels of fatigue and pain in the past 7 days. Considering most hospitalizations last <7 days, the PROMIS instrument may better reflect the declines associated with the acute illness related to the hospitalization than the SF12 score. Additionally, the discrepancy between the association between hospital‐based GSRH and utilization in our study and the absence, thereof, in Hasan et al. is noteworthy. The difference here may be explained by their use of a 0‐ to 100‐point response scale in contrast to our study's verbally labeled 5‐point scale in the PROMIS instrument. The range of rating scales for survey questions is traditionally governed by the tension between the difficulty with mapping respondents judgment on an excessively large scale on one hand, and the failure of insufficient response options to discriminate between respondents with different underlying judgment on the other.[33] We suspect the former to be a drawback of the unlabeled 100‐point response scale, and conjecture that an association might be found in the Multi‐Center Hospitalist Study data if the responses were grouped into summative categories.
We recognize several limitations in our study. The first is the generalizability of our patient population to others, not insignificantly because of the high proportion of the uninsured (around 70% during the study period) and racial/ethnic minorities among them. Although utilization patterns are clearly affected by socioeconomic status,[34] there may also be differences in the way validated PRO measures are calibrated between patients of public and private healthcare systems.[35] Another limitation is our inability to count utilization events at institutions outside of the CCHHS during our study. However, because the study was conducted prior to Cook County's Medicaid expansion demonstration program as part of the Affordable Care Act,[36] many patients established in our system faced barriers to receiving nonemergency care outside of the CCHHS supporting our assumption that few of our patients were discharged from other hospitals. Causality cannot be established in observational studies. Consequently, high prior‐symptom burden may be associated with utilizations through unmeasured variables. Measures of symptom burden are vulnerable to overendorsement and amplification.[37, 38] Inferences based on statistical significance are affected by sample size, and our conclusions may change if conducted with a larger number of participants. Our response rates were excellent through the survey waves, but we did not achieve perfect follow‐up. Worse levels of PRO responses and higher levels of utilization among censored patients biased our results toward the null. Finally, although we did not find any predominant comorbidities associated with hospital‐based utilizations in our sample, our analyses may be vulnerable to inadequate control for illness severity, which may also have biased our results.
PRO measures are likely to be useful in clinical medicine.[39] But to fully apply the powers of PROs in informing clinically and operationally relevant outcomes, we must actively develop a system for obtaining these measures in routine clinical care. The availability of patient downtime makes hospitalizations conducive to gathering patient‐generated data, and may further enhance patient‐provider communication if survey output was readily available in electronic medical records. Exploring innovative strategies for collecting PROs in the hospital and beyond remains our future work.
Disclosures
Funded by the Agency for Healthcare Research and Quality: R24 HS19481‐01 to support technology implementation. The authors report no conflicts of interest, relevant financial interests, activities, relationships, and affiliations that influenced this work. The first and senior authors had full access to all data and take responsibility for their integrity and the accuracy of the data analysis.
- Potentially avoidable 30‐day hospital readmissions in medical patients: derivation and validation of a prediction model. JAMA Intern Med. 2013;173:632–638. , , , .
- Derivation and validation of an index to predict early death or unplanned readmission after discharge from hospital to the community. CMAJ. 2010;182:551–557. , , , et al.
- Redefining readmission risk factors for general medicine patients. J Hosp Med. 2011;6:54–60. , , , .
- Risk prediction models for hospital readmission: a systematic review. JAMA. 2011;306:1688–1698. , , , et al.
- Predicting mortality and healthcare utilization with a single question. Health Serv Res. 2005;40:1234–1246. , , , .
- Repeated hospitalizations and self‐rated health among the elderly: a multivariate failure time analysis. Am J Epidemiol. 2001;153 3 232–241. , , .
- Screening elders for risk of hospital admission. J Am Geriatr Soc. 1993;41:811–817. , , , , , .
- Baseline quality of life as a predictor of mortality and hospitalization in 5,025 patients with congestive heart failure. SOLVD Investigations. Studies of Left Ventricular Dysfunction Investigators. Am J Cardiol. 1996;78:890–895. , , , et al.
- Using quality of life to predict hospitalization and mortality in patients with obstructive lung disease. Chest. 2002;122:429–436. , , , , .
- Intraindividual change in SF‐36 in ambulatory clinic primary care patients predicted mortality and hospitalizations. J Clin Epidemiol. 2004;57:277–283. , , , .
- Use of health‐related, quality‐of‐life metrics to predict mortality and hospitalizations in community‐dwelling seniors. J Am Geriatr Soc. 2006;54:667–673. , , , et al.
- Medical outcomes study short form‐36: a consistent and powerful predictor of morbidity and mortality in dialysis patients. Am J Kidney Dis. 2003;41:1286–1292. , , , .
- Relationship of depression to increased risk of mortality and rehospitalization in patients with congestive heart failure. Arch Intern Med. 2001;161:1849–1856. , , , et al.
- Depression as a predictor of mortality and hospitalization among hemodialysis patients in the United States and Europe. Kidney Int. 2002;62:199–207. , , , et al.
- Health status predicts long‐term outcomes in outpatients with coronary disease. Circulation. 2002;106:43–49. , , , , .
- Hospital readmission in general medicine patients: a prediction model. J Gen Intern Med. 2010;25:211–219. , , , et al.
- Persistent angina pectoris in ischaemic cardiomyopathy: increased rehospitalization and major adverse cardiac events. Eur J Heart Fail. 2014;16:854–860. , , , , , .
- Relation of dyspnea severity on admission for acute heart failure with outcomes and costs. Am J Cardiol. 2015;115:75–81. , , , et al.
- Two views of self‐rated general health status. Soc Sci Med. 2003;56:203–217. , , .
- Self‐rated health and physical disability in elderly survivors of a major medical event. J Gerontol B Psychol Sci Soc Sci. 1996;51:S96–S104. , , .
- Predicting changes in perceived health status. Am J Public Health. 1984;74:611–614. , , .
- Quality of life before and after intensive care. Anaesthesia. 2005;60:322–329. , , , , .
- Trick WE. Health perceptions and symptom burden in primary care: measuring health using audio computer‐assisted self‐interviews [published online ahead of print December 7, 2014]. Qual Life Res. doi: 10.1007/s11136‐014‐0884‐4. , , , , ,
- Adapting a clinical comorbidity index for use with ICD‐9‐CM administrative databases. J Clin Epidemiol. 1992;45:613–619. , , .
- The Memorial Symptom Assessment Scale: an instrument for the evaluation of symptom prevalence, characteristics and distress. Eur J Cancer. 1994;30A:1326–1336. , , , et al.
- The Memorial Symptom Assessment Scale short form (MSAS‐SF). Cancer. 2000;89:1162–1171. , , , , .
- Development of physical and mental health summary schores from the Patient‐Reported Outcomes Measurement Information System (PROMIS) global items. Qual Life Res. 2009;18:873–880. , , , , .
- Improvements in signs and symptoms during hospitalization for acute heart failure follow different patterns and depend on the measurement scales used: an international, prospective registry to evaluate the evolution of measures of disease severity in acute heart failure (MEASURE‐AHF). J Card Fail. 2008;14:777–784. , , , et al.
- Longitudinal assessment of symptom severity among hospitalized elders diagnosed with cancer, heart failure, and chronic obstructive pulmonary disease. J Hosp Med. 2012;7:567–572. , , , .
- The patient experience and health outcomes. N Engl J Med. 2013;368:201–203. , , , .
- "Quicker and sicker" under Medicare's prospective payment system for hospitals: new evidence on an old issue from a national longitudinal survey. Bull Econ Res. 2011;63:1–27. , , , .
- Agency for Healthcare Research and Quality. Asthma care quality improvement measures. Available at: http://www.ahrq.gov/professionals/quality‐patient‐safety/quality‐resources/tools/asthmaqual/asthmacare/appendix‐d.html. Accessed January 30, 2015.
- The Psychology of Survey Response. New York, NY: Cambridge University Press; 2000. , , .
- Health care for children and youth in the United States: annual report on patterns of coverage, utilization, quality, and expenditures by income. Ambul Pediatr. 2005;5:6–44. , , , et al.
- Levels of symptom burden during chemotherapy for advanced lung cancer: differences between public hospitals and a tertiary cancer center. J Clin Oncol. 2011;29:2859–2865. , , , et al.
- http://kff.org/medicaid/issue-brief/profiles-of-medicaid-outreach-and-enrollment-strategies-the-cook-county-early-expansion-initiative. Published April 7, 2014. Accessed December 2, 2014. . Profiles of Medicaid outreach and enrollment strategies: the Cook County early expansion initiative. The Henry J. Kaiser Family Foundation. Available at:
- A primary care perspective on prevailing assumptions about persistent medically unexplained physical symptoms. Int J Psychiatry Med. 2002;32:125–140. , , .
- Symptom burden and comorbidities impact the consistency of responses on patient‐reported functional outcomes. Arch Phys Med Rehabil. 2014;95:79–86. , , , .
- Implementing patient‐reported outcomes assessment in clinical practice: a review of the options and considerations. Qual Life Res. 2012;21:1305–1314. , , , et al.
Despite widespread efforts to predict 30‐day rehospitalizations among discharged general medical patients,[1, 2, 3] not many strategies have incorporated patient‐reported outcome (PRO) measures in predictive models.[4] This despite the many longitudinal studies of the ambulatory population that demonstrate the higher likelihood of hospitalizations among those who score poorly on General Self‐Rated Health (GSRH),[5, 6, 7] baseline or declining Health‐Related Quality of Life,[8, 9, 10, 11, 12] psychological symptoms,[13, 14] and physical symptoms assessments.[15] One of the few existing studies that included PRO measures in 30‐day readmission models showed the predictive value of the 12‐item short form (SF12) Physical Component Score.[16] Others showed that persistent symptoms were associated with readmissions in patients with heart disease.[17, 18]
The paucity of efforts to connect PRO measures to utilization may be due to the limited availability of these measures in routine clinical records and the incomplete knowledge about how various PRO measures may fluctuate during episodes of acute illnesses and their treatments during hospitalizations. Health perception measures reflect both enduring features like self‐concept as well as dynamic features like a person's immediate health status.[19] As such, GSRH reflects the presence of chronic illnesses but is also responsive to acute events.[20, 21] Similarly, Health‐Related Quality of Life measures are dynamic as they decline around episodes of acute illness but are stable over a longer time window in their tendency to recover.[22] We do not know how fluctuations in measures of symptom burden, perceived health, and quality of life around the hospital‐to‐home transition may differentially inform readmission risk. Using a longitudinal cohort study, we addressed 2 questions: (1) How do PRO measures change when measured serially during the hospital‐to‐home transition? (2) How does the relative timing of each PRO measure variably inform the risk of subsequent utilization events including hospital readmissions?
METHODS
We conducted a longitudinal cohort study using data originally collected for a trial (
The following instruments were administered at each interview. The physical symptom severity portion of the Memorial Symptom Assessment Scale (MSAS) solicited the severity rank (none/a little bit/somewhat/quite a bit/very much) of 17 physical symptoms in the last week; the score was calculated by averaging the severity rank of the 12 most common symptom in the sample.[25, 26] The Patient Reported Outcomes Measurement Information System (PROMIS) Global Health Short Form is an instrument assessing GSRH (1 item), Social Activities (1 item), Global Physical Health (4 items), and Global Mental Health (4 items including a single‐item quality‐of‐life measure). Fatigue and pain for Global Physical Health, and emotional health for Global Mental Health were assessed over the past 7 days. Each of the 2 Global Health scores was standardized to a national mean of 50 and standard deviation of 10.[27]
The rate of survey completion at each follow‐up was calculated. Characteristics of participants were tabulated. Characteristics of patients censored prior to study completion were compared with patients with complete data. Box plots for MSAS physical symptom severity, and Global Physical and Mental Health scores were constructed to illustrate the comparisons of the mean scores between each consecutive survey period using t tests assuming unequal variance. A similar box plot of GSRH illustrated the comparison of the median score between consecutive surveys using the rank sum test. Hospital‐based utilization events were defined as either an emergency department visit or hospitalization at 1 of the 2 hospitals of the Cook County Health & Hospitals System (CCHHS). After accounting for patient data censored due to death (date reported by family) or withdrawal from study, Kaplan‐Meier curves showing time to first hospital‐based utilization event during each interval between surveys were drawn separately for above‐ and below‐median MSAS, Global Physical and Mental Health scores, and for poor or fair versus good, very good, or excellent GSRH assessment. The null hypothesis that the survivor functions were equal between the better and worse median quantiles or GSRH categories was tested using the log‐rank test at 14 and 30 days from survey completion. Hazard ratios for time to first utilization event within 14 days of each survey were calculated for the MSAS score, Global Physical and Mental Health as continuous variables, and GSRH response categories relative to poor using bivariate and multivariate Cox proportional hazard equations. Multivariate models incorporated the following 5 covariates: at least 1 utilization event during the study period prior to the survey, Charlson score, age, gender, and race/ethnicity category. Likelihood ratio statistics were calculated to test the hypothesis that the model including the PRO measure and covariates predicted the outcome equally well compared to the nested model with only covariates. We used the traditional threshold of .05 when reporting significance. All analyses were performed in Stata 13 (StataCorp, College Station, TX). The methods for patient consent, data collection, analyses, and reporting were reviewed and approved by the CCHHS institutional review board.
RESULTS
A total of 196 patients completed the initial survey. The completion rates were 98%, 90%, and 88% for the 30‐, 90‐, and 180‐day follow‐up surveys, respectively. As shown in Table 1, participants average age was 52 years, and about half were women. The majority was non‐Hispanic black, and 21% preferred to complete the survey in Spanish. Diabetes, congestive heart failure, cancer, and chronic pulmonary disease were each prevalent in at least one‐fifth of our patient cohort. Demographic characteristics were similar between the 160 patients who completed all 3 follow‐up surveys and the 36 who missed at least 1 follow‐up survey. Among the latter group, 1 withdrew at 30 days, 1 withdrew and 4 had died at 90 days, and 1 withdrew and 9 had died at 180 days.
| |
Age, y, mean (SD) | 52 (10) |
Female, n (%) | 100 (51) |
Race/ethnicity category, n (%) | |
Non‐Hispanic black | 117 (60) |
Hispanic | 52 (27) |
Non‐Hispanic white | 20 (10) |
Other | 6 (3) |
Language, n (%) | |
English | 155 (79) |
Spanish | 41 (21) |
Charlson Comorbidity Index, median (range) | 1 (09) |
Charlson comorbidities, n (%) | |
Diabetes | 71 (36) |
Congestive heart failure | 52 (27) |
Cancer (with and without metastases) | 43 (22) |
Chronic pulmonary disease | 40 (20) |
Myocardial infarction | 17 (9) |
Renal disease | 11 (6) |
Figure 1 shows a timeline of the follow‐up surveys and utilization events in the form of overlapping histograms. The majority of 30‐day follow‐up questionnaires were completed earlier than targeted, at a median of 17 (interquartile range [IQR] 16, 20) days after discharge. Similarly, questionnaires targeted for 90 and 180 days were completed at medians of 78 (IQR 7684) and 167 (IQR 166169) days from discharge. Fifty‐four (28%) patients experienced a first utilization event in the first 30 days following discharge. During the 60‐, 90‐, and 30‐day intervals after the first, second, and third follow‐up surveys, respectively, 63 (33%), 54 (31%), and 16 (9%) patients experienced a first utilization event.
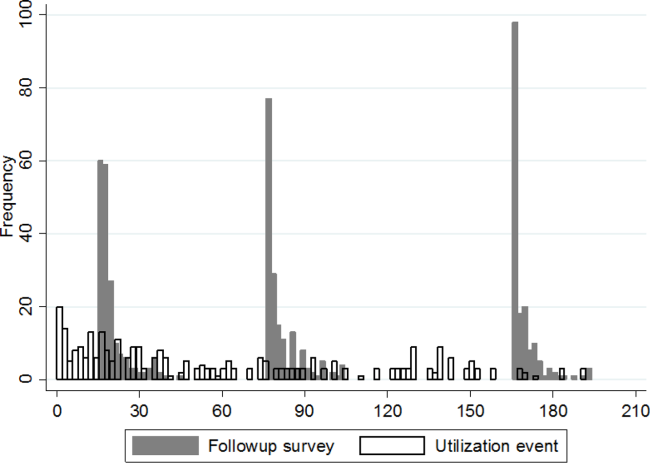
A significant improvement in MSAS physical symptom severity was detected between the hospitalization and the 30‐day follow‐up (Figure 2A). Although the mean Global Physical Health score was below the national mean of 50 at every survey period, a similar improvement in the measure was noted between the hospitalization and the 30‐day follow‐up (Figure 2B). The mean Global Mental Health score was also below the national mean but remained stable throughout the study (Figure 2C). The median GSRH was stable at 2 (IQR 23) at every survey wave (Figure 2D). Of note, compared to patients who completed all 3 follow‐up surveys, patients who missed at least 1 follow‐up reported higher MSAS score (1.5 vs 1.8, P=0.03), lower Global Physical Health (36.1 vs 33.5, P=0.09), and lower Global Mental Health (44.7 vs 41.0, P=0.03) during their hospitalization. In addition, patients with complete data experienced an average of 1.2 utilization events during the study, whereas those with missing data experienced an average of 2.1 utilization events (P=0.03).
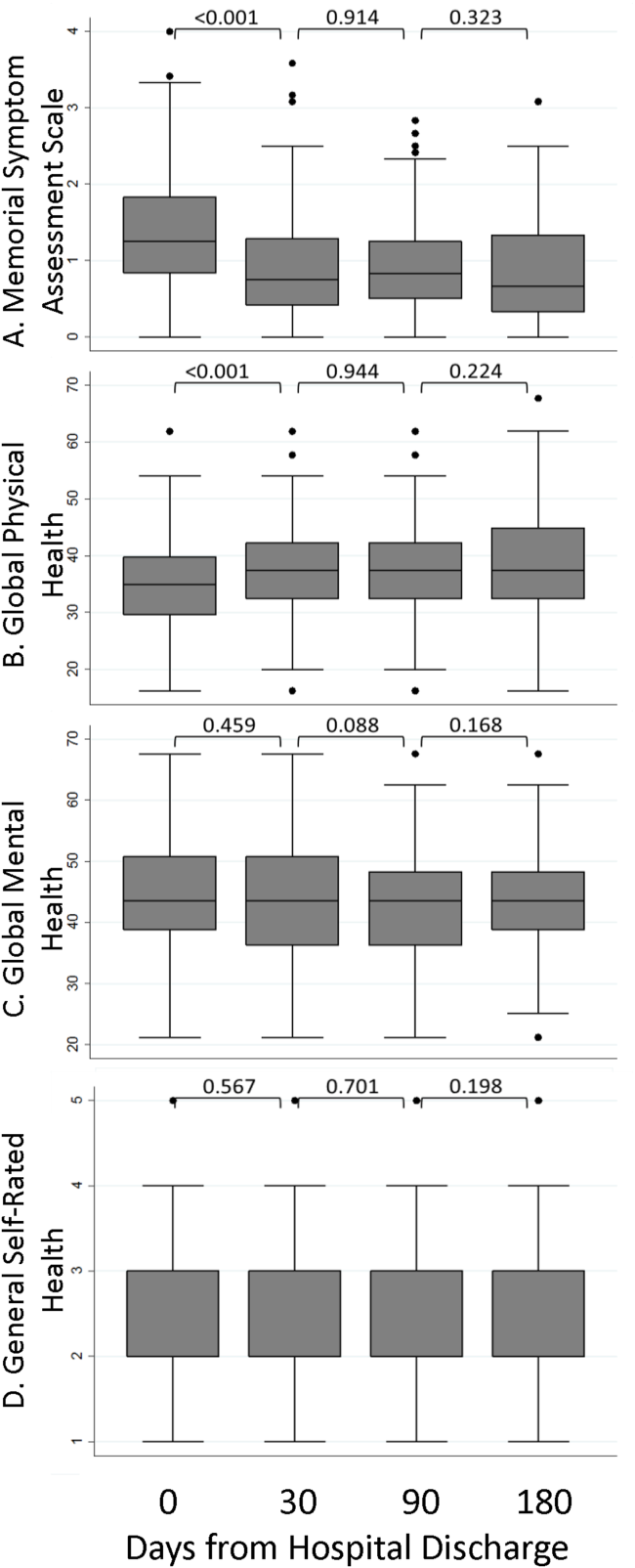
The MSAS physical symptom severity and Global Physical Health scores from the index hospitalizations did not identify patients with a first utilization event within 30 days. However, patients with poor Global Mental Health and GSRH in the hospital were more likely to experience a utilization event within 14 days of discharge (Figure 3). During the postdischarge period, patients scoring poorly on each of the PRO measures trended toward a greater risk of an early utilization event, but the association between utilization and MSAS was most consistently significant (Figure 3A). In general, the associations with MSAS, Global Physical Health, and GSRH were stronger with the risk of utilization events within 14 days than within 30 days (Figure 3A,B,D). The Global Mental Health score was not associated with a subsequent utilization when measured during the 180‐day postdischarge period.
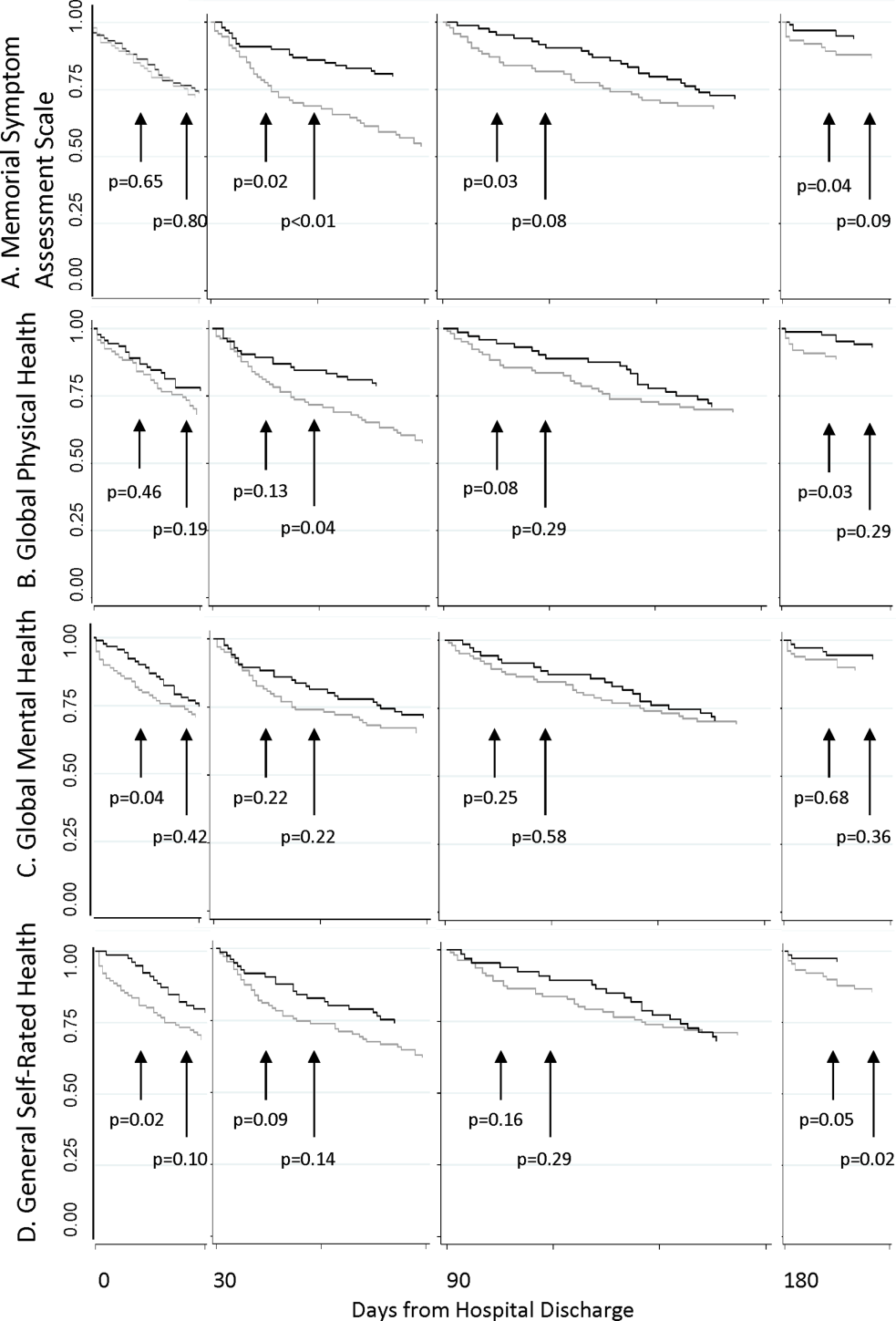
As shown in Table 2, Cox proportional hazard models incorporating covariates preserved most of the significant associations seen in the unadjusted analyses. Global Mental Health and good relative to poor GSRH obtained during the hospitalization remained significant. MSAS obtained at each postdischarge follow‐up trended positively with utilization and was statistically significant at 90 and 180 days. Global Physical Health obtained at each postdischarge follow‐up similarly trended negatively with utilization and was significant at 180 days. Each multivariate model incorporating a PRO measure with a significant coefficient contributed to better fit of the predictive model compared to the nested model without the PRO measure.
Unadjusted Hazard Ratio | P | Adjusted Hazard Ratio* | P | Likelihood Ratio | P | |
---|---|---|---|---|---|---|
| ||||||
Hospital discharge | ||||||
MSAS | 1.47 | 0.11 | 1.38 | 0.19 | 1.65 | 0.20 |
Global Physical Health | 0.96 | 0.10 | 0.96 | 0.13 | 2.29 | 0.13 |
Global Mental Health | 0.96 | 0.05 | 0.96 | 0.05 | 4.05 | 0.04 |
GSRH | ||||||
Fair | 1.09 | 0.85 | 1.26 | 0.61 | 12.27 | 0.02 |
Good | 0.24 | 0.04 | 0.23 | 0.03 | ||
Very good | 1.09 | 0.90 | 1.40 | 0.63 | ||
Excellent | NC | NS | NC | NS | ||
30 days | ||||||
MSAS | 1.54 | 0.07 | 1.40 | 0.20 | 1.57 | 0.21 |
Global Physical Health | 0.96 | 0.08 | 0.97 | 0.24 | 1.42 | 0.23 |
Global Mental Health | 0.98 | 0.42 | 0.99 | 0.62 | 0.25 | 0.62 |
GSRH | ||||||
Fair | 0.92 | 0.86 | 1.19 | 0.72 | 8.85 | 0.07 |
Good | 0.85 | 0.31 | 0.94 | 0.91 | ||
Very good | NC | NS | NC | NS | ||
Excellent | 2.69 | 0.36 | 6.28 | 0.11 | ||
90 days | ||||||
MSAS | 2.23 | 0.03 | 2.20 | 0.05 | 3.79 | 0.05 |
Global Physical Health | 0.94 | 0.07 | 0.95 | 0.11 | 2.75 | 0.10 |
Global Mental Health | 0.96 | 0.20 | 0.95 | 0.15 | 2.11 | 0.15 |
GSRH | ||||||
Fair | 0.75 | 0.63 | 0.67 | 0.53 | 6.67 | 0.15 |
Good | 0.32 | 0.19 | 0.28 | 0.15 | ||
Very good | NC | NS | NC | NS | ||
Excellent | 2.12 | 0.50 | 2.20 | 0.49 | ||
180 days | ||||||
MSAS | 2.39 | 0.03 | 3.51 | 0.01 | 7.04 | 0.01 |
Global Physical Health | 0.93 | 0.06 | 0.93 | 0.03 | 4.61 | 0.03 |
Global Mental Health | 0.97 | 0.38 | 0.96 | 0.33 | 0.95 | 0.33 |
GSRH | ||||||
Fair | 0.98 | 0.98 | 0.64 | 0.55 | 7.13 | 0.13 |
Good | 0.33 | 0.23 | 0.20 | 0.09 | ||
Very good | NC | NS | NC | NS | ||
Excellent | NC | NS | NC | NS |
DISCUSSION
In this longitudinal cohort study, patients, on average, reported relatively severe symptoms, low PROMIS Global Physical and Mental Health scores, and poor GSRH during the inpatient stay in an urban safety‐net hospital. Symptom severity and Global Physical Health improved, on average, by 30 days before stabilizing, but their poor levels in the hospital did not predict 30‐day hospital‐based utilization events. On the other hand, Global Mental Health and GSRH were stable through hospitalizations, and patients scoring poorly on these measures were at greater risk of utilization events within 14 days of discharge. PRO measures obtained during the 180‐day postdischarge period trended toward distinguishing populations with greater baseline risk of proximate utilization events. However, MSAS physical symptom severity and Global Physical Health were more consistently predictive of these events at statistically significant levels compared to Global Mental Health and GSRH in our relatively small sample of patients. Each of these measures selectively improved the fit‐of‐risk prediction models for hospital‐based utilization.
Some of the heterogeneity in readmission risk is explained by differences in PRO measures. Although the MSAS score and Global Physical Health assessment were reliable predictors of utilization when measured in ambulatory settings, they were less discriminating during acute hospitalizations when everyone, on average, reported severe symptoms and poor function. Our results were consistent with other studies that demonstrated the fairly rapid recovery in symptoms that follow hospitalizations,[28, 29] and these measures may become informative of utilization risk as early as 2 weeks postdischarge. GSRH and Global Mental Health (a measure of health‐related quality of life) only predicted utilizations immediately at hospital discharge. As multidimensional measures that reflect physical, social, and emotional capacity, these measures may indicate vulnerabilities in patients least able to handle the stresses of the early postdischarge period.
There is growing momentum around collecting PRO measures in routine clinical care as quality indicators that capture patient‐centered concerns.[30] Our study explored a novel application of these measures whose routine collection will likely proliferate, not solely for the purpose of helping healthcare systems identify patients at risk of unplanned resource utilization. Although multidimensional PRO measures seldom reflect conditions directly modifiable by simple interventions, we believe that the association between physical symptom burden and utilization in our data reveals a possible target for practice improvement. Hospitalists have contributed enormously to shorter lengths of stay that risk sicker and quicker discharges.[31] To mitigate its potential side effects on symptom management, a discharge plan that acknowledges physical symptoms that sometimes persist or recur beyond the hospitalization may be appropriate. This may be accomplished by ensuring that acute symptoms are resolving, giving clear instructions for symptom management at home, as now the standard of care for conditions like asthma,[32] and explicitly communicating the presence of residual symptoms to providers entrusted with continuity care. As an effective feedback measure that can drive continuous quality improvement, we believe that a technology‐based surveillance strategy that spans both the inpatient and outpatient domains is necessary.[23]
There are some notable similarities and differences between the results of our study and a recent hospital‐based study of PRO measures that used data from the Multi‐Center Hospitalist Project.[16] The Physical Component Score of the SF12 is similar to the PROMIS Global Physical Health score in that both incorporate measures of physical function, perceived health, pain, and energy level. Curiously, the SF12 Physical Component Score, but not the PROMIS Global Physical Health score, was associated with 30‐day rehospitalizations. An important difference between the measures is where the SF12 asks about limitations during the past 4 weeks the PROMIS instrument inquires about physical function in general and levels of fatigue and pain in the past 7 days. Considering most hospitalizations last <7 days, the PROMIS instrument may better reflect the declines associated with the acute illness related to the hospitalization than the SF12 score. Additionally, the discrepancy between the association between hospital‐based GSRH and utilization in our study and the absence, thereof, in Hasan et al. is noteworthy. The difference here may be explained by their use of a 0‐ to 100‐point response scale in contrast to our study's verbally labeled 5‐point scale in the PROMIS instrument. The range of rating scales for survey questions is traditionally governed by the tension between the difficulty with mapping respondents judgment on an excessively large scale on one hand, and the failure of insufficient response options to discriminate between respondents with different underlying judgment on the other.[33] We suspect the former to be a drawback of the unlabeled 100‐point response scale, and conjecture that an association might be found in the Multi‐Center Hospitalist Study data if the responses were grouped into summative categories.
We recognize several limitations in our study. The first is the generalizability of our patient population to others, not insignificantly because of the high proportion of the uninsured (around 70% during the study period) and racial/ethnic minorities among them. Although utilization patterns are clearly affected by socioeconomic status,[34] there may also be differences in the way validated PRO measures are calibrated between patients of public and private healthcare systems.[35] Another limitation is our inability to count utilization events at institutions outside of the CCHHS during our study. However, because the study was conducted prior to Cook County's Medicaid expansion demonstration program as part of the Affordable Care Act,[36] many patients established in our system faced barriers to receiving nonemergency care outside of the CCHHS supporting our assumption that few of our patients were discharged from other hospitals. Causality cannot be established in observational studies. Consequently, high prior‐symptom burden may be associated with utilizations through unmeasured variables. Measures of symptom burden are vulnerable to overendorsement and amplification.[37, 38] Inferences based on statistical significance are affected by sample size, and our conclusions may change if conducted with a larger number of participants. Our response rates were excellent through the survey waves, but we did not achieve perfect follow‐up. Worse levels of PRO responses and higher levels of utilization among censored patients biased our results toward the null. Finally, although we did not find any predominant comorbidities associated with hospital‐based utilizations in our sample, our analyses may be vulnerable to inadequate control for illness severity, which may also have biased our results.
PRO measures are likely to be useful in clinical medicine.[39] But to fully apply the powers of PROs in informing clinically and operationally relevant outcomes, we must actively develop a system for obtaining these measures in routine clinical care. The availability of patient downtime makes hospitalizations conducive to gathering patient‐generated data, and may further enhance patient‐provider communication if survey output was readily available in electronic medical records. Exploring innovative strategies for collecting PROs in the hospital and beyond remains our future work.
Disclosures
Funded by the Agency for Healthcare Research and Quality: R24 HS19481‐01 to support technology implementation. The authors report no conflicts of interest, relevant financial interests, activities, relationships, and affiliations that influenced this work. The first and senior authors had full access to all data and take responsibility for their integrity and the accuracy of the data analysis.
Despite widespread efforts to predict 30‐day rehospitalizations among discharged general medical patients,[1, 2, 3] not many strategies have incorporated patient‐reported outcome (PRO) measures in predictive models.[4] This despite the many longitudinal studies of the ambulatory population that demonstrate the higher likelihood of hospitalizations among those who score poorly on General Self‐Rated Health (GSRH),[5, 6, 7] baseline or declining Health‐Related Quality of Life,[8, 9, 10, 11, 12] psychological symptoms,[13, 14] and physical symptoms assessments.[15] One of the few existing studies that included PRO measures in 30‐day readmission models showed the predictive value of the 12‐item short form (SF12) Physical Component Score.[16] Others showed that persistent symptoms were associated with readmissions in patients with heart disease.[17, 18]
The paucity of efforts to connect PRO measures to utilization may be due to the limited availability of these measures in routine clinical records and the incomplete knowledge about how various PRO measures may fluctuate during episodes of acute illnesses and their treatments during hospitalizations. Health perception measures reflect both enduring features like self‐concept as well as dynamic features like a person's immediate health status.[19] As such, GSRH reflects the presence of chronic illnesses but is also responsive to acute events.[20, 21] Similarly, Health‐Related Quality of Life measures are dynamic as they decline around episodes of acute illness but are stable over a longer time window in their tendency to recover.[22] We do not know how fluctuations in measures of symptom burden, perceived health, and quality of life around the hospital‐to‐home transition may differentially inform readmission risk. Using a longitudinal cohort study, we addressed 2 questions: (1) How do PRO measures change when measured serially during the hospital‐to‐home transition? (2) How does the relative timing of each PRO measure variably inform the risk of subsequent utilization events including hospital readmissions?
METHODS
We conducted a longitudinal cohort study using data originally collected for a trial (
The following instruments were administered at each interview. The physical symptom severity portion of the Memorial Symptom Assessment Scale (MSAS) solicited the severity rank (none/a little bit/somewhat/quite a bit/very much) of 17 physical symptoms in the last week; the score was calculated by averaging the severity rank of the 12 most common symptom in the sample.[25, 26] The Patient Reported Outcomes Measurement Information System (PROMIS) Global Health Short Form is an instrument assessing GSRH (1 item), Social Activities (1 item), Global Physical Health (4 items), and Global Mental Health (4 items including a single‐item quality‐of‐life measure). Fatigue and pain for Global Physical Health, and emotional health for Global Mental Health were assessed over the past 7 days. Each of the 2 Global Health scores was standardized to a national mean of 50 and standard deviation of 10.[27]
The rate of survey completion at each follow‐up was calculated. Characteristics of participants were tabulated. Characteristics of patients censored prior to study completion were compared with patients with complete data. Box plots for MSAS physical symptom severity, and Global Physical and Mental Health scores were constructed to illustrate the comparisons of the mean scores between each consecutive survey period using t tests assuming unequal variance. A similar box plot of GSRH illustrated the comparison of the median score between consecutive surveys using the rank sum test. Hospital‐based utilization events were defined as either an emergency department visit or hospitalization at 1 of the 2 hospitals of the Cook County Health & Hospitals System (CCHHS). After accounting for patient data censored due to death (date reported by family) or withdrawal from study, Kaplan‐Meier curves showing time to first hospital‐based utilization event during each interval between surveys were drawn separately for above‐ and below‐median MSAS, Global Physical and Mental Health scores, and for poor or fair versus good, very good, or excellent GSRH assessment. The null hypothesis that the survivor functions were equal between the better and worse median quantiles or GSRH categories was tested using the log‐rank test at 14 and 30 days from survey completion. Hazard ratios for time to first utilization event within 14 days of each survey were calculated for the MSAS score, Global Physical and Mental Health as continuous variables, and GSRH response categories relative to poor using bivariate and multivariate Cox proportional hazard equations. Multivariate models incorporated the following 5 covariates: at least 1 utilization event during the study period prior to the survey, Charlson score, age, gender, and race/ethnicity category. Likelihood ratio statistics were calculated to test the hypothesis that the model including the PRO measure and covariates predicted the outcome equally well compared to the nested model with only covariates. We used the traditional threshold of .05 when reporting significance. All analyses were performed in Stata 13 (StataCorp, College Station, TX). The methods for patient consent, data collection, analyses, and reporting were reviewed and approved by the CCHHS institutional review board.
RESULTS
A total of 196 patients completed the initial survey. The completion rates were 98%, 90%, and 88% for the 30‐, 90‐, and 180‐day follow‐up surveys, respectively. As shown in Table 1, participants average age was 52 years, and about half were women. The majority was non‐Hispanic black, and 21% preferred to complete the survey in Spanish. Diabetes, congestive heart failure, cancer, and chronic pulmonary disease were each prevalent in at least one‐fifth of our patient cohort. Demographic characteristics were similar between the 160 patients who completed all 3 follow‐up surveys and the 36 who missed at least 1 follow‐up survey. Among the latter group, 1 withdrew at 30 days, 1 withdrew and 4 had died at 90 days, and 1 withdrew and 9 had died at 180 days.
| |
Age, y, mean (SD) | 52 (10) |
Female, n (%) | 100 (51) |
Race/ethnicity category, n (%) | |
Non‐Hispanic black | 117 (60) |
Hispanic | 52 (27) |
Non‐Hispanic white | 20 (10) |
Other | 6 (3) |
Language, n (%) | |
English | 155 (79) |
Spanish | 41 (21) |
Charlson Comorbidity Index, median (range) | 1 (09) |
Charlson comorbidities, n (%) | |
Diabetes | 71 (36) |
Congestive heart failure | 52 (27) |
Cancer (with and without metastases) | 43 (22) |
Chronic pulmonary disease | 40 (20) |
Myocardial infarction | 17 (9) |
Renal disease | 11 (6) |
Figure 1 shows a timeline of the follow‐up surveys and utilization events in the form of overlapping histograms. The majority of 30‐day follow‐up questionnaires were completed earlier than targeted, at a median of 17 (interquartile range [IQR] 16, 20) days after discharge. Similarly, questionnaires targeted for 90 and 180 days were completed at medians of 78 (IQR 7684) and 167 (IQR 166169) days from discharge. Fifty‐four (28%) patients experienced a first utilization event in the first 30 days following discharge. During the 60‐, 90‐, and 30‐day intervals after the first, second, and third follow‐up surveys, respectively, 63 (33%), 54 (31%), and 16 (9%) patients experienced a first utilization event.
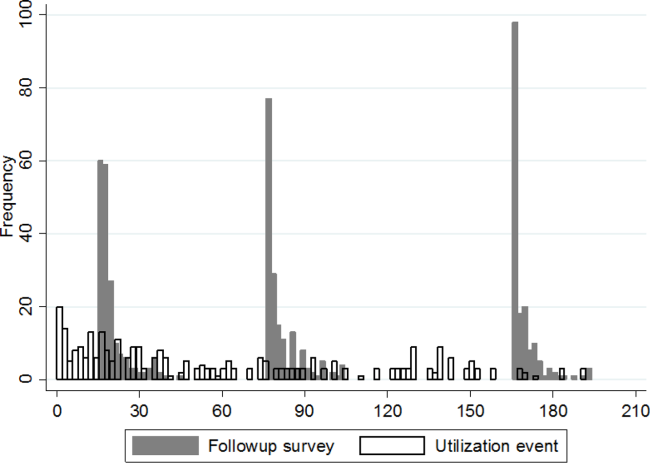
A significant improvement in MSAS physical symptom severity was detected between the hospitalization and the 30‐day follow‐up (Figure 2A). Although the mean Global Physical Health score was below the national mean of 50 at every survey period, a similar improvement in the measure was noted between the hospitalization and the 30‐day follow‐up (Figure 2B). The mean Global Mental Health score was also below the national mean but remained stable throughout the study (Figure 2C). The median GSRH was stable at 2 (IQR 23) at every survey wave (Figure 2D). Of note, compared to patients who completed all 3 follow‐up surveys, patients who missed at least 1 follow‐up reported higher MSAS score (1.5 vs 1.8, P=0.03), lower Global Physical Health (36.1 vs 33.5, P=0.09), and lower Global Mental Health (44.7 vs 41.0, P=0.03) during their hospitalization. In addition, patients with complete data experienced an average of 1.2 utilization events during the study, whereas those with missing data experienced an average of 2.1 utilization events (P=0.03).
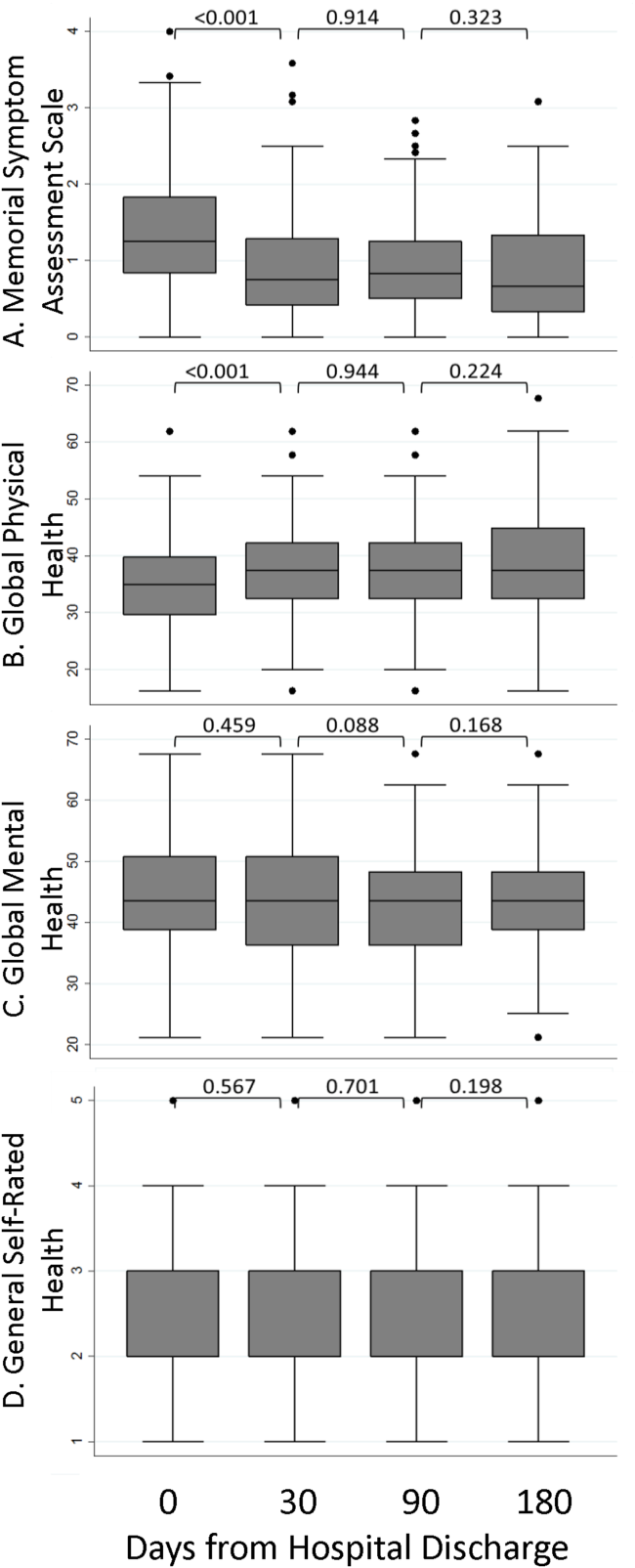
The MSAS physical symptom severity and Global Physical Health scores from the index hospitalizations did not identify patients with a first utilization event within 30 days. However, patients with poor Global Mental Health and GSRH in the hospital were more likely to experience a utilization event within 14 days of discharge (Figure 3). During the postdischarge period, patients scoring poorly on each of the PRO measures trended toward a greater risk of an early utilization event, but the association between utilization and MSAS was most consistently significant (Figure 3A). In general, the associations with MSAS, Global Physical Health, and GSRH were stronger with the risk of utilization events within 14 days than within 30 days (Figure 3A,B,D). The Global Mental Health score was not associated with a subsequent utilization when measured during the 180‐day postdischarge period.
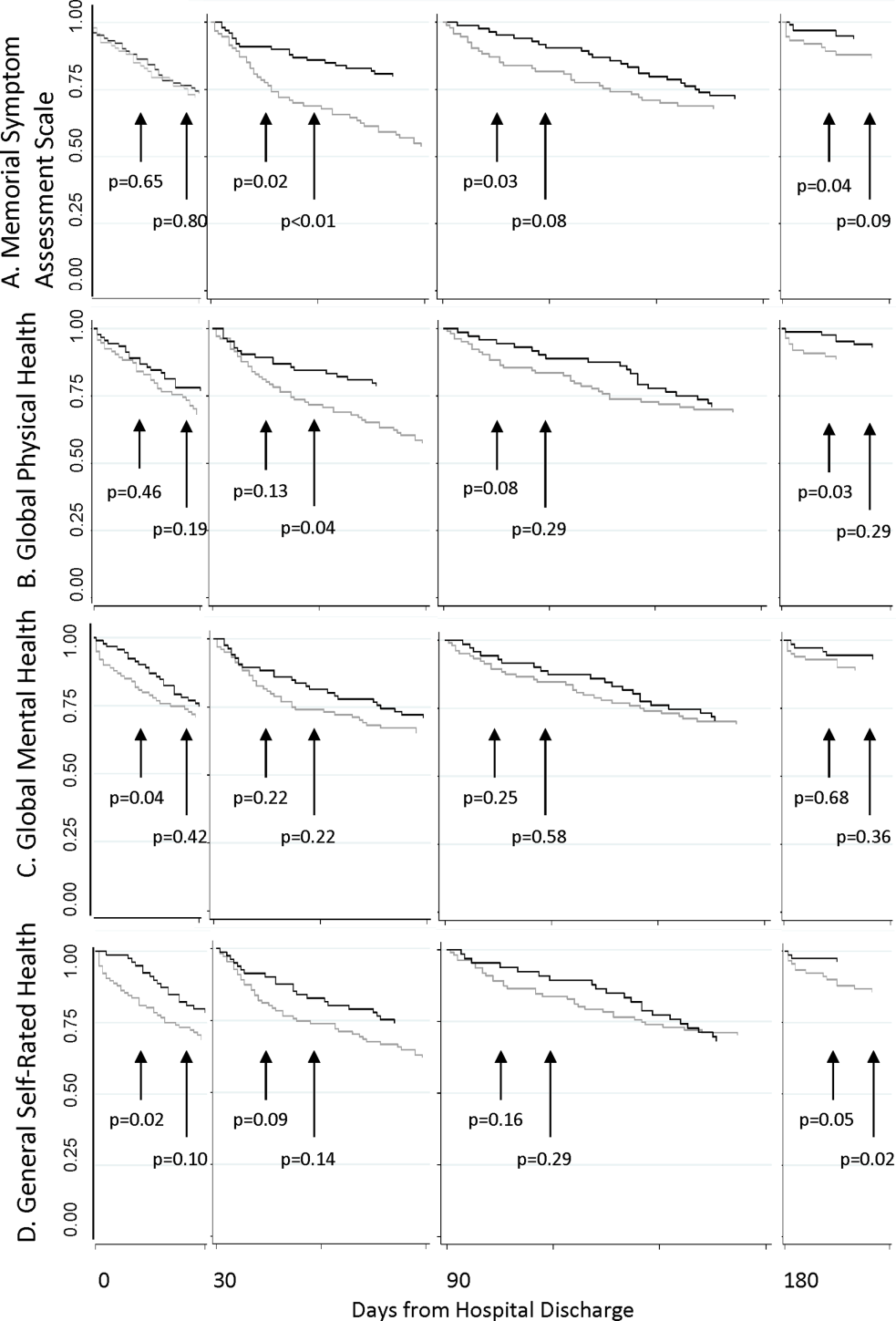
As shown in Table 2, Cox proportional hazard models incorporating covariates preserved most of the significant associations seen in the unadjusted analyses. Global Mental Health and good relative to poor GSRH obtained during the hospitalization remained significant. MSAS obtained at each postdischarge follow‐up trended positively with utilization and was statistically significant at 90 and 180 days. Global Physical Health obtained at each postdischarge follow‐up similarly trended negatively with utilization and was significant at 180 days. Each multivariate model incorporating a PRO measure with a significant coefficient contributed to better fit of the predictive model compared to the nested model without the PRO measure.
Unadjusted Hazard Ratio | P | Adjusted Hazard Ratio* | P | Likelihood Ratio | P | |
---|---|---|---|---|---|---|
| ||||||
Hospital discharge | ||||||
MSAS | 1.47 | 0.11 | 1.38 | 0.19 | 1.65 | 0.20 |
Global Physical Health | 0.96 | 0.10 | 0.96 | 0.13 | 2.29 | 0.13 |
Global Mental Health | 0.96 | 0.05 | 0.96 | 0.05 | 4.05 | 0.04 |
GSRH | ||||||
Fair | 1.09 | 0.85 | 1.26 | 0.61 | 12.27 | 0.02 |
Good | 0.24 | 0.04 | 0.23 | 0.03 | ||
Very good | 1.09 | 0.90 | 1.40 | 0.63 | ||
Excellent | NC | NS | NC | NS | ||
30 days | ||||||
MSAS | 1.54 | 0.07 | 1.40 | 0.20 | 1.57 | 0.21 |
Global Physical Health | 0.96 | 0.08 | 0.97 | 0.24 | 1.42 | 0.23 |
Global Mental Health | 0.98 | 0.42 | 0.99 | 0.62 | 0.25 | 0.62 |
GSRH | ||||||
Fair | 0.92 | 0.86 | 1.19 | 0.72 | 8.85 | 0.07 |
Good | 0.85 | 0.31 | 0.94 | 0.91 | ||
Very good | NC | NS | NC | NS | ||
Excellent | 2.69 | 0.36 | 6.28 | 0.11 | ||
90 days | ||||||
MSAS | 2.23 | 0.03 | 2.20 | 0.05 | 3.79 | 0.05 |
Global Physical Health | 0.94 | 0.07 | 0.95 | 0.11 | 2.75 | 0.10 |
Global Mental Health | 0.96 | 0.20 | 0.95 | 0.15 | 2.11 | 0.15 |
GSRH | ||||||
Fair | 0.75 | 0.63 | 0.67 | 0.53 | 6.67 | 0.15 |
Good | 0.32 | 0.19 | 0.28 | 0.15 | ||
Very good | NC | NS | NC | NS | ||
Excellent | 2.12 | 0.50 | 2.20 | 0.49 | ||
180 days | ||||||
MSAS | 2.39 | 0.03 | 3.51 | 0.01 | 7.04 | 0.01 |
Global Physical Health | 0.93 | 0.06 | 0.93 | 0.03 | 4.61 | 0.03 |
Global Mental Health | 0.97 | 0.38 | 0.96 | 0.33 | 0.95 | 0.33 |
GSRH | ||||||
Fair | 0.98 | 0.98 | 0.64 | 0.55 | 7.13 | 0.13 |
Good | 0.33 | 0.23 | 0.20 | 0.09 | ||
Very good | NC | NS | NC | NS | ||
Excellent | NC | NS | NC | NS |
DISCUSSION
In this longitudinal cohort study, patients, on average, reported relatively severe symptoms, low PROMIS Global Physical and Mental Health scores, and poor GSRH during the inpatient stay in an urban safety‐net hospital. Symptom severity and Global Physical Health improved, on average, by 30 days before stabilizing, but their poor levels in the hospital did not predict 30‐day hospital‐based utilization events. On the other hand, Global Mental Health and GSRH were stable through hospitalizations, and patients scoring poorly on these measures were at greater risk of utilization events within 14 days of discharge. PRO measures obtained during the 180‐day postdischarge period trended toward distinguishing populations with greater baseline risk of proximate utilization events. However, MSAS physical symptom severity and Global Physical Health were more consistently predictive of these events at statistically significant levels compared to Global Mental Health and GSRH in our relatively small sample of patients. Each of these measures selectively improved the fit‐of‐risk prediction models for hospital‐based utilization.
Some of the heterogeneity in readmission risk is explained by differences in PRO measures. Although the MSAS score and Global Physical Health assessment were reliable predictors of utilization when measured in ambulatory settings, they were less discriminating during acute hospitalizations when everyone, on average, reported severe symptoms and poor function. Our results were consistent with other studies that demonstrated the fairly rapid recovery in symptoms that follow hospitalizations,[28, 29] and these measures may become informative of utilization risk as early as 2 weeks postdischarge. GSRH and Global Mental Health (a measure of health‐related quality of life) only predicted utilizations immediately at hospital discharge. As multidimensional measures that reflect physical, social, and emotional capacity, these measures may indicate vulnerabilities in patients least able to handle the stresses of the early postdischarge period.
There is growing momentum around collecting PRO measures in routine clinical care as quality indicators that capture patient‐centered concerns.[30] Our study explored a novel application of these measures whose routine collection will likely proliferate, not solely for the purpose of helping healthcare systems identify patients at risk of unplanned resource utilization. Although multidimensional PRO measures seldom reflect conditions directly modifiable by simple interventions, we believe that the association between physical symptom burden and utilization in our data reveals a possible target for practice improvement. Hospitalists have contributed enormously to shorter lengths of stay that risk sicker and quicker discharges.[31] To mitigate its potential side effects on symptom management, a discharge plan that acknowledges physical symptoms that sometimes persist or recur beyond the hospitalization may be appropriate. This may be accomplished by ensuring that acute symptoms are resolving, giving clear instructions for symptom management at home, as now the standard of care for conditions like asthma,[32] and explicitly communicating the presence of residual symptoms to providers entrusted with continuity care. As an effective feedback measure that can drive continuous quality improvement, we believe that a technology‐based surveillance strategy that spans both the inpatient and outpatient domains is necessary.[23]
There are some notable similarities and differences between the results of our study and a recent hospital‐based study of PRO measures that used data from the Multi‐Center Hospitalist Project.[16] The Physical Component Score of the SF12 is similar to the PROMIS Global Physical Health score in that both incorporate measures of physical function, perceived health, pain, and energy level. Curiously, the SF12 Physical Component Score, but not the PROMIS Global Physical Health score, was associated with 30‐day rehospitalizations. An important difference between the measures is where the SF12 asks about limitations during the past 4 weeks the PROMIS instrument inquires about physical function in general and levels of fatigue and pain in the past 7 days. Considering most hospitalizations last <7 days, the PROMIS instrument may better reflect the declines associated with the acute illness related to the hospitalization than the SF12 score. Additionally, the discrepancy between the association between hospital‐based GSRH and utilization in our study and the absence, thereof, in Hasan et al. is noteworthy. The difference here may be explained by their use of a 0‐ to 100‐point response scale in contrast to our study's verbally labeled 5‐point scale in the PROMIS instrument. The range of rating scales for survey questions is traditionally governed by the tension between the difficulty with mapping respondents judgment on an excessively large scale on one hand, and the failure of insufficient response options to discriminate between respondents with different underlying judgment on the other.[33] We suspect the former to be a drawback of the unlabeled 100‐point response scale, and conjecture that an association might be found in the Multi‐Center Hospitalist Study data if the responses were grouped into summative categories.
We recognize several limitations in our study. The first is the generalizability of our patient population to others, not insignificantly because of the high proportion of the uninsured (around 70% during the study period) and racial/ethnic minorities among them. Although utilization patterns are clearly affected by socioeconomic status,[34] there may also be differences in the way validated PRO measures are calibrated between patients of public and private healthcare systems.[35] Another limitation is our inability to count utilization events at institutions outside of the CCHHS during our study. However, because the study was conducted prior to Cook County's Medicaid expansion demonstration program as part of the Affordable Care Act,[36] many patients established in our system faced barriers to receiving nonemergency care outside of the CCHHS supporting our assumption that few of our patients were discharged from other hospitals. Causality cannot be established in observational studies. Consequently, high prior‐symptom burden may be associated with utilizations through unmeasured variables. Measures of symptom burden are vulnerable to overendorsement and amplification.[37, 38] Inferences based on statistical significance are affected by sample size, and our conclusions may change if conducted with a larger number of participants. Our response rates were excellent through the survey waves, but we did not achieve perfect follow‐up. Worse levels of PRO responses and higher levels of utilization among censored patients biased our results toward the null. Finally, although we did not find any predominant comorbidities associated with hospital‐based utilizations in our sample, our analyses may be vulnerable to inadequate control for illness severity, which may also have biased our results.
PRO measures are likely to be useful in clinical medicine.[39] But to fully apply the powers of PROs in informing clinically and operationally relevant outcomes, we must actively develop a system for obtaining these measures in routine clinical care. The availability of patient downtime makes hospitalizations conducive to gathering patient‐generated data, and may further enhance patient‐provider communication if survey output was readily available in electronic medical records. Exploring innovative strategies for collecting PROs in the hospital and beyond remains our future work.
Disclosures
Funded by the Agency for Healthcare Research and Quality: R24 HS19481‐01 to support technology implementation. The authors report no conflicts of interest, relevant financial interests, activities, relationships, and affiliations that influenced this work. The first and senior authors had full access to all data and take responsibility for their integrity and the accuracy of the data analysis.
- Potentially avoidable 30‐day hospital readmissions in medical patients: derivation and validation of a prediction model. JAMA Intern Med. 2013;173:632–638. , , , .
- Derivation and validation of an index to predict early death or unplanned readmission after discharge from hospital to the community. CMAJ. 2010;182:551–557. , , , et al.
- Redefining readmission risk factors for general medicine patients. J Hosp Med. 2011;6:54–60. , , , .
- Risk prediction models for hospital readmission: a systematic review. JAMA. 2011;306:1688–1698. , , , et al.
- Predicting mortality and healthcare utilization with a single question. Health Serv Res. 2005;40:1234–1246. , , , .
- Repeated hospitalizations and self‐rated health among the elderly: a multivariate failure time analysis. Am J Epidemiol. 2001;153 3 232–241. , , .
- Screening elders for risk of hospital admission. J Am Geriatr Soc. 1993;41:811–817. , , , , , .
- Baseline quality of life as a predictor of mortality and hospitalization in 5,025 patients with congestive heart failure. SOLVD Investigations. Studies of Left Ventricular Dysfunction Investigators. Am J Cardiol. 1996;78:890–895. , , , et al.
- Using quality of life to predict hospitalization and mortality in patients with obstructive lung disease. Chest. 2002;122:429–436. , , , , .
- Intraindividual change in SF‐36 in ambulatory clinic primary care patients predicted mortality and hospitalizations. J Clin Epidemiol. 2004;57:277–283. , , , .
- Use of health‐related, quality‐of‐life metrics to predict mortality and hospitalizations in community‐dwelling seniors. J Am Geriatr Soc. 2006;54:667–673. , , , et al.
- Medical outcomes study short form‐36: a consistent and powerful predictor of morbidity and mortality in dialysis patients. Am J Kidney Dis. 2003;41:1286–1292. , , , .
- Relationship of depression to increased risk of mortality and rehospitalization in patients with congestive heart failure. Arch Intern Med. 2001;161:1849–1856. , , , et al.
- Depression as a predictor of mortality and hospitalization among hemodialysis patients in the United States and Europe. Kidney Int. 2002;62:199–207. , , , et al.
- Health status predicts long‐term outcomes in outpatients with coronary disease. Circulation. 2002;106:43–49. , , , , .
- Hospital readmission in general medicine patients: a prediction model. J Gen Intern Med. 2010;25:211–219. , , , et al.
- Persistent angina pectoris in ischaemic cardiomyopathy: increased rehospitalization and major adverse cardiac events. Eur J Heart Fail. 2014;16:854–860. , , , , , .
- Relation of dyspnea severity on admission for acute heart failure with outcomes and costs. Am J Cardiol. 2015;115:75–81. , , , et al.
- Two views of self‐rated general health status. Soc Sci Med. 2003;56:203–217. , , .
- Self‐rated health and physical disability in elderly survivors of a major medical event. J Gerontol B Psychol Sci Soc Sci. 1996;51:S96–S104. , , .
- Predicting changes in perceived health status. Am J Public Health. 1984;74:611–614. , , .
- Quality of life before and after intensive care. Anaesthesia. 2005;60:322–329. , , , , .
- Trick WE. Health perceptions and symptom burden in primary care: measuring health using audio computer‐assisted self‐interviews [published online ahead of print December 7, 2014]. Qual Life Res. doi: 10.1007/s11136‐014‐0884‐4. , , , , ,
- Adapting a clinical comorbidity index for use with ICD‐9‐CM administrative databases. J Clin Epidemiol. 1992;45:613–619. , , .
- The Memorial Symptom Assessment Scale: an instrument for the evaluation of symptom prevalence, characteristics and distress. Eur J Cancer. 1994;30A:1326–1336. , , , et al.
- The Memorial Symptom Assessment Scale short form (MSAS‐SF). Cancer. 2000;89:1162–1171. , , , , .
- Development of physical and mental health summary schores from the Patient‐Reported Outcomes Measurement Information System (PROMIS) global items. Qual Life Res. 2009;18:873–880. , , , , .
- Improvements in signs and symptoms during hospitalization for acute heart failure follow different patterns and depend on the measurement scales used: an international, prospective registry to evaluate the evolution of measures of disease severity in acute heart failure (MEASURE‐AHF). J Card Fail. 2008;14:777–784. , , , et al.
- Longitudinal assessment of symptom severity among hospitalized elders diagnosed with cancer, heart failure, and chronic obstructive pulmonary disease. J Hosp Med. 2012;7:567–572. , , , .
- The patient experience and health outcomes. N Engl J Med. 2013;368:201–203. , , , .
- "Quicker and sicker" under Medicare's prospective payment system for hospitals: new evidence on an old issue from a national longitudinal survey. Bull Econ Res. 2011;63:1–27. , , , .
- Agency for Healthcare Research and Quality. Asthma care quality improvement measures. Available at: http://www.ahrq.gov/professionals/quality‐patient‐safety/quality‐resources/tools/asthmaqual/asthmacare/appendix‐d.html. Accessed January 30, 2015.
- The Psychology of Survey Response. New York, NY: Cambridge University Press; 2000. , , .
- Health care for children and youth in the United States: annual report on patterns of coverage, utilization, quality, and expenditures by income. Ambul Pediatr. 2005;5:6–44. , , , et al.
- Levels of symptom burden during chemotherapy for advanced lung cancer: differences between public hospitals and a tertiary cancer center. J Clin Oncol. 2011;29:2859–2865. , , , et al.
- http://kff.org/medicaid/issue-brief/profiles-of-medicaid-outreach-and-enrollment-strategies-the-cook-county-early-expansion-initiative. Published April 7, 2014. Accessed December 2, 2014. . Profiles of Medicaid outreach and enrollment strategies: the Cook County early expansion initiative. The Henry J. Kaiser Family Foundation. Available at:
- A primary care perspective on prevailing assumptions about persistent medically unexplained physical symptoms. Int J Psychiatry Med. 2002;32:125–140. , , .
- Symptom burden and comorbidities impact the consistency of responses on patient‐reported functional outcomes. Arch Phys Med Rehabil. 2014;95:79–86. , , , .
- Implementing patient‐reported outcomes assessment in clinical practice: a review of the options and considerations. Qual Life Res. 2012;21:1305–1314. , , , et al.
- Potentially avoidable 30‐day hospital readmissions in medical patients: derivation and validation of a prediction model. JAMA Intern Med. 2013;173:632–638. , , , .
- Derivation and validation of an index to predict early death or unplanned readmission after discharge from hospital to the community. CMAJ. 2010;182:551–557. , , , et al.
- Redefining readmission risk factors for general medicine patients. J Hosp Med. 2011;6:54–60. , , , .
- Risk prediction models for hospital readmission: a systematic review. JAMA. 2011;306:1688–1698. , , , et al.
- Predicting mortality and healthcare utilization with a single question. Health Serv Res. 2005;40:1234–1246. , , , .
- Repeated hospitalizations and self‐rated health among the elderly: a multivariate failure time analysis. Am J Epidemiol. 2001;153 3 232–241. , , .
- Screening elders for risk of hospital admission. J Am Geriatr Soc. 1993;41:811–817. , , , , , .
- Baseline quality of life as a predictor of mortality and hospitalization in 5,025 patients with congestive heart failure. SOLVD Investigations. Studies of Left Ventricular Dysfunction Investigators. Am J Cardiol. 1996;78:890–895. , , , et al.
- Using quality of life to predict hospitalization and mortality in patients with obstructive lung disease. Chest. 2002;122:429–436. , , , , .
- Intraindividual change in SF‐36 in ambulatory clinic primary care patients predicted mortality and hospitalizations. J Clin Epidemiol. 2004;57:277–283. , , , .
- Use of health‐related, quality‐of‐life metrics to predict mortality and hospitalizations in community‐dwelling seniors. J Am Geriatr Soc. 2006;54:667–673. , , , et al.
- Medical outcomes study short form‐36: a consistent and powerful predictor of morbidity and mortality in dialysis patients. Am J Kidney Dis. 2003;41:1286–1292. , , , .
- Relationship of depression to increased risk of mortality and rehospitalization in patients with congestive heart failure. Arch Intern Med. 2001;161:1849–1856. , , , et al.
- Depression as a predictor of mortality and hospitalization among hemodialysis patients in the United States and Europe. Kidney Int. 2002;62:199–207. , , , et al.
- Health status predicts long‐term outcomes in outpatients with coronary disease. Circulation. 2002;106:43–49. , , , , .
- Hospital readmission in general medicine patients: a prediction model. J Gen Intern Med. 2010;25:211–219. , , , et al.
- Persistent angina pectoris in ischaemic cardiomyopathy: increased rehospitalization and major adverse cardiac events. Eur J Heart Fail. 2014;16:854–860. , , , , , .
- Relation of dyspnea severity on admission for acute heart failure with outcomes and costs. Am J Cardiol. 2015;115:75–81. , , , et al.
- Two views of self‐rated general health status. Soc Sci Med. 2003;56:203–217. , , .
- Self‐rated health and physical disability in elderly survivors of a major medical event. J Gerontol B Psychol Sci Soc Sci. 1996;51:S96–S104. , , .
- Predicting changes in perceived health status. Am J Public Health. 1984;74:611–614. , , .
- Quality of life before and after intensive care. Anaesthesia. 2005;60:322–329. , , , , .
- Trick WE. Health perceptions and symptom burden in primary care: measuring health using audio computer‐assisted self‐interviews [published online ahead of print December 7, 2014]. Qual Life Res. doi: 10.1007/s11136‐014‐0884‐4. , , , , ,
- Adapting a clinical comorbidity index for use with ICD‐9‐CM administrative databases. J Clin Epidemiol. 1992;45:613–619. , , .
- The Memorial Symptom Assessment Scale: an instrument for the evaluation of symptom prevalence, characteristics and distress. Eur J Cancer. 1994;30A:1326–1336. , , , et al.
- The Memorial Symptom Assessment Scale short form (MSAS‐SF). Cancer. 2000;89:1162–1171. , , , , .
- Development of physical and mental health summary schores from the Patient‐Reported Outcomes Measurement Information System (PROMIS) global items. Qual Life Res. 2009;18:873–880. , , , , .
- Improvements in signs and symptoms during hospitalization for acute heart failure follow different patterns and depend on the measurement scales used: an international, prospective registry to evaluate the evolution of measures of disease severity in acute heart failure (MEASURE‐AHF). J Card Fail. 2008;14:777–784. , , , et al.
- Longitudinal assessment of symptom severity among hospitalized elders diagnosed with cancer, heart failure, and chronic obstructive pulmonary disease. J Hosp Med. 2012;7:567–572. , , , .
- The patient experience and health outcomes. N Engl J Med. 2013;368:201–203. , , , .
- "Quicker and sicker" under Medicare's prospective payment system for hospitals: new evidence on an old issue from a national longitudinal survey. Bull Econ Res. 2011;63:1–27. , , , .
- Agency for Healthcare Research and Quality. Asthma care quality improvement measures. Available at: http://www.ahrq.gov/professionals/quality‐patient‐safety/quality‐resources/tools/asthmaqual/asthmacare/appendix‐d.html. Accessed January 30, 2015.
- The Psychology of Survey Response. New York, NY: Cambridge University Press; 2000. , , .
- Health care for children and youth in the United States: annual report on patterns of coverage, utilization, quality, and expenditures by income. Ambul Pediatr. 2005;5:6–44. , , , et al.
- Levels of symptom burden during chemotherapy for advanced lung cancer: differences between public hospitals and a tertiary cancer center. J Clin Oncol. 2011;29:2859–2865. , , , et al.
- http://kff.org/medicaid/issue-brief/profiles-of-medicaid-outreach-and-enrollment-strategies-the-cook-county-early-expansion-initiative. Published April 7, 2014. Accessed December 2, 2014. . Profiles of Medicaid outreach and enrollment strategies: the Cook County early expansion initiative. The Henry J. Kaiser Family Foundation. Available at:
- A primary care perspective on prevailing assumptions about persistent medically unexplained physical symptoms. Int J Psychiatry Med. 2002;32:125–140. , , .
- Symptom burden and comorbidities impact the consistency of responses on patient‐reported functional outcomes. Arch Phys Med Rehabil. 2014;95:79–86. , , , .
- Implementing patient‐reported outcomes assessment in clinical practice: a review of the options and considerations. Qual Life Res. 2012;21:1305–1314. , , , et al.
© 2015 Society of Hospital Medicine
Management of Chronic Conditions
Over the past 2 decades, the care of the hospitalized patient has changed dramatically. Hospitalists now account for the care of more than one‐third of general medicine inpatients, and this number is likely to grow.[1] The emergence of hospital medicine has resulted in a partnership between primary care physicians (PCPs) and hospitalists wherein hospitalists focus on acute medical issues requiring hospitalization, whereas more chronic issues unrelated to the reason for hospitalization remain largely the domain of the PCP.[2, 3]
However, several evolving financial and quality incentives have already begun to blur the distinction between inpatient and outpatient care. First, as private and public payers increasingly scrutinize readmission rates, it has become clear that the responsibility for patient outcomes extends beyond the day of discharge.[4] The birth of Accountable Care Organizations and patient‐centered medical homes may further blur distinctions between what has traditionally constituted inpatient and outpatient care.[5] Bundled payments may force providers to ensure that each visit, whether hospital‐ or clinic‐based, is taken as an opportunity to enact meaningful change.[6] The Centers for Medicare and Medicaid Services (CMS) are already tracking hospital performance on institution of medical therapy for certain conditions regardless of their relatedness to the reason for hospitalization.[7]
No published literature has yet examined the attitudes of inpatient and outpatient providers regarding this issue. Through a case‐based survey conducted at 3 large urban academic medical centers, we aimed to assess opinions among hospitalists and PCPs regarding the role of hospitalists in the management of conditions unrelated to the reason for admission. Our study had 2 main objectives: (1) to determine whether surveyed physicians were more likely to rate an inpatient intervention as appropriate when it related to the reason for admission as compared to interventions unrelated to the reason for admission; and (2) to determine whether these attitudes differed between PCPs and hospitalists.
METHODS
Setting and Subjects
We surveyed hospitalists and hospital‐based PCPs at Beth Israel Deaconess Medical Center (BIDMC), Brigham and Women's Hospital, and Massachusetts General Hospital, 3 large academic medical centers in Boston, Massachusetts. Each hospitalist group includes both teaching and nonteaching services and admits patients from both the surveyed hospital‐based PCP groups and other nonhospital‐based PCP groups. All 3 study sites use electronic medical records with patient information for each hospital‐based PCP available to treating hospitalists.
Survey Design
Using a commercially available online product (SurveyMonkey, Palo Alto, CA), we created a 3‐part case‐based survey instrument. The first section included demographic questions regarding age, sex, primary clinical role (hospitalist or PCP), prior experience as a PCP (for hospitalists only) or a hospitalist (for PCPs only; defined as a position with >30% of clinical time as the attending of record in the inpatient setting), years of clinical experience, and hospital affiliation.
The second section aimed to indirectly assess physician opinions on the appropriateness of inpatient management of conditions unrelated to the reason for admission. It consisted of 6 paired case scenarios, each with an inpatient management decision for a hypothetical hospitalist (Table 1). For each pair, 1 case dealt with management of the condition prompting admission (eg, starting aspirin in a patient admitted with acute nonST‐elevation myocardial infarction). The partner case involved the same intervention (eg, starting aspirin) but for a patient with a chronic condition (eg, history of prior myocardial infarction) and an alternate admitting diagnosis (eg, cellulitis). In an attempt to mitigate concerns regarding the flow of information and communication between providers, the survey asked respondents to assume that the hospitalist has access to the patient's outpatient electronic medical record, and that the hospitalist communicates the details of any hospitalizations at the time of discharge. For each case, the physician was asked to rate the appropriateness of enacting the intervention without discussing it with the PCP on a 5‐point scale from very inappropriate to very appropriate. When a physician answered that an intervention was inappropriate or very inappropriate, an additional question soliciting reasons for inappropriateness was included, with multiple predefined answer choices, as well as the option of a free‐text reply under the other designation.
| |
Starting aspirin (related to the reason for admission) | A 60‐year‐old patient is admitted with a nonST‐elevation MI, medically managed without cardiac catheterization or percutaneous coronary intervention. Knowing that aspirin reduces mortality as part of secondary prevention in cardiovascular disease, how appropriate is it for the hospitalist to start the patient on this medication without discussing it with the primary care physician? |
Starting aspirin (unrelated to the reason for admission) | A 60‐year‐old patient with a past medical history of a prior nonST‐elevation MI that was medically managed is admitted to the hospital for treatment of cellulitis. The hospitalist notes the patient is not on aspirin at home. Knowing that aspirin reduces mortality as part of secondary prevention in cardiovascular disease, how appropriate is it for the hospitalist to start the patient on this medication without discussing it with the primary care physician? |
Starting spironolactone (related to the reason for admission) | A 70‐year‐old patient with a past medical history significant for NYHA class II congestive heart failure (LVEF of 20%) is admitted for acute on chronic, left‐sided systolic congestive heart failure. The patient has been maintained on furosemide, metoprolol, and lisinopril. Admission serum potassium and creatinine are both normal. Knowing that spironolactone decreases mortality in heart failure, how appropriate is it for the hospitalist to start this medication without discussing it with the primary care physician? |
Starting spironolactone (unrelated to the reason for admission) | A 70‐year‐old patient with a past history of NYHA class II congestive heart failure (LVEF of 20%) on furosemide, metoprolol, and lisinopril is admitted with pneumonia. Serum potassium and creatinine are both normal. Knowing that spironolactone decreases mortality in heart failure, how appropriate is it for the hospitalist to start this medication without discussing it with the primary care physician? |
Starting warfarin (related to the reason for admission) | A 75‐year‐old patient with a past medical history of hypertension and diabetes is admitted with new atrial fibrillation. Given the patient's CHADS2 score of 3, the hospitalist calculates that the patient has a significant risk of thromboembolic stroke. Knowing that warfarin will decrease the risk of thromboembolic stroke, how appropriate is it for the hospitalist to start the patient on this medication without discussing it with the primary care physician (assume that an outpatient anticoagulation clinic is able to see the patient within 3 days of discharge)? |
Starting warfarin (unrelated to the reason for admission) | A 75‐year‐old patient with a past medical history of hypertension, diabetes, and atrial fibrillation is admitted with pneumonia. The patient is not anticoagulation therapy. Given the patient's CHADS2 score of 3, the hospitalist calculates that the patient has a significant risk of thromboembolic stroke. Knowing that warfarin will decrease the risk of thromboembolic stroke, how appropriate is it for the hospitalist to start the patient on this medication without discussing it with the primary care physician (assume that an outpatient anticoagulation clinic is able to see the patient within 3 days of discharge)? |
Stopping proton pump inhibitor (related to the reason for admission) | A 65‐year‐old patient with a past medical history of GERD maintained on a proton pump inhibitor is admitted for treatment of Clostridium difficile colitis. The patient denies having any GERD‐like symptoms for several years. Knowing that proton pump inhibitors can increase the risk of C difficile colitis and recurrence (as well as pneumonia and osteoporosis), how appropriate is it for the hospitalist to initiate a taper of this medication without discussing it with the primary care physician? |
Stopping proton pump inhibitor (unrelated to the reason for admission) | A 65‐year‐old patient with a past medical history of GERD maintained on a proton pump inhibitor is admitted for treatment of a urinary tract infection. The patient denies having any GERD‐like symptoms for several years. Knowing that proton pump inhibitors can increase the risk of C difficile colitis and recurrence (as well as pneumonia and osteoporosis), how appropriate is it for the hospitalist to initiate a taper of this medication without discussing it with the primary care physician? |
Stopping statin or fibrate (related to the reason for admission) | A 60‐year‐old patient with a history of hyperlipidemia is admitted with an elevated creatine kinase to 5000. The hospitalist notes that the patient is on both simvastatin and gemfibrozil. The patient's most recent serum LDL was at goal. Knowing that coadministration of simvastatin and gemfibrozil can increase the risk of rhabdomyolysis, how appropriate is it for the hospitalist to stop one of these medications without discussing it with the primary care physician? |
Stopping statin or fibrate (unrelated to the reason for admission) | A 60‐year‐old patient is admitted with an acute diarrheal illness. The hospitalist notes that the patient is on both simvastatin and gemfibrozil. The patient's most recent LDL was at goal. Knowing that coadministration of simvastatin and gemfibrozil can increase the risk of rhabdomyolysis, how appropriate is it for the hospitalist to stop one of these medications without discussing it with the primary care physician? |
Changing statin (related to the reason for admission) | A 65‐year‐old patient with a past medical history of hyperlipidemia on maximum‐dose simvastatin is admitted with a nonST‐elevation MI. The patient's cholesterol is noted to be above goal. Knowing that improving lipid management reduces mortality in cardiovascular disease, how appropriate is it for the hospitalist to replace simvastatin with atorvastatin without discussing it with the primary care physician? |
Changing statin (unrelated to the reason for admission) | A 65‐year‐old patient with a past medical history of a prior nonST‐elevation MI that was medically managed and hyperlipidemia on maximum‐dose simvastatin is admitted with pneumonia. Incidentally, the hospitalist notes that the patient's cholesterol has been above goal for the last 2 years. Knowing that improving lipid management reduces mortality in cardiovascular disease, how appropriate is it for the hospitalist to replace simvastatin with atorvastatin without discussing it with the primary care physician? |
The third section aimed to directly assess physicians' opinions. It consisted of questions regarding the appropriateness of inpatient management of conditions related to and unrelated to a patient's reason for admission.
Prior to administration, we conducted focus groups of hospitalists and PCPs to help hypothesize current physician perceptions on inpatient management, assess physician understanding of survey cases and questions, and to evaluate survey length.
Survey Administration
Between October 23, 2012 and November 10, 2012, 3 emails containing a link to the online survey were sent to all hospitalist and hospital‐based PCPs at the 3 study institutions. The BIDMC Committee on Clinical Investigations, to whom authority was ceded by the remaining 2 study institutions, certified this research protocol as exempt.
Statistical Analysis
We hypothesized that respondents as a whole would be more likely to rate an intervention as appropriate or very appropriate if it was related to the reason for admission, compared to unrelated, and that there would be no difference between PCPs and hospitalists.
We used 2 and Fisher exact tests (where applicable) to compare categorical variables, and a nonparametric median test for continuous variables. We used the Fisher exact test to compare the percent of respondents rating each intervention as appropriate or very appropriate by relatedness or unrelatedness to the reason for admission, and by PCP vs hospitalist. To derive the relative risk (RR) of rating each intervention as appropriate or very appropriate by PCPs compared to hospitalists, adjusting for potential confounders including years out of residency and sex, we used multivariable generalized estimating equation models, each with a Poisson distribution error term, a log link, and an exchangeable working correlation structure to account for dependency of observations arising from clustering at either the hospital or participant level, depending on the comparison: for comparisons within a given case, we controlled for clustering at the hospital level; for comparisons of cases in aggregate, owing to multiple responses from each participant, we controlled for clustering at the individual level.
Assuming a 50% response rate from both PCPs and hospitalists, and that 50% of PCPs would rate a given intervention as appropriate, we calculated that we would have 90% power to detect a 50% increase in the proportion of hospitalists rating an intervention as appropriate as compared to PCPs, using an of .05.
RESULTS
Demographics
One hundred sixty‐two out of 295 providers (55%) responded to the survey (Table 2). The response rate did not differ between hospitalists (70 out of 128; 55%) and PCPs (92 out of 167; 55%). Female respondents made up 58.7% of the PCP and 50.0% of the hospitalist groups (P=0.34). On average, PCPs were older (P<0.001) with a greater median number of years since graduation from residency (P<0.001). A greater percentage of hospitalists spent more than three‐quarters of their time clinically (42.9% vs 19.6%, P=0.009).
Total, n=162 (100.0%) | PCP, n=92 (6.8%) | Hospitalist, n=70 (43.2%) | P Valuea | |
---|---|---|---|---|
| ||||
Hospital, n (%) | ||||
BIDMC | 79 (48.8) | 48 (60.8) | 31 (39.2) | 0.115 |
BWH | 36 (22.2) | 15 (41.7) | 21 (58.3) | |
MGH | 47 (29.0) | 29 (61.7) | 18 (38.3) | |
Sex, n (%) | ||||
Male | 73 (45.1) | 38 (41.3) | 35 (50.0) | 0.339 |
Female | 89 (54.9) | 54 (58.7) | 35 (50.0) | |
Age interval, y, n (%) | ||||
2534 | 36 (22.2) | 9 (9.8) | 27 (38.6) | <0.001 |
3544 | 67 (41.4) | 34 (37.0) | 33 (47.1) | |
4554 | 35 (21.6) | 29 (31.5) | 6 (8.6) | |
5564 | 19 (11.7) | 16 (17.4) | 3 (4.3) | |
6574 | 5 (3.1) | 4 (4.4) | 1 (1.4) | |
Years out of residency, median (IQR) | 10 (417) | 15 (74) | 5 (211) | <0.001 |
Clinical FTE, n (%) | ||||
0.25 | 30 (18.6) | 22 (23.9) | 8 (11.4) | 0.009 |
0.260.50 | 41 (25.3) | 25 (27.2) | 16 (22.9) | |
0.510.75 | 43 (26.5) | 27 (29.4) | 16 (22.9) | |
>0.75 | 48 (29.6) | 18 (19.6) | 30 (42.9) | |
Worked as PCP?b | ||||
Yes | 6 (8.6) | |||
No | 64 (91.4) | |||
Worked as hospitalist? | ||||
Yes | 11 (12.0) | |||
No | 81 (88.0) | |||
AOR for admitted patients | ||||
Always | 16 (17.4) | |||
Mostly | 8 (8.7) | |||
Rarely | 7 (7.6) | |||
Never | 60 (65.2) |
Appropriateness of Inpatient Management Based on Admitting Diagnosis
For each of the 6 case pairings individually and in aggregate, respondents were significantly more likely to deem the intervention appropriate or very appropriate if it was related to the reason for admission, compared to those interventions unrelated to the reason for admission (in aggregate, 78.9% vs 38.8% respectively, P<0.001). For example, whereas 96.9% felt that the addition of aspirin in a patient admitted with acute myocardial infarction (MI) was appropriate, only 54.3% felt it appropriate to start aspirin in a patient with a prior history of MI admitted with cellulitis (P<0.001). Significant differences (all P values <0.001) were seen for all case pairs: starting spironolactone (68.1% when related to the reason for reason for admission vs 43.1% when unrelated to reason for admission); starting warfarin (62.3% vs 23.3%), stopping proton pump inhibitor (72.3% vs 42.8%), stopping statin or fibrate (90.6% vs 28.3%), and changing statin (83.0% vs 40.5%).
Appropriateness of Inpatient Management based on Primary Role
Table 3 compares the percent of PCPs and hospitalists rating each intervention as appropriate or very appropriate, by relatedness of the intervention to the reason for admission. In both unadjusted and adjusted comparisons for all cases in aggregate, PCPs were significantly more likely than hospitalists to rate the inpatient interventions as appropriate or very appropriate when the intervention was related to the reason for admission (83.4% of PCP responses vs 73.0% of hospitalist responses, P<0.001; RR: 1.2, 95% confidence interval [CI]: 1.11.3), unrelated to the reason for admission (44.7% vs 31.1%, P<0.001; RR: 1.5, 95% CI: 1.11.9), and overall (64.1% vs 52.1%, P<0.001; RR: 1.3, 95% CI: 1.11.4).
Relationship to Admission Diagnosis | PCP, n (%) | Hospitalist, n (%) | P Value | Adjusted RR | 95% CI |
---|---|---|---|---|---|
| |||||
Related | 453 (83.4) | 303 (73.0) | <0.001 | 1.2a | 1.11.3 |
Unrelated | 242 (44.7) | 129 (31.1) | <0.001 | 1.5a | 1.11.9 |
Overall | 695 (64.1) | 432 (52.1) | <0.001 | 1.3b | 1.11.4 |
Reasons for Inappropriate Designation
Among those respondents rating an intervention as inappropriate or very inappropriate, the 3 most common reasons selected as explanation for perceived inappropriateness from our predefined answer choices were: This medication will necessitate follow‐up testing/monitoring, for which the PCP will be responsible (chosen by physicians in 49.4% of instances); I am not confident that the hospitalist will have access to all of the medical history necessary to make this decision (35.7%); and Even if the hospitalist has all of the medical history and reviews it, the PCP should be involved in all decisions surrounding new medications (34.6%). The least common explanation chosen was I do not believe this is an appropriate pharmacologic intervention for this particular medical problem (6.5%). See Table 4 for a complete list of explanations, overall and stratified by PCP/hospitalist.
Predefined Reason for Inappropriateness | Total, n=583 (%) | PCP, n=318 (%) | Hospitalist, n=265 (%) | P Value |
---|---|---|---|---|
| ||||
This medication will necessitate follow‐up testing/monitoring, for which the PCP will be responsible. | 288 (49.4) | 151 (47.5) | 137 (51.7) | 0.32 |
I am not confident that the hospitalist will have access to all of the medical history necessary to make this decision. | 208 (35.7) | 98 (30.8) | 110 (41.5) | 0.009 |
Even if the hospitalist has all of the medical history and reviews it, the PCP should be involved in all decisions surrounding new medications. | 201 (34.5) | 125 (39.3) | 76 (28.7) | 0.009 |
I am not confident that the hospitalist will adequately review the medical history necessary to make this decision. | 184 (31.6) | 130 (40.9) | 54 (20.4) | <0.001 |
Even if the hospitalist has all of the medical history, I do not believe hospitalization is the right time to start this new medication | 106 (21.4) | 69 (21.7) | 56 (21.1) | 0.92 |
I am not confident that the hospitalist will appropriately discuss the risks and benefits of this new medication with the patient. | 106 (18.2) | 85 (26.7) | 21 (7.9) | <0.001 |
The benefit of this medication will be too remote to justify starting it in the acute setting. | 66 (11.3) | 40 (12.6) | 26 (9.8) | 0.36 |
I do not believe this is an appropriate pharmacologic intervention for this particular medical problem. | 38 (6.5) | 27 (8.5) | 11 (4.2) | 0.04 |
There were significant differences in the proportion of PCPs and hospitalists choosing several of the prespecified reasons for inappropriateness. Although hospitalists were more likely than PCPs to select I am not confident that the hospitalist will have access to all of the medical history necessary to make this decision (chosen by 41.5% of hospitalists vs 30.8% of PCPs, P=0.009), PCPs were more likely than hospitalists to select, I am not confident that the hospitalist will adequately review the medical history necessary to make this decision (chosen by 40.9% of PCPs vs 20.4% of hospitalists, P<0.001) and I am not confident that the hospitalist will appropriately discuss the risks and benefits of this new medication with the patient (26.7% of PCPs vs 9.8% of hospitalists, P<0.001).
Opinions on Current Management of Conditions Related and Unrelated to Admission
A minority of PCPs and hospitalists agreed or strongly agreed that hospitalists should play a larger role in the management of medical conditions unrelated to the reason for admission (28.1% of PCPs vs 34.8% of hospitalists; P=0.39).
DISCUSSION
In this survey‐based study of PCPs and hospitalists across 3 Boston‐area academic medical centers, we found that: (1) physicians were more likely to see inpatient interventions as appropriate when those interventions dealt with the reason for admission as compared to interventions unrelated to the reason for admission; and (2) PCPs were more likely than hospitalists to feel that inpatient interventions were appropriate, even when they targeted chronic conditions unrelated to the reason for admission. To our knowledge, this study represents the first investigation into the attitudes of PCPs and hospitalists regarding the inpatient management of conditions unrelated to the reason for admission.
That surveyed physicians, regardless of role, were less likely to report an intervention unrelated to the reason for hospitalization as appropriateeven those with likely mortality benefitsuggests that opportunities to affect meaningful change may be missed in a healthcare system that adheres to strict inpatient and outpatient roles. For several of the cases, a change in therapy could lead to benefit soon after implementation. For example, aldosterone antagonists reduce mortality as early as 1 month after initiation in select patients.[8] If a major goal of inpatient care is to reduce 30‐day mortality, it could be argued that hospitalists should more actively adjust congestive heart failure therapy in appropriate inpatients, even when this is not their admitting diagnosis.
For some conditions, CMS is already tracking hospital performance. Since 2003, hospitals have been required to document whether a patient with congestive heart failure (either acute or chronic and regardless of the relationship to admission) was prescribed an angiotensin‐converting enzyme (ACE) inhibitor or angiotensin receptor blocker (ARB) at the time of discharge.[7] CMS has determined that the proven benefits of ACE inhibitors and ARBs confer hospital accountability for their inclusion in appropriate patients, independent of the acuity of heart failure. There are many potential therapeutic maneuvers on which health systems (and their physicians) may be graded, and accepting the view that a hospitalization provides a window of opportunity for medical optimization may allow for more fruitful interventions and more patient‐centered care.
Despite the potential benefits of addressing chronic medical issues during hospitalization, there are important limitations on what can and/or should be done in the hospital setting. Hospitalizations are a time of fluctuating clinical status, which continues beyond discharge and is often accompanied by several medication changes.[9] In our study, more than 20% of those who believed that a medication intervention was inappropriate selected I do not believe hospitalization is the right time to start this new medication as one of their explanations. Although some medication interventions have been shown in randomized controlled trials to reduce short‐term mortality, the ability to generalize these findings to the average hospitalized patient with multiple comorbidities, concurrent medication changes, and rapidly fluctuating clinical status is limited. Furthermore, there are interventions most would agree should not be dealt with in the hospital (eg, screening colonoscopy) and encounters that may be too short to allow for change (eg, 24‐hour observation). These issues notwithstanding, the average 4‐day hospitalization likely provides an opportunity for monitored change that may currently be underutilized.
Our study suggests several additional explanations for physicians' current practice and opinions. Only 6.5% of respondents who answered that an intervention was inappropriate indicated as a justification that I do not believe this is an appropriate pharmacologic intervention for this particular medical problem. This suggests that the hesitancy has little to do with a lack of benefit but instead relates to systems issues (eg, access to all pertinent records and concerns regarding follow‐up testing) and perceived limitations to what a hospitalist should and should not do without actively involving the PCP. There are likely additional concerns that the medical record and/or patient histories do not fully outline the rationale for exclusion or inclusion of particular medications. Advances in information technology that enhance information exchange and enable streamlined communication may help to address these perceived barriers. However, an additional barrier may be trust, as PCPs appear more concerned that hospitalists will not review all the pertinent records or discuss risks and benefits before enacting important medication changes. Increased attempts at communication between hospitalists and outpatient providers may help to build trust and alleviate concerns regarding the loss of information that often occurs both on admission and at discharge.
We also noted that PCPs were more likely than hospitalists to feel that inpatient interventions were appropriate, even when targeting chronic conditions unrelated to the reason for admission. It may be that PCPs, with an increasing number of problems to address per outpatient visit,[10, 11] are more open to hospitalists managing any medical problems during their patients' admissions. At the same time, with increased acuity[12, 13, 14] and shortened length of stays,[15, 16] hospitalists have only a finite amount of time to ensure acute issues are managed, leaving potentially modifiable chronic conditions to the outpatient setting. These differences aside, a minority of both PCPs and hospitalists in our study were ready to embrace the idea of hospitalists playing a larger role in the management of conditions unrelated to the reason for hospitalization.
Even though our study benefits from its multisite design, there are a number of limitations. First, although we crafted our survey with input from general medicine focus groups, our survey instrument has not been validated. In addition, the cases are necessarily contrived and do not take into account the complexities of inpatient medicine. Furthermore, though our goal was to create paired cases that isolate a management decision as being simply based on whether it was related or unrelated to the reason for admission, it is possible that other factors, not captured by our survey, influenced the responses. For example, the benefits of aspirin as part of secondary prevention are not equal to the benefits in an acute MI.[17]
In an attempt to isolate the hospitalists' role in these management decisions, respondents were instructed to assume that the decisions were being made without discussing it with the primary care physician, but that the hospitalist would communicate the details of any hospitalization at the time of discharge. They were also instructed to assume that the hospitalist has access to the patient's outpatient electronic medical record. These assumptions were made to address concerns regarding the flow of information and communication, and to simulate the ideal system from a communication and information accessibility standpoint. Had these assumptions not been placed, the responses may have differed. It is likely that PCPs and hospitalists practicing in systems without shared, accessible inpatient/outpatient medical records would be even more reluctant to enact medication changes unrelated to the reason for admission.
Along the same lines, our physician cohort consisted of several metropolitan academic physician groups, in which hospitalists have had a presence for almost 20 years. As a result, our findings may not be generalizable to other academic hospitals, community‐based hospitalist programs, or nonhospital‐based PCP practices. Finally, we do not know whether survey nonresponders differed from responders in ways that could have meaningfully affected our results.
In conclusion, our findings suggest that both PCPs and hospitalists see the management of conditions unrelated to the reason for admission as less appropriate than the management of conditions related to the reason for admission. Our findings also suggest that PCPs may be more open to this practice when compared to hospitalists. Failure to capitalize on opportunities for meaningful medical interventions, independent of patient location, suggests a possible lack of patient centeredness in the current partnership between PCPs and hospitalists. Further studies should examine existing barriers and investigate interventions designed to address those barriers, in an effort to improve both quality of care and the degree of patient‐centeredness in our current healthcare system.
Disclosures: Dr. Herzig is supported by a grant from the National Institute on Aging (K23 AG042459). Dr. Herzig had full access to all of the data in the study and takes responsibility for the integrity of the data and the accuracy of the data analysis. Author contributions: study concept and design, Breu, Allen‐Dicker, Mueller, Herzig; acquisition of data, Breu, Allen‐Dicker, Mueller, Palamara, Herzig; analysis and interpretation of data, Breu, Allen‐Dicker, Hinami, Herzig; drafting of the manuscript, Breu; critical revision of the manuscript for important intellectual content, Breu, Allen‐Dicker, Mueller, Palamara, Hinami, Herzig; statistical analysis, Allen‐Dicker, Hinami, Herzig; study supervision, Breu, Herzig. This study was presented as a poster at the Society of Hospital Medicine National Meeting, Washington, DC, May 17, 2013.
- Growth in the care of older patients by hospitalists in the United States. N Engl J Med. 2009;360(11):1102–1112. , , , .
- The emerging role of “hospitalists” in the American health care system. N Engl J Med. 1996;335(7):514–517. , .
- An introduction to the hospitalist model. Ann Intern Med. 1999;130(4 pt 2):338–342. .
- Hospital readmission as an accountability measure. JAMA. 2011;305(5):504–505. , .
- A national strategy to put accountable care into practice. Health Aff (Millwood). 2010;29(5):982–990. , , , , .
- Keeping score under a global payment system. N Engl J Med. 2012;366(5):393–395. .
- Reporting Hospital Quality Data for Annual Payment Update. Available at: http://www.cms.gov/Medicare/Quality‐Initiatives‐Patient‐Assessment‐Instruments/HospitalQualityInits/Downloads/HospitalRHQDAPU200808. Accessed December 18, 2013.
- Eplerenone in patients with systolic heart failure and mild symptoms. N Engl J Med. 2011;364(1):11–21. , , , et al.
- How are drug regimen changes during hospitalisation handled after discharge: a cohort study. BMJ Open. 2012;2(6):e001461. , , , , , .
- Primary care visit duration and quality: does good care take longer? Arch Intern Med. 2009;169(20):1866–1872. , , .
- The increasing number of clinical items addressed during the time of adult primary care visits. J Gen Intern Med. 2008;23(12):2058–2065. , , ,
- Multiple chronic conditions among adults aged 45 and over: trends over the past 10 years. NCHS Data Brief. 2012;(100):1–8. , , .
- Prevalence of multiple chronic conditions in the United States' Medicare population. Health Qual Life Outcomes. 2009;7(1):82. , , .
- Multiple chronic conditions: prevalence, health consequences, and implications for quality, care management, and costs. J Gen Intern Med. 2007;22(suppl 3):391–395. , , , et al.
- Associations between reduced hospital length of stay and 30‐day readmission rate and mortality: 14‐year experience in 129 Veterans Affairs hospitals. Ann Intern Med. 2012;157(12):837–845. , , , et al.
- Trends in length of stay and short‐term outcomes among Medicare patients hospitalized for heart failure, 1993–2006. JAMA. 2010;303(21):2141–2147. , , , et al.
- Antithrombotic Trialists' Collaboration. Collaborative meta‐analysis of randomised trials of antiplatelet therapy for prevention of death, myocardial infarction, and stroke in high risk patients. BMJ. 2002;324(7329):71–86.
Over the past 2 decades, the care of the hospitalized patient has changed dramatically. Hospitalists now account for the care of more than one‐third of general medicine inpatients, and this number is likely to grow.[1] The emergence of hospital medicine has resulted in a partnership between primary care physicians (PCPs) and hospitalists wherein hospitalists focus on acute medical issues requiring hospitalization, whereas more chronic issues unrelated to the reason for hospitalization remain largely the domain of the PCP.[2, 3]
However, several evolving financial and quality incentives have already begun to blur the distinction between inpatient and outpatient care. First, as private and public payers increasingly scrutinize readmission rates, it has become clear that the responsibility for patient outcomes extends beyond the day of discharge.[4] The birth of Accountable Care Organizations and patient‐centered medical homes may further blur distinctions between what has traditionally constituted inpatient and outpatient care.[5] Bundled payments may force providers to ensure that each visit, whether hospital‐ or clinic‐based, is taken as an opportunity to enact meaningful change.[6] The Centers for Medicare and Medicaid Services (CMS) are already tracking hospital performance on institution of medical therapy for certain conditions regardless of their relatedness to the reason for hospitalization.[7]
No published literature has yet examined the attitudes of inpatient and outpatient providers regarding this issue. Through a case‐based survey conducted at 3 large urban academic medical centers, we aimed to assess opinions among hospitalists and PCPs regarding the role of hospitalists in the management of conditions unrelated to the reason for admission. Our study had 2 main objectives: (1) to determine whether surveyed physicians were more likely to rate an inpatient intervention as appropriate when it related to the reason for admission as compared to interventions unrelated to the reason for admission; and (2) to determine whether these attitudes differed between PCPs and hospitalists.
METHODS
Setting and Subjects
We surveyed hospitalists and hospital‐based PCPs at Beth Israel Deaconess Medical Center (BIDMC), Brigham and Women's Hospital, and Massachusetts General Hospital, 3 large academic medical centers in Boston, Massachusetts. Each hospitalist group includes both teaching and nonteaching services and admits patients from both the surveyed hospital‐based PCP groups and other nonhospital‐based PCP groups. All 3 study sites use electronic medical records with patient information for each hospital‐based PCP available to treating hospitalists.
Survey Design
Using a commercially available online product (SurveyMonkey, Palo Alto, CA), we created a 3‐part case‐based survey instrument. The first section included demographic questions regarding age, sex, primary clinical role (hospitalist or PCP), prior experience as a PCP (for hospitalists only) or a hospitalist (for PCPs only; defined as a position with >30% of clinical time as the attending of record in the inpatient setting), years of clinical experience, and hospital affiliation.
The second section aimed to indirectly assess physician opinions on the appropriateness of inpatient management of conditions unrelated to the reason for admission. It consisted of 6 paired case scenarios, each with an inpatient management decision for a hypothetical hospitalist (Table 1). For each pair, 1 case dealt with management of the condition prompting admission (eg, starting aspirin in a patient admitted with acute nonST‐elevation myocardial infarction). The partner case involved the same intervention (eg, starting aspirin) but for a patient with a chronic condition (eg, history of prior myocardial infarction) and an alternate admitting diagnosis (eg, cellulitis). In an attempt to mitigate concerns regarding the flow of information and communication between providers, the survey asked respondents to assume that the hospitalist has access to the patient's outpatient electronic medical record, and that the hospitalist communicates the details of any hospitalizations at the time of discharge. For each case, the physician was asked to rate the appropriateness of enacting the intervention without discussing it with the PCP on a 5‐point scale from very inappropriate to very appropriate. When a physician answered that an intervention was inappropriate or very inappropriate, an additional question soliciting reasons for inappropriateness was included, with multiple predefined answer choices, as well as the option of a free‐text reply under the other designation.
| |
Starting aspirin (related to the reason for admission) | A 60‐year‐old patient is admitted with a nonST‐elevation MI, medically managed without cardiac catheterization or percutaneous coronary intervention. Knowing that aspirin reduces mortality as part of secondary prevention in cardiovascular disease, how appropriate is it for the hospitalist to start the patient on this medication without discussing it with the primary care physician? |
Starting aspirin (unrelated to the reason for admission) | A 60‐year‐old patient with a past medical history of a prior nonST‐elevation MI that was medically managed is admitted to the hospital for treatment of cellulitis. The hospitalist notes the patient is not on aspirin at home. Knowing that aspirin reduces mortality as part of secondary prevention in cardiovascular disease, how appropriate is it for the hospitalist to start the patient on this medication without discussing it with the primary care physician? |
Starting spironolactone (related to the reason for admission) | A 70‐year‐old patient with a past medical history significant for NYHA class II congestive heart failure (LVEF of 20%) is admitted for acute on chronic, left‐sided systolic congestive heart failure. The patient has been maintained on furosemide, metoprolol, and lisinopril. Admission serum potassium and creatinine are both normal. Knowing that spironolactone decreases mortality in heart failure, how appropriate is it for the hospitalist to start this medication without discussing it with the primary care physician? |
Starting spironolactone (unrelated to the reason for admission) | A 70‐year‐old patient with a past history of NYHA class II congestive heart failure (LVEF of 20%) on furosemide, metoprolol, and lisinopril is admitted with pneumonia. Serum potassium and creatinine are both normal. Knowing that spironolactone decreases mortality in heart failure, how appropriate is it for the hospitalist to start this medication without discussing it with the primary care physician? |
Starting warfarin (related to the reason for admission) | A 75‐year‐old patient with a past medical history of hypertension and diabetes is admitted with new atrial fibrillation. Given the patient's CHADS2 score of 3, the hospitalist calculates that the patient has a significant risk of thromboembolic stroke. Knowing that warfarin will decrease the risk of thromboembolic stroke, how appropriate is it for the hospitalist to start the patient on this medication without discussing it with the primary care physician (assume that an outpatient anticoagulation clinic is able to see the patient within 3 days of discharge)? |
Starting warfarin (unrelated to the reason for admission) | A 75‐year‐old patient with a past medical history of hypertension, diabetes, and atrial fibrillation is admitted with pneumonia. The patient is not anticoagulation therapy. Given the patient's CHADS2 score of 3, the hospitalist calculates that the patient has a significant risk of thromboembolic stroke. Knowing that warfarin will decrease the risk of thromboembolic stroke, how appropriate is it for the hospitalist to start the patient on this medication without discussing it with the primary care physician (assume that an outpatient anticoagulation clinic is able to see the patient within 3 days of discharge)? |
Stopping proton pump inhibitor (related to the reason for admission) | A 65‐year‐old patient with a past medical history of GERD maintained on a proton pump inhibitor is admitted for treatment of Clostridium difficile colitis. The patient denies having any GERD‐like symptoms for several years. Knowing that proton pump inhibitors can increase the risk of C difficile colitis and recurrence (as well as pneumonia and osteoporosis), how appropriate is it for the hospitalist to initiate a taper of this medication without discussing it with the primary care physician? |
Stopping proton pump inhibitor (unrelated to the reason for admission) | A 65‐year‐old patient with a past medical history of GERD maintained on a proton pump inhibitor is admitted for treatment of a urinary tract infection. The patient denies having any GERD‐like symptoms for several years. Knowing that proton pump inhibitors can increase the risk of C difficile colitis and recurrence (as well as pneumonia and osteoporosis), how appropriate is it for the hospitalist to initiate a taper of this medication without discussing it with the primary care physician? |
Stopping statin or fibrate (related to the reason for admission) | A 60‐year‐old patient with a history of hyperlipidemia is admitted with an elevated creatine kinase to 5000. The hospitalist notes that the patient is on both simvastatin and gemfibrozil. The patient's most recent serum LDL was at goal. Knowing that coadministration of simvastatin and gemfibrozil can increase the risk of rhabdomyolysis, how appropriate is it for the hospitalist to stop one of these medications without discussing it with the primary care physician? |
Stopping statin or fibrate (unrelated to the reason for admission) | A 60‐year‐old patient is admitted with an acute diarrheal illness. The hospitalist notes that the patient is on both simvastatin and gemfibrozil. The patient's most recent LDL was at goal. Knowing that coadministration of simvastatin and gemfibrozil can increase the risk of rhabdomyolysis, how appropriate is it for the hospitalist to stop one of these medications without discussing it with the primary care physician? |
Changing statin (related to the reason for admission) | A 65‐year‐old patient with a past medical history of hyperlipidemia on maximum‐dose simvastatin is admitted with a nonST‐elevation MI. The patient's cholesterol is noted to be above goal. Knowing that improving lipid management reduces mortality in cardiovascular disease, how appropriate is it for the hospitalist to replace simvastatin with atorvastatin without discussing it with the primary care physician? |
Changing statin (unrelated to the reason for admission) | A 65‐year‐old patient with a past medical history of a prior nonST‐elevation MI that was medically managed and hyperlipidemia on maximum‐dose simvastatin is admitted with pneumonia. Incidentally, the hospitalist notes that the patient's cholesterol has been above goal for the last 2 years. Knowing that improving lipid management reduces mortality in cardiovascular disease, how appropriate is it for the hospitalist to replace simvastatin with atorvastatin without discussing it with the primary care physician? |
The third section aimed to directly assess physicians' opinions. It consisted of questions regarding the appropriateness of inpatient management of conditions related to and unrelated to a patient's reason for admission.
Prior to administration, we conducted focus groups of hospitalists and PCPs to help hypothesize current physician perceptions on inpatient management, assess physician understanding of survey cases and questions, and to evaluate survey length.
Survey Administration
Between October 23, 2012 and November 10, 2012, 3 emails containing a link to the online survey were sent to all hospitalist and hospital‐based PCPs at the 3 study institutions. The BIDMC Committee on Clinical Investigations, to whom authority was ceded by the remaining 2 study institutions, certified this research protocol as exempt.
Statistical Analysis
We hypothesized that respondents as a whole would be more likely to rate an intervention as appropriate or very appropriate if it was related to the reason for admission, compared to unrelated, and that there would be no difference between PCPs and hospitalists.
We used 2 and Fisher exact tests (where applicable) to compare categorical variables, and a nonparametric median test for continuous variables. We used the Fisher exact test to compare the percent of respondents rating each intervention as appropriate or very appropriate by relatedness or unrelatedness to the reason for admission, and by PCP vs hospitalist. To derive the relative risk (RR) of rating each intervention as appropriate or very appropriate by PCPs compared to hospitalists, adjusting for potential confounders including years out of residency and sex, we used multivariable generalized estimating equation models, each with a Poisson distribution error term, a log link, and an exchangeable working correlation structure to account for dependency of observations arising from clustering at either the hospital or participant level, depending on the comparison: for comparisons within a given case, we controlled for clustering at the hospital level; for comparisons of cases in aggregate, owing to multiple responses from each participant, we controlled for clustering at the individual level.
Assuming a 50% response rate from both PCPs and hospitalists, and that 50% of PCPs would rate a given intervention as appropriate, we calculated that we would have 90% power to detect a 50% increase in the proportion of hospitalists rating an intervention as appropriate as compared to PCPs, using an of .05.
RESULTS
Demographics
One hundred sixty‐two out of 295 providers (55%) responded to the survey (Table 2). The response rate did not differ between hospitalists (70 out of 128; 55%) and PCPs (92 out of 167; 55%). Female respondents made up 58.7% of the PCP and 50.0% of the hospitalist groups (P=0.34). On average, PCPs were older (P<0.001) with a greater median number of years since graduation from residency (P<0.001). A greater percentage of hospitalists spent more than three‐quarters of their time clinically (42.9% vs 19.6%, P=0.009).
Total, n=162 (100.0%) | PCP, n=92 (6.8%) | Hospitalist, n=70 (43.2%) | P Valuea | |
---|---|---|---|---|
| ||||
Hospital, n (%) | ||||
BIDMC | 79 (48.8) | 48 (60.8) | 31 (39.2) | 0.115 |
BWH | 36 (22.2) | 15 (41.7) | 21 (58.3) | |
MGH | 47 (29.0) | 29 (61.7) | 18 (38.3) | |
Sex, n (%) | ||||
Male | 73 (45.1) | 38 (41.3) | 35 (50.0) | 0.339 |
Female | 89 (54.9) | 54 (58.7) | 35 (50.0) | |
Age interval, y, n (%) | ||||
2534 | 36 (22.2) | 9 (9.8) | 27 (38.6) | <0.001 |
3544 | 67 (41.4) | 34 (37.0) | 33 (47.1) | |
4554 | 35 (21.6) | 29 (31.5) | 6 (8.6) | |
5564 | 19 (11.7) | 16 (17.4) | 3 (4.3) | |
6574 | 5 (3.1) | 4 (4.4) | 1 (1.4) | |
Years out of residency, median (IQR) | 10 (417) | 15 (74) | 5 (211) | <0.001 |
Clinical FTE, n (%) | ||||
0.25 | 30 (18.6) | 22 (23.9) | 8 (11.4) | 0.009 |
0.260.50 | 41 (25.3) | 25 (27.2) | 16 (22.9) | |
0.510.75 | 43 (26.5) | 27 (29.4) | 16 (22.9) | |
>0.75 | 48 (29.6) | 18 (19.6) | 30 (42.9) | |
Worked as PCP?b | ||||
Yes | 6 (8.6) | |||
No | 64 (91.4) | |||
Worked as hospitalist? | ||||
Yes | 11 (12.0) | |||
No | 81 (88.0) | |||
AOR for admitted patients | ||||
Always | 16 (17.4) | |||
Mostly | 8 (8.7) | |||
Rarely | 7 (7.6) | |||
Never | 60 (65.2) |
Appropriateness of Inpatient Management Based on Admitting Diagnosis
For each of the 6 case pairings individually and in aggregate, respondents were significantly more likely to deem the intervention appropriate or very appropriate if it was related to the reason for admission, compared to those interventions unrelated to the reason for admission (in aggregate, 78.9% vs 38.8% respectively, P<0.001). For example, whereas 96.9% felt that the addition of aspirin in a patient admitted with acute myocardial infarction (MI) was appropriate, only 54.3% felt it appropriate to start aspirin in a patient with a prior history of MI admitted with cellulitis (P<0.001). Significant differences (all P values <0.001) were seen for all case pairs: starting spironolactone (68.1% when related to the reason for reason for admission vs 43.1% when unrelated to reason for admission); starting warfarin (62.3% vs 23.3%), stopping proton pump inhibitor (72.3% vs 42.8%), stopping statin or fibrate (90.6% vs 28.3%), and changing statin (83.0% vs 40.5%).
Appropriateness of Inpatient Management based on Primary Role
Table 3 compares the percent of PCPs and hospitalists rating each intervention as appropriate or very appropriate, by relatedness of the intervention to the reason for admission. In both unadjusted and adjusted comparisons for all cases in aggregate, PCPs were significantly more likely than hospitalists to rate the inpatient interventions as appropriate or very appropriate when the intervention was related to the reason for admission (83.4% of PCP responses vs 73.0% of hospitalist responses, P<0.001; RR: 1.2, 95% confidence interval [CI]: 1.11.3), unrelated to the reason for admission (44.7% vs 31.1%, P<0.001; RR: 1.5, 95% CI: 1.11.9), and overall (64.1% vs 52.1%, P<0.001; RR: 1.3, 95% CI: 1.11.4).
Relationship to Admission Diagnosis | PCP, n (%) | Hospitalist, n (%) | P Value | Adjusted RR | 95% CI |
---|---|---|---|---|---|
| |||||
Related | 453 (83.4) | 303 (73.0) | <0.001 | 1.2a | 1.11.3 |
Unrelated | 242 (44.7) | 129 (31.1) | <0.001 | 1.5a | 1.11.9 |
Overall | 695 (64.1) | 432 (52.1) | <0.001 | 1.3b | 1.11.4 |
Reasons for Inappropriate Designation
Among those respondents rating an intervention as inappropriate or very inappropriate, the 3 most common reasons selected as explanation for perceived inappropriateness from our predefined answer choices were: This medication will necessitate follow‐up testing/monitoring, for which the PCP will be responsible (chosen by physicians in 49.4% of instances); I am not confident that the hospitalist will have access to all of the medical history necessary to make this decision (35.7%); and Even if the hospitalist has all of the medical history and reviews it, the PCP should be involved in all decisions surrounding new medications (34.6%). The least common explanation chosen was I do not believe this is an appropriate pharmacologic intervention for this particular medical problem (6.5%). See Table 4 for a complete list of explanations, overall and stratified by PCP/hospitalist.
Predefined Reason for Inappropriateness | Total, n=583 (%) | PCP, n=318 (%) | Hospitalist, n=265 (%) | P Value |
---|---|---|---|---|
| ||||
This medication will necessitate follow‐up testing/monitoring, for which the PCP will be responsible. | 288 (49.4) | 151 (47.5) | 137 (51.7) | 0.32 |
I am not confident that the hospitalist will have access to all of the medical history necessary to make this decision. | 208 (35.7) | 98 (30.8) | 110 (41.5) | 0.009 |
Even if the hospitalist has all of the medical history and reviews it, the PCP should be involved in all decisions surrounding new medications. | 201 (34.5) | 125 (39.3) | 76 (28.7) | 0.009 |
I am not confident that the hospitalist will adequately review the medical history necessary to make this decision. | 184 (31.6) | 130 (40.9) | 54 (20.4) | <0.001 |
Even if the hospitalist has all of the medical history, I do not believe hospitalization is the right time to start this new medication | 106 (21.4) | 69 (21.7) | 56 (21.1) | 0.92 |
I am not confident that the hospitalist will appropriately discuss the risks and benefits of this new medication with the patient. | 106 (18.2) | 85 (26.7) | 21 (7.9) | <0.001 |
The benefit of this medication will be too remote to justify starting it in the acute setting. | 66 (11.3) | 40 (12.6) | 26 (9.8) | 0.36 |
I do not believe this is an appropriate pharmacologic intervention for this particular medical problem. | 38 (6.5) | 27 (8.5) | 11 (4.2) | 0.04 |
There were significant differences in the proportion of PCPs and hospitalists choosing several of the prespecified reasons for inappropriateness. Although hospitalists were more likely than PCPs to select I am not confident that the hospitalist will have access to all of the medical history necessary to make this decision (chosen by 41.5% of hospitalists vs 30.8% of PCPs, P=0.009), PCPs were more likely than hospitalists to select, I am not confident that the hospitalist will adequately review the medical history necessary to make this decision (chosen by 40.9% of PCPs vs 20.4% of hospitalists, P<0.001) and I am not confident that the hospitalist will appropriately discuss the risks and benefits of this new medication with the patient (26.7% of PCPs vs 9.8% of hospitalists, P<0.001).
Opinions on Current Management of Conditions Related and Unrelated to Admission
A minority of PCPs and hospitalists agreed or strongly agreed that hospitalists should play a larger role in the management of medical conditions unrelated to the reason for admission (28.1% of PCPs vs 34.8% of hospitalists; P=0.39).
DISCUSSION
In this survey‐based study of PCPs and hospitalists across 3 Boston‐area academic medical centers, we found that: (1) physicians were more likely to see inpatient interventions as appropriate when those interventions dealt with the reason for admission as compared to interventions unrelated to the reason for admission; and (2) PCPs were more likely than hospitalists to feel that inpatient interventions were appropriate, even when they targeted chronic conditions unrelated to the reason for admission. To our knowledge, this study represents the first investigation into the attitudes of PCPs and hospitalists regarding the inpatient management of conditions unrelated to the reason for admission.
That surveyed physicians, regardless of role, were less likely to report an intervention unrelated to the reason for hospitalization as appropriateeven those with likely mortality benefitsuggests that opportunities to affect meaningful change may be missed in a healthcare system that adheres to strict inpatient and outpatient roles. For several of the cases, a change in therapy could lead to benefit soon after implementation. For example, aldosterone antagonists reduce mortality as early as 1 month after initiation in select patients.[8] If a major goal of inpatient care is to reduce 30‐day mortality, it could be argued that hospitalists should more actively adjust congestive heart failure therapy in appropriate inpatients, even when this is not their admitting diagnosis.
For some conditions, CMS is already tracking hospital performance. Since 2003, hospitals have been required to document whether a patient with congestive heart failure (either acute or chronic and regardless of the relationship to admission) was prescribed an angiotensin‐converting enzyme (ACE) inhibitor or angiotensin receptor blocker (ARB) at the time of discharge.[7] CMS has determined that the proven benefits of ACE inhibitors and ARBs confer hospital accountability for their inclusion in appropriate patients, independent of the acuity of heart failure. There are many potential therapeutic maneuvers on which health systems (and their physicians) may be graded, and accepting the view that a hospitalization provides a window of opportunity for medical optimization may allow for more fruitful interventions and more patient‐centered care.
Despite the potential benefits of addressing chronic medical issues during hospitalization, there are important limitations on what can and/or should be done in the hospital setting. Hospitalizations are a time of fluctuating clinical status, which continues beyond discharge and is often accompanied by several medication changes.[9] In our study, more than 20% of those who believed that a medication intervention was inappropriate selected I do not believe hospitalization is the right time to start this new medication as one of their explanations. Although some medication interventions have been shown in randomized controlled trials to reduce short‐term mortality, the ability to generalize these findings to the average hospitalized patient with multiple comorbidities, concurrent medication changes, and rapidly fluctuating clinical status is limited. Furthermore, there are interventions most would agree should not be dealt with in the hospital (eg, screening colonoscopy) and encounters that may be too short to allow for change (eg, 24‐hour observation). These issues notwithstanding, the average 4‐day hospitalization likely provides an opportunity for monitored change that may currently be underutilized.
Our study suggests several additional explanations for physicians' current practice and opinions. Only 6.5% of respondents who answered that an intervention was inappropriate indicated as a justification that I do not believe this is an appropriate pharmacologic intervention for this particular medical problem. This suggests that the hesitancy has little to do with a lack of benefit but instead relates to systems issues (eg, access to all pertinent records and concerns regarding follow‐up testing) and perceived limitations to what a hospitalist should and should not do without actively involving the PCP. There are likely additional concerns that the medical record and/or patient histories do not fully outline the rationale for exclusion or inclusion of particular medications. Advances in information technology that enhance information exchange and enable streamlined communication may help to address these perceived barriers. However, an additional barrier may be trust, as PCPs appear more concerned that hospitalists will not review all the pertinent records or discuss risks and benefits before enacting important medication changes. Increased attempts at communication between hospitalists and outpatient providers may help to build trust and alleviate concerns regarding the loss of information that often occurs both on admission and at discharge.
We also noted that PCPs were more likely than hospitalists to feel that inpatient interventions were appropriate, even when targeting chronic conditions unrelated to the reason for admission. It may be that PCPs, with an increasing number of problems to address per outpatient visit,[10, 11] are more open to hospitalists managing any medical problems during their patients' admissions. At the same time, with increased acuity[12, 13, 14] and shortened length of stays,[15, 16] hospitalists have only a finite amount of time to ensure acute issues are managed, leaving potentially modifiable chronic conditions to the outpatient setting. These differences aside, a minority of both PCPs and hospitalists in our study were ready to embrace the idea of hospitalists playing a larger role in the management of conditions unrelated to the reason for hospitalization.
Even though our study benefits from its multisite design, there are a number of limitations. First, although we crafted our survey with input from general medicine focus groups, our survey instrument has not been validated. In addition, the cases are necessarily contrived and do not take into account the complexities of inpatient medicine. Furthermore, though our goal was to create paired cases that isolate a management decision as being simply based on whether it was related or unrelated to the reason for admission, it is possible that other factors, not captured by our survey, influenced the responses. For example, the benefits of aspirin as part of secondary prevention are not equal to the benefits in an acute MI.[17]
In an attempt to isolate the hospitalists' role in these management decisions, respondents were instructed to assume that the decisions were being made without discussing it with the primary care physician, but that the hospitalist would communicate the details of any hospitalization at the time of discharge. They were also instructed to assume that the hospitalist has access to the patient's outpatient electronic medical record. These assumptions were made to address concerns regarding the flow of information and communication, and to simulate the ideal system from a communication and information accessibility standpoint. Had these assumptions not been placed, the responses may have differed. It is likely that PCPs and hospitalists practicing in systems without shared, accessible inpatient/outpatient medical records would be even more reluctant to enact medication changes unrelated to the reason for admission.
Along the same lines, our physician cohort consisted of several metropolitan academic physician groups, in which hospitalists have had a presence for almost 20 years. As a result, our findings may not be generalizable to other academic hospitals, community‐based hospitalist programs, or nonhospital‐based PCP practices. Finally, we do not know whether survey nonresponders differed from responders in ways that could have meaningfully affected our results.
In conclusion, our findings suggest that both PCPs and hospitalists see the management of conditions unrelated to the reason for admission as less appropriate than the management of conditions related to the reason for admission. Our findings also suggest that PCPs may be more open to this practice when compared to hospitalists. Failure to capitalize on opportunities for meaningful medical interventions, independent of patient location, suggests a possible lack of patient centeredness in the current partnership between PCPs and hospitalists. Further studies should examine existing barriers and investigate interventions designed to address those barriers, in an effort to improve both quality of care and the degree of patient‐centeredness in our current healthcare system.
Disclosures: Dr. Herzig is supported by a grant from the National Institute on Aging (K23 AG042459). Dr. Herzig had full access to all of the data in the study and takes responsibility for the integrity of the data and the accuracy of the data analysis. Author contributions: study concept and design, Breu, Allen‐Dicker, Mueller, Herzig; acquisition of data, Breu, Allen‐Dicker, Mueller, Palamara, Herzig; analysis and interpretation of data, Breu, Allen‐Dicker, Hinami, Herzig; drafting of the manuscript, Breu; critical revision of the manuscript for important intellectual content, Breu, Allen‐Dicker, Mueller, Palamara, Hinami, Herzig; statistical analysis, Allen‐Dicker, Hinami, Herzig; study supervision, Breu, Herzig. This study was presented as a poster at the Society of Hospital Medicine National Meeting, Washington, DC, May 17, 2013.
Over the past 2 decades, the care of the hospitalized patient has changed dramatically. Hospitalists now account for the care of more than one‐third of general medicine inpatients, and this number is likely to grow.[1] The emergence of hospital medicine has resulted in a partnership between primary care physicians (PCPs) and hospitalists wherein hospitalists focus on acute medical issues requiring hospitalization, whereas more chronic issues unrelated to the reason for hospitalization remain largely the domain of the PCP.[2, 3]
However, several evolving financial and quality incentives have already begun to blur the distinction between inpatient and outpatient care. First, as private and public payers increasingly scrutinize readmission rates, it has become clear that the responsibility for patient outcomes extends beyond the day of discharge.[4] The birth of Accountable Care Organizations and patient‐centered medical homes may further blur distinctions between what has traditionally constituted inpatient and outpatient care.[5] Bundled payments may force providers to ensure that each visit, whether hospital‐ or clinic‐based, is taken as an opportunity to enact meaningful change.[6] The Centers for Medicare and Medicaid Services (CMS) are already tracking hospital performance on institution of medical therapy for certain conditions regardless of their relatedness to the reason for hospitalization.[7]
No published literature has yet examined the attitudes of inpatient and outpatient providers regarding this issue. Through a case‐based survey conducted at 3 large urban academic medical centers, we aimed to assess opinions among hospitalists and PCPs regarding the role of hospitalists in the management of conditions unrelated to the reason for admission. Our study had 2 main objectives: (1) to determine whether surveyed physicians were more likely to rate an inpatient intervention as appropriate when it related to the reason for admission as compared to interventions unrelated to the reason for admission; and (2) to determine whether these attitudes differed between PCPs and hospitalists.
METHODS
Setting and Subjects
We surveyed hospitalists and hospital‐based PCPs at Beth Israel Deaconess Medical Center (BIDMC), Brigham and Women's Hospital, and Massachusetts General Hospital, 3 large academic medical centers in Boston, Massachusetts. Each hospitalist group includes both teaching and nonteaching services and admits patients from both the surveyed hospital‐based PCP groups and other nonhospital‐based PCP groups. All 3 study sites use electronic medical records with patient information for each hospital‐based PCP available to treating hospitalists.
Survey Design
Using a commercially available online product (SurveyMonkey, Palo Alto, CA), we created a 3‐part case‐based survey instrument. The first section included demographic questions regarding age, sex, primary clinical role (hospitalist or PCP), prior experience as a PCP (for hospitalists only) or a hospitalist (for PCPs only; defined as a position with >30% of clinical time as the attending of record in the inpatient setting), years of clinical experience, and hospital affiliation.
The second section aimed to indirectly assess physician opinions on the appropriateness of inpatient management of conditions unrelated to the reason for admission. It consisted of 6 paired case scenarios, each with an inpatient management decision for a hypothetical hospitalist (Table 1). For each pair, 1 case dealt with management of the condition prompting admission (eg, starting aspirin in a patient admitted with acute nonST‐elevation myocardial infarction). The partner case involved the same intervention (eg, starting aspirin) but for a patient with a chronic condition (eg, history of prior myocardial infarction) and an alternate admitting diagnosis (eg, cellulitis). In an attempt to mitigate concerns regarding the flow of information and communication between providers, the survey asked respondents to assume that the hospitalist has access to the patient's outpatient electronic medical record, and that the hospitalist communicates the details of any hospitalizations at the time of discharge. For each case, the physician was asked to rate the appropriateness of enacting the intervention without discussing it with the PCP on a 5‐point scale from very inappropriate to very appropriate. When a physician answered that an intervention was inappropriate or very inappropriate, an additional question soliciting reasons for inappropriateness was included, with multiple predefined answer choices, as well as the option of a free‐text reply under the other designation.
| |
Starting aspirin (related to the reason for admission) | A 60‐year‐old patient is admitted with a nonST‐elevation MI, medically managed without cardiac catheterization or percutaneous coronary intervention. Knowing that aspirin reduces mortality as part of secondary prevention in cardiovascular disease, how appropriate is it for the hospitalist to start the patient on this medication without discussing it with the primary care physician? |
Starting aspirin (unrelated to the reason for admission) | A 60‐year‐old patient with a past medical history of a prior nonST‐elevation MI that was medically managed is admitted to the hospital for treatment of cellulitis. The hospitalist notes the patient is not on aspirin at home. Knowing that aspirin reduces mortality as part of secondary prevention in cardiovascular disease, how appropriate is it for the hospitalist to start the patient on this medication without discussing it with the primary care physician? |
Starting spironolactone (related to the reason for admission) | A 70‐year‐old patient with a past medical history significant for NYHA class II congestive heart failure (LVEF of 20%) is admitted for acute on chronic, left‐sided systolic congestive heart failure. The patient has been maintained on furosemide, metoprolol, and lisinopril. Admission serum potassium and creatinine are both normal. Knowing that spironolactone decreases mortality in heart failure, how appropriate is it for the hospitalist to start this medication without discussing it with the primary care physician? |
Starting spironolactone (unrelated to the reason for admission) | A 70‐year‐old patient with a past history of NYHA class II congestive heart failure (LVEF of 20%) on furosemide, metoprolol, and lisinopril is admitted with pneumonia. Serum potassium and creatinine are both normal. Knowing that spironolactone decreases mortality in heart failure, how appropriate is it for the hospitalist to start this medication without discussing it with the primary care physician? |
Starting warfarin (related to the reason for admission) | A 75‐year‐old patient with a past medical history of hypertension and diabetes is admitted with new atrial fibrillation. Given the patient's CHADS2 score of 3, the hospitalist calculates that the patient has a significant risk of thromboembolic stroke. Knowing that warfarin will decrease the risk of thromboembolic stroke, how appropriate is it for the hospitalist to start the patient on this medication without discussing it with the primary care physician (assume that an outpatient anticoagulation clinic is able to see the patient within 3 days of discharge)? |
Starting warfarin (unrelated to the reason for admission) | A 75‐year‐old patient with a past medical history of hypertension, diabetes, and atrial fibrillation is admitted with pneumonia. The patient is not anticoagulation therapy. Given the patient's CHADS2 score of 3, the hospitalist calculates that the patient has a significant risk of thromboembolic stroke. Knowing that warfarin will decrease the risk of thromboembolic stroke, how appropriate is it for the hospitalist to start the patient on this medication without discussing it with the primary care physician (assume that an outpatient anticoagulation clinic is able to see the patient within 3 days of discharge)? |
Stopping proton pump inhibitor (related to the reason for admission) | A 65‐year‐old patient with a past medical history of GERD maintained on a proton pump inhibitor is admitted for treatment of Clostridium difficile colitis. The patient denies having any GERD‐like symptoms for several years. Knowing that proton pump inhibitors can increase the risk of C difficile colitis and recurrence (as well as pneumonia and osteoporosis), how appropriate is it for the hospitalist to initiate a taper of this medication without discussing it with the primary care physician? |
Stopping proton pump inhibitor (unrelated to the reason for admission) | A 65‐year‐old patient with a past medical history of GERD maintained on a proton pump inhibitor is admitted for treatment of a urinary tract infection. The patient denies having any GERD‐like symptoms for several years. Knowing that proton pump inhibitors can increase the risk of C difficile colitis and recurrence (as well as pneumonia and osteoporosis), how appropriate is it for the hospitalist to initiate a taper of this medication without discussing it with the primary care physician? |
Stopping statin or fibrate (related to the reason for admission) | A 60‐year‐old patient with a history of hyperlipidemia is admitted with an elevated creatine kinase to 5000. The hospitalist notes that the patient is on both simvastatin and gemfibrozil. The patient's most recent serum LDL was at goal. Knowing that coadministration of simvastatin and gemfibrozil can increase the risk of rhabdomyolysis, how appropriate is it for the hospitalist to stop one of these medications without discussing it with the primary care physician? |
Stopping statin or fibrate (unrelated to the reason for admission) | A 60‐year‐old patient is admitted with an acute diarrheal illness. The hospitalist notes that the patient is on both simvastatin and gemfibrozil. The patient's most recent LDL was at goal. Knowing that coadministration of simvastatin and gemfibrozil can increase the risk of rhabdomyolysis, how appropriate is it for the hospitalist to stop one of these medications without discussing it with the primary care physician? |
Changing statin (related to the reason for admission) | A 65‐year‐old patient with a past medical history of hyperlipidemia on maximum‐dose simvastatin is admitted with a nonST‐elevation MI. The patient's cholesterol is noted to be above goal. Knowing that improving lipid management reduces mortality in cardiovascular disease, how appropriate is it for the hospitalist to replace simvastatin with atorvastatin without discussing it with the primary care physician? |
Changing statin (unrelated to the reason for admission) | A 65‐year‐old patient with a past medical history of a prior nonST‐elevation MI that was medically managed and hyperlipidemia on maximum‐dose simvastatin is admitted with pneumonia. Incidentally, the hospitalist notes that the patient's cholesterol has been above goal for the last 2 years. Knowing that improving lipid management reduces mortality in cardiovascular disease, how appropriate is it for the hospitalist to replace simvastatin with atorvastatin without discussing it with the primary care physician? |
The third section aimed to directly assess physicians' opinions. It consisted of questions regarding the appropriateness of inpatient management of conditions related to and unrelated to a patient's reason for admission.
Prior to administration, we conducted focus groups of hospitalists and PCPs to help hypothesize current physician perceptions on inpatient management, assess physician understanding of survey cases and questions, and to evaluate survey length.
Survey Administration
Between October 23, 2012 and November 10, 2012, 3 emails containing a link to the online survey were sent to all hospitalist and hospital‐based PCPs at the 3 study institutions. The BIDMC Committee on Clinical Investigations, to whom authority was ceded by the remaining 2 study institutions, certified this research protocol as exempt.
Statistical Analysis
We hypothesized that respondents as a whole would be more likely to rate an intervention as appropriate or very appropriate if it was related to the reason for admission, compared to unrelated, and that there would be no difference between PCPs and hospitalists.
We used 2 and Fisher exact tests (where applicable) to compare categorical variables, and a nonparametric median test for continuous variables. We used the Fisher exact test to compare the percent of respondents rating each intervention as appropriate or very appropriate by relatedness or unrelatedness to the reason for admission, and by PCP vs hospitalist. To derive the relative risk (RR) of rating each intervention as appropriate or very appropriate by PCPs compared to hospitalists, adjusting for potential confounders including years out of residency and sex, we used multivariable generalized estimating equation models, each with a Poisson distribution error term, a log link, and an exchangeable working correlation structure to account for dependency of observations arising from clustering at either the hospital or participant level, depending on the comparison: for comparisons within a given case, we controlled for clustering at the hospital level; for comparisons of cases in aggregate, owing to multiple responses from each participant, we controlled for clustering at the individual level.
Assuming a 50% response rate from both PCPs and hospitalists, and that 50% of PCPs would rate a given intervention as appropriate, we calculated that we would have 90% power to detect a 50% increase in the proportion of hospitalists rating an intervention as appropriate as compared to PCPs, using an of .05.
RESULTS
Demographics
One hundred sixty‐two out of 295 providers (55%) responded to the survey (Table 2). The response rate did not differ between hospitalists (70 out of 128; 55%) and PCPs (92 out of 167; 55%). Female respondents made up 58.7% of the PCP and 50.0% of the hospitalist groups (P=0.34). On average, PCPs were older (P<0.001) with a greater median number of years since graduation from residency (P<0.001). A greater percentage of hospitalists spent more than three‐quarters of their time clinically (42.9% vs 19.6%, P=0.009).
Total, n=162 (100.0%) | PCP, n=92 (6.8%) | Hospitalist, n=70 (43.2%) | P Valuea | |
---|---|---|---|---|
| ||||
Hospital, n (%) | ||||
BIDMC | 79 (48.8) | 48 (60.8) | 31 (39.2) | 0.115 |
BWH | 36 (22.2) | 15 (41.7) | 21 (58.3) | |
MGH | 47 (29.0) | 29 (61.7) | 18 (38.3) | |
Sex, n (%) | ||||
Male | 73 (45.1) | 38 (41.3) | 35 (50.0) | 0.339 |
Female | 89 (54.9) | 54 (58.7) | 35 (50.0) | |
Age interval, y, n (%) | ||||
2534 | 36 (22.2) | 9 (9.8) | 27 (38.6) | <0.001 |
3544 | 67 (41.4) | 34 (37.0) | 33 (47.1) | |
4554 | 35 (21.6) | 29 (31.5) | 6 (8.6) | |
5564 | 19 (11.7) | 16 (17.4) | 3 (4.3) | |
6574 | 5 (3.1) | 4 (4.4) | 1 (1.4) | |
Years out of residency, median (IQR) | 10 (417) | 15 (74) | 5 (211) | <0.001 |
Clinical FTE, n (%) | ||||
0.25 | 30 (18.6) | 22 (23.9) | 8 (11.4) | 0.009 |
0.260.50 | 41 (25.3) | 25 (27.2) | 16 (22.9) | |
0.510.75 | 43 (26.5) | 27 (29.4) | 16 (22.9) | |
>0.75 | 48 (29.6) | 18 (19.6) | 30 (42.9) | |
Worked as PCP?b | ||||
Yes | 6 (8.6) | |||
No | 64 (91.4) | |||
Worked as hospitalist? | ||||
Yes | 11 (12.0) | |||
No | 81 (88.0) | |||
AOR for admitted patients | ||||
Always | 16 (17.4) | |||
Mostly | 8 (8.7) | |||
Rarely | 7 (7.6) | |||
Never | 60 (65.2) |
Appropriateness of Inpatient Management Based on Admitting Diagnosis
For each of the 6 case pairings individually and in aggregate, respondents were significantly more likely to deem the intervention appropriate or very appropriate if it was related to the reason for admission, compared to those interventions unrelated to the reason for admission (in aggregate, 78.9% vs 38.8% respectively, P<0.001). For example, whereas 96.9% felt that the addition of aspirin in a patient admitted with acute myocardial infarction (MI) was appropriate, only 54.3% felt it appropriate to start aspirin in a patient with a prior history of MI admitted with cellulitis (P<0.001). Significant differences (all P values <0.001) were seen for all case pairs: starting spironolactone (68.1% when related to the reason for reason for admission vs 43.1% when unrelated to reason for admission); starting warfarin (62.3% vs 23.3%), stopping proton pump inhibitor (72.3% vs 42.8%), stopping statin or fibrate (90.6% vs 28.3%), and changing statin (83.0% vs 40.5%).
Appropriateness of Inpatient Management based on Primary Role
Table 3 compares the percent of PCPs and hospitalists rating each intervention as appropriate or very appropriate, by relatedness of the intervention to the reason for admission. In both unadjusted and adjusted comparisons for all cases in aggregate, PCPs were significantly more likely than hospitalists to rate the inpatient interventions as appropriate or very appropriate when the intervention was related to the reason for admission (83.4% of PCP responses vs 73.0% of hospitalist responses, P<0.001; RR: 1.2, 95% confidence interval [CI]: 1.11.3), unrelated to the reason for admission (44.7% vs 31.1%, P<0.001; RR: 1.5, 95% CI: 1.11.9), and overall (64.1% vs 52.1%, P<0.001; RR: 1.3, 95% CI: 1.11.4).
Relationship to Admission Diagnosis | PCP, n (%) | Hospitalist, n (%) | P Value | Adjusted RR | 95% CI |
---|---|---|---|---|---|
| |||||
Related | 453 (83.4) | 303 (73.0) | <0.001 | 1.2a | 1.11.3 |
Unrelated | 242 (44.7) | 129 (31.1) | <0.001 | 1.5a | 1.11.9 |
Overall | 695 (64.1) | 432 (52.1) | <0.001 | 1.3b | 1.11.4 |
Reasons for Inappropriate Designation
Among those respondents rating an intervention as inappropriate or very inappropriate, the 3 most common reasons selected as explanation for perceived inappropriateness from our predefined answer choices were: This medication will necessitate follow‐up testing/monitoring, for which the PCP will be responsible (chosen by physicians in 49.4% of instances); I am not confident that the hospitalist will have access to all of the medical history necessary to make this decision (35.7%); and Even if the hospitalist has all of the medical history and reviews it, the PCP should be involved in all decisions surrounding new medications (34.6%). The least common explanation chosen was I do not believe this is an appropriate pharmacologic intervention for this particular medical problem (6.5%). See Table 4 for a complete list of explanations, overall and stratified by PCP/hospitalist.
Predefined Reason for Inappropriateness | Total, n=583 (%) | PCP, n=318 (%) | Hospitalist, n=265 (%) | P Value |
---|---|---|---|---|
| ||||
This medication will necessitate follow‐up testing/monitoring, for which the PCP will be responsible. | 288 (49.4) | 151 (47.5) | 137 (51.7) | 0.32 |
I am not confident that the hospitalist will have access to all of the medical history necessary to make this decision. | 208 (35.7) | 98 (30.8) | 110 (41.5) | 0.009 |
Even if the hospitalist has all of the medical history and reviews it, the PCP should be involved in all decisions surrounding new medications. | 201 (34.5) | 125 (39.3) | 76 (28.7) | 0.009 |
I am not confident that the hospitalist will adequately review the medical history necessary to make this decision. | 184 (31.6) | 130 (40.9) | 54 (20.4) | <0.001 |
Even if the hospitalist has all of the medical history, I do not believe hospitalization is the right time to start this new medication | 106 (21.4) | 69 (21.7) | 56 (21.1) | 0.92 |
I am not confident that the hospitalist will appropriately discuss the risks and benefits of this new medication with the patient. | 106 (18.2) | 85 (26.7) | 21 (7.9) | <0.001 |
The benefit of this medication will be too remote to justify starting it in the acute setting. | 66 (11.3) | 40 (12.6) | 26 (9.8) | 0.36 |
I do not believe this is an appropriate pharmacologic intervention for this particular medical problem. | 38 (6.5) | 27 (8.5) | 11 (4.2) | 0.04 |
There were significant differences in the proportion of PCPs and hospitalists choosing several of the prespecified reasons for inappropriateness. Although hospitalists were more likely than PCPs to select I am not confident that the hospitalist will have access to all of the medical history necessary to make this decision (chosen by 41.5% of hospitalists vs 30.8% of PCPs, P=0.009), PCPs were more likely than hospitalists to select, I am not confident that the hospitalist will adequately review the medical history necessary to make this decision (chosen by 40.9% of PCPs vs 20.4% of hospitalists, P<0.001) and I am not confident that the hospitalist will appropriately discuss the risks and benefits of this new medication with the patient (26.7% of PCPs vs 9.8% of hospitalists, P<0.001).
Opinions on Current Management of Conditions Related and Unrelated to Admission
A minority of PCPs and hospitalists agreed or strongly agreed that hospitalists should play a larger role in the management of medical conditions unrelated to the reason for admission (28.1% of PCPs vs 34.8% of hospitalists; P=0.39).
DISCUSSION
In this survey‐based study of PCPs and hospitalists across 3 Boston‐area academic medical centers, we found that: (1) physicians were more likely to see inpatient interventions as appropriate when those interventions dealt with the reason for admission as compared to interventions unrelated to the reason for admission; and (2) PCPs were more likely than hospitalists to feel that inpatient interventions were appropriate, even when they targeted chronic conditions unrelated to the reason for admission. To our knowledge, this study represents the first investigation into the attitudes of PCPs and hospitalists regarding the inpatient management of conditions unrelated to the reason for admission.
That surveyed physicians, regardless of role, were less likely to report an intervention unrelated to the reason for hospitalization as appropriateeven those with likely mortality benefitsuggests that opportunities to affect meaningful change may be missed in a healthcare system that adheres to strict inpatient and outpatient roles. For several of the cases, a change in therapy could lead to benefit soon after implementation. For example, aldosterone antagonists reduce mortality as early as 1 month after initiation in select patients.[8] If a major goal of inpatient care is to reduce 30‐day mortality, it could be argued that hospitalists should more actively adjust congestive heart failure therapy in appropriate inpatients, even when this is not their admitting diagnosis.
For some conditions, CMS is already tracking hospital performance. Since 2003, hospitals have been required to document whether a patient with congestive heart failure (either acute or chronic and regardless of the relationship to admission) was prescribed an angiotensin‐converting enzyme (ACE) inhibitor or angiotensin receptor blocker (ARB) at the time of discharge.[7] CMS has determined that the proven benefits of ACE inhibitors and ARBs confer hospital accountability for their inclusion in appropriate patients, independent of the acuity of heart failure. There are many potential therapeutic maneuvers on which health systems (and their physicians) may be graded, and accepting the view that a hospitalization provides a window of opportunity for medical optimization may allow for more fruitful interventions and more patient‐centered care.
Despite the potential benefits of addressing chronic medical issues during hospitalization, there are important limitations on what can and/or should be done in the hospital setting. Hospitalizations are a time of fluctuating clinical status, which continues beyond discharge and is often accompanied by several medication changes.[9] In our study, more than 20% of those who believed that a medication intervention was inappropriate selected I do not believe hospitalization is the right time to start this new medication as one of their explanations. Although some medication interventions have been shown in randomized controlled trials to reduce short‐term mortality, the ability to generalize these findings to the average hospitalized patient with multiple comorbidities, concurrent medication changes, and rapidly fluctuating clinical status is limited. Furthermore, there are interventions most would agree should not be dealt with in the hospital (eg, screening colonoscopy) and encounters that may be too short to allow for change (eg, 24‐hour observation). These issues notwithstanding, the average 4‐day hospitalization likely provides an opportunity for monitored change that may currently be underutilized.
Our study suggests several additional explanations for physicians' current practice and opinions. Only 6.5% of respondents who answered that an intervention was inappropriate indicated as a justification that I do not believe this is an appropriate pharmacologic intervention for this particular medical problem. This suggests that the hesitancy has little to do with a lack of benefit but instead relates to systems issues (eg, access to all pertinent records and concerns regarding follow‐up testing) and perceived limitations to what a hospitalist should and should not do without actively involving the PCP. There are likely additional concerns that the medical record and/or patient histories do not fully outline the rationale for exclusion or inclusion of particular medications. Advances in information technology that enhance information exchange and enable streamlined communication may help to address these perceived barriers. However, an additional barrier may be trust, as PCPs appear more concerned that hospitalists will not review all the pertinent records or discuss risks and benefits before enacting important medication changes. Increased attempts at communication between hospitalists and outpatient providers may help to build trust and alleviate concerns regarding the loss of information that often occurs both on admission and at discharge.
We also noted that PCPs were more likely than hospitalists to feel that inpatient interventions were appropriate, even when targeting chronic conditions unrelated to the reason for admission. It may be that PCPs, with an increasing number of problems to address per outpatient visit,[10, 11] are more open to hospitalists managing any medical problems during their patients' admissions. At the same time, with increased acuity[12, 13, 14] and shortened length of stays,[15, 16] hospitalists have only a finite amount of time to ensure acute issues are managed, leaving potentially modifiable chronic conditions to the outpatient setting. These differences aside, a minority of both PCPs and hospitalists in our study were ready to embrace the idea of hospitalists playing a larger role in the management of conditions unrelated to the reason for hospitalization.
Even though our study benefits from its multisite design, there are a number of limitations. First, although we crafted our survey with input from general medicine focus groups, our survey instrument has not been validated. In addition, the cases are necessarily contrived and do not take into account the complexities of inpatient medicine. Furthermore, though our goal was to create paired cases that isolate a management decision as being simply based on whether it was related or unrelated to the reason for admission, it is possible that other factors, not captured by our survey, influenced the responses. For example, the benefits of aspirin as part of secondary prevention are not equal to the benefits in an acute MI.[17]
In an attempt to isolate the hospitalists' role in these management decisions, respondents were instructed to assume that the decisions were being made without discussing it with the primary care physician, but that the hospitalist would communicate the details of any hospitalization at the time of discharge. They were also instructed to assume that the hospitalist has access to the patient's outpatient electronic medical record. These assumptions were made to address concerns regarding the flow of information and communication, and to simulate the ideal system from a communication and information accessibility standpoint. Had these assumptions not been placed, the responses may have differed. It is likely that PCPs and hospitalists practicing in systems without shared, accessible inpatient/outpatient medical records would be even more reluctant to enact medication changes unrelated to the reason for admission.
Along the same lines, our physician cohort consisted of several metropolitan academic physician groups, in which hospitalists have had a presence for almost 20 years. As a result, our findings may not be generalizable to other academic hospitals, community‐based hospitalist programs, or nonhospital‐based PCP practices. Finally, we do not know whether survey nonresponders differed from responders in ways that could have meaningfully affected our results.
In conclusion, our findings suggest that both PCPs and hospitalists see the management of conditions unrelated to the reason for admission as less appropriate than the management of conditions related to the reason for admission. Our findings also suggest that PCPs may be more open to this practice when compared to hospitalists. Failure to capitalize on opportunities for meaningful medical interventions, independent of patient location, suggests a possible lack of patient centeredness in the current partnership between PCPs and hospitalists. Further studies should examine existing barriers and investigate interventions designed to address those barriers, in an effort to improve both quality of care and the degree of patient‐centeredness in our current healthcare system.
Disclosures: Dr. Herzig is supported by a grant from the National Institute on Aging (K23 AG042459). Dr. Herzig had full access to all of the data in the study and takes responsibility for the integrity of the data and the accuracy of the data analysis. Author contributions: study concept and design, Breu, Allen‐Dicker, Mueller, Herzig; acquisition of data, Breu, Allen‐Dicker, Mueller, Palamara, Herzig; analysis and interpretation of data, Breu, Allen‐Dicker, Hinami, Herzig; drafting of the manuscript, Breu; critical revision of the manuscript for important intellectual content, Breu, Allen‐Dicker, Mueller, Palamara, Hinami, Herzig; statistical analysis, Allen‐Dicker, Hinami, Herzig; study supervision, Breu, Herzig. This study was presented as a poster at the Society of Hospital Medicine National Meeting, Washington, DC, May 17, 2013.
- Growth in the care of older patients by hospitalists in the United States. N Engl J Med. 2009;360(11):1102–1112. , , , .
- The emerging role of “hospitalists” in the American health care system. N Engl J Med. 1996;335(7):514–517. , .
- An introduction to the hospitalist model. Ann Intern Med. 1999;130(4 pt 2):338–342. .
- Hospital readmission as an accountability measure. JAMA. 2011;305(5):504–505. , .
- A national strategy to put accountable care into practice. Health Aff (Millwood). 2010;29(5):982–990. , , , , .
- Keeping score under a global payment system. N Engl J Med. 2012;366(5):393–395. .
- Reporting Hospital Quality Data for Annual Payment Update. Available at: http://www.cms.gov/Medicare/Quality‐Initiatives‐Patient‐Assessment‐Instruments/HospitalQualityInits/Downloads/HospitalRHQDAPU200808. Accessed December 18, 2013.
- Eplerenone in patients with systolic heart failure and mild symptoms. N Engl J Med. 2011;364(1):11–21. , , , et al.
- How are drug regimen changes during hospitalisation handled after discharge: a cohort study. BMJ Open. 2012;2(6):e001461. , , , , , .
- Primary care visit duration and quality: does good care take longer? Arch Intern Med. 2009;169(20):1866–1872. , , .
- The increasing number of clinical items addressed during the time of adult primary care visits. J Gen Intern Med. 2008;23(12):2058–2065. , , ,
- Multiple chronic conditions among adults aged 45 and over: trends over the past 10 years. NCHS Data Brief. 2012;(100):1–8. , , .
- Prevalence of multiple chronic conditions in the United States' Medicare population. Health Qual Life Outcomes. 2009;7(1):82. , , .
- Multiple chronic conditions: prevalence, health consequences, and implications for quality, care management, and costs. J Gen Intern Med. 2007;22(suppl 3):391–395. , , , et al.
- Associations between reduced hospital length of stay and 30‐day readmission rate and mortality: 14‐year experience in 129 Veterans Affairs hospitals. Ann Intern Med. 2012;157(12):837–845. , , , et al.
- Trends in length of stay and short‐term outcomes among Medicare patients hospitalized for heart failure, 1993–2006. JAMA. 2010;303(21):2141–2147. , , , et al.
- Antithrombotic Trialists' Collaboration. Collaborative meta‐analysis of randomised trials of antiplatelet therapy for prevention of death, myocardial infarction, and stroke in high risk patients. BMJ. 2002;324(7329):71–86.
- Growth in the care of older patients by hospitalists in the United States. N Engl J Med. 2009;360(11):1102–1112. , , , .
- The emerging role of “hospitalists” in the American health care system. N Engl J Med. 1996;335(7):514–517. , .
- An introduction to the hospitalist model. Ann Intern Med. 1999;130(4 pt 2):338–342. .
- Hospital readmission as an accountability measure. JAMA. 2011;305(5):504–505. , .
- A national strategy to put accountable care into practice. Health Aff (Millwood). 2010;29(5):982–990. , , , , .
- Keeping score under a global payment system. N Engl J Med. 2012;366(5):393–395. .
- Reporting Hospital Quality Data for Annual Payment Update. Available at: http://www.cms.gov/Medicare/Quality‐Initiatives‐Patient‐Assessment‐Instruments/HospitalQualityInits/Downloads/HospitalRHQDAPU200808. Accessed December 18, 2013.
- Eplerenone in patients with systolic heart failure and mild symptoms. N Engl J Med. 2011;364(1):11–21. , , , et al.
- How are drug regimen changes during hospitalisation handled after discharge: a cohort study. BMJ Open. 2012;2(6):e001461. , , , , , .
- Primary care visit duration and quality: does good care take longer? Arch Intern Med. 2009;169(20):1866–1872. , , .
- The increasing number of clinical items addressed during the time of adult primary care visits. J Gen Intern Med. 2008;23(12):2058–2065. , , ,
- Multiple chronic conditions among adults aged 45 and over: trends over the past 10 years. NCHS Data Brief. 2012;(100):1–8. , , .
- Prevalence of multiple chronic conditions in the United States' Medicare population. Health Qual Life Outcomes. 2009;7(1):82. , , .
- Multiple chronic conditions: prevalence, health consequences, and implications for quality, care management, and costs. J Gen Intern Med. 2007;22(suppl 3):391–395. , , , et al.
- Associations between reduced hospital length of stay and 30‐day readmission rate and mortality: 14‐year experience in 129 Veterans Affairs hospitals. Ann Intern Med. 2012;157(12):837–845. , , , et al.
- Trends in length of stay and short‐term outcomes among Medicare patients hospitalized for heart failure, 1993–2006. JAMA. 2010;303(21):2141–2147. , , , et al.
- Antithrombotic Trialists' Collaboration. Collaborative meta‐analysis of randomised trials of antiplatelet therapy for prevention of death, myocardial infarction, and stroke in high risk patients. BMJ. 2002;324(7329):71–86.
© 2014 Society of Hospital Medicine
Hospitalist‐Job Fit
Person‐organization fit concerns the conditions and consequences of compatibility between people and the organizations for which they work.[1] Studies of other industries have demonstrated that person‐organization fit informs the way individuals join, perform in, and are retained by organizations.[2] Person‐job fit is a closely related subordinate concept that concerns the alignment of workers and their job in as much as workers have needs that their job supplies, or conversely, jobs have requirements that certain workers' abilities can help meet.[3] Explorations of job fit in physicians and their work have recently emerged in a few investigations published in medical journals.[4, 5, 6, 7, 8] Further expanding the understanding of fit between physicians and their employment is important, because the decline of solo practices and recent emphasis on team‐based care have led to a growing number of US physicians working in organizations.[9]
The movement of physicians into employed situations may continue if certain types of Accountable Care Organizations take root.[10] And physicians may be primed to join employer organizations based on current career priorities of individuals in American society. Surveys of medical residents entering the workforce reveal more physicians preferring the security of being employees than starting their own practices.[11] Given these trends, job fit will inform our understanding of how personal and job characteristics facilitate recruitment, performance, satisfaction, and longevity of physician employees.
BACKGROUND
Virtually all hospitalists work in organizationshospitalsand are employees of hospitals, medical schools, physician group practices, or management companies, and therefore invariably function within organizational structures and systems.[7] In spite of their rapid growth in numbers, many employers have faced difficulties recruiting and retaining enough hospitalists to fill their staffing needs. Consequently, the US hospitalist workforce today is characterized by high salaries, work load, and attrition rates.[12]
In this evolving unsaturated market, the attraction‐selection‐attrition framework[13] provides a theoretical construct that predicts that hospitalists and their employers would seek congruence of goals and values early in their relationship through a process of trial and error. This framework assumes that early interactions between workers and organizations serve as opportunities for them to understand if job fit is poor and dissociate or remain affiliated as long as job fit is mutually acceptable. Therefore, job switching on average is expected to increase job fit because workers and organizations gain a better understanding of their own goals and values and choose more wisely the next time.
Other theoretical frameworks, such as the job characteristic model,[14] suggest that over time as workers stay at the same job, they continue to maintain and improve job fit through various workplace‐ or self‐modification strategies. For example, seniority status may have privileges (eg, less undesirable call), or workers may create privileged niches through the acquisition of new skills and abilities over time. Hospitalists' tendency to diversify their work‐related activities by incorporating administrative and teaching responsibilities[15] may thus contribute to improving job fit. Additionally, as a measure of complementarity among people who work together, job fit may be influenced by the quality of relationships among hospitalists and their coworkers through their reorientation to the prevailing organizational climate[16, 17] and increasing socialization.[18] Finally, given that experiential learning is known to contribute to better hospitalist work performance,[19] job fit may affect productivity and clinical outcomes vis‐‐vis quality of work life.
To test the validity of these assumptions in a sample of hospitalists, we critically appraised the following 4 hypotheses:
- Hypothesis 1 (H1): Job attrition and reselection improves job fit among hospitalists entering the job market.
- Hypothesis 2 (H2): Better job fit is achieved through hospitalists engaging a variety of personal skills and abilities.
- Hypothesis 3 (H3): Job fit increases with hospitalists' job duration together with socialization and internalization of organizational values.
- Hypothesis 4 (H4): Job fit is correlated with hospitalists' quality of work life.
METHODS
Analysis was performed on data from the 2009 to 2010 Hospital Medicine Physician Worklife Survey. The sample frame included nonmembers and members of Society of Hospital Medicine (SHM). Details about sampling strategy, data collection, and data quality are available in previous publications.[7, 20] The 118‐item survey instrument, including 9 demographic items and 24 practice and job characteristic items, was administered by mail. Examples of information solicited through these items included respondents' practice model, the number of hospitalist jobs they have held, and the specific kinds of clinical and nonclinical activities they performed as part of their current job.
We used a reliable but broad and generic measure of self‐perceived person‐job fit.[21] The survey items of the 5‐point Likert‐type scale anchored between strongly disagree and strongly agree were: I feel that my work utilizes my full abilities, I feel competent and fully able to handle my job, my job gives me the chance to do the things I feel I do best, I feel that my job and I are well‐matched, I feel I have adequate preparation for the job I now hold. The quality of hospitalists' relationships with physician colleagues, staff, and patients as well as job satisfaction was measured using scales adapted from the Physician Worklife Study.[22] Organizational climate was measured using an adapted scale from the Minimizing Error, Maximizing Outcome study incorporating 3 items from the cohesiveness subscale, 4 items from the organizational trust subscale, and 1 item from the quality emphasis subscale that were most pertinent to hospitalists' relationship with their organizations.[23] Intent to leave practice or reduce work hours was measured using 5 items from the Multi‐Center Hospitalist Survey Project.[24] Frequency of participation in suboptimal patient care was measured by adapting 3 items from the suboptimal reported practice subscale and 2 items from the suboptimal patient care subscale developed by Shanafelt et al.[25] Stress and job burnout were assessed using validated measures.[26, 27] Detailed descriptions of the response rate calculation and imputation of missing item data are available in previous publications.[7, 20]
Mean, variance, range, and skew were used to characterize the responses to the job fit survey scale. A table of respondent characteristics was constructed. A visual representation of job fit by individual hospitalist year in current practice was created, first, by plotting a locally weighted scatterplot smoothing curve to examine the shape of the general relationship, and second, by fitting a similarly contoured functional polynomial curve with 95% confidence intervals (CI) to a plot of the mean and interquartile range of job fit for each year in current practice. Spearman partial correlations were calculated for job fit and each of the 5 items addressing likelihood of leaving practice or reducing workload adjusted for gender to control for the higher proportion of women who plan to work part time. Median (interquartile range) job fit was calculated for categories defined by the number of job changes and compared with the reference category (no job change) using the nonparametric rank sum test for comparing non‐normally distributed data. Multivariate logistic regression models were used to calculate the odds ratio (OR) of participating in each of several clinical and nonclinical hospitalist activities between respondents whose job fit score was optimal (5 on a 5‐point scale) and less than optimal controlling for covariates that influence the likelihood of participating in these activities (years in current practice, practice model, and specialty training). A Spearman correlation matrix was created to assess interscale correlations among organizational parameters (years in current practice, job fit, organizational climate, and relationship with colleagues, staff, and patients). Finally, a separate Spearman correlation matrix was created to assess the interscale correlations among individual worker parameters (job fit, suboptimal patient care, job burnout, stress, and job satisfaction). Statistical significance was defined as P value <0.05, and all analyses were performed on Stata 11.0 (StataCorp, College Station, TX). The Northwestern University institutional review board approved this study.
RESULTS
Respondents included 816 hospitalists belonging to around 700 unique organizations. The adjusted response rate from the stratified sample was 26%. Respondents and nonrespondents were similar with regard to geographic region and model of practice, but respondents were more likely to be members of the SHM than nonrespondents. Panel A of Table 1 shows the demographic characteristics of the respondents. The mean age was 44.3 years, and about one‐third were women. The average hospitalist had about 7 years of experience in the specialty and about 5 years with their current hospitalist job. The majority were trained in internal medicine or one of its subspecialties, whereas pediatricians, family physicians, and physicians with other training made up the remainder.
Panel A | Panel B | ||
---|---|---|---|
Total | Assimilation Period Hospitalists | Advancement Period Hospitalists | |
| |||
Total, n | 816 | 103 | 713 |
Female, n (%) | 284 (35) | 37 (36) | 247 (35) |
Age, mean (SD) | 44.3 (9.0) | 41.9 (9.3) | 44.7 (8.9) |
Years postresidency experience as hospitalist, mean (SD) | 6.9 (4.5) | 4.3 (3.1) | 7.2 (4.6) |
Years in current practice, mean (SD) | 5.1 (3.9) | 0.9 (0.3) | 6.7 (3.8) |
Specialty training, n (%) | |||
Internal medicine | 555 (68) | 75 (73) | 480 (67) |
Pediatrics | 117 (14) | 8 (8) | 109 (15.3) |
Family medicine | 49 (6) | 7 (7) | 42 (6) |
Other | 95 (11) | 13 (13) | 82 (12) |
Job fit was highly skewed toward optimum fit, with a mean of 4.3 on a scale of 1 to 5, with a narrow standard deviation of 0.7. The poorest job fit was reported by 0.3%, whereas optimal fit was reported by 21% of respondents. Job fit plotted against years in current practice had a logarithmic appearance typical of learning curves (Figure 1). An inflection point was visualized at around 2 years. For the purposes of this article, we refer to hospitalists' experience in the first 2 years of a job as an assimilation period, which is marked by a steep increase in job fit early when rapid learning or attrition took place. The years beyond the inflection point are characterized as an advancement period, when a more attenuated rise in job fit was experienced with time. The Spearman correlation between job fit and years in practice during the advancement period was 0.145 (n = 678, P < 0.001). Panel B of Table 1 displays the characteristics of respondents separately for the assimilation and advancement cohorts. Assimilation hospitalists in our sample had a mean age of 41.9 years and mean on‐the‐job experience of 4.3 years, reflecting that many hospitalists in the first 2 years of a job have made at least 1 job change in the past.
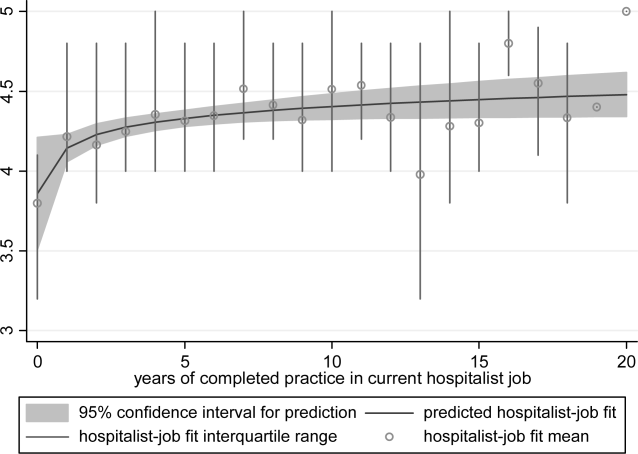
To show the effects of attrition and reselection, we first evaluated the proposition that hospitalists experience attrition (ie, intend to leave their jobs) in response to poor fit. Table 2 shows the correlations between job fit and the self‐reported intent to leave practice or reduce workload separately for the assimilation and advancement periods. For hospitalists in the assimilation period, job fit was negatively correlated with intent to leave current practice within 2 years and to leave hospital medicine within 5 years (P = 0.010 and 0.043, respectively). Hospitalists with <2 years in their current job, therefore, tended to consider attrition but not workload reduction to deal with poor job fit. On the other hand, hospitalists in the advancement period considered both attrition and workload reduction strategies in response to poor fit (all P < 0.001).
Assimilation Period Hospitalists | Advancement Period Hospitalists | |||
---|---|---|---|---|
Rho | P Value | Rho | P Value | |
Likelihood that a hospitalist will: | ||||
Leave current practice within 2 years | 0.253 | 0.010 | 0.367 | <0.001 |
Decrease total work hours within 5 years | 0.060 | 0.548 | 0.179 | <0.001 |
Decrease clinical work hours within 5 years | 0.072 | 0.469 | 0.144 | <0.001 |
Leave hospital medicine within 5 years | 0.200 | 0.043 | 0.231 | <0.001 |
Leave direct patient care within 5 years | 0.040 | 0.691 | 0.212 | <0.001 |
In Table 3, we further compared the median job fit across categories for the number of job switches. The median job fit during the assimilation period of hospitalists who had made 1 job change was slightly but statistically higher than the job fit of their counterparts who never left their first job (4.4 vs 4.0, P = 0.046). This suggests that job switching by hospitalists early in their jobs is associated with improved job fit (H1). However, the fit during the assimilation period of hospitalists who switched jobs twice or more was statistically no different from the fit of those in their first jobs, suggesting that the effect of the attrition‐reselection strategy is weak or inconsistent. The job fit for advancement period hospitalists was also different across the job change and no‐change categories. However, in the case of hospitalists later in their jobs, the median job fit was slightly but statistically lower among those who made job changes, revealing the potential drop in job fit that occurs when a hospitalist already established in his or her job starts over again in a new setting.
n | Age, Mean (95% CI), y | Hospitalist‐Job Fit, Median (IQR) | P Valuea | |
---|---|---|---|---|
| ||||
Assimilation period hospitalistsb | ||||
No job change | 29 | 42.3 (37.347.3) | 4.0 (3.84.4) | Reference |
1 job change | 39 | 40.3 (38.142.5) | 4.4 (4.04.8) | 0.046 |
2 or more job changes | 27 | 43.8 (41.046.6) | 4.4 (3.84.8) | 0.153 |
Advancement period hospitalistsc | ||||
No job change | 390 | 44.5 (43.645.5) | 4.6 (4.05.0) | Reference |
1 job change | 183 | 45.0 (43.746.3) | 4.2 (4.04.8) | 0.002 |
2 or more job changes | 99 | 44.9 (43.146.6) | 4.2 (3.84.8) | 0.002 |
We hypothesized that hospitalists who achieved high job fit within a particular job were more likely to have engaged in activities that utilize a wider spectrum of their abilities. As shown in Table 4, hospitalists in the highest quartile of job fit were associated with a general trend toward higher odds of participating in a variety of common clinical and nonclinical hospitalist activities, but only the odds ratio associated with teaching achieved statistical significance (OR: 1.53, 95% CI: 1.01‐2.31) (H2).
Participation, n/N (%) | Odds Ratio (95% CI) | P Value | |
---|---|---|---|
| |||
Administrative or committee work | 704/816 (86) | 0.73 (0.431.26) | 0.262 |
Quality improvement or patient safety initiatives | 678/816 (83) | 1.13 (0.642.00) | 0.680 |
Information technology design or implementation | 379/816 (46) | 1.18 (0.801.73) | 0.408 |
Any of the above leadership activities | 758/816 (93) | 1.31 (0.563.05) | 0.535 |
Teaching | 442/816 (54) | 1.53 (1.012.31) | 0.046 |
Research | 120/816 (15) | 1.07 (0.601.92) | 0.816 |
Any of the above academic activities | 457/816 (56) | 1.50 (0.992.27) | 0.057 |
Code team or rapid response team | 437/816 (54) | 1.13 (0.771.68) | 0.533 |
Intensive care unit | 254/816 (31) | 0.84 (0.531.35) | 0.469 |
Skilled nursing facility or long‐term acute care facility | 126/816 (15) | 1.06 (0.621.81) | 0.835 |
Outpatient general medical practice | 44/816 (5) | 1.75 (0.813.80) | 0.157 |
Any of the above clinical activities | 681/816 (79) | 1.02 (0.601.76) | 0.930 |
Socialization with peers and the gradual sharing of values within organizations are hypothesized mechanisms for increasing job fit with time. We found that the number of years in current practice was positively correlated with job fit (Spearman coefficient R = 0.149, P < 0.001), organizational climate (R = 0.128, P < 0.001), and relationship with nonphysician staff (R = 0.102, P < 0.01). The association between years in practice and relationship with physician colleagues were weaker (R = 0.079, P < 0.05). Consistent with the episodic nature of patients' encounters with hospitalists, the measure of patient relationships was not significantly associated with length of time in job. In addition, we found substantial correlations among job fit, organizational climate, and all the relational measures (all R > 0.280, P < 0.001), indicating that hospitalists increasingly share the values of their organizations over time (H3).
Finally, we also hypothesized that poor job fit is associated with poor performance and quality of work life. Strong correlations with job fit were noted for stress (R = 0.307, P < 0.001), job burnout (R = 0.360, P < 0.001), and job satisfaction (R = 0.570, P < 0.001). Job fit (R = 0.147, P < 0.001), job burnout (R = 0.236, P < 0.001), stress (R = 0.305, P < 0.001), and job satisfaction (R = 0.224, P < 0.001) were all significantly correlated with the frequency of participating in suboptimal care (H4).
DISCUSSION
In this exploratory analysis, we validated in the hospitalist workforce several assumptions about person‐job fit that have been observed in workers of other industries. We observed attrition‐reselection (ie, job switching) as a strategy used by physicians to achieve better fit early in their job tenure, whereas job modification appeared to be more effective than attrition‐reselection among physicians already established in their jobs. We provided weak but plausible evidence that physicians with optimal job fit had a tendency to participate in activities (eg, teaching) that engage a wider set of interests and abilities. We also demonstrated the growth in hospitalists sharing the values of their organization through the time‐dependent associations among organizational climate, relational measures, and job fit. Finally, we found that physicians with suboptimal job fit were more likely to report poor performance in their work compared to those indicating optimal fit.
Our previous analysis of data from the Hospital Medicine Physician Worklife Survey exposed the widely variable work characteristics of hospitalist jobs in the US market and the equally variable preferences and priorities of individual hospitalists in selecting their work setting.[7] The implication of our present study is that hospitalists achieve the high levels of observed job fit using various strategies that aid their alignment with their employment. One of these strategies involves time, but physician longevity in practice may be both a determinant and product of good job fit. Although early job attrition may be necessary for fitting the right hospitalists to the right jobs, employers may appreciate the importance of retaining experienced hospitalists not only for cost and performance considerations but also for the growth of social capital in organizations consisting of enduring individuals. As our data suggest that hospitalists grow with their jobs, physicians may experience better fit with jobs that flexibly couple their work demands with benefits that address their individual work‐life needs over time. Another implication of this study is that job fit is a useful and predictive measure of job selection, performance, and retention. In light of studies that expose the limitations of job satisfaction as a measure influenced more by workers' dispositional affect (ie, their temperament and outlook) than their compatibility with their jobs,[28] job fit may add a functional dimension to traditional employee feedback measures.
There are limitations to this analysis. The most notable is the low survey response rate. Two reasons contributed to the fairly low rate of return. First, the original sampling frame included many outdated addresses and names of individuals who did not meet inclusion criteria. Although all sampled individuals who would have been excluded from the study could not be identified, we calculated our response rate without accounting for the proportion of potential ineligibles in the denominator population [Response Rate 2 (RR2) according to standards of the American Association of Public Opinion Research].[29] Second, the response rates of physician surveys have seen a steady decline over the years.[30] Respondents to our survey may be older and more experienced than US hospitalists in general. Although concerns about bias from under‐reporting cannot be fully addressed, we believe that the study sample is adequate for this preliminary study intended to translate the evidence of observed phenomena from the nonphysician to the physician workforces. The suboptimal response characteristics (high skew and low variability) of the generic person‐job fit survey scale used in this study indicate that a reliable survey instrument specifically designed to measure physician‐job fit need to be constructed de novo and validated for any future study. Although we performed simple analyses to support our assertions, few of our subanalyses may be underpowered, contributing to overinterpretation of the data. Additional empirical work is also necessary to assess the generalizability of this study's claims in other medical and surgical specialties. Such studies would also allow measurement of the sensitivity and specificity of physicians' self‐identification of poor job fit. Finally, additional investigations of this time‐dependent construct are more appropriately performed using a longitudinal study design to overcome the limitations inherent in this cross‐sectional analysis. Our conclusions about the time‐dependent features of job fit may be explained by other characteristics such as generational and cultural differences among hospitalists with varying experience.
As the US healthcare system reorganizes to bolster accountability,[31] we anticipate increasing interdependence between physicians and their employer organizations. Ultimately, the desired accountability in healthcare is likely to be obtained if physicians function not only as passive and interchangeable employees but as active stakeholders in the achievement of each organization's goals. A methodology for assessing the alignment of physicians and their jobs will continue to be important along the way.
Disclosure
Nothing to report.
- Person‐organization fit: an integrative review of its conceptualizations, measurement, and implications. Personnel Psychol. 1996;49:1–49. .
- Consequences of individuals' fit at work: a meta‐analysis of person‐job, person‐organization, person‐group, and person‐supervisor fit. Personnel Psychol. 2005;58(2):281–342. , , .
- Person‐job fit: a conceptual integration, literature review and methodological critique. In: Cooper CL, Robertson IT, eds. International Review of Industrial and Organizational Psychology. Vol.6. New York, NY:John Wiley 1991. .
- Organizational culture, person‐culture fit, and turnover: a replication in the health care industry. J Organ Behav. 1999;20(2):175–184. .
- Organizational culture and physician satisfaction with dimensions of group practice. Health Serv Res. 2007;42(3 pt 1):1150–1176. , , , .
- Career fit and burnout among academic faculty. Arch Intern Med. 2009;169(10):990–995. , , , et al.
- Job characteristics, satisfaction, and burnout across hospitalist practice models. J Hosp Med. 2012;7(5):402–410. , , , , .
- Provider‐hospital “fit” and patient outcomes: evidence from Massachusetts cardiac surgeons, 2002–2004. Health Serv Res. 2011;46(1 pt 1):1–26. .
- The evolving primary care physician. N Engl J Med. 2012;366(20):1849–1853. .
- Hospitals' race to employ physicians—the logic behind a money‐losing proposition. N Engl J Med. 2011;364(19):1790–1793. , .
- 2011 Survey of Final‐Year Medical Residents. Irving, TX:Merritt Hawkins;2011.
- State of Hospital Medicine: 2010 Report Based on 2009 Data. Englewood, CO:Society of Hospital Medicine and the Medical Group Management Association;2010.
- The ASA framework: an update. Personnel Psychol. 1995;48(4):747–773. , , .
- Work Redesign. Reading. MA:Addison‐Wesley;1980. , .
- The expanding role of hospitalists in the United States. Swiss Med Wkly. 2006;136(37–38):591–596. , .
- Organizational socialization as a learning‐process—the role of information acquisition. Personnel Psychol. 1992;45(4):849–874. , .
- The moderating effect of tenure in person‐environment fit: a field study in educational organizations. J Occup Organ Psych. 1997;70:173–188. , .
- Matching people and organizations—selection and socialization in public accounting firms. Admin Sci Quart. 1991;36(3):459–484. .
- Effects of physician experience on costs and outcomes on an academic general medicine service: results of a trial of hospitalists. Ann Intern Med. 2002;137(11):866–874. , , , et al.
- Worklife and satisfaction of hospitalists: toward flourishing careers. J Gen Intern Med. 2012;27(1):28–36. , , , , .
- Karasek's model in the People's Republic of China: effects of job demands, control, and individual differences. Acad Manage J. 1996;39(6):1594–1618. .
- Measuring physician job satisfaction in a changing workplace and a challenging environment. SGIM Career Satisfaction Study Group. Society of General Internal Medicine. Med Care. 1999;37(11):1174–1182. , , , et al.
- Organizational climate, stress, and error in primary care: The MEMO Study. Adv Patient Saf. 2005;1:65–77. , , , et al.
- Effects of inpatient experience on outcomes and costs in a multicenter trial of academic hospitalists. J Gen Intern Med. 2005;20(suppl 1):141–142. , , , et al.
- Burnout and self‐reported patient care in an internal medicine residency program. Ann Intern Med. 2002;136(5):358–367. , , , .
- Effect of job demands and social support on worker stress—a study of VDT users. Behav Inform Technol. 1995;14(1):32–40. , .
- Validation of a single‐item measure of burnout against the Maslach Burnout Inventory among physicians. Stress Health. 2004;20(2):75–79. , , .
- Job satisfaction: a meta‐analysis of stabilities. J Organ Behav. 2001;22(5):483–504. , .
- The American Association for Public Opinion Research. Standard definitions: final dispositions of case codes and outcome rates for surveys.7th ed. Available at: http://www.aapor.org/Standard_Definitions2.htm. Accessed May 2,2012.
- Response rates and response bias for 50 surveys of pediatricians. Health Serv Res. 2005;40(1):213–226. , , , .
- Accountable care organizations: accountable for what, to whom, and how. JAMA. 2010;304(15):1715–6. , .
Person‐organization fit concerns the conditions and consequences of compatibility between people and the organizations for which they work.[1] Studies of other industries have demonstrated that person‐organization fit informs the way individuals join, perform in, and are retained by organizations.[2] Person‐job fit is a closely related subordinate concept that concerns the alignment of workers and their job in as much as workers have needs that their job supplies, or conversely, jobs have requirements that certain workers' abilities can help meet.[3] Explorations of job fit in physicians and their work have recently emerged in a few investigations published in medical journals.[4, 5, 6, 7, 8] Further expanding the understanding of fit between physicians and their employment is important, because the decline of solo practices and recent emphasis on team‐based care have led to a growing number of US physicians working in organizations.[9]
The movement of physicians into employed situations may continue if certain types of Accountable Care Organizations take root.[10] And physicians may be primed to join employer organizations based on current career priorities of individuals in American society. Surveys of medical residents entering the workforce reveal more physicians preferring the security of being employees than starting their own practices.[11] Given these trends, job fit will inform our understanding of how personal and job characteristics facilitate recruitment, performance, satisfaction, and longevity of physician employees.
BACKGROUND
Virtually all hospitalists work in organizationshospitalsand are employees of hospitals, medical schools, physician group practices, or management companies, and therefore invariably function within organizational structures and systems.[7] In spite of their rapid growth in numbers, many employers have faced difficulties recruiting and retaining enough hospitalists to fill their staffing needs. Consequently, the US hospitalist workforce today is characterized by high salaries, work load, and attrition rates.[12]
In this evolving unsaturated market, the attraction‐selection‐attrition framework[13] provides a theoretical construct that predicts that hospitalists and their employers would seek congruence of goals and values early in their relationship through a process of trial and error. This framework assumes that early interactions between workers and organizations serve as opportunities for them to understand if job fit is poor and dissociate or remain affiliated as long as job fit is mutually acceptable. Therefore, job switching on average is expected to increase job fit because workers and organizations gain a better understanding of their own goals and values and choose more wisely the next time.
Other theoretical frameworks, such as the job characteristic model,[14] suggest that over time as workers stay at the same job, they continue to maintain and improve job fit through various workplace‐ or self‐modification strategies. For example, seniority status may have privileges (eg, less undesirable call), or workers may create privileged niches through the acquisition of new skills and abilities over time. Hospitalists' tendency to diversify their work‐related activities by incorporating administrative and teaching responsibilities[15] may thus contribute to improving job fit. Additionally, as a measure of complementarity among people who work together, job fit may be influenced by the quality of relationships among hospitalists and their coworkers through their reorientation to the prevailing organizational climate[16, 17] and increasing socialization.[18] Finally, given that experiential learning is known to contribute to better hospitalist work performance,[19] job fit may affect productivity and clinical outcomes vis‐‐vis quality of work life.
To test the validity of these assumptions in a sample of hospitalists, we critically appraised the following 4 hypotheses:
- Hypothesis 1 (H1): Job attrition and reselection improves job fit among hospitalists entering the job market.
- Hypothesis 2 (H2): Better job fit is achieved through hospitalists engaging a variety of personal skills and abilities.
- Hypothesis 3 (H3): Job fit increases with hospitalists' job duration together with socialization and internalization of organizational values.
- Hypothesis 4 (H4): Job fit is correlated with hospitalists' quality of work life.
METHODS
Analysis was performed on data from the 2009 to 2010 Hospital Medicine Physician Worklife Survey. The sample frame included nonmembers and members of Society of Hospital Medicine (SHM). Details about sampling strategy, data collection, and data quality are available in previous publications.[7, 20] The 118‐item survey instrument, including 9 demographic items and 24 practice and job characteristic items, was administered by mail. Examples of information solicited through these items included respondents' practice model, the number of hospitalist jobs they have held, and the specific kinds of clinical and nonclinical activities they performed as part of their current job.
We used a reliable but broad and generic measure of self‐perceived person‐job fit.[21] The survey items of the 5‐point Likert‐type scale anchored between strongly disagree and strongly agree were: I feel that my work utilizes my full abilities, I feel competent and fully able to handle my job, my job gives me the chance to do the things I feel I do best, I feel that my job and I are well‐matched, I feel I have adequate preparation for the job I now hold. The quality of hospitalists' relationships with physician colleagues, staff, and patients as well as job satisfaction was measured using scales adapted from the Physician Worklife Study.[22] Organizational climate was measured using an adapted scale from the Minimizing Error, Maximizing Outcome study incorporating 3 items from the cohesiveness subscale, 4 items from the organizational trust subscale, and 1 item from the quality emphasis subscale that were most pertinent to hospitalists' relationship with their organizations.[23] Intent to leave practice or reduce work hours was measured using 5 items from the Multi‐Center Hospitalist Survey Project.[24] Frequency of participation in suboptimal patient care was measured by adapting 3 items from the suboptimal reported practice subscale and 2 items from the suboptimal patient care subscale developed by Shanafelt et al.[25] Stress and job burnout were assessed using validated measures.[26, 27] Detailed descriptions of the response rate calculation and imputation of missing item data are available in previous publications.[7, 20]
Mean, variance, range, and skew were used to characterize the responses to the job fit survey scale. A table of respondent characteristics was constructed. A visual representation of job fit by individual hospitalist year in current practice was created, first, by plotting a locally weighted scatterplot smoothing curve to examine the shape of the general relationship, and second, by fitting a similarly contoured functional polynomial curve with 95% confidence intervals (CI) to a plot of the mean and interquartile range of job fit for each year in current practice. Spearman partial correlations were calculated for job fit and each of the 5 items addressing likelihood of leaving practice or reducing workload adjusted for gender to control for the higher proportion of women who plan to work part time. Median (interquartile range) job fit was calculated for categories defined by the number of job changes and compared with the reference category (no job change) using the nonparametric rank sum test for comparing non‐normally distributed data. Multivariate logistic regression models were used to calculate the odds ratio (OR) of participating in each of several clinical and nonclinical hospitalist activities between respondents whose job fit score was optimal (5 on a 5‐point scale) and less than optimal controlling for covariates that influence the likelihood of participating in these activities (years in current practice, practice model, and specialty training). A Spearman correlation matrix was created to assess interscale correlations among organizational parameters (years in current practice, job fit, organizational climate, and relationship with colleagues, staff, and patients). Finally, a separate Spearman correlation matrix was created to assess the interscale correlations among individual worker parameters (job fit, suboptimal patient care, job burnout, stress, and job satisfaction). Statistical significance was defined as P value <0.05, and all analyses were performed on Stata 11.0 (StataCorp, College Station, TX). The Northwestern University institutional review board approved this study.
RESULTS
Respondents included 816 hospitalists belonging to around 700 unique organizations. The adjusted response rate from the stratified sample was 26%. Respondents and nonrespondents were similar with regard to geographic region and model of practice, but respondents were more likely to be members of the SHM than nonrespondents. Panel A of Table 1 shows the demographic characteristics of the respondents. The mean age was 44.3 years, and about one‐third were women. The average hospitalist had about 7 years of experience in the specialty and about 5 years with their current hospitalist job. The majority were trained in internal medicine or one of its subspecialties, whereas pediatricians, family physicians, and physicians with other training made up the remainder.
Panel A | Panel B | ||
---|---|---|---|
Total | Assimilation Period Hospitalists | Advancement Period Hospitalists | |
| |||
Total, n | 816 | 103 | 713 |
Female, n (%) | 284 (35) | 37 (36) | 247 (35) |
Age, mean (SD) | 44.3 (9.0) | 41.9 (9.3) | 44.7 (8.9) |
Years postresidency experience as hospitalist, mean (SD) | 6.9 (4.5) | 4.3 (3.1) | 7.2 (4.6) |
Years in current practice, mean (SD) | 5.1 (3.9) | 0.9 (0.3) | 6.7 (3.8) |
Specialty training, n (%) | |||
Internal medicine | 555 (68) | 75 (73) | 480 (67) |
Pediatrics | 117 (14) | 8 (8) | 109 (15.3) |
Family medicine | 49 (6) | 7 (7) | 42 (6) |
Other | 95 (11) | 13 (13) | 82 (12) |
Job fit was highly skewed toward optimum fit, with a mean of 4.3 on a scale of 1 to 5, with a narrow standard deviation of 0.7. The poorest job fit was reported by 0.3%, whereas optimal fit was reported by 21% of respondents. Job fit plotted against years in current practice had a logarithmic appearance typical of learning curves (Figure 1). An inflection point was visualized at around 2 years. For the purposes of this article, we refer to hospitalists' experience in the first 2 years of a job as an assimilation period, which is marked by a steep increase in job fit early when rapid learning or attrition took place. The years beyond the inflection point are characterized as an advancement period, when a more attenuated rise in job fit was experienced with time. The Spearman correlation between job fit and years in practice during the advancement period was 0.145 (n = 678, P < 0.001). Panel B of Table 1 displays the characteristics of respondents separately for the assimilation and advancement cohorts. Assimilation hospitalists in our sample had a mean age of 41.9 years and mean on‐the‐job experience of 4.3 years, reflecting that many hospitalists in the first 2 years of a job have made at least 1 job change in the past.
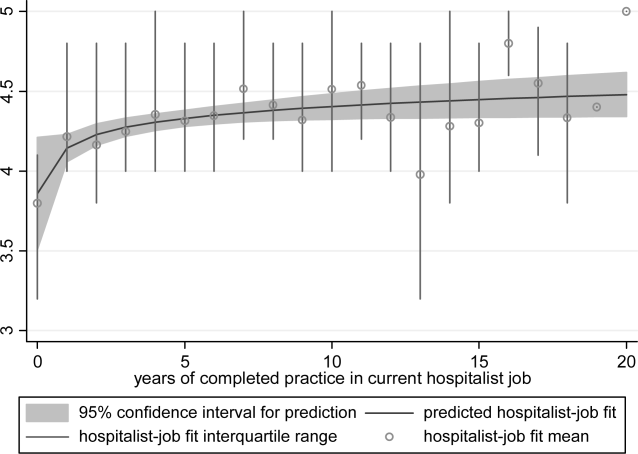
To show the effects of attrition and reselection, we first evaluated the proposition that hospitalists experience attrition (ie, intend to leave their jobs) in response to poor fit. Table 2 shows the correlations between job fit and the self‐reported intent to leave practice or reduce workload separately for the assimilation and advancement periods. For hospitalists in the assimilation period, job fit was negatively correlated with intent to leave current practice within 2 years and to leave hospital medicine within 5 years (P = 0.010 and 0.043, respectively). Hospitalists with <2 years in their current job, therefore, tended to consider attrition but not workload reduction to deal with poor job fit. On the other hand, hospitalists in the advancement period considered both attrition and workload reduction strategies in response to poor fit (all P < 0.001).
Assimilation Period Hospitalists | Advancement Period Hospitalists | |||
---|---|---|---|---|
Rho | P Value | Rho | P Value | |
Likelihood that a hospitalist will: | ||||
Leave current practice within 2 years | 0.253 | 0.010 | 0.367 | <0.001 |
Decrease total work hours within 5 years | 0.060 | 0.548 | 0.179 | <0.001 |
Decrease clinical work hours within 5 years | 0.072 | 0.469 | 0.144 | <0.001 |
Leave hospital medicine within 5 years | 0.200 | 0.043 | 0.231 | <0.001 |
Leave direct patient care within 5 years | 0.040 | 0.691 | 0.212 | <0.001 |
In Table 3, we further compared the median job fit across categories for the number of job switches. The median job fit during the assimilation period of hospitalists who had made 1 job change was slightly but statistically higher than the job fit of their counterparts who never left their first job (4.4 vs 4.0, P = 0.046). This suggests that job switching by hospitalists early in their jobs is associated with improved job fit (H1). However, the fit during the assimilation period of hospitalists who switched jobs twice or more was statistically no different from the fit of those in their first jobs, suggesting that the effect of the attrition‐reselection strategy is weak or inconsistent. The job fit for advancement period hospitalists was also different across the job change and no‐change categories. However, in the case of hospitalists later in their jobs, the median job fit was slightly but statistically lower among those who made job changes, revealing the potential drop in job fit that occurs when a hospitalist already established in his or her job starts over again in a new setting.
n | Age, Mean (95% CI), y | Hospitalist‐Job Fit, Median (IQR) | P Valuea | |
---|---|---|---|---|
| ||||
Assimilation period hospitalistsb | ||||
No job change | 29 | 42.3 (37.347.3) | 4.0 (3.84.4) | Reference |
1 job change | 39 | 40.3 (38.142.5) | 4.4 (4.04.8) | 0.046 |
2 or more job changes | 27 | 43.8 (41.046.6) | 4.4 (3.84.8) | 0.153 |
Advancement period hospitalistsc | ||||
No job change | 390 | 44.5 (43.645.5) | 4.6 (4.05.0) | Reference |
1 job change | 183 | 45.0 (43.746.3) | 4.2 (4.04.8) | 0.002 |
2 or more job changes | 99 | 44.9 (43.146.6) | 4.2 (3.84.8) | 0.002 |
We hypothesized that hospitalists who achieved high job fit within a particular job were more likely to have engaged in activities that utilize a wider spectrum of their abilities. As shown in Table 4, hospitalists in the highest quartile of job fit were associated with a general trend toward higher odds of participating in a variety of common clinical and nonclinical hospitalist activities, but only the odds ratio associated with teaching achieved statistical significance (OR: 1.53, 95% CI: 1.01‐2.31) (H2).
Participation, n/N (%) | Odds Ratio (95% CI) | P Value | |
---|---|---|---|
| |||
Administrative or committee work | 704/816 (86) | 0.73 (0.431.26) | 0.262 |
Quality improvement or patient safety initiatives | 678/816 (83) | 1.13 (0.642.00) | 0.680 |
Information technology design or implementation | 379/816 (46) | 1.18 (0.801.73) | 0.408 |
Any of the above leadership activities | 758/816 (93) | 1.31 (0.563.05) | 0.535 |
Teaching | 442/816 (54) | 1.53 (1.012.31) | 0.046 |
Research | 120/816 (15) | 1.07 (0.601.92) | 0.816 |
Any of the above academic activities | 457/816 (56) | 1.50 (0.992.27) | 0.057 |
Code team or rapid response team | 437/816 (54) | 1.13 (0.771.68) | 0.533 |
Intensive care unit | 254/816 (31) | 0.84 (0.531.35) | 0.469 |
Skilled nursing facility or long‐term acute care facility | 126/816 (15) | 1.06 (0.621.81) | 0.835 |
Outpatient general medical practice | 44/816 (5) | 1.75 (0.813.80) | 0.157 |
Any of the above clinical activities | 681/816 (79) | 1.02 (0.601.76) | 0.930 |
Socialization with peers and the gradual sharing of values within organizations are hypothesized mechanisms for increasing job fit with time. We found that the number of years in current practice was positively correlated with job fit (Spearman coefficient R = 0.149, P < 0.001), organizational climate (R = 0.128, P < 0.001), and relationship with nonphysician staff (R = 0.102, P < 0.01). The association between years in practice and relationship with physician colleagues were weaker (R = 0.079, P < 0.05). Consistent with the episodic nature of patients' encounters with hospitalists, the measure of patient relationships was not significantly associated with length of time in job. In addition, we found substantial correlations among job fit, organizational climate, and all the relational measures (all R > 0.280, P < 0.001), indicating that hospitalists increasingly share the values of their organizations over time (H3).
Finally, we also hypothesized that poor job fit is associated with poor performance and quality of work life. Strong correlations with job fit were noted for stress (R = 0.307, P < 0.001), job burnout (R = 0.360, P < 0.001), and job satisfaction (R = 0.570, P < 0.001). Job fit (R = 0.147, P < 0.001), job burnout (R = 0.236, P < 0.001), stress (R = 0.305, P < 0.001), and job satisfaction (R = 0.224, P < 0.001) were all significantly correlated with the frequency of participating in suboptimal care (H4).
DISCUSSION
In this exploratory analysis, we validated in the hospitalist workforce several assumptions about person‐job fit that have been observed in workers of other industries. We observed attrition‐reselection (ie, job switching) as a strategy used by physicians to achieve better fit early in their job tenure, whereas job modification appeared to be more effective than attrition‐reselection among physicians already established in their jobs. We provided weak but plausible evidence that physicians with optimal job fit had a tendency to participate in activities (eg, teaching) that engage a wider set of interests and abilities. We also demonstrated the growth in hospitalists sharing the values of their organization through the time‐dependent associations among organizational climate, relational measures, and job fit. Finally, we found that physicians with suboptimal job fit were more likely to report poor performance in their work compared to those indicating optimal fit.
Our previous analysis of data from the Hospital Medicine Physician Worklife Survey exposed the widely variable work characteristics of hospitalist jobs in the US market and the equally variable preferences and priorities of individual hospitalists in selecting their work setting.[7] The implication of our present study is that hospitalists achieve the high levels of observed job fit using various strategies that aid their alignment with their employment. One of these strategies involves time, but physician longevity in practice may be both a determinant and product of good job fit. Although early job attrition may be necessary for fitting the right hospitalists to the right jobs, employers may appreciate the importance of retaining experienced hospitalists not only for cost and performance considerations but also for the growth of social capital in organizations consisting of enduring individuals. As our data suggest that hospitalists grow with their jobs, physicians may experience better fit with jobs that flexibly couple their work demands with benefits that address their individual work‐life needs over time. Another implication of this study is that job fit is a useful and predictive measure of job selection, performance, and retention. In light of studies that expose the limitations of job satisfaction as a measure influenced more by workers' dispositional affect (ie, their temperament and outlook) than their compatibility with their jobs,[28] job fit may add a functional dimension to traditional employee feedback measures.
There are limitations to this analysis. The most notable is the low survey response rate. Two reasons contributed to the fairly low rate of return. First, the original sampling frame included many outdated addresses and names of individuals who did not meet inclusion criteria. Although all sampled individuals who would have been excluded from the study could not be identified, we calculated our response rate without accounting for the proportion of potential ineligibles in the denominator population [Response Rate 2 (RR2) according to standards of the American Association of Public Opinion Research].[29] Second, the response rates of physician surveys have seen a steady decline over the years.[30] Respondents to our survey may be older and more experienced than US hospitalists in general. Although concerns about bias from under‐reporting cannot be fully addressed, we believe that the study sample is adequate for this preliminary study intended to translate the evidence of observed phenomena from the nonphysician to the physician workforces. The suboptimal response characteristics (high skew and low variability) of the generic person‐job fit survey scale used in this study indicate that a reliable survey instrument specifically designed to measure physician‐job fit need to be constructed de novo and validated for any future study. Although we performed simple analyses to support our assertions, few of our subanalyses may be underpowered, contributing to overinterpretation of the data. Additional empirical work is also necessary to assess the generalizability of this study's claims in other medical and surgical specialties. Such studies would also allow measurement of the sensitivity and specificity of physicians' self‐identification of poor job fit. Finally, additional investigations of this time‐dependent construct are more appropriately performed using a longitudinal study design to overcome the limitations inherent in this cross‐sectional analysis. Our conclusions about the time‐dependent features of job fit may be explained by other characteristics such as generational and cultural differences among hospitalists with varying experience.
As the US healthcare system reorganizes to bolster accountability,[31] we anticipate increasing interdependence between physicians and their employer organizations. Ultimately, the desired accountability in healthcare is likely to be obtained if physicians function not only as passive and interchangeable employees but as active stakeholders in the achievement of each organization's goals. A methodology for assessing the alignment of physicians and their jobs will continue to be important along the way.
Disclosure
Nothing to report.
Person‐organization fit concerns the conditions and consequences of compatibility between people and the organizations for which they work.[1] Studies of other industries have demonstrated that person‐organization fit informs the way individuals join, perform in, and are retained by organizations.[2] Person‐job fit is a closely related subordinate concept that concerns the alignment of workers and their job in as much as workers have needs that their job supplies, or conversely, jobs have requirements that certain workers' abilities can help meet.[3] Explorations of job fit in physicians and their work have recently emerged in a few investigations published in medical journals.[4, 5, 6, 7, 8] Further expanding the understanding of fit between physicians and their employment is important, because the decline of solo practices and recent emphasis on team‐based care have led to a growing number of US physicians working in organizations.[9]
The movement of physicians into employed situations may continue if certain types of Accountable Care Organizations take root.[10] And physicians may be primed to join employer organizations based on current career priorities of individuals in American society. Surveys of medical residents entering the workforce reveal more physicians preferring the security of being employees than starting their own practices.[11] Given these trends, job fit will inform our understanding of how personal and job characteristics facilitate recruitment, performance, satisfaction, and longevity of physician employees.
BACKGROUND
Virtually all hospitalists work in organizationshospitalsand are employees of hospitals, medical schools, physician group practices, or management companies, and therefore invariably function within organizational structures and systems.[7] In spite of their rapid growth in numbers, many employers have faced difficulties recruiting and retaining enough hospitalists to fill their staffing needs. Consequently, the US hospitalist workforce today is characterized by high salaries, work load, and attrition rates.[12]
In this evolving unsaturated market, the attraction‐selection‐attrition framework[13] provides a theoretical construct that predicts that hospitalists and their employers would seek congruence of goals and values early in their relationship through a process of trial and error. This framework assumes that early interactions between workers and organizations serve as opportunities for them to understand if job fit is poor and dissociate or remain affiliated as long as job fit is mutually acceptable. Therefore, job switching on average is expected to increase job fit because workers and organizations gain a better understanding of their own goals and values and choose more wisely the next time.
Other theoretical frameworks, such as the job characteristic model,[14] suggest that over time as workers stay at the same job, they continue to maintain and improve job fit through various workplace‐ or self‐modification strategies. For example, seniority status may have privileges (eg, less undesirable call), or workers may create privileged niches through the acquisition of new skills and abilities over time. Hospitalists' tendency to diversify their work‐related activities by incorporating administrative and teaching responsibilities[15] may thus contribute to improving job fit. Additionally, as a measure of complementarity among people who work together, job fit may be influenced by the quality of relationships among hospitalists and their coworkers through their reorientation to the prevailing organizational climate[16, 17] and increasing socialization.[18] Finally, given that experiential learning is known to contribute to better hospitalist work performance,[19] job fit may affect productivity and clinical outcomes vis‐‐vis quality of work life.
To test the validity of these assumptions in a sample of hospitalists, we critically appraised the following 4 hypotheses:
- Hypothesis 1 (H1): Job attrition and reselection improves job fit among hospitalists entering the job market.
- Hypothesis 2 (H2): Better job fit is achieved through hospitalists engaging a variety of personal skills and abilities.
- Hypothesis 3 (H3): Job fit increases with hospitalists' job duration together with socialization and internalization of organizational values.
- Hypothesis 4 (H4): Job fit is correlated with hospitalists' quality of work life.
METHODS
Analysis was performed on data from the 2009 to 2010 Hospital Medicine Physician Worklife Survey. The sample frame included nonmembers and members of Society of Hospital Medicine (SHM). Details about sampling strategy, data collection, and data quality are available in previous publications.[7, 20] The 118‐item survey instrument, including 9 demographic items and 24 practice and job characteristic items, was administered by mail. Examples of information solicited through these items included respondents' practice model, the number of hospitalist jobs they have held, and the specific kinds of clinical and nonclinical activities they performed as part of their current job.
We used a reliable but broad and generic measure of self‐perceived person‐job fit.[21] The survey items of the 5‐point Likert‐type scale anchored between strongly disagree and strongly agree were: I feel that my work utilizes my full abilities, I feel competent and fully able to handle my job, my job gives me the chance to do the things I feel I do best, I feel that my job and I are well‐matched, I feel I have adequate preparation for the job I now hold. The quality of hospitalists' relationships with physician colleagues, staff, and patients as well as job satisfaction was measured using scales adapted from the Physician Worklife Study.[22] Organizational climate was measured using an adapted scale from the Minimizing Error, Maximizing Outcome study incorporating 3 items from the cohesiveness subscale, 4 items from the organizational trust subscale, and 1 item from the quality emphasis subscale that were most pertinent to hospitalists' relationship with their organizations.[23] Intent to leave practice or reduce work hours was measured using 5 items from the Multi‐Center Hospitalist Survey Project.[24] Frequency of participation in suboptimal patient care was measured by adapting 3 items from the suboptimal reported practice subscale and 2 items from the suboptimal patient care subscale developed by Shanafelt et al.[25] Stress and job burnout were assessed using validated measures.[26, 27] Detailed descriptions of the response rate calculation and imputation of missing item data are available in previous publications.[7, 20]
Mean, variance, range, and skew were used to characterize the responses to the job fit survey scale. A table of respondent characteristics was constructed. A visual representation of job fit by individual hospitalist year in current practice was created, first, by plotting a locally weighted scatterplot smoothing curve to examine the shape of the general relationship, and second, by fitting a similarly contoured functional polynomial curve with 95% confidence intervals (CI) to a plot of the mean and interquartile range of job fit for each year in current practice. Spearman partial correlations were calculated for job fit and each of the 5 items addressing likelihood of leaving practice or reducing workload adjusted for gender to control for the higher proportion of women who plan to work part time. Median (interquartile range) job fit was calculated for categories defined by the number of job changes and compared with the reference category (no job change) using the nonparametric rank sum test for comparing non‐normally distributed data. Multivariate logistic regression models were used to calculate the odds ratio (OR) of participating in each of several clinical and nonclinical hospitalist activities between respondents whose job fit score was optimal (5 on a 5‐point scale) and less than optimal controlling for covariates that influence the likelihood of participating in these activities (years in current practice, practice model, and specialty training). A Spearman correlation matrix was created to assess interscale correlations among organizational parameters (years in current practice, job fit, organizational climate, and relationship with colleagues, staff, and patients). Finally, a separate Spearman correlation matrix was created to assess the interscale correlations among individual worker parameters (job fit, suboptimal patient care, job burnout, stress, and job satisfaction). Statistical significance was defined as P value <0.05, and all analyses were performed on Stata 11.0 (StataCorp, College Station, TX). The Northwestern University institutional review board approved this study.
RESULTS
Respondents included 816 hospitalists belonging to around 700 unique organizations. The adjusted response rate from the stratified sample was 26%. Respondents and nonrespondents were similar with regard to geographic region and model of practice, but respondents were more likely to be members of the SHM than nonrespondents. Panel A of Table 1 shows the demographic characteristics of the respondents. The mean age was 44.3 years, and about one‐third were women. The average hospitalist had about 7 years of experience in the specialty and about 5 years with their current hospitalist job. The majority were trained in internal medicine or one of its subspecialties, whereas pediatricians, family physicians, and physicians with other training made up the remainder.
Panel A | Panel B | ||
---|---|---|---|
Total | Assimilation Period Hospitalists | Advancement Period Hospitalists | |
| |||
Total, n | 816 | 103 | 713 |
Female, n (%) | 284 (35) | 37 (36) | 247 (35) |
Age, mean (SD) | 44.3 (9.0) | 41.9 (9.3) | 44.7 (8.9) |
Years postresidency experience as hospitalist, mean (SD) | 6.9 (4.5) | 4.3 (3.1) | 7.2 (4.6) |
Years in current practice, mean (SD) | 5.1 (3.9) | 0.9 (0.3) | 6.7 (3.8) |
Specialty training, n (%) | |||
Internal medicine | 555 (68) | 75 (73) | 480 (67) |
Pediatrics | 117 (14) | 8 (8) | 109 (15.3) |
Family medicine | 49 (6) | 7 (7) | 42 (6) |
Other | 95 (11) | 13 (13) | 82 (12) |
Job fit was highly skewed toward optimum fit, with a mean of 4.3 on a scale of 1 to 5, with a narrow standard deviation of 0.7. The poorest job fit was reported by 0.3%, whereas optimal fit was reported by 21% of respondents. Job fit plotted against years in current practice had a logarithmic appearance typical of learning curves (Figure 1). An inflection point was visualized at around 2 years. For the purposes of this article, we refer to hospitalists' experience in the first 2 years of a job as an assimilation period, which is marked by a steep increase in job fit early when rapid learning or attrition took place. The years beyond the inflection point are characterized as an advancement period, when a more attenuated rise in job fit was experienced with time. The Spearman correlation between job fit and years in practice during the advancement period was 0.145 (n = 678, P < 0.001). Panel B of Table 1 displays the characteristics of respondents separately for the assimilation and advancement cohorts. Assimilation hospitalists in our sample had a mean age of 41.9 years and mean on‐the‐job experience of 4.3 years, reflecting that many hospitalists in the first 2 years of a job have made at least 1 job change in the past.
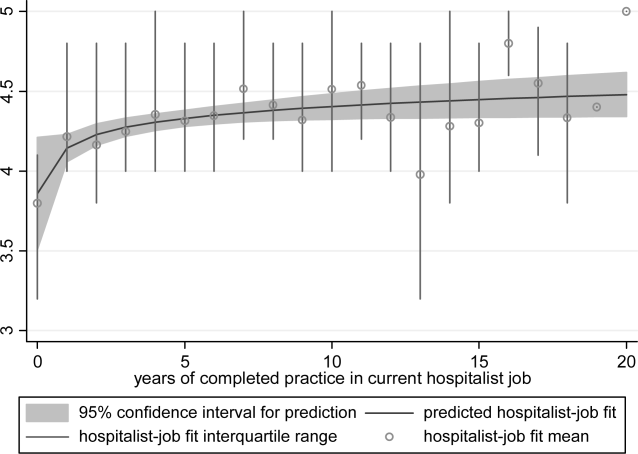
To show the effects of attrition and reselection, we first evaluated the proposition that hospitalists experience attrition (ie, intend to leave their jobs) in response to poor fit. Table 2 shows the correlations between job fit and the self‐reported intent to leave practice or reduce workload separately for the assimilation and advancement periods. For hospitalists in the assimilation period, job fit was negatively correlated with intent to leave current practice within 2 years and to leave hospital medicine within 5 years (P = 0.010 and 0.043, respectively). Hospitalists with <2 years in their current job, therefore, tended to consider attrition but not workload reduction to deal with poor job fit. On the other hand, hospitalists in the advancement period considered both attrition and workload reduction strategies in response to poor fit (all P < 0.001).
Assimilation Period Hospitalists | Advancement Period Hospitalists | |||
---|---|---|---|---|
Rho | P Value | Rho | P Value | |
Likelihood that a hospitalist will: | ||||
Leave current practice within 2 years | 0.253 | 0.010 | 0.367 | <0.001 |
Decrease total work hours within 5 years | 0.060 | 0.548 | 0.179 | <0.001 |
Decrease clinical work hours within 5 years | 0.072 | 0.469 | 0.144 | <0.001 |
Leave hospital medicine within 5 years | 0.200 | 0.043 | 0.231 | <0.001 |
Leave direct patient care within 5 years | 0.040 | 0.691 | 0.212 | <0.001 |
In Table 3, we further compared the median job fit across categories for the number of job switches. The median job fit during the assimilation period of hospitalists who had made 1 job change was slightly but statistically higher than the job fit of their counterparts who never left their first job (4.4 vs 4.0, P = 0.046). This suggests that job switching by hospitalists early in their jobs is associated with improved job fit (H1). However, the fit during the assimilation period of hospitalists who switched jobs twice or more was statistically no different from the fit of those in their first jobs, suggesting that the effect of the attrition‐reselection strategy is weak or inconsistent. The job fit for advancement period hospitalists was also different across the job change and no‐change categories. However, in the case of hospitalists later in their jobs, the median job fit was slightly but statistically lower among those who made job changes, revealing the potential drop in job fit that occurs when a hospitalist already established in his or her job starts over again in a new setting.
n | Age, Mean (95% CI), y | Hospitalist‐Job Fit, Median (IQR) | P Valuea | |
---|---|---|---|---|
| ||||
Assimilation period hospitalistsb | ||||
No job change | 29 | 42.3 (37.347.3) | 4.0 (3.84.4) | Reference |
1 job change | 39 | 40.3 (38.142.5) | 4.4 (4.04.8) | 0.046 |
2 or more job changes | 27 | 43.8 (41.046.6) | 4.4 (3.84.8) | 0.153 |
Advancement period hospitalistsc | ||||
No job change | 390 | 44.5 (43.645.5) | 4.6 (4.05.0) | Reference |
1 job change | 183 | 45.0 (43.746.3) | 4.2 (4.04.8) | 0.002 |
2 or more job changes | 99 | 44.9 (43.146.6) | 4.2 (3.84.8) | 0.002 |
We hypothesized that hospitalists who achieved high job fit within a particular job were more likely to have engaged in activities that utilize a wider spectrum of their abilities. As shown in Table 4, hospitalists in the highest quartile of job fit were associated with a general trend toward higher odds of participating in a variety of common clinical and nonclinical hospitalist activities, but only the odds ratio associated with teaching achieved statistical significance (OR: 1.53, 95% CI: 1.01‐2.31) (H2).
Participation, n/N (%) | Odds Ratio (95% CI) | P Value | |
---|---|---|---|
| |||
Administrative or committee work | 704/816 (86) | 0.73 (0.431.26) | 0.262 |
Quality improvement or patient safety initiatives | 678/816 (83) | 1.13 (0.642.00) | 0.680 |
Information technology design or implementation | 379/816 (46) | 1.18 (0.801.73) | 0.408 |
Any of the above leadership activities | 758/816 (93) | 1.31 (0.563.05) | 0.535 |
Teaching | 442/816 (54) | 1.53 (1.012.31) | 0.046 |
Research | 120/816 (15) | 1.07 (0.601.92) | 0.816 |
Any of the above academic activities | 457/816 (56) | 1.50 (0.992.27) | 0.057 |
Code team or rapid response team | 437/816 (54) | 1.13 (0.771.68) | 0.533 |
Intensive care unit | 254/816 (31) | 0.84 (0.531.35) | 0.469 |
Skilled nursing facility or long‐term acute care facility | 126/816 (15) | 1.06 (0.621.81) | 0.835 |
Outpatient general medical practice | 44/816 (5) | 1.75 (0.813.80) | 0.157 |
Any of the above clinical activities | 681/816 (79) | 1.02 (0.601.76) | 0.930 |
Socialization with peers and the gradual sharing of values within organizations are hypothesized mechanisms for increasing job fit with time. We found that the number of years in current practice was positively correlated with job fit (Spearman coefficient R = 0.149, P < 0.001), organizational climate (R = 0.128, P < 0.001), and relationship with nonphysician staff (R = 0.102, P < 0.01). The association between years in practice and relationship with physician colleagues were weaker (R = 0.079, P < 0.05). Consistent with the episodic nature of patients' encounters with hospitalists, the measure of patient relationships was not significantly associated with length of time in job. In addition, we found substantial correlations among job fit, organizational climate, and all the relational measures (all R > 0.280, P < 0.001), indicating that hospitalists increasingly share the values of their organizations over time (H3).
Finally, we also hypothesized that poor job fit is associated with poor performance and quality of work life. Strong correlations with job fit were noted for stress (R = 0.307, P < 0.001), job burnout (R = 0.360, P < 0.001), and job satisfaction (R = 0.570, P < 0.001). Job fit (R = 0.147, P < 0.001), job burnout (R = 0.236, P < 0.001), stress (R = 0.305, P < 0.001), and job satisfaction (R = 0.224, P < 0.001) were all significantly correlated with the frequency of participating in suboptimal care (H4).
DISCUSSION
In this exploratory analysis, we validated in the hospitalist workforce several assumptions about person‐job fit that have been observed in workers of other industries. We observed attrition‐reselection (ie, job switching) as a strategy used by physicians to achieve better fit early in their job tenure, whereas job modification appeared to be more effective than attrition‐reselection among physicians already established in their jobs. We provided weak but plausible evidence that physicians with optimal job fit had a tendency to participate in activities (eg, teaching) that engage a wider set of interests and abilities. We also demonstrated the growth in hospitalists sharing the values of their organization through the time‐dependent associations among organizational climate, relational measures, and job fit. Finally, we found that physicians with suboptimal job fit were more likely to report poor performance in their work compared to those indicating optimal fit.
Our previous analysis of data from the Hospital Medicine Physician Worklife Survey exposed the widely variable work characteristics of hospitalist jobs in the US market and the equally variable preferences and priorities of individual hospitalists in selecting their work setting.[7] The implication of our present study is that hospitalists achieve the high levels of observed job fit using various strategies that aid their alignment with their employment. One of these strategies involves time, but physician longevity in practice may be both a determinant and product of good job fit. Although early job attrition may be necessary for fitting the right hospitalists to the right jobs, employers may appreciate the importance of retaining experienced hospitalists not only for cost and performance considerations but also for the growth of social capital in organizations consisting of enduring individuals. As our data suggest that hospitalists grow with their jobs, physicians may experience better fit with jobs that flexibly couple their work demands with benefits that address their individual work‐life needs over time. Another implication of this study is that job fit is a useful and predictive measure of job selection, performance, and retention. In light of studies that expose the limitations of job satisfaction as a measure influenced more by workers' dispositional affect (ie, their temperament and outlook) than their compatibility with their jobs,[28] job fit may add a functional dimension to traditional employee feedback measures.
There are limitations to this analysis. The most notable is the low survey response rate. Two reasons contributed to the fairly low rate of return. First, the original sampling frame included many outdated addresses and names of individuals who did not meet inclusion criteria. Although all sampled individuals who would have been excluded from the study could not be identified, we calculated our response rate without accounting for the proportion of potential ineligibles in the denominator population [Response Rate 2 (RR2) according to standards of the American Association of Public Opinion Research].[29] Second, the response rates of physician surveys have seen a steady decline over the years.[30] Respondents to our survey may be older and more experienced than US hospitalists in general. Although concerns about bias from under‐reporting cannot be fully addressed, we believe that the study sample is adequate for this preliminary study intended to translate the evidence of observed phenomena from the nonphysician to the physician workforces. The suboptimal response characteristics (high skew and low variability) of the generic person‐job fit survey scale used in this study indicate that a reliable survey instrument specifically designed to measure physician‐job fit need to be constructed de novo and validated for any future study. Although we performed simple analyses to support our assertions, few of our subanalyses may be underpowered, contributing to overinterpretation of the data. Additional empirical work is also necessary to assess the generalizability of this study's claims in other medical and surgical specialties. Such studies would also allow measurement of the sensitivity and specificity of physicians' self‐identification of poor job fit. Finally, additional investigations of this time‐dependent construct are more appropriately performed using a longitudinal study design to overcome the limitations inherent in this cross‐sectional analysis. Our conclusions about the time‐dependent features of job fit may be explained by other characteristics such as generational and cultural differences among hospitalists with varying experience.
As the US healthcare system reorganizes to bolster accountability,[31] we anticipate increasing interdependence between physicians and their employer organizations. Ultimately, the desired accountability in healthcare is likely to be obtained if physicians function not only as passive and interchangeable employees but as active stakeholders in the achievement of each organization's goals. A methodology for assessing the alignment of physicians and their jobs will continue to be important along the way.
Disclosure
Nothing to report.
- Person‐organization fit: an integrative review of its conceptualizations, measurement, and implications. Personnel Psychol. 1996;49:1–49. .
- Consequences of individuals' fit at work: a meta‐analysis of person‐job, person‐organization, person‐group, and person‐supervisor fit. Personnel Psychol. 2005;58(2):281–342. , , .
- Person‐job fit: a conceptual integration, literature review and methodological critique. In: Cooper CL, Robertson IT, eds. International Review of Industrial and Organizational Psychology. Vol.6. New York, NY:John Wiley 1991. .
- Organizational culture, person‐culture fit, and turnover: a replication in the health care industry. J Organ Behav. 1999;20(2):175–184. .
- Organizational culture and physician satisfaction with dimensions of group practice. Health Serv Res. 2007;42(3 pt 1):1150–1176. , , , .
- Career fit and burnout among academic faculty. Arch Intern Med. 2009;169(10):990–995. , , , et al.
- Job characteristics, satisfaction, and burnout across hospitalist practice models. J Hosp Med. 2012;7(5):402–410. , , , , .
- Provider‐hospital “fit” and patient outcomes: evidence from Massachusetts cardiac surgeons, 2002–2004. Health Serv Res. 2011;46(1 pt 1):1–26. .
- The evolving primary care physician. N Engl J Med. 2012;366(20):1849–1853. .
- Hospitals' race to employ physicians—the logic behind a money‐losing proposition. N Engl J Med. 2011;364(19):1790–1793. , .
- 2011 Survey of Final‐Year Medical Residents. Irving, TX:Merritt Hawkins;2011.
- State of Hospital Medicine: 2010 Report Based on 2009 Data. Englewood, CO:Society of Hospital Medicine and the Medical Group Management Association;2010.
- The ASA framework: an update. Personnel Psychol. 1995;48(4):747–773. , , .
- Work Redesign. Reading. MA:Addison‐Wesley;1980. , .
- The expanding role of hospitalists in the United States. Swiss Med Wkly. 2006;136(37–38):591–596. , .
- Organizational socialization as a learning‐process—the role of information acquisition. Personnel Psychol. 1992;45(4):849–874. , .
- The moderating effect of tenure in person‐environment fit: a field study in educational organizations. J Occup Organ Psych. 1997;70:173–188. , .
- Matching people and organizations—selection and socialization in public accounting firms. Admin Sci Quart. 1991;36(3):459–484. .
- Effects of physician experience on costs and outcomes on an academic general medicine service: results of a trial of hospitalists. Ann Intern Med. 2002;137(11):866–874. , , , et al.
- Worklife and satisfaction of hospitalists: toward flourishing careers. J Gen Intern Med. 2012;27(1):28–36. , , , , .
- Karasek's model in the People's Republic of China: effects of job demands, control, and individual differences. Acad Manage J. 1996;39(6):1594–1618. .
- Measuring physician job satisfaction in a changing workplace and a challenging environment. SGIM Career Satisfaction Study Group. Society of General Internal Medicine. Med Care. 1999;37(11):1174–1182. , , , et al.
- Organizational climate, stress, and error in primary care: The MEMO Study. Adv Patient Saf. 2005;1:65–77. , , , et al.
- Effects of inpatient experience on outcomes and costs in a multicenter trial of academic hospitalists. J Gen Intern Med. 2005;20(suppl 1):141–142. , , , et al.
- Burnout and self‐reported patient care in an internal medicine residency program. Ann Intern Med. 2002;136(5):358–367. , , , .
- Effect of job demands and social support on worker stress—a study of VDT users. Behav Inform Technol. 1995;14(1):32–40. , .
- Validation of a single‐item measure of burnout against the Maslach Burnout Inventory among physicians. Stress Health. 2004;20(2):75–79. , , .
- Job satisfaction: a meta‐analysis of stabilities. J Organ Behav. 2001;22(5):483–504. , .
- The American Association for Public Opinion Research. Standard definitions: final dispositions of case codes and outcome rates for surveys.7th ed. Available at: http://www.aapor.org/Standard_Definitions2.htm. Accessed May 2,2012.
- Response rates and response bias for 50 surveys of pediatricians. Health Serv Res. 2005;40(1):213–226. , , , .
- Accountable care organizations: accountable for what, to whom, and how. JAMA. 2010;304(15):1715–6. , .
- Person‐organization fit: an integrative review of its conceptualizations, measurement, and implications. Personnel Psychol. 1996;49:1–49. .
- Consequences of individuals' fit at work: a meta‐analysis of person‐job, person‐organization, person‐group, and person‐supervisor fit. Personnel Psychol. 2005;58(2):281–342. , , .
- Person‐job fit: a conceptual integration, literature review and methodological critique. In: Cooper CL, Robertson IT, eds. International Review of Industrial and Organizational Psychology. Vol.6. New York, NY:John Wiley 1991. .
- Organizational culture, person‐culture fit, and turnover: a replication in the health care industry. J Organ Behav. 1999;20(2):175–184. .
- Organizational culture and physician satisfaction with dimensions of group practice. Health Serv Res. 2007;42(3 pt 1):1150–1176. , , , .
- Career fit and burnout among academic faculty. Arch Intern Med. 2009;169(10):990–995. , , , et al.
- Job characteristics, satisfaction, and burnout across hospitalist practice models. J Hosp Med. 2012;7(5):402–410. , , , , .
- Provider‐hospital “fit” and patient outcomes: evidence from Massachusetts cardiac surgeons, 2002–2004. Health Serv Res. 2011;46(1 pt 1):1–26. .
- The evolving primary care physician. N Engl J Med. 2012;366(20):1849–1853. .
- Hospitals' race to employ physicians—the logic behind a money‐losing proposition. N Engl J Med. 2011;364(19):1790–1793. , .
- 2011 Survey of Final‐Year Medical Residents. Irving, TX:Merritt Hawkins;2011.
- State of Hospital Medicine: 2010 Report Based on 2009 Data. Englewood, CO:Society of Hospital Medicine and the Medical Group Management Association;2010.
- The ASA framework: an update. Personnel Psychol. 1995;48(4):747–773. , , .
- Work Redesign. Reading. MA:Addison‐Wesley;1980. , .
- The expanding role of hospitalists in the United States. Swiss Med Wkly. 2006;136(37–38):591–596. , .
- Organizational socialization as a learning‐process—the role of information acquisition. Personnel Psychol. 1992;45(4):849–874. , .
- The moderating effect of tenure in person‐environment fit: a field study in educational organizations. J Occup Organ Psych. 1997;70:173–188. , .
- Matching people and organizations—selection and socialization in public accounting firms. Admin Sci Quart. 1991;36(3):459–484. .
- Effects of physician experience on costs and outcomes on an academic general medicine service: results of a trial of hospitalists. Ann Intern Med. 2002;137(11):866–874. , , , et al.
- Worklife and satisfaction of hospitalists: toward flourishing careers. J Gen Intern Med. 2012;27(1):28–36. , , , , .
- Karasek's model in the People's Republic of China: effects of job demands, control, and individual differences. Acad Manage J. 1996;39(6):1594–1618. .
- Measuring physician job satisfaction in a changing workplace and a challenging environment. SGIM Career Satisfaction Study Group. Society of General Internal Medicine. Med Care. 1999;37(11):1174–1182. , , , et al.
- Organizational climate, stress, and error in primary care: The MEMO Study. Adv Patient Saf. 2005;1:65–77. , , , et al.
- Effects of inpatient experience on outcomes and costs in a multicenter trial of academic hospitalists. J Gen Intern Med. 2005;20(suppl 1):141–142. , , , et al.
- Burnout and self‐reported patient care in an internal medicine residency program. Ann Intern Med. 2002;136(5):358–367. , , , .
- Effect of job demands and social support on worker stress—a study of VDT users. Behav Inform Technol. 1995;14(1):32–40. , .
- Validation of a single‐item measure of burnout against the Maslach Burnout Inventory among physicians. Stress Health. 2004;20(2):75–79. , , .
- Job satisfaction: a meta‐analysis of stabilities. J Organ Behav. 2001;22(5):483–504. , .
- The American Association for Public Opinion Research. Standard definitions: final dispositions of case codes and outcome rates for surveys.7th ed. Available at: http://www.aapor.org/Standard_Definitions2.htm. Accessed May 2,2012.
- Response rates and response bias for 50 surveys of pediatricians. Health Serv Res. 2005;40(1):213–226. , , , .
- Accountable care organizations: accountable for what, to whom, and how. JAMA. 2010;304(15):1715–6. , .
Copyright © 2012 Society of Hospital Medicine
Hospitalist Practice Models
Over the past 15 years, there has been dramatic growth in the number of hospitalist physicians in the United States and in the number of hospitals served by them.13 Hospitals are motivated to hire experienced hospitalists to staff their inpatient services,4 with goals that include obtaining cost‐savings and higher quality.59 The rapid growth of Hospital Medicine saw multiple types of hospital practice models emerge with differing job characteristics, clinical duties, workload, and compensation schemes.10 The extent of the variability of hospitalist jobs across practice models is not known.
Intensifying recruitment efforts and the concomitant increase in compensation for hospitalists over the last decade suggest that demand for hospitalists is strong and sustained.11 As a result, today's cohort of hospitalists has a wide range of choices of types of jobs, practice models, and locations. The diversity of available hospitalist jobs is characterized, for example, by setting (community hospital vs academic hospital), employer (hospital vs private practice), job duties (the amount and type of clinical work, and other administrative, teaching, or research duties), and intensity (work hours and duties to maximize income or lifestyle). How these choices relate to job satisfaction and burnout are also unknown.
The Society of Hospital Medicine (SHM) has administered surveys to hospitalist group leaders biennially since 2003.1215 These surveys, however, do not address issues related to individual hospitalist worklife, recruitment, and retention. In 2005, SHM convened a Career Satisfaction Task Force that designed and executed a national survey of hospitalists in 2009‐2010. The objective of this study is to evaluate how job characteristics vary by practice model, and the association of these characteristics and practice models with job satisfaction and burnout.
METHODS
Survey Instrument
A detailed description of the survey design, sampling strategy, data collection, and response rate calculations is described elsewhere.16 Portions of the 118‐item survey instrument assessed characteristics of the respondents' hospitalist group (12 items), details about their individual work patterns (12 items), and demographics (9 items). Work patterns were evaluated by the average number of clinical work days, consecutive days, hours per month, percentage of work assigned to night duty, and number of patient encounters. Average hours spent on nonclinical work, and the percentage of time allocated for clinical, administrative, teaching, and research activities were solicited. Additional items assessed specific clinical responsibilities, pretax earnings in FY2010, the availability of information technology capabilities, and the adequacy of available resources. Job and specialty satisfaction and 11 satisfaction domain measures were measured using validated scales.1726 Burnout symptoms were measured using a validated single‐item measure.26, 27
Sampling Strategy
We surveyed a national stratified sample of hospitalists in the US and Puerto Rico. We used the largest database of hospitalists (>24,000 names) currently available and maintained by the SHM as our sampling frame. We linked hospitalist employer information to hospital statistics from the American Hospital Association database28 to stratify the sample by number of hospital beds, geographic region, employment model, and specialty training, oversampling pediatric hospitalists due to small numbers. A respondent sample of about 700 hospitalists was calculated to be adequate to detect a 0.5 point difference in job satisfaction scores between subgroups assuming 90% power and alpha of 0.05. However, we sampled a total of 5389 addresses from the database to overcome the traditionally low physician response rates, duplicate sampling, bad addresses, and non‐hospitalists being included in the sampling frame. In addition, 2 multistate hospitalist companies (EmCare, In Compass Health) and 1 for‐profit hospital chain (HCA, Inc) financially sponsored this project with the stipulation that all of their hospitalist employees (n = 884) would be surveyed.
Data Collection
The healthcare consulting firm, Press Ganey, provided support with survey layout and administration following the modified Dillman method.29 Three rounds of coded surveys and solicitation letters from the investigators were mailed 2 weeks apart in November and December 2009. Because of low response rates to the mailed survey, an online survey was created using Survey Monkey and sent to 650 surveyees for whom e‐mail addresses were available, and administered at a kiosk for sample physicians during the SHM 2010 annual meeting.
Data Analysis
Nonresponse bias was measured by comparing characteristics between respondents of separate survey waves.30 We determined the validity of mailing addresses immediately following the survey period by mapping each address using Google, and if the address was a hospital, researching online whether or not the intended recipient was currently employed there. Practice characteristics were compared across 5 model categories distilled from the SHM & Medical Group Management Association survey: local hospitalist‐only group, multistate hospitalist group, multispecialty physician group, employer hospital, and university or medical school. Weighted proportions, means, and medians were calculated to account for oversampling of pediatric hospitalists. Differences in categorical measures were assessed using the chi‐square test and the design‐based F test for comparing weighted data. Weighted means (99% confidence intervals) and medians (interquartile ranges) were calculated. Because each parameter yielded a single outlier value across the 5 practice models, differences across weighted means were assessed using generalized linear models with the single outlier value chosen as the reference mean. Pair‐wise Wilcoxon rank sum test was used to compare median values. In these 4‐way comparisons of means and medians, significance was defined as P value of 0.0125 per Bonferroni correction. A single survey item solicited respondents to choose exactly 4 of 13 considerations most pertinent to job satisfaction. The proportion of respondents who scored 4 on a 5‐point Likert scale of the 11 satisfaction domains and 2 global measures of satisfaction, and burnout symptoms defined as 3 on a 5‐point single item measure were bar‐graphed. Chi‐square statistics were used to evaluate for differences across practice models. Statistical significance was defined by alpha less than 0.05, unless otherwise specified. All analyses were performed using STATA version 11.0 (College Station, TX). This study was approved by the Loyola University Institutional Review Board.
Survey data required cleaning prior to analysis. Missing gender information was imputed using the respondents' name. Responses to the item that asked to indicate the proportion of work dedicated to administrative responsibilities, clinical care, teaching, and research that did not add up to 100% were dropped. Two responses that indicated full‐time equivalent (FTE) of 0%, but whose respondents otherwise completed the survey implying they worked as clinical hospitalists, were replaced with values calculated from the given number of work hours relative to the median work hours in our sample. Out of range or implausible responses to the following items were dropped from analyses: the average number of billable encounters during a typical day or shift, number of shifts performing clinical activities during a typical month, pretax earnings, the year the respondent completed residency training, and the number of whole years practiced as a hospitalist. The proportion of selective item nonresponse was small and we did not, otherwise, impute missing data.
RESULTS
Response Rate
Of the 5389 originally sampled addresses, 1868 were undeliverable. Addresses were further excluded if they appeared in duplicate or were outdated. This yielded a total of 3105 eligible surveyees in the sample. As illustrated in Figure 1, 841 responded to the mailed survey and 5 responded to the Web‐based survey. After rejecting 67 non‐hospitalist respondents and 3 duplicate surveys, a total of 776 surveys were included in the final analysis. The adjusted response rate was 25.6% (776/3035). Members of SHM were more likely to return the survey than nonmembers. The adjusted response rate from hospitalists affiliated with the 3 sponsoring institutions was 6% (40/662). Because these respondents were more likely to be non‐members of SHM, we opted to analyze the responses from the sponsor hospitalists together with the sampled hospitalists. The demographics of the resulting pool of 816 respondents affiliated with over 650 unique hospitalist groups were representative of the original survey frame. We analyzed data from 794 of these who responded to the item indicating their hospitalist practice model. Demographic characteristics of responders and nonresponders to the practice model survey item were similar.
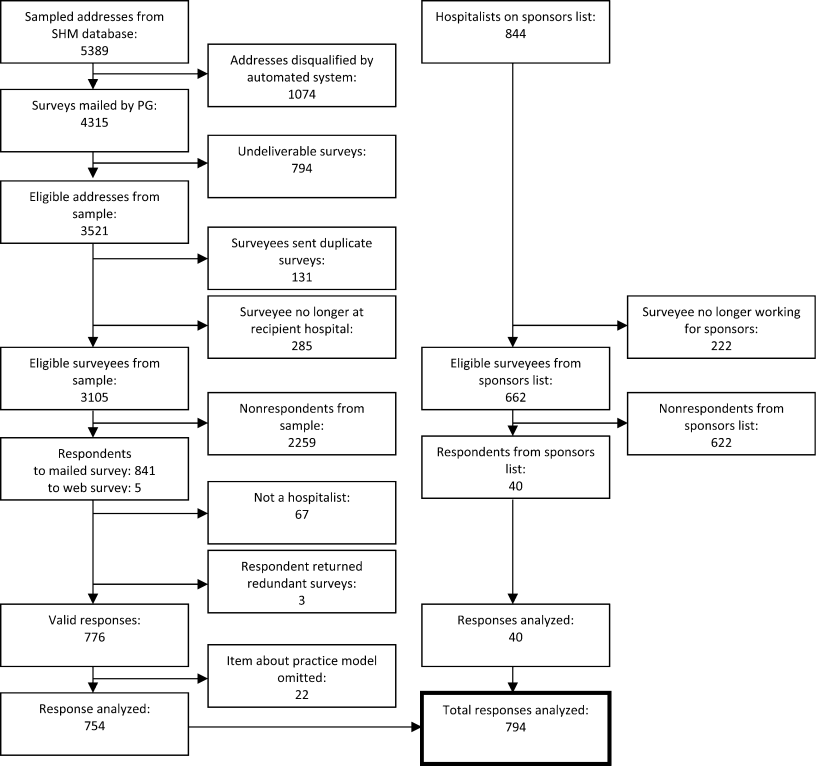
Characteristics of Hospitalists and Their Groups
Table 1 summarizes the characteristics of hospitalist respondents and their organizations by practice model. More (44%) respondents identified their practice model as directly employed by the hospital than other models, including multispecialty physician group (15%), multistate hospitalist group (14%), university or medical school (14%), local hospitalist group (12%), and other (2%). The median age of hospitalist respondents was 42 years, with 6.8 years of mean experience as a hospitalist. One third were women, 84% were married, and 46% had dependent children 6 years old or younger at home. Notably, hospitalists in multistate groups had fewer years of experience, and fewer hospitalists in local and multistate groups were married compared to hospitalists in other practice models.
Local Hospitalist‐Only Group | Multi‐State Hospitalist Group | Multispecialty Physician Group | Employer Hospital | University or Medical School | ||
---|---|---|---|---|---|---|
n = 95 | n = 111 | n = 115 | n = 348 | n = 107 | P Value | |
| ||||||
Hospitalist characteristics | ||||||
Age, weighted mean (99% CI) | 45 (42, 48) | 44 (42, 47) | 45 (43, 47) | 45 (43, 46) | 43 (40, 46) | |
Years hospitalist experience, weighted mean (99% CI) | 8 (6, 9)* | 5 (4, 6)* | 8 (7, 9) | 7 (6, 7) | 8 (6, 9) | <0.010* |
Women, weighted % | 29 | 30 | 39 | 31 | 43 | 0.118 |
Married, weighted % | 76 | 77 | 82 | 89 | 81 | 0.009 |
At least 1 dependent child younger than age 6 living in home, weighted % | 47 | 48 | 43 | 47 | 45 | 0.905 |
Pediatric specialty, n (%) | <10 | <10 | 11 (10%) | 57 (16%) | 36 (34%) | <0.001 |
Hospitalist group characteristics | ||||||
Region, weighted % | <0.001 | |||||
Northeast (AHA 1 & 2) | 13 | 10 | 16 | 27 | 13 | |
South (AHA 3 & 4) | 19 | 37 | 13 | 24 | 21 | |
Midwest (AHA 5 & 6) | 23 | 24 | 25 | 22 | 26 | |
Mountain (AHA 7 & 8) | 22 | 20 | 16 | 13 | 24 | |
West (AHA 9) | 24 | 10 | 31 | 14 | 16 | |
No. beds of primary hospital, weighted % | <0.001 | |||||
Up to 149 | 17 | 26 | 12 | 24 | 14 | |
150299 | 30 | 36 | 36 | 33 | 21 | |
300449 | 26 | 24 | 29 | 20 | 19 | |
450599 | 13 | 8 | 17 | 11 | 21 | |
600 or more | 12 | 6 | 7 | 13 | 24 | |
No. of hospital facilities served by current practice, weighted % | <0.001 | |||||
1 | 53 | 70 | 67 | 77 | 66 | |
2 | 20 | 22 | 20 | 16 | 24 | |
3 or more | 27 | 9 | 13 | 7 | 10 | |
No. of physicians in current practice, median (IQR) | 10 (5, 18) | 8 (6, 12)* | 14 (8, 25)* | 12 (6, 18) | 12 (7, 20) | <0.001*, 0.001 |
No. of non‐physician providers in current practice, median (IQR) | 0 (0, 2) | 0 (0, 2) | 0 (0, 3) | 1 (0, 2) | 0 (0, 2) | |
Available information technology capabilities, weighted % | ||||||
EHR to access physician notes | 57 | 57 | 75 | 58 | 79 | <0.001 |
EHR to access nursing documentations | 68 | 67 | 74 | 75 | 76 | 0.357 |
EHR to access laboratory or test results | 97 | 89 | 95 | 96 | 96 | 0.054 |
Electronic order entry | 30 | 19 | 53 | 38 | 56 | <0.001 |
Electronic billing | 38 | 31 | 36 | 36 | 38 | 0.818 |
Access to EHR at home or off site | 78 | 73 | 78 | 82 | 84 | 0.235 |
Access to Up‐to‐Date or other clinical guideline resources | 80 | 77 | 91 | 92 | 96 | <0.001 |
Access to schedules, calendars, or other organizational resources | 56 | 57 | 66 | 67 | 75 | 0.024 |
E‐mail, Web‐based paging, or other communication resources | 74 | 63 | 88 | 89 | 90 | <0.001 |
Several differences in respondent group characteristics by practice model were found. Respondents in multistate hospitalist groups were more likely from the South and Midwest, while respondents from multispecialty groups were likely from the West. More multistate group practices were based in smaller hospitals, while academic hospitalists tended to practice in hospitals with 600 or more beds. Respondents employed by hospitals were more likely to practice at 1 hospital facility only, while local group practices were more likely to practice at 3 or more facilities. The median number of physicians in a hospitalist group was 11 (interquartile range [IQR] 6, 19). Local and multistate groups had fewer hospitalists compared to other models. Nonphysician providers were employed by nearly half of all hospitalist practices. Although almost all groups had access to some information technology, more academic hospitalists had access to electronic order entry, electronic physician notes, electronic clinical guidelines resources and communication technology, while local and multistate groups were least likely to have access to these resources.
Work Pattern Variations
Table 2 further details hospitalist work hours by practice model. The majority of hospitalists (78%) reported their position was full‐time (FTE 1.0), while 13% reported working less than full‐time (FTE <1.0). Only 5% of local group hospitalists worked part‐time, while 20% of multispecialty group hospitalists did. An additional 9% reported FTE >1.0, indicating their work hours exceeded the definition of a full‐time physician in their practice. Among full‐time hospitalists, local group members worked a greater number of shifts per month than employees of multispecialty groups, hospitals, and academic medical centers. Academic hospitalists reported higher numbers of consecutive clinical days worked on average, but fewer night shifts compared to hospitalists employed by multistate groups, multispecialty groups, and hospitals; fewer billable encounters than hospitalists in local and multistate groups; and more nonclinical work hours than hospitalists of any other practice model. Academic hospitalists also spent more time on teaching and research than other practice models. Hospitalists spent 11%‐18% of their time on administrative and committee responsibilities, with the least amount spent by hospitalists in multistate groups and the most in academic practice.
Local Hospitalist‐Only Group | Multi‐State Hospitalist Group | Multispecialty Physician Group | Employer Hospital | University or Medical School | ||
---|---|---|---|---|---|---|
n = 95 | n = 111 | n = 115 | n = 348 | n = 107 | P Value | |
| ||||||
FTE, weighted % | 0.058 | |||||
FTE < 1.0 | 6 | 13 | 20 | 12 | 14 | |
FTE = 1.0 | 85 | 75 | 74 | 80 | 82 | |
FTE > 1.0 | 10 | 13 | 6 | 8 | 5 | |
Workload parameters, weighted mean (99% CI) | ||||||
Clinical shifts per month for FTE 1.0 | 19 (17, 20)* | 17 (16, 19) | 15 (14, 17)* | 16 (15, 16) | 15 (13, 17) | <0.001* |
Hours per clinical shift | 10 (9, 11) | 11 (10, 11)* | 10 (10, 11.0) | 11 (10, 11.0) | 10 (9, 10)* | 0.006*, 0.002 |
Consecutive days on clinical shift | 8 (6, 9) | 7 (6, 7)* | 6 (6, 7) | 7 (6, 7) | 9 (7, 10)* | 0.002*, <0.001 |
% Clinical shifts on nights | 20 (15, 25) | 23 (18, 28)* | 23 (17, 29) | 21 (17, 24) | 14 (9, 18)* | 0.001*, 0.002 |
% Night shifts spent in hospital | 61 (49, 74)* | 63 (52, 75) | 72 (62, 83) | 73 (67, 80) | 43 (29, 57)* | 0.010*, 0.003, <0.001 |
Billable encounters per clinical shift | 17 (14, 19)* | 17 (16, 18) | 14 (13, 15) | 15 (14, 16) | 13 (11, 14)* | <0.001*, 0.002 |
Hours nonclinical work per month | 23 (12, 34)* | 19 (11, 27) | 31 (20, 42) | 30 (24, 36) | 71 (55, 86)* | <0.001* |
Hours clinical and nonclinical work per month for FTE 1.0 | 202 (186, 219) | 211 (196, 226) | 184 (170, 198)* | 193 (186, 201) | 221 (203, 238)* | <0.001* |
Professional activity, weighted mean % (99% CI) | ||||||
Clinical | 84 (78, 89)* | 86 (81, 90) | 78 (72, 84) | 79 (76, 82) | 58 (51, 64)* | <0.001* |
Teaching | 2.3 (1, 5)* | 3 (1, 4) | 6 (4, 9) | 6 (5, 8) | 17 (14, 20)* | <0.001* |
Administration and Committee work | 13 (8, 19) | 11 (8, 15)* | 16 (10, 21) | 14 (12, 17) | 19 (14, 24)* | 0.001* |
Research | 0 (0, 0)* | 1 (0, 2) | 0 (0, 1) | 1 (0, 1) | 7 (3, 11)* | <0.001* |
Table 3 tabulates other work pattern characteristics. Most hospitalists indicated that their current clinical work as hospitalists involved the general medical wards (100%), medical consultations (98%), and comanagement with specialists (92%). There were wide differences in participation in comanagement (100%, local groups vs 71%, academic), intensive care unit (ICU) responsibilities (94%, multistate groups vs 27%, academic), and nursing home care (30%, local groups vs 8%, academic). Among activities that are potentially not reimbursable, academic hospitalists were less likely to participate in coordination of patient transfers and code or rapid response teams, while multistate groups were least likely to participate in quality improvement activities. In total, 99% of hospitalists reported participating in at least 1 potentially nonreimbursable clinical activity.
Local Hospitalist‐Only Group | Multi‐State Hospitalist Group | Multispecialty Physician Group | Employer Hospital | University or Medical School | ||
---|---|---|---|---|---|---|
n = 95 | n = 111 | n = 115 | n = 348 | n = 107 | P Value | |
| ||||||
Reimbursable activities, overlapping weighted % | ||||||
General medical ward | 100 | 99 | 100 | 99 | 99 | 0.809 |
Medical consultations | 99 | 99 | 100 | 98 | 95 | 0.043 |
Comanagement with specialists | 100 | 96 | 96 | 93 | 71 | <0.001 |
Preoperative evaluations | 92 | 92 | 90 | 88 | 77 | 0.002 |
Intensive care unit | 86 | 94 | 67 | 75 | 27 | <0.001 |
Skilled nursing facility or long‐term acute care facility | 30 | 19 | 12 | 16 | 8 | <0.001 |
Outpatient general medical practice | 4 | 4 | 5 | 5 | 10 | 0.241 |
Potentially nonreimbursable activities, overlapping weighted % | ||||||
Coordination of patient transfers | 92 | 94 | 95 | 93 | 82 | 0.005 |
Quality improvement or patient safety initiatives | 81 | 78 | 83 | 89 | 89 | 0.029 |
Code team or rapid response team | 56 | 57 | 53 | 62 | 37 | <0.001 |
Information technology design or implementation | 42 | 39 | 47 | 51 | 51 | 0.154 |
Admission triage for emergency department | 49 | 46 | 43 | 40 | 31 | 0.132 |
Compensation scheme, weighted % | <0.001 | |||||
Salary only | 18 | 21 | 30 | 29 | 47 | |
Salary plus performance incentive | 54 | 72 | 59 | 67 | 53 | |
Fee‐for‐service | 20 | 1 | 7 | 2 | 0 | |
Capitation | 0 | 0 | 0 | 0 | 0 | |
Other | 9 | 7 | 4 | 3 | 0 | |
Compensation links to incentives, overlapping weighted % | ||||||
No incentives | 40 | 28 | 29 | 29 | 48 | 0.003 |
Patient satisfaction | 23 | 39 | 38 | 38 | 14 | <0.001 |
Length of stay | 18 | 17 | 20 | 13 | 10 | 0.208 |
Overall cost | 8 | 11 | 9 | 5 | 6 | 0.270 |
Test utilization | 2 | 2 | 7 | 1 | 0 | <0.001 |
Clinical processes and outcomes | 26 | 34 | 44 | 43 | 24 | <0.001 |
Other | 17 | 29 | 26 | 31 | 25 | 0.087 |
Earnings, weighted mean dollars (99% CI) | 226,065 (202,891, 249,240)* | 225,613 (210,772, 240,454) | 202,617 (186,036, 219,198) | 206,087 (198,413, 213,460) | 166,478 (151,135, 181,821)* | <0.001* |
Hospitalist compensation schemes were significantly different across the practice models. Salary‐only schemes were most common among academic hospitalists (47%), while 72% of multistate groups used performance incentives in addition to salary. More local groups used fee‐for‐service compensation than other models. Incentives differed by practice model, with more multistate groups having incentives based on patient satisfaction, while more multispecialty physician groups had incentives based on clinical processes and outcomes than other models. Finally, mean earnings for academic hospitalists were significantly lower than for hospitalists of other practice models. Local and multistate group hospitalists earned more than any other practice model (all P <0.001), and $60,000 more than the lowest compensated academic hospitalists.
Components of Job Satisfaction
Hospitalists' rankings of the most important factors for job satisfaction revealed differences across models (Figure 2). Overall, hospitalists were most likely to consider optimal workload and compensation as important factors for job satisfaction from a list of 13 considerations. Local groups and academics were least likely to rank optimal workload as a top factor, and local group hospitalists were more likely to rank optimal autonomy than those of other models. Academic hospitalists had less concern for substantial pay, and more concern for the variety of tasks they perform and recognition by leaders, than other hospitalists.
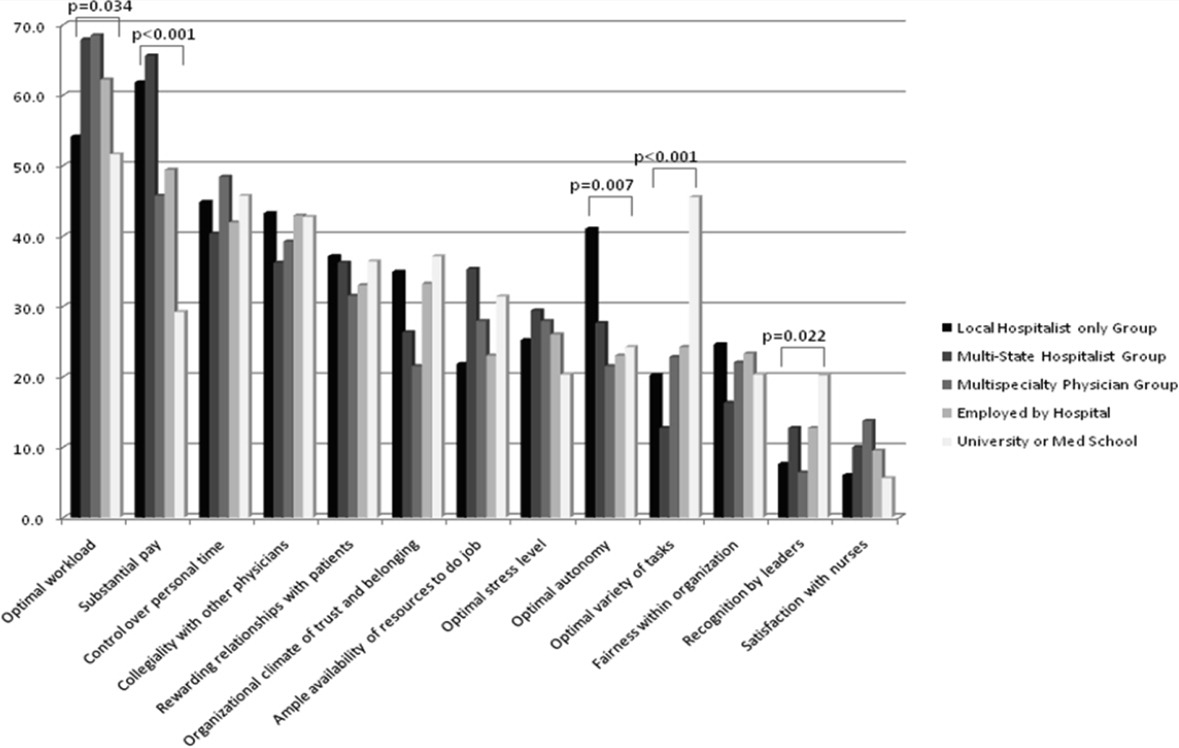
Job Satisfaction and Burnout Risk
Differences in the ratings of 4 of the 11 satisfaction and job characteristic domains were found across the practice models (Figure 3). Multispecialty group hospitalists were less satisfied with autonomy and their relationship with patients than other practice models, and along with multistate groups, reported the highest perceived workload. Organizational fairness was rated much higher by local group hospitalists than other practice models. Despite these differences in work patterns and satisfaction, there were no differences found in level of global job satisfaction, specialty satisfaction, or burnout across the practice models. Overall, 62% of respondents reported high job satisfaction (4 on a 1 to 5 scale), and 30% indicated burnout symptoms.
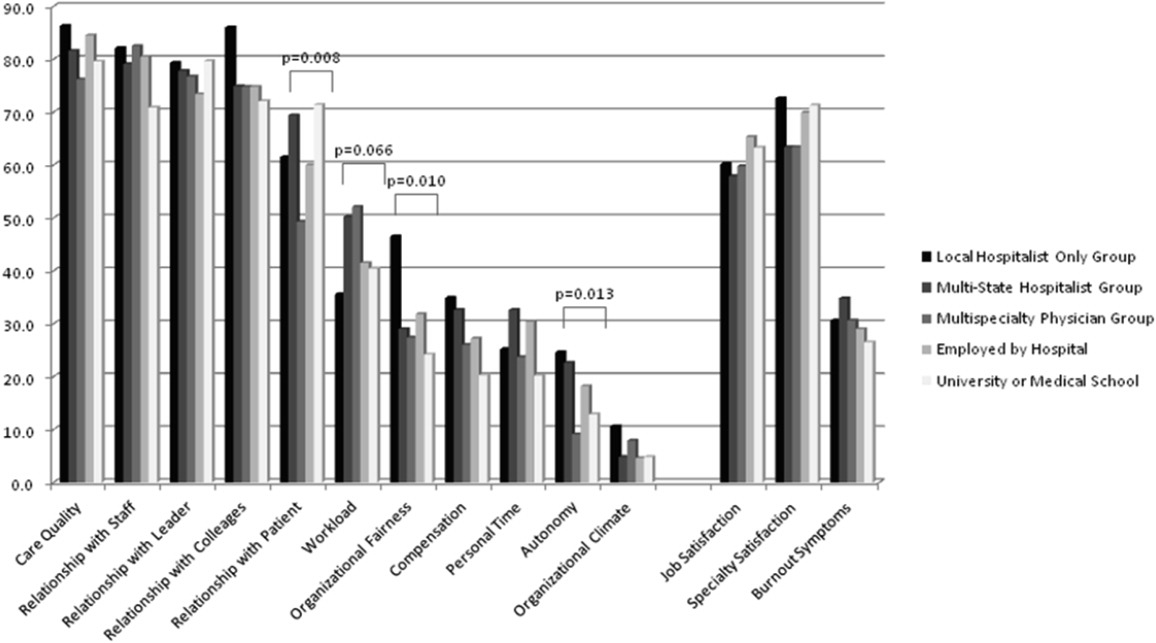
DISCUSSION
In our sample of US hospitalists, we found major differences in work patterns and compensation across hospitalist practice models, but no differences in job satisfaction, specialty satisfaction, and burnout. In particular, differences across these models included variations in hospitalist workload, hours, pay, and distribution of work activities. We found that hospitalists perform a variety of clinical and nonclinical tasks, for many of which there are not standard reimbursement mechanisms. We also found that features of a job that individual hospitalists considered most important vary by practice model.
Previous analysis of this data explored the overall state of hospitalist satisfaction.16 The present analysis offers a glimpse into hospitalists' systems‐orientation through a deeper look at their work patterns. The growth in the number of hospitalists who participate in intensive care medicine, specialty comanagement, and other work that involves close working relationships with specialist physicians confirms collaborative care as one of the dominant drivers of the hospitalist movement. At the level of indirect patient care, nearly all hospitalists contributed to work that facilitates coordination, quality, patient safety, or information technology. Understanding the integrative value of hospitalists outside of their clinical productivity may be of interest to hospital administrators.
Global satisfaction measures were similar across practice models. This finding is particularly interesting given the major differences in job characteristics seen among the practice models. This similarity in global satisfaction despite real differences in the nature of the job suggests that individuals find settings that allow them to address their individual professional goals. Our study demonstrates that, in 2010, Hospital Medicine has evolved enough to accommodate a wide variety of goals and needs.
While global satisfaction did not differ among practice types, hospitalists from various models did report differences in factors considered important to global satisfaction. While workload and pay were rated as influential across most models, the degree of importance was significantly different. In academic settings, substantial pay was not a top consideration for overall job satisfaction, whereas in local and multistate hospitalist groups, pay was a very close second in importance to optimal workload. These results may prove helpful for individual hospitalists trying to find their optimal job. For example, someone who is less concerned about workload, but wants to be paid well and have a high degree of autonomy, may find satisfaction in local hospitalist groups. However, for someone who is willing to sacrifice a higher salary for variety of activities, academic Hospital Medicine may be a better fit.
There is a concerning aspect of hospitalist job satisfaction that different practice models do not seem to solve. Control over personal time is a top consideration for many hospitalists across practice models, yet their satisfaction with personal time is low. As control over personal time is seen as a draw to the Hospital Medicine specialty, group leaders may need to evaluate their programs to ensure that schedules and workload support efforts for hospitalists to balance work and homelife commitments.
There are additional findings that are important for Hospital Medicine group leaders. Regardless of practice model, compensation and workload are often used as tools to recruit and retain hospitalists. While these tools may be effective, leaders may find more nuanced approaches to improving their hospitalists' overall satisfaction. Leaders of local hospitalist groups may find their hospitalists tolerant of heavier workloads as long as they are adequately rewarded and are given real autonomy over their work. However, leaders of academic programs may be missing the primary factor that can improve their hospitalists' satisfaction. Rather than asking for higher salaries to remain competitive, it may be more effective to advocate for time and training for their hospitalists to pursue important other activities beyond direct clinical care. Given that resources will always be limited, group leaders need to understand all of the elements that can contribute to hospitalist job satisfaction.
We point out several limitations to this study. First, our adjusted response rate of 25.6% is low for survey research, in general. As mentioned above, hospitalists are not easily identified in any available national physician database. Therefore, we deliberately designed our sampling strategy to error on the side of including ineligible surveyees to reduce systematic exclusion of practicing hospitalists. Using simple post hoc methods, we identified many nonhospitalists and bad addresses from our sample, but because these methods were exclusionary as opposed to confirmatory, we believe that a significant proportion of remaining nonrespondents may also have been ineligible for the survey. Although this does not fully address concerns about potential response bias, we believe that our sample representing a large number of hospitalist groups is adequate to make estimations about a nationally representative sample of practicing hospitalists. Second, in spite of our inclusive approach, we may still have excluded categories of practicing hospitalists. We were careful not to allow SHM members to represent all US hospitalists and included non‐members in the sampling frame, but the possibility of systematic exclusion that may alter our results remains a concern. Additionally, one of our goals was to characterize pediatric hospitalists independently from their adult‐patient counterparts. Despite oversampling of pediatricians, their sample was too small for a more detailed comparison across practice models. Also, self‐reported data about workload and compensation are subject to inaccuracies related to recall and cognitive biases. Last, this is a cross‐sectional study of hospitalist satisfaction at one point in time. Consequently, our sample may not be representative of very dissatisfied hospitalists who have already left their jobs.
The diversity found across existing practice models and the characteristics of the practices provide physicians with the opportunity to bring their unique skills and motivations to the hospitalist movement. As hospitals and other organizations seek to create, maintain, or grow hospitalist programs, the data provided here may prove useful to understand the relationship between practice characteristics and individual job satisfaction. Additionally, hospitalists looking for a job can consider these results as additional information to guide their choice of practice model and work patterns.
Acknowledgements
The authors thank Kenneth A. Rasinski for assistance with survey items refinement, and members of the SHM Career Satisfaction Task Force for their assistance in survey development.
- The status of hospital medicine groups in the United States.J Hosp Med.2006;1(2):75–80. , , , .
- Growth in the care of older patients by hospitalists in the United States.N Engl J Med.2009;360(11):1102–1112. , , , .
- The state of hospital medicine in 2008.Med Clin North Am.2008;92(2):265–273,vii. .
- Health care market trends and the evolution of hospitalist use and roles.J Gen Intern Med.2005;20(2):101–107. , , , .
- Effects of physician experience on costs and outcomes on an academic general medicine service: results of a trial of hospitalists.Ann Intern Med.2002;137(11):866–874. , , , et al.
- The Park Nicollet experience in establishing a hospitalist system.Ann Intern Med.1999;130(4 pt 2):350–354. .
- Effects of an HMO hospitalist program on inpatient utilization.Am J Manag Care.2001;7(11):1051–1057. , .
- The impact of hospitalists on the cost and quality of inpatient care in the United States: a research synthesis.Med Care Res Rev.2005;62(4):379–406. , .
- Pediatric hospitalists: a systematic review of the literature.Pediatrics.2006;117(5):1736–1744. , , , .
- The hospitalist movement 5 years later.JAMA.2002;287(4):487–494. , .
- Trends in market demand for internal medicine 1999 to 2004: an analysis of physician job advertisements.J Gen Intern Med.2006;21(10):1079–1085. , , , , , .
- SHM. 2003–2004 Survey by the Society of Hospital Medicine on Productivity and Compensation: Analysis of Results. 2004 [updated 2004]. Available at: http://www.hospitalmedicine.org/AM/Template. cfm?Section=Practice_Resources Available at: http://cme.medscape.com/viewarticle/578134. Accessed October 21,2010.
- State of Hospital Medicine: 2010 Report Based on 2009 Data.Englewood, CO and Philadelphia, PA:Medical Group Management Association and Society of Hospital Medicine;2010.
- Worklife and satisfaction of hospitalists: toward flourishing careers.J Gen Intern Med.2011, Jul 20. PMID: 21773849. , , , , .
- Worklife and satisfaction of general internists.Arch Intern Med.2002;162(6):649–656. , , , et al.
- Organizational climate, stress, and error in primary care: the MEMO study. In: Henriksen K, Battles JB, Marks ES, Lewin DI, eds.Advances in Patient Safety: From Research to Implementation. Vol 1: Research Findings.Rockville, MD:Agency for Healthcare Research and Quality;2005;1:65–77. , , , et al.
- Hospitalists and the practice of inpatient medicine: results of a survey of the National Association of Inpatient Physicians.Ann Intern Med.1999;130(4 pt 2):343–349. , , , .
- Physician attitudes toward and prevalence of the hospitalist model of care: results of a national survey.Am J Med.2000;109(8):648–653. , , , , , .
- Taking the Measure of Work: A Guide to Validated Scales for Organizational Research and Diagnosis.Thousand Oaks, CA:Sage Publications;2002. .
- Job Demands and Worker Health.Ann Arbor, MI:University of Michigan, Institute for Social Research;1980. , , , , .
- On the dimensionality of organizational justice: a construct validation of a measure.J Appl Psychol.2001;86(3):386–400. .
- Effect of job demands and social support on worker stress—a study of VDT users.Behav Inform Technol.1995;14(1):32–40. , .
- Measuring physician job satisfaction in a changing workplace and a challenging environment. SGIM Career Satisfaction Study Group. Society of General Internal Medicine.Med Care.1999;37(11):1174–1182. , , , et al.
- Working conditions in primary care: physician reactions and care quality.Ann Intern Med.2009;151(1):28–U48. , , , et al.
- Validation of a single‐item measure of burnout against the Maslach Burnout Inventory among physicians.Stress Health.2004;20(2):75–79. , , .
- American Hospital Association. AHA Hospital Statistics. 2009 [updated 2009]. Available at: http://www.ahadata.com/ahadata/html/AHAStatistics.html. Accessed April 12,2011.
- How to obtain excellent response rates when surveying physicians.Fam Pract.2009;26(1):65–68. , , , et al.
- Estimating nonresponse bias in mail surveys.J Marketing Res.1977;14(3):396–402. , .
Over the past 15 years, there has been dramatic growth in the number of hospitalist physicians in the United States and in the number of hospitals served by them.13 Hospitals are motivated to hire experienced hospitalists to staff their inpatient services,4 with goals that include obtaining cost‐savings and higher quality.59 The rapid growth of Hospital Medicine saw multiple types of hospital practice models emerge with differing job characteristics, clinical duties, workload, and compensation schemes.10 The extent of the variability of hospitalist jobs across practice models is not known.
Intensifying recruitment efforts and the concomitant increase in compensation for hospitalists over the last decade suggest that demand for hospitalists is strong and sustained.11 As a result, today's cohort of hospitalists has a wide range of choices of types of jobs, practice models, and locations. The diversity of available hospitalist jobs is characterized, for example, by setting (community hospital vs academic hospital), employer (hospital vs private practice), job duties (the amount and type of clinical work, and other administrative, teaching, or research duties), and intensity (work hours and duties to maximize income or lifestyle). How these choices relate to job satisfaction and burnout are also unknown.
The Society of Hospital Medicine (SHM) has administered surveys to hospitalist group leaders biennially since 2003.1215 These surveys, however, do not address issues related to individual hospitalist worklife, recruitment, and retention. In 2005, SHM convened a Career Satisfaction Task Force that designed and executed a national survey of hospitalists in 2009‐2010. The objective of this study is to evaluate how job characteristics vary by practice model, and the association of these characteristics and practice models with job satisfaction and burnout.
METHODS
Survey Instrument
A detailed description of the survey design, sampling strategy, data collection, and response rate calculations is described elsewhere.16 Portions of the 118‐item survey instrument assessed characteristics of the respondents' hospitalist group (12 items), details about their individual work patterns (12 items), and demographics (9 items). Work patterns were evaluated by the average number of clinical work days, consecutive days, hours per month, percentage of work assigned to night duty, and number of patient encounters. Average hours spent on nonclinical work, and the percentage of time allocated for clinical, administrative, teaching, and research activities were solicited. Additional items assessed specific clinical responsibilities, pretax earnings in FY2010, the availability of information technology capabilities, and the adequacy of available resources. Job and specialty satisfaction and 11 satisfaction domain measures were measured using validated scales.1726 Burnout symptoms were measured using a validated single‐item measure.26, 27
Sampling Strategy
We surveyed a national stratified sample of hospitalists in the US and Puerto Rico. We used the largest database of hospitalists (>24,000 names) currently available and maintained by the SHM as our sampling frame. We linked hospitalist employer information to hospital statistics from the American Hospital Association database28 to stratify the sample by number of hospital beds, geographic region, employment model, and specialty training, oversampling pediatric hospitalists due to small numbers. A respondent sample of about 700 hospitalists was calculated to be adequate to detect a 0.5 point difference in job satisfaction scores between subgroups assuming 90% power and alpha of 0.05. However, we sampled a total of 5389 addresses from the database to overcome the traditionally low physician response rates, duplicate sampling, bad addresses, and non‐hospitalists being included in the sampling frame. In addition, 2 multistate hospitalist companies (EmCare, In Compass Health) and 1 for‐profit hospital chain (HCA, Inc) financially sponsored this project with the stipulation that all of their hospitalist employees (n = 884) would be surveyed.
Data Collection
The healthcare consulting firm, Press Ganey, provided support with survey layout and administration following the modified Dillman method.29 Three rounds of coded surveys and solicitation letters from the investigators were mailed 2 weeks apart in November and December 2009. Because of low response rates to the mailed survey, an online survey was created using Survey Monkey and sent to 650 surveyees for whom e‐mail addresses were available, and administered at a kiosk for sample physicians during the SHM 2010 annual meeting.
Data Analysis
Nonresponse bias was measured by comparing characteristics between respondents of separate survey waves.30 We determined the validity of mailing addresses immediately following the survey period by mapping each address using Google, and if the address was a hospital, researching online whether or not the intended recipient was currently employed there. Practice characteristics were compared across 5 model categories distilled from the SHM & Medical Group Management Association survey: local hospitalist‐only group, multistate hospitalist group, multispecialty physician group, employer hospital, and university or medical school. Weighted proportions, means, and medians were calculated to account for oversampling of pediatric hospitalists. Differences in categorical measures were assessed using the chi‐square test and the design‐based F test for comparing weighted data. Weighted means (99% confidence intervals) and medians (interquartile ranges) were calculated. Because each parameter yielded a single outlier value across the 5 practice models, differences across weighted means were assessed using generalized linear models with the single outlier value chosen as the reference mean. Pair‐wise Wilcoxon rank sum test was used to compare median values. In these 4‐way comparisons of means and medians, significance was defined as P value of 0.0125 per Bonferroni correction. A single survey item solicited respondents to choose exactly 4 of 13 considerations most pertinent to job satisfaction. The proportion of respondents who scored 4 on a 5‐point Likert scale of the 11 satisfaction domains and 2 global measures of satisfaction, and burnout symptoms defined as 3 on a 5‐point single item measure were bar‐graphed. Chi‐square statistics were used to evaluate for differences across practice models. Statistical significance was defined by alpha less than 0.05, unless otherwise specified. All analyses were performed using STATA version 11.0 (College Station, TX). This study was approved by the Loyola University Institutional Review Board.
Survey data required cleaning prior to analysis. Missing gender information was imputed using the respondents' name. Responses to the item that asked to indicate the proportion of work dedicated to administrative responsibilities, clinical care, teaching, and research that did not add up to 100% were dropped. Two responses that indicated full‐time equivalent (FTE) of 0%, but whose respondents otherwise completed the survey implying they worked as clinical hospitalists, were replaced with values calculated from the given number of work hours relative to the median work hours in our sample. Out of range or implausible responses to the following items were dropped from analyses: the average number of billable encounters during a typical day or shift, number of shifts performing clinical activities during a typical month, pretax earnings, the year the respondent completed residency training, and the number of whole years practiced as a hospitalist. The proportion of selective item nonresponse was small and we did not, otherwise, impute missing data.
RESULTS
Response Rate
Of the 5389 originally sampled addresses, 1868 were undeliverable. Addresses were further excluded if they appeared in duplicate or were outdated. This yielded a total of 3105 eligible surveyees in the sample. As illustrated in Figure 1, 841 responded to the mailed survey and 5 responded to the Web‐based survey. After rejecting 67 non‐hospitalist respondents and 3 duplicate surveys, a total of 776 surveys were included in the final analysis. The adjusted response rate was 25.6% (776/3035). Members of SHM were more likely to return the survey than nonmembers. The adjusted response rate from hospitalists affiliated with the 3 sponsoring institutions was 6% (40/662). Because these respondents were more likely to be non‐members of SHM, we opted to analyze the responses from the sponsor hospitalists together with the sampled hospitalists. The demographics of the resulting pool of 816 respondents affiliated with over 650 unique hospitalist groups were representative of the original survey frame. We analyzed data from 794 of these who responded to the item indicating their hospitalist practice model. Demographic characteristics of responders and nonresponders to the practice model survey item were similar.
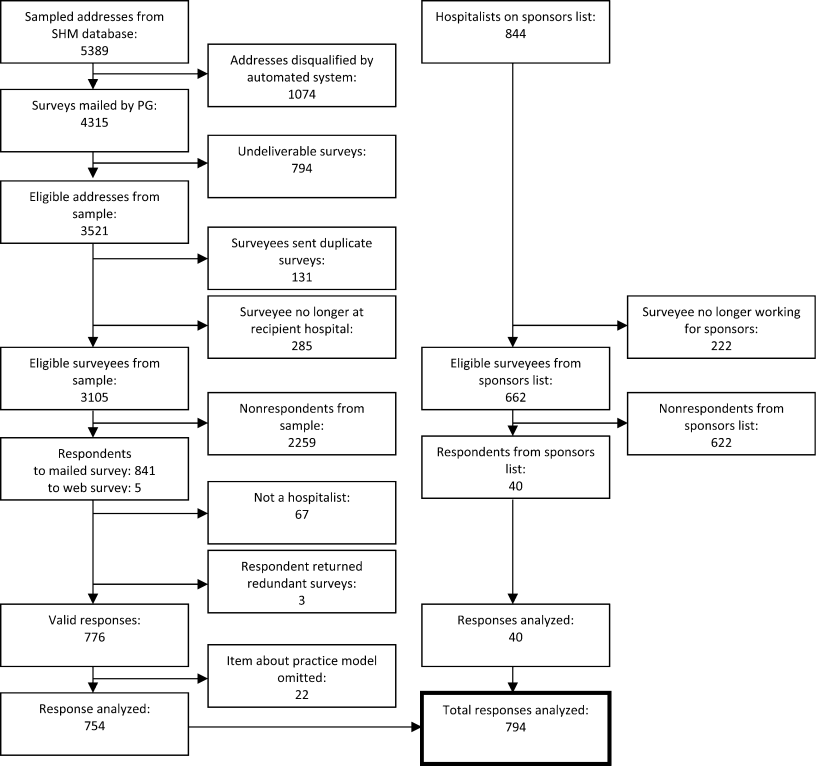
Characteristics of Hospitalists and Their Groups
Table 1 summarizes the characteristics of hospitalist respondents and their organizations by practice model. More (44%) respondents identified their practice model as directly employed by the hospital than other models, including multispecialty physician group (15%), multistate hospitalist group (14%), university or medical school (14%), local hospitalist group (12%), and other (2%). The median age of hospitalist respondents was 42 years, with 6.8 years of mean experience as a hospitalist. One third were women, 84% were married, and 46% had dependent children 6 years old or younger at home. Notably, hospitalists in multistate groups had fewer years of experience, and fewer hospitalists in local and multistate groups were married compared to hospitalists in other practice models.
Local Hospitalist‐Only Group | Multi‐State Hospitalist Group | Multispecialty Physician Group | Employer Hospital | University or Medical School | ||
---|---|---|---|---|---|---|
n = 95 | n = 111 | n = 115 | n = 348 | n = 107 | P Value | |
| ||||||
Hospitalist characteristics | ||||||
Age, weighted mean (99% CI) | 45 (42, 48) | 44 (42, 47) | 45 (43, 47) | 45 (43, 46) | 43 (40, 46) | |
Years hospitalist experience, weighted mean (99% CI) | 8 (6, 9)* | 5 (4, 6)* | 8 (7, 9) | 7 (6, 7) | 8 (6, 9) | <0.010* |
Women, weighted % | 29 | 30 | 39 | 31 | 43 | 0.118 |
Married, weighted % | 76 | 77 | 82 | 89 | 81 | 0.009 |
At least 1 dependent child younger than age 6 living in home, weighted % | 47 | 48 | 43 | 47 | 45 | 0.905 |
Pediatric specialty, n (%) | <10 | <10 | 11 (10%) | 57 (16%) | 36 (34%) | <0.001 |
Hospitalist group characteristics | ||||||
Region, weighted % | <0.001 | |||||
Northeast (AHA 1 & 2) | 13 | 10 | 16 | 27 | 13 | |
South (AHA 3 & 4) | 19 | 37 | 13 | 24 | 21 | |
Midwest (AHA 5 & 6) | 23 | 24 | 25 | 22 | 26 | |
Mountain (AHA 7 & 8) | 22 | 20 | 16 | 13 | 24 | |
West (AHA 9) | 24 | 10 | 31 | 14 | 16 | |
No. beds of primary hospital, weighted % | <0.001 | |||||
Up to 149 | 17 | 26 | 12 | 24 | 14 | |
150299 | 30 | 36 | 36 | 33 | 21 | |
300449 | 26 | 24 | 29 | 20 | 19 | |
450599 | 13 | 8 | 17 | 11 | 21 | |
600 or more | 12 | 6 | 7 | 13 | 24 | |
No. of hospital facilities served by current practice, weighted % | <0.001 | |||||
1 | 53 | 70 | 67 | 77 | 66 | |
2 | 20 | 22 | 20 | 16 | 24 | |
3 or more | 27 | 9 | 13 | 7 | 10 | |
No. of physicians in current practice, median (IQR) | 10 (5, 18) | 8 (6, 12)* | 14 (8, 25)* | 12 (6, 18) | 12 (7, 20) | <0.001*, 0.001 |
No. of non‐physician providers in current practice, median (IQR) | 0 (0, 2) | 0 (0, 2) | 0 (0, 3) | 1 (0, 2) | 0 (0, 2) | |
Available information technology capabilities, weighted % | ||||||
EHR to access physician notes | 57 | 57 | 75 | 58 | 79 | <0.001 |
EHR to access nursing documentations | 68 | 67 | 74 | 75 | 76 | 0.357 |
EHR to access laboratory or test results | 97 | 89 | 95 | 96 | 96 | 0.054 |
Electronic order entry | 30 | 19 | 53 | 38 | 56 | <0.001 |
Electronic billing | 38 | 31 | 36 | 36 | 38 | 0.818 |
Access to EHR at home or off site | 78 | 73 | 78 | 82 | 84 | 0.235 |
Access to Up‐to‐Date or other clinical guideline resources | 80 | 77 | 91 | 92 | 96 | <0.001 |
Access to schedules, calendars, or other organizational resources | 56 | 57 | 66 | 67 | 75 | 0.024 |
E‐mail, Web‐based paging, or other communication resources | 74 | 63 | 88 | 89 | 90 | <0.001 |
Several differences in respondent group characteristics by practice model were found. Respondents in multistate hospitalist groups were more likely from the South and Midwest, while respondents from multispecialty groups were likely from the West. More multistate group practices were based in smaller hospitals, while academic hospitalists tended to practice in hospitals with 600 or more beds. Respondents employed by hospitals were more likely to practice at 1 hospital facility only, while local group practices were more likely to practice at 3 or more facilities. The median number of physicians in a hospitalist group was 11 (interquartile range [IQR] 6, 19). Local and multistate groups had fewer hospitalists compared to other models. Nonphysician providers were employed by nearly half of all hospitalist practices. Although almost all groups had access to some information technology, more academic hospitalists had access to electronic order entry, electronic physician notes, electronic clinical guidelines resources and communication technology, while local and multistate groups were least likely to have access to these resources.
Work Pattern Variations
Table 2 further details hospitalist work hours by practice model. The majority of hospitalists (78%) reported their position was full‐time (FTE 1.0), while 13% reported working less than full‐time (FTE <1.0). Only 5% of local group hospitalists worked part‐time, while 20% of multispecialty group hospitalists did. An additional 9% reported FTE >1.0, indicating their work hours exceeded the definition of a full‐time physician in their practice. Among full‐time hospitalists, local group members worked a greater number of shifts per month than employees of multispecialty groups, hospitals, and academic medical centers. Academic hospitalists reported higher numbers of consecutive clinical days worked on average, but fewer night shifts compared to hospitalists employed by multistate groups, multispecialty groups, and hospitals; fewer billable encounters than hospitalists in local and multistate groups; and more nonclinical work hours than hospitalists of any other practice model. Academic hospitalists also spent more time on teaching and research than other practice models. Hospitalists spent 11%‐18% of their time on administrative and committee responsibilities, with the least amount spent by hospitalists in multistate groups and the most in academic practice.
Local Hospitalist‐Only Group | Multi‐State Hospitalist Group | Multispecialty Physician Group | Employer Hospital | University or Medical School | ||
---|---|---|---|---|---|---|
n = 95 | n = 111 | n = 115 | n = 348 | n = 107 | P Value | |
| ||||||
FTE, weighted % | 0.058 | |||||
FTE < 1.0 | 6 | 13 | 20 | 12 | 14 | |
FTE = 1.0 | 85 | 75 | 74 | 80 | 82 | |
FTE > 1.0 | 10 | 13 | 6 | 8 | 5 | |
Workload parameters, weighted mean (99% CI) | ||||||
Clinical shifts per month for FTE 1.0 | 19 (17, 20)* | 17 (16, 19) | 15 (14, 17)* | 16 (15, 16) | 15 (13, 17) | <0.001* |
Hours per clinical shift | 10 (9, 11) | 11 (10, 11)* | 10 (10, 11.0) | 11 (10, 11.0) | 10 (9, 10)* | 0.006*, 0.002 |
Consecutive days on clinical shift | 8 (6, 9) | 7 (6, 7)* | 6 (6, 7) | 7 (6, 7) | 9 (7, 10)* | 0.002*, <0.001 |
% Clinical shifts on nights | 20 (15, 25) | 23 (18, 28)* | 23 (17, 29) | 21 (17, 24) | 14 (9, 18)* | 0.001*, 0.002 |
% Night shifts spent in hospital | 61 (49, 74)* | 63 (52, 75) | 72 (62, 83) | 73 (67, 80) | 43 (29, 57)* | 0.010*, 0.003, <0.001 |
Billable encounters per clinical shift | 17 (14, 19)* | 17 (16, 18) | 14 (13, 15) | 15 (14, 16) | 13 (11, 14)* | <0.001*, 0.002 |
Hours nonclinical work per month | 23 (12, 34)* | 19 (11, 27) | 31 (20, 42) | 30 (24, 36) | 71 (55, 86)* | <0.001* |
Hours clinical and nonclinical work per month for FTE 1.0 | 202 (186, 219) | 211 (196, 226) | 184 (170, 198)* | 193 (186, 201) | 221 (203, 238)* | <0.001* |
Professional activity, weighted mean % (99% CI) | ||||||
Clinical | 84 (78, 89)* | 86 (81, 90) | 78 (72, 84) | 79 (76, 82) | 58 (51, 64)* | <0.001* |
Teaching | 2.3 (1, 5)* | 3 (1, 4) | 6 (4, 9) | 6 (5, 8) | 17 (14, 20)* | <0.001* |
Administration and Committee work | 13 (8, 19) | 11 (8, 15)* | 16 (10, 21) | 14 (12, 17) | 19 (14, 24)* | 0.001* |
Research | 0 (0, 0)* | 1 (0, 2) | 0 (0, 1) | 1 (0, 1) | 7 (3, 11)* | <0.001* |
Table 3 tabulates other work pattern characteristics. Most hospitalists indicated that their current clinical work as hospitalists involved the general medical wards (100%), medical consultations (98%), and comanagement with specialists (92%). There were wide differences in participation in comanagement (100%, local groups vs 71%, academic), intensive care unit (ICU) responsibilities (94%, multistate groups vs 27%, academic), and nursing home care (30%, local groups vs 8%, academic). Among activities that are potentially not reimbursable, academic hospitalists were less likely to participate in coordination of patient transfers and code or rapid response teams, while multistate groups were least likely to participate in quality improvement activities. In total, 99% of hospitalists reported participating in at least 1 potentially nonreimbursable clinical activity.
Local Hospitalist‐Only Group | Multi‐State Hospitalist Group | Multispecialty Physician Group | Employer Hospital | University or Medical School | ||
---|---|---|---|---|---|---|
n = 95 | n = 111 | n = 115 | n = 348 | n = 107 | P Value | |
| ||||||
Reimbursable activities, overlapping weighted % | ||||||
General medical ward | 100 | 99 | 100 | 99 | 99 | 0.809 |
Medical consultations | 99 | 99 | 100 | 98 | 95 | 0.043 |
Comanagement with specialists | 100 | 96 | 96 | 93 | 71 | <0.001 |
Preoperative evaluations | 92 | 92 | 90 | 88 | 77 | 0.002 |
Intensive care unit | 86 | 94 | 67 | 75 | 27 | <0.001 |
Skilled nursing facility or long‐term acute care facility | 30 | 19 | 12 | 16 | 8 | <0.001 |
Outpatient general medical practice | 4 | 4 | 5 | 5 | 10 | 0.241 |
Potentially nonreimbursable activities, overlapping weighted % | ||||||
Coordination of patient transfers | 92 | 94 | 95 | 93 | 82 | 0.005 |
Quality improvement or patient safety initiatives | 81 | 78 | 83 | 89 | 89 | 0.029 |
Code team or rapid response team | 56 | 57 | 53 | 62 | 37 | <0.001 |
Information technology design or implementation | 42 | 39 | 47 | 51 | 51 | 0.154 |
Admission triage for emergency department | 49 | 46 | 43 | 40 | 31 | 0.132 |
Compensation scheme, weighted % | <0.001 | |||||
Salary only | 18 | 21 | 30 | 29 | 47 | |
Salary plus performance incentive | 54 | 72 | 59 | 67 | 53 | |
Fee‐for‐service | 20 | 1 | 7 | 2 | 0 | |
Capitation | 0 | 0 | 0 | 0 | 0 | |
Other | 9 | 7 | 4 | 3 | 0 | |
Compensation links to incentives, overlapping weighted % | ||||||
No incentives | 40 | 28 | 29 | 29 | 48 | 0.003 |
Patient satisfaction | 23 | 39 | 38 | 38 | 14 | <0.001 |
Length of stay | 18 | 17 | 20 | 13 | 10 | 0.208 |
Overall cost | 8 | 11 | 9 | 5 | 6 | 0.270 |
Test utilization | 2 | 2 | 7 | 1 | 0 | <0.001 |
Clinical processes and outcomes | 26 | 34 | 44 | 43 | 24 | <0.001 |
Other | 17 | 29 | 26 | 31 | 25 | 0.087 |
Earnings, weighted mean dollars (99% CI) | 226,065 (202,891, 249,240)* | 225,613 (210,772, 240,454) | 202,617 (186,036, 219,198) | 206,087 (198,413, 213,460) | 166,478 (151,135, 181,821)* | <0.001* |
Hospitalist compensation schemes were significantly different across the practice models. Salary‐only schemes were most common among academic hospitalists (47%), while 72% of multistate groups used performance incentives in addition to salary. More local groups used fee‐for‐service compensation than other models. Incentives differed by practice model, with more multistate groups having incentives based on patient satisfaction, while more multispecialty physician groups had incentives based on clinical processes and outcomes than other models. Finally, mean earnings for academic hospitalists were significantly lower than for hospitalists of other practice models. Local and multistate group hospitalists earned more than any other practice model (all P <0.001), and $60,000 more than the lowest compensated academic hospitalists.
Components of Job Satisfaction
Hospitalists' rankings of the most important factors for job satisfaction revealed differences across models (Figure 2). Overall, hospitalists were most likely to consider optimal workload and compensation as important factors for job satisfaction from a list of 13 considerations. Local groups and academics were least likely to rank optimal workload as a top factor, and local group hospitalists were more likely to rank optimal autonomy than those of other models. Academic hospitalists had less concern for substantial pay, and more concern for the variety of tasks they perform and recognition by leaders, than other hospitalists.
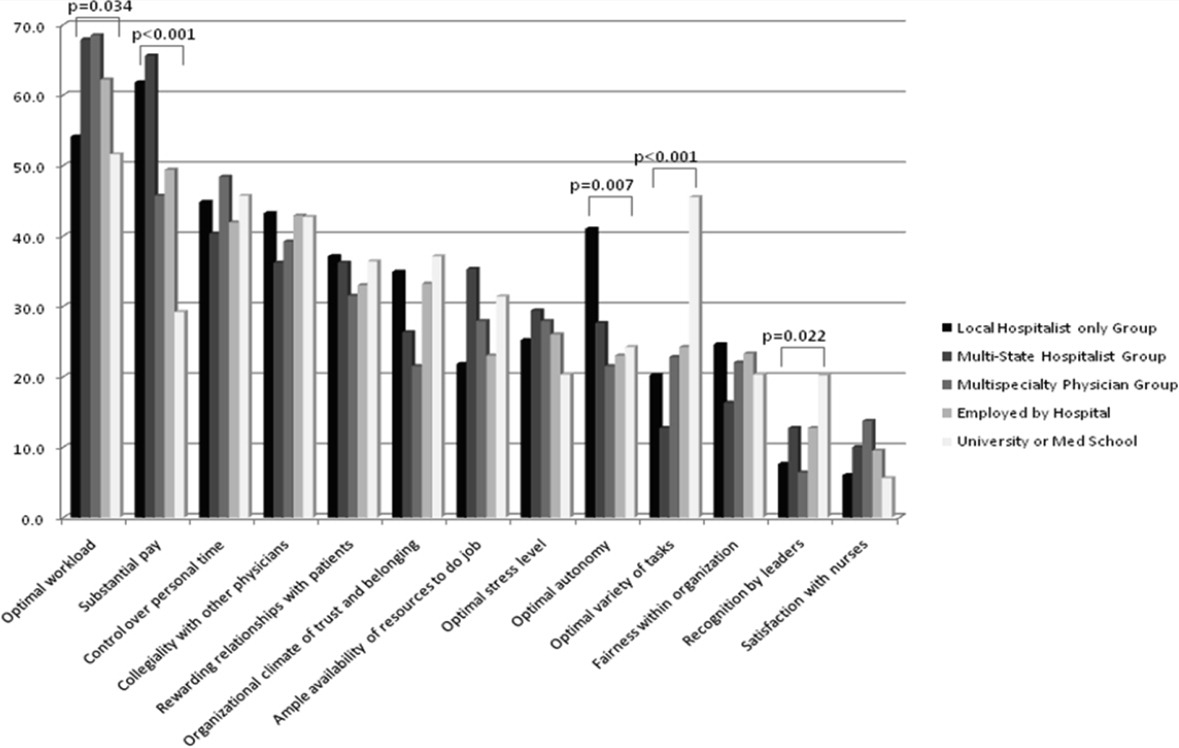
Job Satisfaction and Burnout Risk
Differences in the ratings of 4 of the 11 satisfaction and job characteristic domains were found across the practice models (Figure 3). Multispecialty group hospitalists were less satisfied with autonomy and their relationship with patients than other practice models, and along with multistate groups, reported the highest perceived workload. Organizational fairness was rated much higher by local group hospitalists than other practice models. Despite these differences in work patterns and satisfaction, there were no differences found in level of global job satisfaction, specialty satisfaction, or burnout across the practice models. Overall, 62% of respondents reported high job satisfaction (4 on a 1 to 5 scale), and 30% indicated burnout symptoms.
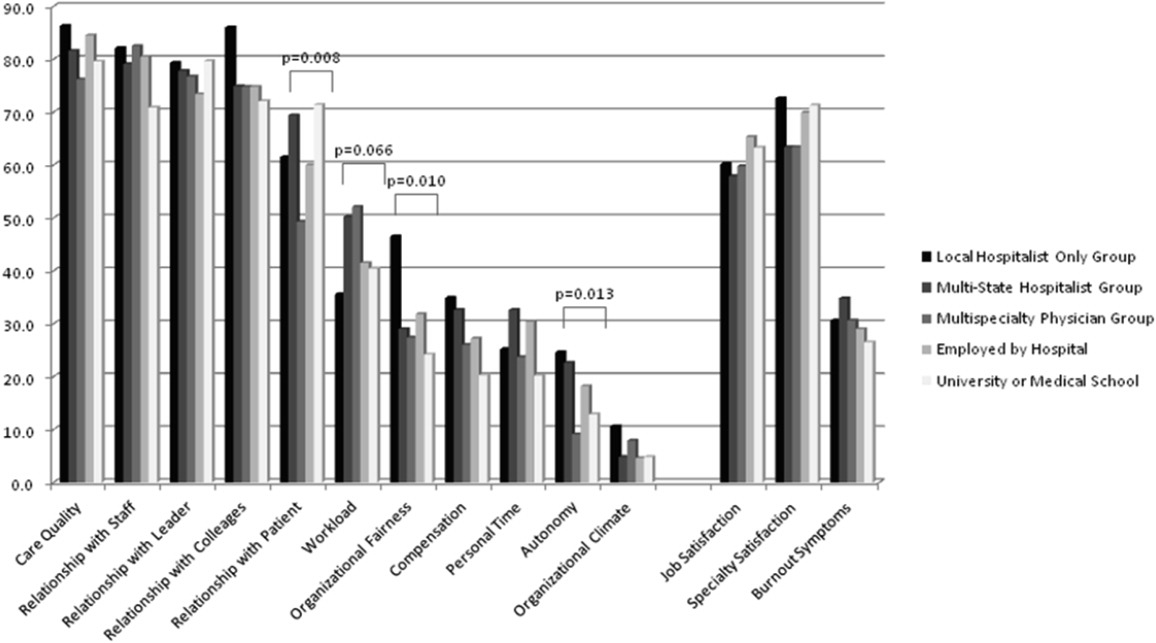
DISCUSSION
In our sample of US hospitalists, we found major differences in work patterns and compensation across hospitalist practice models, but no differences in job satisfaction, specialty satisfaction, and burnout. In particular, differences across these models included variations in hospitalist workload, hours, pay, and distribution of work activities. We found that hospitalists perform a variety of clinical and nonclinical tasks, for many of which there are not standard reimbursement mechanisms. We also found that features of a job that individual hospitalists considered most important vary by practice model.
Previous analysis of this data explored the overall state of hospitalist satisfaction.16 The present analysis offers a glimpse into hospitalists' systems‐orientation through a deeper look at their work patterns. The growth in the number of hospitalists who participate in intensive care medicine, specialty comanagement, and other work that involves close working relationships with specialist physicians confirms collaborative care as one of the dominant drivers of the hospitalist movement. At the level of indirect patient care, nearly all hospitalists contributed to work that facilitates coordination, quality, patient safety, or information technology. Understanding the integrative value of hospitalists outside of their clinical productivity may be of interest to hospital administrators.
Global satisfaction measures were similar across practice models. This finding is particularly interesting given the major differences in job characteristics seen among the practice models. This similarity in global satisfaction despite real differences in the nature of the job suggests that individuals find settings that allow them to address their individual professional goals. Our study demonstrates that, in 2010, Hospital Medicine has evolved enough to accommodate a wide variety of goals and needs.
While global satisfaction did not differ among practice types, hospitalists from various models did report differences in factors considered important to global satisfaction. While workload and pay were rated as influential across most models, the degree of importance was significantly different. In academic settings, substantial pay was not a top consideration for overall job satisfaction, whereas in local and multistate hospitalist groups, pay was a very close second in importance to optimal workload. These results may prove helpful for individual hospitalists trying to find their optimal job. For example, someone who is less concerned about workload, but wants to be paid well and have a high degree of autonomy, may find satisfaction in local hospitalist groups. However, for someone who is willing to sacrifice a higher salary for variety of activities, academic Hospital Medicine may be a better fit.
There is a concerning aspect of hospitalist job satisfaction that different practice models do not seem to solve. Control over personal time is a top consideration for many hospitalists across practice models, yet their satisfaction with personal time is low. As control over personal time is seen as a draw to the Hospital Medicine specialty, group leaders may need to evaluate their programs to ensure that schedules and workload support efforts for hospitalists to balance work and homelife commitments.
There are additional findings that are important for Hospital Medicine group leaders. Regardless of practice model, compensation and workload are often used as tools to recruit and retain hospitalists. While these tools may be effective, leaders may find more nuanced approaches to improving their hospitalists' overall satisfaction. Leaders of local hospitalist groups may find their hospitalists tolerant of heavier workloads as long as they are adequately rewarded and are given real autonomy over their work. However, leaders of academic programs may be missing the primary factor that can improve their hospitalists' satisfaction. Rather than asking for higher salaries to remain competitive, it may be more effective to advocate for time and training for their hospitalists to pursue important other activities beyond direct clinical care. Given that resources will always be limited, group leaders need to understand all of the elements that can contribute to hospitalist job satisfaction.
We point out several limitations to this study. First, our adjusted response rate of 25.6% is low for survey research, in general. As mentioned above, hospitalists are not easily identified in any available national physician database. Therefore, we deliberately designed our sampling strategy to error on the side of including ineligible surveyees to reduce systematic exclusion of practicing hospitalists. Using simple post hoc methods, we identified many nonhospitalists and bad addresses from our sample, but because these methods were exclusionary as opposed to confirmatory, we believe that a significant proportion of remaining nonrespondents may also have been ineligible for the survey. Although this does not fully address concerns about potential response bias, we believe that our sample representing a large number of hospitalist groups is adequate to make estimations about a nationally representative sample of practicing hospitalists. Second, in spite of our inclusive approach, we may still have excluded categories of practicing hospitalists. We were careful not to allow SHM members to represent all US hospitalists and included non‐members in the sampling frame, but the possibility of systematic exclusion that may alter our results remains a concern. Additionally, one of our goals was to characterize pediatric hospitalists independently from their adult‐patient counterparts. Despite oversampling of pediatricians, their sample was too small for a more detailed comparison across practice models. Also, self‐reported data about workload and compensation are subject to inaccuracies related to recall and cognitive biases. Last, this is a cross‐sectional study of hospitalist satisfaction at one point in time. Consequently, our sample may not be representative of very dissatisfied hospitalists who have already left their jobs.
The diversity found across existing practice models and the characteristics of the practices provide physicians with the opportunity to bring their unique skills and motivations to the hospitalist movement. As hospitals and other organizations seek to create, maintain, or grow hospitalist programs, the data provided here may prove useful to understand the relationship between practice characteristics and individual job satisfaction. Additionally, hospitalists looking for a job can consider these results as additional information to guide their choice of practice model and work patterns.
Acknowledgements
The authors thank Kenneth A. Rasinski for assistance with survey items refinement, and members of the SHM Career Satisfaction Task Force for their assistance in survey development.
Over the past 15 years, there has been dramatic growth in the number of hospitalist physicians in the United States and in the number of hospitals served by them.13 Hospitals are motivated to hire experienced hospitalists to staff their inpatient services,4 with goals that include obtaining cost‐savings and higher quality.59 The rapid growth of Hospital Medicine saw multiple types of hospital practice models emerge with differing job characteristics, clinical duties, workload, and compensation schemes.10 The extent of the variability of hospitalist jobs across practice models is not known.
Intensifying recruitment efforts and the concomitant increase in compensation for hospitalists over the last decade suggest that demand for hospitalists is strong and sustained.11 As a result, today's cohort of hospitalists has a wide range of choices of types of jobs, practice models, and locations. The diversity of available hospitalist jobs is characterized, for example, by setting (community hospital vs academic hospital), employer (hospital vs private practice), job duties (the amount and type of clinical work, and other administrative, teaching, or research duties), and intensity (work hours and duties to maximize income or lifestyle). How these choices relate to job satisfaction and burnout are also unknown.
The Society of Hospital Medicine (SHM) has administered surveys to hospitalist group leaders biennially since 2003.1215 These surveys, however, do not address issues related to individual hospitalist worklife, recruitment, and retention. In 2005, SHM convened a Career Satisfaction Task Force that designed and executed a national survey of hospitalists in 2009‐2010. The objective of this study is to evaluate how job characteristics vary by practice model, and the association of these characteristics and practice models with job satisfaction and burnout.
METHODS
Survey Instrument
A detailed description of the survey design, sampling strategy, data collection, and response rate calculations is described elsewhere.16 Portions of the 118‐item survey instrument assessed characteristics of the respondents' hospitalist group (12 items), details about their individual work patterns (12 items), and demographics (9 items). Work patterns were evaluated by the average number of clinical work days, consecutive days, hours per month, percentage of work assigned to night duty, and number of patient encounters. Average hours spent on nonclinical work, and the percentage of time allocated for clinical, administrative, teaching, and research activities were solicited. Additional items assessed specific clinical responsibilities, pretax earnings in FY2010, the availability of information technology capabilities, and the adequacy of available resources. Job and specialty satisfaction and 11 satisfaction domain measures were measured using validated scales.1726 Burnout symptoms were measured using a validated single‐item measure.26, 27
Sampling Strategy
We surveyed a national stratified sample of hospitalists in the US and Puerto Rico. We used the largest database of hospitalists (>24,000 names) currently available and maintained by the SHM as our sampling frame. We linked hospitalist employer information to hospital statistics from the American Hospital Association database28 to stratify the sample by number of hospital beds, geographic region, employment model, and specialty training, oversampling pediatric hospitalists due to small numbers. A respondent sample of about 700 hospitalists was calculated to be adequate to detect a 0.5 point difference in job satisfaction scores between subgroups assuming 90% power and alpha of 0.05. However, we sampled a total of 5389 addresses from the database to overcome the traditionally low physician response rates, duplicate sampling, bad addresses, and non‐hospitalists being included in the sampling frame. In addition, 2 multistate hospitalist companies (EmCare, In Compass Health) and 1 for‐profit hospital chain (HCA, Inc) financially sponsored this project with the stipulation that all of their hospitalist employees (n = 884) would be surveyed.
Data Collection
The healthcare consulting firm, Press Ganey, provided support with survey layout and administration following the modified Dillman method.29 Three rounds of coded surveys and solicitation letters from the investigators were mailed 2 weeks apart in November and December 2009. Because of low response rates to the mailed survey, an online survey was created using Survey Monkey and sent to 650 surveyees for whom e‐mail addresses were available, and administered at a kiosk for sample physicians during the SHM 2010 annual meeting.
Data Analysis
Nonresponse bias was measured by comparing characteristics between respondents of separate survey waves.30 We determined the validity of mailing addresses immediately following the survey period by mapping each address using Google, and if the address was a hospital, researching online whether or not the intended recipient was currently employed there. Practice characteristics were compared across 5 model categories distilled from the SHM & Medical Group Management Association survey: local hospitalist‐only group, multistate hospitalist group, multispecialty physician group, employer hospital, and university or medical school. Weighted proportions, means, and medians were calculated to account for oversampling of pediatric hospitalists. Differences in categorical measures were assessed using the chi‐square test and the design‐based F test for comparing weighted data. Weighted means (99% confidence intervals) and medians (interquartile ranges) were calculated. Because each parameter yielded a single outlier value across the 5 practice models, differences across weighted means were assessed using generalized linear models with the single outlier value chosen as the reference mean. Pair‐wise Wilcoxon rank sum test was used to compare median values. In these 4‐way comparisons of means and medians, significance was defined as P value of 0.0125 per Bonferroni correction. A single survey item solicited respondents to choose exactly 4 of 13 considerations most pertinent to job satisfaction. The proportion of respondents who scored 4 on a 5‐point Likert scale of the 11 satisfaction domains and 2 global measures of satisfaction, and burnout symptoms defined as 3 on a 5‐point single item measure were bar‐graphed. Chi‐square statistics were used to evaluate for differences across practice models. Statistical significance was defined by alpha less than 0.05, unless otherwise specified. All analyses were performed using STATA version 11.0 (College Station, TX). This study was approved by the Loyola University Institutional Review Board.
Survey data required cleaning prior to analysis. Missing gender information was imputed using the respondents' name. Responses to the item that asked to indicate the proportion of work dedicated to administrative responsibilities, clinical care, teaching, and research that did not add up to 100% were dropped. Two responses that indicated full‐time equivalent (FTE) of 0%, but whose respondents otherwise completed the survey implying they worked as clinical hospitalists, were replaced with values calculated from the given number of work hours relative to the median work hours in our sample. Out of range or implausible responses to the following items were dropped from analyses: the average number of billable encounters during a typical day or shift, number of shifts performing clinical activities during a typical month, pretax earnings, the year the respondent completed residency training, and the number of whole years practiced as a hospitalist. The proportion of selective item nonresponse was small and we did not, otherwise, impute missing data.
RESULTS
Response Rate
Of the 5389 originally sampled addresses, 1868 were undeliverable. Addresses were further excluded if they appeared in duplicate or were outdated. This yielded a total of 3105 eligible surveyees in the sample. As illustrated in Figure 1, 841 responded to the mailed survey and 5 responded to the Web‐based survey. After rejecting 67 non‐hospitalist respondents and 3 duplicate surveys, a total of 776 surveys were included in the final analysis. The adjusted response rate was 25.6% (776/3035). Members of SHM were more likely to return the survey than nonmembers. The adjusted response rate from hospitalists affiliated with the 3 sponsoring institutions was 6% (40/662). Because these respondents were more likely to be non‐members of SHM, we opted to analyze the responses from the sponsor hospitalists together with the sampled hospitalists. The demographics of the resulting pool of 816 respondents affiliated with over 650 unique hospitalist groups were representative of the original survey frame. We analyzed data from 794 of these who responded to the item indicating their hospitalist practice model. Demographic characteristics of responders and nonresponders to the practice model survey item were similar.
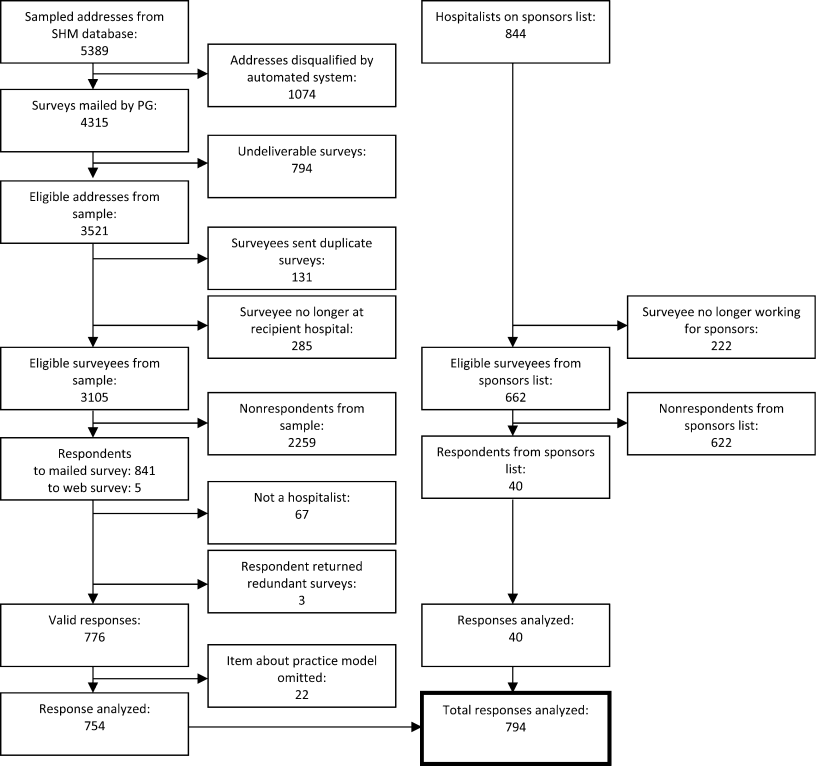
Characteristics of Hospitalists and Their Groups
Table 1 summarizes the characteristics of hospitalist respondents and their organizations by practice model. More (44%) respondents identified their practice model as directly employed by the hospital than other models, including multispecialty physician group (15%), multistate hospitalist group (14%), university or medical school (14%), local hospitalist group (12%), and other (2%). The median age of hospitalist respondents was 42 years, with 6.8 years of mean experience as a hospitalist. One third were women, 84% were married, and 46% had dependent children 6 years old or younger at home. Notably, hospitalists in multistate groups had fewer years of experience, and fewer hospitalists in local and multistate groups were married compared to hospitalists in other practice models.
Local Hospitalist‐Only Group | Multi‐State Hospitalist Group | Multispecialty Physician Group | Employer Hospital | University or Medical School | ||
---|---|---|---|---|---|---|
n = 95 | n = 111 | n = 115 | n = 348 | n = 107 | P Value | |
| ||||||
Hospitalist characteristics | ||||||
Age, weighted mean (99% CI) | 45 (42, 48) | 44 (42, 47) | 45 (43, 47) | 45 (43, 46) | 43 (40, 46) | |
Years hospitalist experience, weighted mean (99% CI) | 8 (6, 9)* | 5 (4, 6)* | 8 (7, 9) | 7 (6, 7) | 8 (6, 9) | <0.010* |
Women, weighted % | 29 | 30 | 39 | 31 | 43 | 0.118 |
Married, weighted % | 76 | 77 | 82 | 89 | 81 | 0.009 |
At least 1 dependent child younger than age 6 living in home, weighted % | 47 | 48 | 43 | 47 | 45 | 0.905 |
Pediatric specialty, n (%) | <10 | <10 | 11 (10%) | 57 (16%) | 36 (34%) | <0.001 |
Hospitalist group characteristics | ||||||
Region, weighted % | <0.001 | |||||
Northeast (AHA 1 & 2) | 13 | 10 | 16 | 27 | 13 | |
South (AHA 3 & 4) | 19 | 37 | 13 | 24 | 21 | |
Midwest (AHA 5 & 6) | 23 | 24 | 25 | 22 | 26 | |
Mountain (AHA 7 & 8) | 22 | 20 | 16 | 13 | 24 | |
West (AHA 9) | 24 | 10 | 31 | 14 | 16 | |
No. beds of primary hospital, weighted % | <0.001 | |||||
Up to 149 | 17 | 26 | 12 | 24 | 14 | |
150299 | 30 | 36 | 36 | 33 | 21 | |
300449 | 26 | 24 | 29 | 20 | 19 | |
450599 | 13 | 8 | 17 | 11 | 21 | |
600 or more | 12 | 6 | 7 | 13 | 24 | |
No. of hospital facilities served by current practice, weighted % | <0.001 | |||||
1 | 53 | 70 | 67 | 77 | 66 | |
2 | 20 | 22 | 20 | 16 | 24 | |
3 or more | 27 | 9 | 13 | 7 | 10 | |
No. of physicians in current practice, median (IQR) | 10 (5, 18) | 8 (6, 12)* | 14 (8, 25)* | 12 (6, 18) | 12 (7, 20) | <0.001*, 0.001 |
No. of non‐physician providers in current practice, median (IQR) | 0 (0, 2) | 0 (0, 2) | 0 (0, 3) | 1 (0, 2) | 0 (0, 2) | |
Available information technology capabilities, weighted % | ||||||
EHR to access physician notes | 57 | 57 | 75 | 58 | 79 | <0.001 |
EHR to access nursing documentations | 68 | 67 | 74 | 75 | 76 | 0.357 |
EHR to access laboratory or test results | 97 | 89 | 95 | 96 | 96 | 0.054 |
Electronic order entry | 30 | 19 | 53 | 38 | 56 | <0.001 |
Electronic billing | 38 | 31 | 36 | 36 | 38 | 0.818 |
Access to EHR at home or off site | 78 | 73 | 78 | 82 | 84 | 0.235 |
Access to Up‐to‐Date or other clinical guideline resources | 80 | 77 | 91 | 92 | 96 | <0.001 |
Access to schedules, calendars, or other organizational resources | 56 | 57 | 66 | 67 | 75 | 0.024 |
E‐mail, Web‐based paging, or other communication resources | 74 | 63 | 88 | 89 | 90 | <0.001 |
Several differences in respondent group characteristics by practice model were found. Respondents in multistate hospitalist groups were more likely from the South and Midwest, while respondents from multispecialty groups were likely from the West. More multistate group practices were based in smaller hospitals, while academic hospitalists tended to practice in hospitals with 600 or more beds. Respondents employed by hospitals were more likely to practice at 1 hospital facility only, while local group practices were more likely to practice at 3 or more facilities. The median number of physicians in a hospitalist group was 11 (interquartile range [IQR] 6, 19). Local and multistate groups had fewer hospitalists compared to other models. Nonphysician providers were employed by nearly half of all hospitalist practices. Although almost all groups had access to some information technology, more academic hospitalists had access to electronic order entry, electronic physician notes, electronic clinical guidelines resources and communication technology, while local and multistate groups were least likely to have access to these resources.
Work Pattern Variations
Table 2 further details hospitalist work hours by practice model. The majority of hospitalists (78%) reported their position was full‐time (FTE 1.0), while 13% reported working less than full‐time (FTE <1.0). Only 5% of local group hospitalists worked part‐time, while 20% of multispecialty group hospitalists did. An additional 9% reported FTE >1.0, indicating their work hours exceeded the definition of a full‐time physician in their practice. Among full‐time hospitalists, local group members worked a greater number of shifts per month than employees of multispecialty groups, hospitals, and academic medical centers. Academic hospitalists reported higher numbers of consecutive clinical days worked on average, but fewer night shifts compared to hospitalists employed by multistate groups, multispecialty groups, and hospitals; fewer billable encounters than hospitalists in local and multistate groups; and more nonclinical work hours than hospitalists of any other practice model. Academic hospitalists also spent more time on teaching and research than other practice models. Hospitalists spent 11%‐18% of their time on administrative and committee responsibilities, with the least amount spent by hospitalists in multistate groups and the most in academic practice.
Local Hospitalist‐Only Group | Multi‐State Hospitalist Group | Multispecialty Physician Group | Employer Hospital | University or Medical School | ||
---|---|---|---|---|---|---|
n = 95 | n = 111 | n = 115 | n = 348 | n = 107 | P Value | |
| ||||||
FTE, weighted % | 0.058 | |||||
FTE < 1.0 | 6 | 13 | 20 | 12 | 14 | |
FTE = 1.0 | 85 | 75 | 74 | 80 | 82 | |
FTE > 1.0 | 10 | 13 | 6 | 8 | 5 | |
Workload parameters, weighted mean (99% CI) | ||||||
Clinical shifts per month for FTE 1.0 | 19 (17, 20)* | 17 (16, 19) | 15 (14, 17)* | 16 (15, 16) | 15 (13, 17) | <0.001* |
Hours per clinical shift | 10 (9, 11) | 11 (10, 11)* | 10 (10, 11.0) | 11 (10, 11.0) | 10 (9, 10)* | 0.006*, 0.002 |
Consecutive days on clinical shift | 8 (6, 9) | 7 (6, 7)* | 6 (6, 7) | 7 (6, 7) | 9 (7, 10)* | 0.002*, <0.001 |
% Clinical shifts on nights | 20 (15, 25) | 23 (18, 28)* | 23 (17, 29) | 21 (17, 24) | 14 (9, 18)* | 0.001*, 0.002 |
% Night shifts spent in hospital | 61 (49, 74)* | 63 (52, 75) | 72 (62, 83) | 73 (67, 80) | 43 (29, 57)* | 0.010*, 0.003, <0.001 |
Billable encounters per clinical shift | 17 (14, 19)* | 17 (16, 18) | 14 (13, 15) | 15 (14, 16) | 13 (11, 14)* | <0.001*, 0.002 |
Hours nonclinical work per month | 23 (12, 34)* | 19 (11, 27) | 31 (20, 42) | 30 (24, 36) | 71 (55, 86)* | <0.001* |
Hours clinical and nonclinical work per month for FTE 1.0 | 202 (186, 219) | 211 (196, 226) | 184 (170, 198)* | 193 (186, 201) | 221 (203, 238)* | <0.001* |
Professional activity, weighted mean % (99% CI) | ||||||
Clinical | 84 (78, 89)* | 86 (81, 90) | 78 (72, 84) | 79 (76, 82) | 58 (51, 64)* | <0.001* |
Teaching | 2.3 (1, 5)* | 3 (1, 4) | 6 (4, 9) | 6 (5, 8) | 17 (14, 20)* | <0.001* |
Administration and Committee work | 13 (8, 19) | 11 (8, 15)* | 16 (10, 21) | 14 (12, 17) | 19 (14, 24)* | 0.001* |
Research | 0 (0, 0)* | 1 (0, 2) | 0 (0, 1) | 1 (0, 1) | 7 (3, 11)* | <0.001* |
Table 3 tabulates other work pattern characteristics. Most hospitalists indicated that their current clinical work as hospitalists involved the general medical wards (100%), medical consultations (98%), and comanagement with specialists (92%). There were wide differences in participation in comanagement (100%, local groups vs 71%, academic), intensive care unit (ICU) responsibilities (94%, multistate groups vs 27%, academic), and nursing home care (30%, local groups vs 8%, academic). Among activities that are potentially not reimbursable, academic hospitalists were less likely to participate in coordination of patient transfers and code or rapid response teams, while multistate groups were least likely to participate in quality improvement activities. In total, 99% of hospitalists reported participating in at least 1 potentially nonreimbursable clinical activity.
Local Hospitalist‐Only Group | Multi‐State Hospitalist Group | Multispecialty Physician Group | Employer Hospital | University or Medical School | ||
---|---|---|---|---|---|---|
n = 95 | n = 111 | n = 115 | n = 348 | n = 107 | P Value | |
| ||||||
Reimbursable activities, overlapping weighted % | ||||||
General medical ward | 100 | 99 | 100 | 99 | 99 | 0.809 |
Medical consultations | 99 | 99 | 100 | 98 | 95 | 0.043 |
Comanagement with specialists | 100 | 96 | 96 | 93 | 71 | <0.001 |
Preoperative evaluations | 92 | 92 | 90 | 88 | 77 | 0.002 |
Intensive care unit | 86 | 94 | 67 | 75 | 27 | <0.001 |
Skilled nursing facility or long‐term acute care facility | 30 | 19 | 12 | 16 | 8 | <0.001 |
Outpatient general medical practice | 4 | 4 | 5 | 5 | 10 | 0.241 |
Potentially nonreimbursable activities, overlapping weighted % | ||||||
Coordination of patient transfers | 92 | 94 | 95 | 93 | 82 | 0.005 |
Quality improvement or patient safety initiatives | 81 | 78 | 83 | 89 | 89 | 0.029 |
Code team or rapid response team | 56 | 57 | 53 | 62 | 37 | <0.001 |
Information technology design or implementation | 42 | 39 | 47 | 51 | 51 | 0.154 |
Admission triage for emergency department | 49 | 46 | 43 | 40 | 31 | 0.132 |
Compensation scheme, weighted % | <0.001 | |||||
Salary only | 18 | 21 | 30 | 29 | 47 | |
Salary plus performance incentive | 54 | 72 | 59 | 67 | 53 | |
Fee‐for‐service | 20 | 1 | 7 | 2 | 0 | |
Capitation | 0 | 0 | 0 | 0 | 0 | |
Other | 9 | 7 | 4 | 3 | 0 | |
Compensation links to incentives, overlapping weighted % | ||||||
No incentives | 40 | 28 | 29 | 29 | 48 | 0.003 |
Patient satisfaction | 23 | 39 | 38 | 38 | 14 | <0.001 |
Length of stay | 18 | 17 | 20 | 13 | 10 | 0.208 |
Overall cost | 8 | 11 | 9 | 5 | 6 | 0.270 |
Test utilization | 2 | 2 | 7 | 1 | 0 | <0.001 |
Clinical processes and outcomes | 26 | 34 | 44 | 43 | 24 | <0.001 |
Other | 17 | 29 | 26 | 31 | 25 | 0.087 |
Earnings, weighted mean dollars (99% CI) | 226,065 (202,891, 249,240)* | 225,613 (210,772, 240,454) | 202,617 (186,036, 219,198) | 206,087 (198,413, 213,460) | 166,478 (151,135, 181,821)* | <0.001* |
Hospitalist compensation schemes were significantly different across the practice models. Salary‐only schemes were most common among academic hospitalists (47%), while 72% of multistate groups used performance incentives in addition to salary. More local groups used fee‐for‐service compensation than other models. Incentives differed by practice model, with more multistate groups having incentives based on patient satisfaction, while more multispecialty physician groups had incentives based on clinical processes and outcomes than other models. Finally, mean earnings for academic hospitalists were significantly lower than for hospitalists of other practice models. Local and multistate group hospitalists earned more than any other practice model (all P <0.001), and $60,000 more than the lowest compensated academic hospitalists.
Components of Job Satisfaction
Hospitalists' rankings of the most important factors for job satisfaction revealed differences across models (Figure 2). Overall, hospitalists were most likely to consider optimal workload and compensation as important factors for job satisfaction from a list of 13 considerations. Local groups and academics were least likely to rank optimal workload as a top factor, and local group hospitalists were more likely to rank optimal autonomy than those of other models. Academic hospitalists had less concern for substantial pay, and more concern for the variety of tasks they perform and recognition by leaders, than other hospitalists.
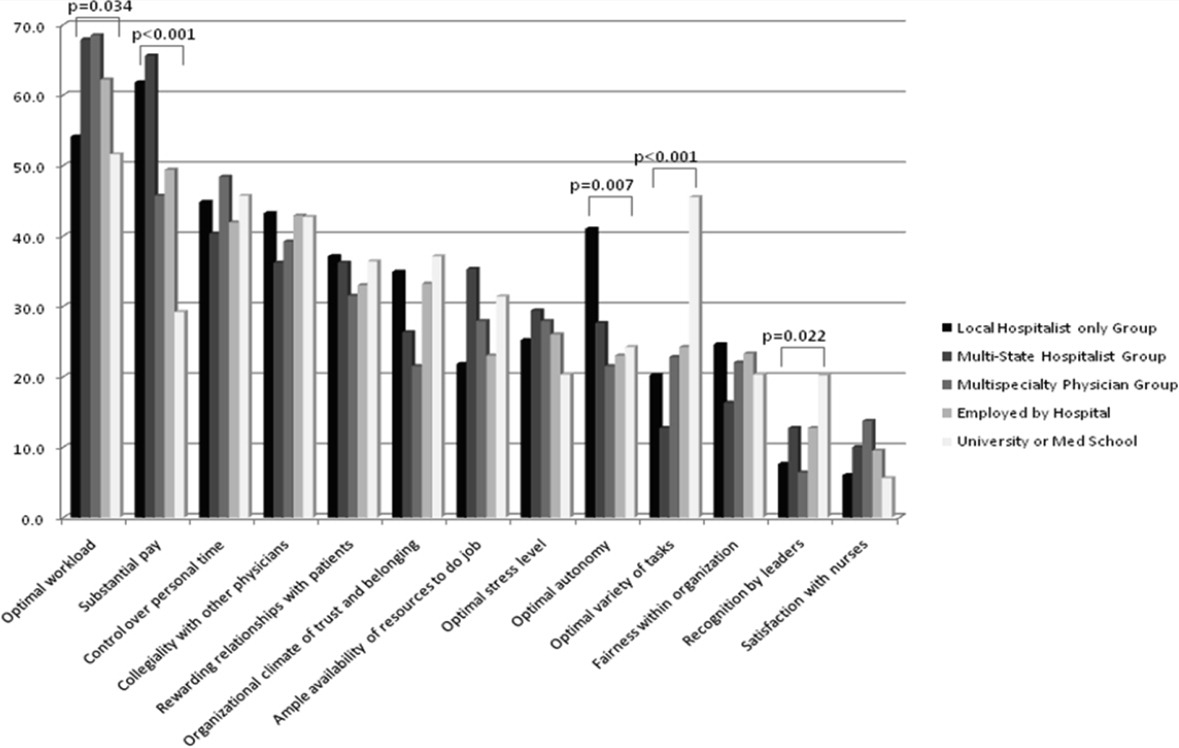
Job Satisfaction and Burnout Risk
Differences in the ratings of 4 of the 11 satisfaction and job characteristic domains were found across the practice models (Figure 3). Multispecialty group hospitalists were less satisfied with autonomy and their relationship with patients than other practice models, and along with multistate groups, reported the highest perceived workload. Organizational fairness was rated much higher by local group hospitalists than other practice models. Despite these differences in work patterns and satisfaction, there were no differences found in level of global job satisfaction, specialty satisfaction, or burnout across the practice models. Overall, 62% of respondents reported high job satisfaction (4 on a 1 to 5 scale), and 30% indicated burnout symptoms.
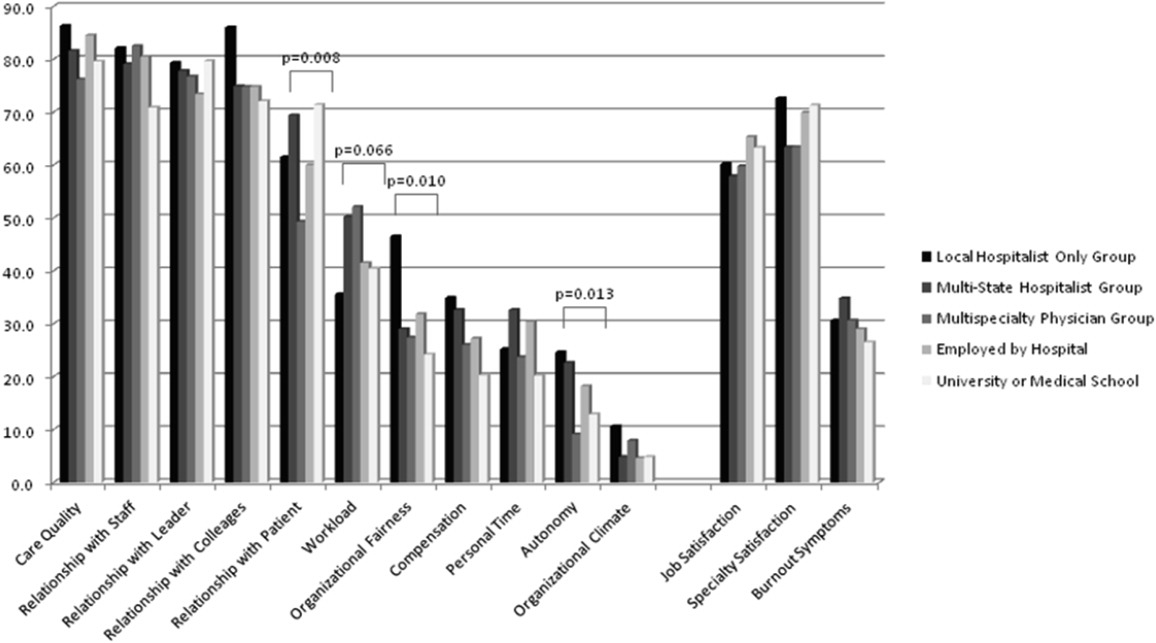
DISCUSSION
In our sample of US hospitalists, we found major differences in work patterns and compensation across hospitalist practice models, but no differences in job satisfaction, specialty satisfaction, and burnout. In particular, differences across these models included variations in hospitalist workload, hours, pay, and distribution of work activities. We found that hospitalists perform a variety of clinical and nonclinical tasks, for many of which there are not standard reimbursement mechanisms. We also found that features of a job that individual hospitalists considered most important vary by practice model.
Previous analysis of this data explored the overall state of hospitalist satisfaction.16 The present analysis offers a glimpse into hospitalists' systems‐orientation through a deeper look at their work patterns. The growth in the number of hospitalists who participate in intensive care medicine, specialty comanagement, and other work that involves close working relationships with specialist physicians confirms collaborative care as one of the dominant drivers of the hospitalist movement. At the level of indirect patient care, nearly all hospitalists contributed to work that facilitates coordination, quality, patient safety, or information technology. Understanding the integrative value of hospitalists outside of their clinical productivity may be of interest to hospital administrators.
Global satisfaction measures were similar across practice models. This finding is particularly interesting given the major differences in job characteristics seen among the practice models. This similarity in global satisfaction despite real differences in the nature of the job suggests that individuals find settings that allow them to address their individual professional goals. Our study demonstrates that, in 2010, Hospital Medicine has evolved enough to accommodate a wide variety of goals and needs.
While global satisfaction did not differ among practice types, hospitalists from various models did report differences in factors considered important to global satisfaction. While workload and pay were rated as influential across most models, the degree of importance was significantly different. In academic settings, substantial pay was not a top consideration for overall job satisfaction, whereas in local and multistate hospitalist groups, pay was a very close second in importance to optimal workload. These results may prove helpful for individual hospitalists trying to find their optimal job. For example, someone who is less concerned about workload, but wants to be paid well and have a high degree of autonomy, may find satisfaction in local hospitalist groups. However, for someone who is willing to sacrifice a higher salary for variety of activities, academic Hospital Medicine may be a better fit.
There is a concerning aspect of hospitalist job satisfaction that different practice models do not seem to solve. Control over personal time is a top consideration for many hospitalists across practice models, yet their satisfaction with personal time is low. As control over personal time is seen as a draw to the Hospital Medicine specialty, group leaders may need to evaluate their programs to ensure that schedules and workload support efforts for hospitalists to balance work and homelife commitments.
There are additional findings that are important for Hospital Medicine group leaders. Regardless of practice model, compensation and workload are often used as tools to recruit and retain hospitalists. While these tools may be effective, leaders may find more nuanced approaches to improving their hospitalists' overall satisfaction. Leaders of local hospitalist groups may find their hospitalists tolerant of heavier workloads as long as they are adequately rewarded and are given real autonomy over their work. However, leaders of academic programs may be missing the primary factor that can improve their hospitalists' satisfaction. Rather than asking for higher salaries to remain competitive, it may be more effective to advocate for time and training for their hospitalists to pursue important other activities beyond direct clinical care. Given that resources will always be limited, group leaders need to understand all of the elements that can contribute to hospitalist job satisfaction.
We point out several limitations to this study. First, our adjusted response rate of 25.6% is low for survey research, in general. As mentioned above, hospitalists are not easily identified in any available national physician database. Therefore, we deliberately designed our sampling strategy to error on the side of including ineligible surveyees to reduce systematic exclusion of practicing hospitalists. Using simple post hoc methods, we identified many nonhospitalists and bad addresses from our sample, but because these methods were exclusionary as opposed to confirmatory, we believe that a significant proportion of remaining nonrespondents may also have been ineligible for the survey. Although this does not fully address concerns about potential response bias, we believe that our sample representing a large number of hospitalist groups is adequate to make estimations about a nationally representative sample of practicing hospitalists. Second, in spite of our inclusive approach, we may still have excluded categories of practicing hospitalists. We were careful not to allow SHM members to represent all US hospitalists and included non‐members in the sampling frame, but the possibility of systematic exclusion that may alter our results remains a concern. Additionally, one of our goals was to characterize pediatric hospitalists independently from their adult‐patient counterparts. Despite oversampling of pediatricians, their sample was too small for a more detailed comparison across practice models. Also, self‐reported data about workload and compensation are subject to inaccuracies related to recall and cognitive biases. Last, this is a cross‐sectional study of hospitalist satisfaction at one point in time. Consequently, our sample may not be representative of very dissatisfied hospitalists who have already left their jobs.
The diversity found across existing practice models and the characteristics of the practices provide physicians with the opportunity to bring their unique skills and motivations to the hospitalist movement. As hospitals and other organizations seek to create, maintain, or grow hospitalist programs, the data provided here may prove useful to understand the relationship between practice characteristics and individual job satisfaction. Additionally, hospitalists looking for a job can consider these results as additional information to guide their choice of practice model and work patterns.
Acknowledgements
The authors thank Kenneth A. Rasinski for assistance with survey items refinement, and members of the SHM Career Satisfaction Task Force for their assistance in survey development.
- The status of hospital medicine groups in the United States.J Hosp Med.2006;1(2):75–80. , , , .
- Growth in the care of older patients by hospitalists in the United States.N Engl J Med.2009;360(11):1102–1112. , , , .
- The state of hospital medicine in 2008.Med Clin North Am.2008;92(2):265–273,vii. .
- Health care market trends and the evolution of hospitalist use and roles.J Gen Intern Med.2005;20(2):101–107. , , , .
- Effects of physician experience on costs and outcomes on an academic general medicine service: results of a trial of hospitalists.Ann Intern Med.2002;137(11):866–874. , , , et al.
- The Park Nicollet experience in establishing a hospitalist system.Ann Intern Med.1999;130(4 pt 2):350–354. .
- Effects of an HMO hospitalist program on inpatient utilization.Am J Manag Care.2001;7(11):1051–1057. , .
- The impact of hospitalists on the cost and quality of inpatient care in the United States: a research synthesis.Med Care Res Rev.2005;62(4):379–406. , .
- Pediatric hospitalists: a systematic review of the literature.Pediatrics.2006;117(5):1736–1744. , , , .
- The hospitalist movement 5 years later.JAMA.2002;287(4):487–494. , .
- Trends in market demand for internal medicine 1999 to 2004: an analysis of physician job advertisements.J Gen Intern Med.2006;21(10):1079–1085. , , , , , .
- SHM. 2003–2004 Survey by the Society of Hospital Medicine on Productivity and Compensation: Analysis of Results. 2004 [updated 2004]. Available at: http://www.hospitalmedicine.org/AM/Template. cfm?Section=Practice_Resources Available at: http://cme.medscape.com/viewarticle/578134. Accessed October 21,2010.
- State of Hospital Medicine: 2010 Report Based on 2009 Data.Englewood, CO and Philadelphia, PA:Medical Group Management Association and Society of Hospital Medicine;2010.
- Worklife and satisfaction of hospitalists: toward flourishing careers.J Gen Intern Med.2011, Jul 20. PMID: 21773849. , , , , .
- Worklife and satisfaction of general internists.Arch Intern Med.2002;162(6):649–656. , , , et al.
- Organizational climate, stress, and error in primary care: the MEMO study. In: Henriksen K, Battles JB, Marks ES, Lewin DI, eds.Advances in Patient Safety: From Research to Implementation. Vol 1: Research Findings.Rockville, MD:Agency for Healthcare Research and Quality;2005;1:65–77. , , , et al.
- Hospitalists and the practice of inpatient medicine: results of a survey of the National Association of Inpatient Physicians.Ann Intern Med.1999;130(4 pt 2):343–349. , , , .
- Physician attitudes toward and prevalence of the hospitalist model of care: results of a national survey.Am J Med.2000;109(8):648–653. , , , , , .
- Taking the Measure of Work: A Guide to Validated Scales for Organizational Research and Diagnosis.Thousand Oaks, CA:Sage Publications;2002. .
- Job Demands and Worker Health.Ann Arbor, MI:University of Michigan, Institute for Social Research;1980. , , , , .
- On the dimensionality of organizational justice: a construct validation of a measure.J Appl Psychol.2001;86(3):386–400. .
- Effect of job demands and social support on worker stress—a study of VDT users.Behav Inform Technol.1995;14(1):32–40. , .
- Measuring physician job satisfaction in a changing workplace and a challenging environment. SGIM Career Satisfaction Study Group. Society of General Internal Medicine.Med Care.1999;37(11):1174–1182. , , , et al.
- Working conditions in primary care: physician reactions and care quality.Ann Intern Med.2009;151(1):28–U48. , , , et al.
- Validation of a single‐item measure of burnout against the Maslach Burnout Inventory among physicians.Stress Health.2004;20(2):75–79. , , .
- American Hospital Association. AHA Hospital Statistics. 2009 [updated 2009]. Available at: http://www.ahadata.com/ahadata/html/AHAStatistics.html. Accessed April 12,2011.
- How to obtain excellent response rates when surveying physicians.Fam Pract.2009;26(1):65–68. , , , et al.
- Estimating nonresponse bias in mail surveys.J Marketing Res.1977;14(3):396–402. , .
- The status of hospital medicine groups in the United States.J Hosp Med.2006;1(2):75–80. , , , .
- Growth in the care of older patients by hospitalists in the United States.N Engl J Med.2009;360(11):1102–1112. , , , .
- The state of hospital medicine in 2008.Med Clin North Am.2008;92(2):265–273,vii. .
- Health care market trends and the evolution of hospitalist use and roles.J Gen Intern Med.2005;20(2):101–107. , , , .
- Effects of physician experience on costs and outcomes on an academic general medicine service: results of a trial of hospitalists.Ann Intern Med.2002;137(11):866–874. , , , et al.
- The Park Nicollet experience in establishing a hospitalist system.Ann Intern Med.1999;130(4 pt 2):350–354. .
- Effects of an HMO hospitalist program on inpatient utilization.Am J Manag Care.2001;7(11):1051–1057. , .
- The impact of hospitalists on the cost and quality of inpatient care in the United States: a research synthesis.Med Care Res Rev.2005;62(4):379–406. , .
- Pediatric hospitalists: a systematic review of the literature.Pediatrics.2006;117(5):1736–1744. , , , .
- The hospitalist movement 5 years later.JAMA.2002;287(4):487–494. , .
- Trends in market demand for internal medicine 1999 to 2004: an analysis of physician job advertisements.J Gen Intern Med.2006;21(10):1079–1085. , , , , , .
- SHM. 2003–2004 Survey by the Society of Hospital Medicine on Productivity and Compensation: Analysis of Results. 2004 [updated 2004]. Available at: http://www.hospitalmedicine.org/AM/Template. cfm?Section=Practice_Resources Available at: http://cme.medscape.com/viewarticle/578134. Accessed October 21,2010.
- State of Hospital Medicine: 2010 Report Based on 2009 Data.Englewood, CO and Philadelphia, PA:Medical Group Management Association and Society of Hospital Medicine;2010.
- Worklife and satisfaction of hospitalists: toward flourishing careers.J Gen Intern Med.2011, Jul 20. PMID: 21773849. , , , , .
- Worklife and satisfaction of general internists.Arch Intern Med.2002;162(6):649–656. , , , et al.
- Organizational climate, stress, and error in primary care: the MEMO study. In: Henriksen K, Battles JB, Marks ES, Lewin DI, eds.Advances in Patient Safety: From Research to Implementation. Vol 1: Research Findings.Rockville, MD:Agency for Healthcare Research and Quality;2005;1:65–77. , , , et al.
- Hospitalists and the practice of inpatient medicine: results of a survey of the National Association of Inpatient Physicians.Ann Intern Med.1999;130(4 pt 2):343–349. , , , .
- Physician attitudes toward and prevalence of the hospitalist model of care: results of a national survey.Am J Med.2000;109(8):648–653. , , , , , .
- Taking the Measure of Work: A Guide to Validated Scales for Organizational Research and Diagnosis.Thousand Oaks, CA:Sage Publications;2002. .
- Job Demands and Worker Health.Ann Arbor, MI:University of Michigan, Institute for Social Research;1980. , , , , .
- On the dimensionality of organizational justice: a construct validation of a measure.J Appl Psychol.2001;86(3):386–400. .
- Effect of job demands and social support on worker stress—a study of VDT users.Behav Inform Technol.1995;14(1):32–40. , .
- Measuring physician job satisfaction in a changing workplace and a challenging environment. SGIM Career Satisfaction Study Group. Society of General Internal Medicine.Med Care.1999;37(11):1174–1182. , , , et al.
- Working conditions in primary care: physician reactions and care quality.Ann Intern Med.2009;151(1):28–U48. , , , et al.
- Validation of a single‐item measure of burnout against the Maslach Burnout Inventory among physicians.Stress Health.2004;20(2):75–79. , , .
- American Hospital Association. AHA Hospital Statistics. 2009 [updated 2009]. Available at: http://www.ahadata.com/ahadata/html/AHAStatistics.html. Accessed April 12,2011.
- How to obtain excellent response rates when surveying physicians.Fam Pract.2009;26(1):65–68. , , , et al.
- Estimating nonresponse bias in mail surveys.J Marketing Res.1977;14(3):396–402. , .
Copyright © 2012 Society of Hospital Medicine
Provider Expectations and Experiences
Comanagement is common in hospital medicine practice. And yet, there is no consensus about how comanagement is different from traditional consultative practice. At its core, hospitalist comanagement is a practice arrangement wherein hospitalists and other specialists manage complex patients collaboratively. Beyond this, Huddleston et al. distinguish comanagement from traditional consultations in the comanaging hospitalists' prerogative to provide direct medical care in addition to consultative advice.1 Siegal focuses on the shared responsibility and authority among partnering providers in the comanagement model.2 Whinney and Michota see comanagement as patient care referral at the onset of a care episode, in contrast to consultations that are activated to address emergent problems.3 In a recent study that found the growing adoption of medical comanagement in Medicare beneficiaries (as much as 40% of surgical hospitalizations in 2006), comanagement was defined as an intensive form of consultation involving a claim for evaluation and management services on greater than 70% of inpatient days.4
In addition to the intensity, frequency, timing, responsibility, and authority of care, comanagement may be described by participating physicians' roles. With recent attention on multidisciplinary teams and an increasing focus on collaborative care, many of the hierarchical relations among healthcare providers are breaking down.5 Several studies of multidisciplinary teams suggest that more egalitarian, rather than hierarchical, problem‐solving and decision‐making among team members are beneficial to patients.67 However, neither the intended nor natural team structure under comanagement is known. We sought to shed some light on provider interactions by characterizing the expectations and experiences of providers of a comanaged service. The findings yielded an opportunity to generate an evolving, but conceptually supported definition of comanagement.
SETTING
We conducted a survey study of providers participating in a comanaged inpatient hepatology service at the University of Chicago Medical Center, a 572‐bed urban teaching hospital. The service was created in 2006, partly to address staffing problems related to housestaff work hour restrictions and partly to improve the care of candidates and recipients of liver transplantation. Nonsurgical floor patients with liver diseases were managed on the service by two collaborating teams of providers. The hepatology team consisted of an attending physician and a fellow, while the hospitalist team consisted of a hospitalist and one or two nonphysician providers (physician assistant or nurse practitioner). The practice model is characterized as comanagement because of the highly interdependent nature of the team's daily tasks and the norms of intensive communication, through formal joint daily rounds and informal direct exchanges of instructions and updates. Hepatologists were mainly responsible for coordinating admissions, managing issues related to liver dysfunction, communicating with transplant surgeons if necessary, and arranging postdischarge care. Hospitalists were responsible for admitting patients, managing routine (eg, ordering daily labs) and urgent issues (eg, responding to critical lab values) during hospitalizations, coordinating with ancillary and consultative staff, and discharging patients. Occasional meetings between the hepatology and hospital medicine groups were used to clarify assignment of responsibilities. Floor nurses received in‐servicing at the commencement of the service. Additional details about the service are described elsewhere.8
DATA COLLECTION AND ANALYSIS
For the purpose of our analysis, we defined interactions between any member of the hospitalist and hepatologist teams as pertinent to comanagement. The hospitalist nonphysician provider (NPP) and hepatologistfellow relationships are governed by the more traditional hierarchical dynamics based on supervision and authority according to laws and regulations. At the beginning of the study period, each participant completed nine items of a Baseline Survey that addressed respondents' expectations and preferences for the management of an ideally comanaged service. Responses were solicited using a 4‐point Likert‐type scale and were dichotomized such that agree and somewhat agree were grouped, while disagree and somewhat disagree were grouped for data analysis. Items were generated to address the salient issues of comanagement after reviewing the pertinent literature.
Subsequently, participants were asked to complete Repeated Surveys immediately before each change in membership of the comanaged team between April and October 2008. The surveys were hand delivered by one of the authors (K.H.) on the last day of each team's rotation and were often completed immediately. The seven items of the Repeated Survey reprised items from the Baseline Survey that were rephrased to allow respondents to report their direct experiences on specific teams. Because all providers rotated on the service more than once during the study period, the average value for each Likert‐type response across multiple surveys completed by a single provider was calculated before being dichotomized at the midpoint (<2.5, agree; 2.5, disagree). We reported proportions of respondents in agreement with survey item statements.
Comparison statistics across providers were generated using the chi‐square test. Differences in proportions between related items of the Baseline and Repeated Surveys were compared using the two‐sample test of proportions. All analyses were conducted using a statistics application (STATA 10.0, College Station, TX) with alpha equal to, or less than, 0.05 considered significant. The Institutional Review Board of the University of Chicago approved this project.
RESULTS
All 43 providers completed the Baseline Survey. During the study period, 32 of these participants rotated on the service and completed 177 of the 233 Repeated Surveys (79%) administered. The responses describe team interactions on the 47 unique combinations of providers comprising the comanaged teams. Details of the response rates are shown in Table 1.
Baseline Survey, Completed/ Administered (%) | Repeated Surveys, Completed/ Administered (%) | Respondents Completing Repeated Surveys, n | Repeated Surveys Completed per Respondent, Median (IQR) | |
---|---|---|---|---|
| ||||
Hospitalists | 18/18 (100) | 36/43 (84) | 15 | 2 (2, 3) |
NPPs | 5/5 (100) | 92/97 (95) | 5 | 20 (18, 20) |
Hepatologists | 6/6 (100) | 26/42 (62) | 6 | 7 (3.75, 8) |
Fellows | 12/12 (100) | 23/42 (55) | 6 | 7 (5.5, 8.5) |
Total | 43/43 (100) | 177/223 (79) | 32 | 4.5 (2, 8.25) |
As shown in Table 2A, items 13, more members of the hospitalist team preferred to be informed about every management decision compared to members of the hepatologist team. Conversely, more of members of the hepatologist team than the hospitalist team preferred their comanaging partners to participate in every decision. A statistically similar proportion of respondents in each of the professional roles indicated desire for greater influence in directing management decisions (Table 2B, item 1).
A. Baseline Survey | Hospitalists, % (n = 18) | NPPs, % (n = 5) | Hepatologists, % (n = 6) | GI Fellows, % (n = 12) | P‐value |
---|---|---|---|---|---|
| |||||
1. I prefer to be informed about every decision. | 83 | 100 | 17 | 42 | <0.01 |
2. I prefer to participate in every decision. | 67 | 100 | 33 | 50 | 0.11 |
3. I prefer that my comanager participate in every decision. | 22 | 20 | 50 | 75 | 0.02 |
4. I prefer to have the final say in every decision. | 50 | 80 | 50 | 33 | 0.38 |
5. There should be one physician leader to direct the overall management of the patients' hospital course. | 89* | 100 | 67 | 83 | 0.43 |
6. Physician consensus should always be sought in every clinical decision. | 22 | 40 | 50 | 67 | 0.11 |
7. I have a clear understanding of my role on the comanagement service. | 61 | 80 | 83 | 75 | 0.66 |
8. I have as much a sense of ownership of patients on the comanaged service as on a non‐comanaged service. | 61 | 60 | 83 | 50 | 0.60 |
9. Comanagement tends to improve patient care. | 94 | 100* | 83 | 100* | 0.47 |
B. Repeated Surveys | Hospitalists, % (n = 15) | NPPs, % (n = 5) | Hepatologists, % (n = 6) | GI Fellows, % (n = 6) | P‐value |
1. I would have liked greater influence in directing the overall management. | 40 | 60 | 0 | 17 | 0.12 |
2. I was responsible for work in clinical areas I was not comfortable managing. | 0 | 0 | 0 | 0 | NA |
3. There was one physician leader to direct the overall management of the patients' hospital course. | 60* | 80 | 67 | 83 | 0.70 |
4. Physician consensus was always sought in every clinical decision. | 40 | 40 | 50 | 67 | 0.72 |
5. I (have/had) a clear understanding of my role on the comanagement service. | 73 | 80 | 100 | 83 | 0.57 |
6. I had as much a sense of ownership of patients on the comanaged service as on a non‐comanaged service. | 53 | 80 | 100 | 67 | 0.20 |
7. Patients on my service received better care than they would have without comanagement. | 93 | 40* | 67 | 50* | 0.06 |
For the majority of surveyed areas, there was concordance between expectations and experiences of providers on comanagement. Most providers, regardless of professional role, agreed that there should be a single physician leader to direct the overall management (Table 2A, item 5). The majority perceived that a single physician directed the overall management of the patients' hospital course, although fewer hospitalists did so compared with baseline expectations (Table 2B, item 3). Many respondents felt at baseline that physician consensus should govern every management decision, and a similar proportion actually experienced consensus‐seeking on service.
We found that the proportion of providers reporting an understanding of their role increased slightly, though not significantly, from before (Table 2A, item 7) to after rotating on the comanaged service (Table 2B, item 5). Although not statistically significant, there was a trend towards hospitalists and gastrointestinal (GI) fellows reporting a lack of patient ownership, both before and after serving on the comanaged service. Finally, nearly all respondents reported that comanagement should improve care quality, although only the attending hospitalist and hepatologist felt that their experience on the comanaged service actually improved patient care (Table 2B, item 7).
DISCUSSION
In this survey of providers participating on a comanaged medical service, most reported understanding their role in the collaborative arrangement and had an initial perception that comanagement should improve patient care quality. We found that hospitalists preferred and were expected to participate in care globally, while hepatologists themselves preferred and were expected not to focus on every management decision. The prevalence of desire for ultimate authority across the professional roles suggests tensions that exist in this care model around how decisions are made. The majority of providers preferred and experienced a single physician leader under comanagement, but many also experienced consensus‐seeking for every management decision.
From these findings, we conclude that decision‐making processes are not uniform under comanagement and that some role ambiguity is present, but there appears to be a pattern of natural roles. This pattern can be defined by focus (general for hospitalists vs specialty‐specific for hepatologists), rather than by responsibilities for managing particular medical problems. The preference among both generalists and specialists for the broader involvement of hospitalist comanagers suggests an implicit recognition of the need for integrated management to overcome the silo‐effect within the comanagement structure.9 Although details about how such integration was achieved are not available in our data, we found that comanagement may be distinct from traditional consultative practice in that the consultants (hospitalists in this case) manage not only general medical problems, such as diabetes or hypertension, but hospitalizations more generally. From a mission‐based standpoint, comanagement may be seen as a collaborative management of complex patients by two or more clinical experts with distinct knowledge, skills, or focus enacted for the purpose of improving care quality.
The focus of comanagement on improving quality is in line with the founding charge of the hospital medicine specialty to raise hospital care quality.10 In fact, the distinction between comanagement and consultation may be meaningful only if comanagers can work with specialists to implement evidence‐based practice, process improvement, and address quality and cost concerns. But as seen in NPPs and fellows' skepticism of improved quality under comanagement, there is still clearly work to be done to validate this model through measurable improvement in patients' experiences and outcomes. Proving the advantages of comanagement as a platform for practice improvement remains future work.11
Collaborative arrangements create natural tensions related to team function.5 This is seen in the similar proportion of hospitalists and hepatologists indicating desire for final decision‐making authority. Although comanagement evokes assumptions about egalitarian provider interactions involving shared decision‐making and responsibility, it seems to function empirically under hierarchical as well as consensus‐seeking forms of decision‐making. Providers at the top of hierarchical teams typically experience their work as interdependent and collaborative, and report more positive interactions with other care providers.12 Based on the fact that no hepatologists wanted more influence over decision‐making, we assume that hepatologists were the physician leaders for most of the studied comanaged teams. Under situations characterized by high levels of complexity and interdependence, a team governed by a single leader may often be more effective than one governed by shared authority.8 However, even under hierarchical models, a more participatory than supervisory leadership can help avoid alienating partners through a pattern of we decide, you carry it out that is often associated with ineffective leadership styles.1314 In fact, this alienating effect on providers in subordinate roles (ie, NPPs and fellows) may have contributed to the negative perception of the team's function on improving patient care.
This study is limited in the following ways. We did not have 100% participation in the Repeated Surveys. Attitudes and experiences of participants in a single comanagement practice are not representative of all comanaging providers. However, the goal of this studyto collect unique survey data from providers themselves to inform an evolving definition of comanagementis modest enough in scope to not require a generalizable sample. Because this study unearthed differences in expectations and experiences within a single site, they may serve as a lower bound for the extent of differences across and within multiple sites. In addition, comanagement enacted for complex medical patients is not as common as the comanagement of surgical patients. Moreover, comanagement models in academic hospitals may have structural features and priorities not found in community settings. Whether or not these disparate models share enough in common to be categorized under a single rubric is a valid question.
Although the teamwork structure and provider roles within comanagement vary, the practice arrangement's preoccupation with quality can be seen as its defining feature. Limited evidence, to date,1, 1519 and the rapid proliferation of the model, suggest that quality and efficiency advantages can be obtained from an effective implementation of comanagement. As in any team‐based care model, a common understanding of roles and expectations are essential to enhancing teamwork. Our interpretation of the mission of comanagement may further enhance teamwork through an explicit articulation of shared goals.
- Medical and surgical comanagement after elective hip and knee arthroplasty: A randomized, controlled trial.Ann Intern Med.2004;141(1):28–38. , , , et al.
- Just because you can, doesn't mean that you should: A call for the rational application of hospitalist comanagement.J Hosp Med.2008;3(5):398–402. .
- Surgical comanagement: A natural evolution of hospitalist practice.J Hosp Med.2008;3(5):394–397. , .
- Comanagement of hospitalized surgical patients by medicine physicians in the United States.Arch Intern Med.2010;170(4):363–368. , , , , .
- Structure and meaning in multidisciplinary teamwork.Sociol Health Illn.1998;20(6):848–873. .
- Human factors and cardiac surgery: A multicenter study.J Thorac Cardiov Surg.2000;119(4):661–670. , , , , .
- The effects of a collaborative model of primary care on the mortality and hospital use of community‐dwelling older adults.J Gerontol A‐Biol.2001;56(2):M106–M112. , , .
- Effects of provider characteristics on care coordination under comanagement.J Hosp Med.2010;5:508–513. , , , , , .
- Crossing the Quality Chasm: A New Health System for the Twenty‐First Century.Washington, DC:Institute of Medicine;2001. , , .
- The emerging role of “hospitalists” in the American health care system.N Engl J Med.1996;335(7):514–517. , .
- Internal medicine comanagement of surgical patients: Can we afford to do this?Arch Intern Med.2010;170(22):1965–1966. .
- Operating room teamwork among physicians and nurses: Teamwork in the eye of the beholder.J Am Coll Surg.2006;202(5):746–752. , , , et al.
- “We decide, you carry it out”: A social network analysis of multidisciplinary longterm care teams.Soc Sci Med.1997;45(9):1411–1421. .
- Patterns of aggressive behavior in experimentally created social climates.J Soc Psychol.1939;10:271–301. , , .
- Comanagement of surgical patients between neurosurgeons and hospitalists.Arch Intern Med.2010;170(22):2004–2010. , , , et al.
- Outcomes for older patients with hip fractures: The impact of orthopedic and geriatric medicine cocare.J Orthop Trauma.2006;20(3):172–180. , , , , , .
- Effects of a hospitalist model on elderly patients with hip fracture.Arch Intern Med.2005;165(7):796–801. , , , et al.
- Hip fractures in geriatric patients. Results of an interdisciplinary hospital care program.Clin Orthop Relat Res.1992(274):213–225. , , , .
- Impact of a comanaged Geriatric Fracture Center on short‐term hip fracture outcomes.Arch Intern Med.2009;169(18):1712–1717. , , , .
Comanagement is common in hospital medicine practice. And yet, there is no consensus about how comanagement is different from traditional consultative practice. At its core, hospitalist comanagement is a practice arrangement wherein hospitalists and other specialists manage complex patients collaboratively. Beyond this, Huddleston et al. distinguish comanagement from traditional consultations in the comanaging hospitalists' prerogative to provide direct medical care in addition to consultative advice.1 Siegal focuses on the shared responsibility and authority among partnering providers in the comanagement model.2 Whinney and Michota see comanagement as patient care referral at the onset of a care episode, in contrast to consultations that are activated to address emergent problems.3 In a recent study that found the growing adoption of medical comanagement in Medicare beneficiaries (as much as 40% of surgical hospitalizations in 2006), comanagement was defined as an intensive form of consultation involving a claim for evaluation and management services on greater than 70% of inpatient days.4
In addition to the intensity, frequency, timing, responsibility, and authority of care, comanagement may be described by participating physicians' roles. With recent attention on multidisciplinary teams and an increasing focus on collaborative care, many of the hierarchical relations among healthcare providers are breaking down.5 Several studies of multidisciplinary teams suggest that more egalitarian, rather than hierarchical, problem‐solving and decision‐making among team members are beneficial to patients.67 However, neither the intended nor natural team structure under comanagement is known. We sought to shed some light on provider interactions by characterizing the expectations and experiences of providers of a comanaged service. The findings yielded an opportunity to generate an evolving, but conceptually supported definition of comanagement.
SETTING
We conducted a survey study of providers participating in a comanaged inpatient hepatology service at the University of Chicago Medical Center, a 572‐bed urban teaching hospital. The service was created in 2006, partly to address staffing problems related to housestaff work hour restrictions and partly to improve the care of candidates and recipients of liver transplantation. Nonsurgical floor patients with liver diseases were managed on the service by two collaborating teams of providers. The hepatology team consisted of an attending physician and a fellow, while the hospitalist team consisted of a hospitalist and one or two nonphysician providers (physician assistant or nurse practitioner). The practice model is characterized as comanagement because of the highly interdependent nature of the team's daily tasks and the norms of intensive communication, through formal joint daily rounds and informal direct exchanges of instructions and updates. Hepatologists were mainly responsible for coordinating admissions, managing issues related to liver dysfunction, communicating with transplant surgeons if necessary, and arranging postdischarge care. Hospitalists were responsible for admitting patients, managing routine (eg, ordering daily labs) and urgent issues (eg, responding to critical lab values) during hospitalizations, coordinating with ancillary and consultative staff, and discharging patients. Occasional meetings between the hepatology and hospital medicine groups were used to clarify assignment of responsibilities. Floor nurses received in‐servicing at the commencement of the service. Additional details about the service are described elsewhere.8
DATA COLLECTION AND ANALYSIS
For the purpose of our analysis, we defined interactions between any member of the hospitalist and hepatologist teams as pertinent to comanagement. The hospitalist nonphysician provider (NPP) and hepatologistfellow relationships are governed by the more traditional hierarchical dynamics based on supervision and authority according to laws and regulations. At the beginning of the study period, each participant completed nine items of a Baseline Survey that addressed respondents' expectations and preferences for the management of an ideally comanaged service. Responses were solicited using a 4‐point Likert‐type scale and were dichotomized such that agree and somewhat agree were grouped, while disagree and somewhat disagree were grouped for data analysis. Items were generated to address the salient issues of comanagement after reviewing the pertinent literature.
Subsequently, participants were asked to complete Repeated Surveys immediately before each change in membership of the comanaged team between April and October 2008. The surveys were hand delivered by one of the authors (K.H.) on the last day of each team's rotation and were often completed immediately. The seven items of the Repeated Survey reprised items from the Baseline Survey that were rephrased to allow respondents to report their direct experiences on specific teams. Because all providers rotated on the service more than once during the study period, the average value for each Likert‐type response across multiple surveys completed by a single provider was calculated before being dichotomized at the midpoint (<2.5, agree; 2.5, disagree). We reported proportions of respondents in agreement with survey item statements.
Comparison statistics across providers were generated using the chi‐square test. Differences in proportions between related items of the Baseline and Repeated Surveys were compared using the two‐sample test of proportions. All analyses were conducted using a statistics application (STATA 10.0, College Station, TX) with alpha equal to, or less than, 0.05 considered significant. The Institutional Review Board of the University of Chicago approved this project.
RESULTS
All 43 providers completed the Baseline Survey. During the study period, 32 of these participants rotated on the service and completed 177 of the 233 Repeated Surveys (79%) administered. The responses describe team interactions on the 47 unique combinations of providers comprising the comanaged teams. Details of the response rates are shown in Table 1.
Baseline Survey, Completed/ Administered (%) | Repeated Surveys, Completed/ Administered (%) | Respondents Completing Repeated Surveys, n | Repeated Surveys Completed per Respondent, Median (IQR) | |
---|---|---|---|---|
| ||||
Hospitalists | 18/18 (100) | 36/43 (84) | 15 | 2 (2, 3) |
NPPs | 5/5 (100) | 92/97 (95) | 5 | 20 (18, 20) |
Hepatologists | 6/6 (100) | 26/42 (62) | 6 | 7 (3.75, 8) |
Fellows | 12/12 (100) | 23/42 (55) | 6 | 7 (5.5, 8.5) |
Total | 43/43 (100) | 177/223 (79) | 32 | 4.5 (2, 8.25) |
As shown in Table 2A, items 13, more members of the hospitalist team preferred to be informed about every management decision compared to members of the hepatologist team. Conversely, more of members of the hepatologist team than the hospitalist team preferred their comanaging partners to participate in every decision. A statistically similar proportion of respondents in each of the professional roles indicated desire for greater influence in directing management decisions (Table 2B, item 1).
A. Baseline Survey | Hospitalists, % (n = 18) | NPPs, % (n = 5) | Hepatologists, % (n = 6) | GI Fellows, % (n = 12) | P‐value |
---|---|---|---|---|---|
| |||||
1. I prefer to be informed about every decision. | 83 | 100 | 17 | 42 | <0.01 |
2. I prefer to participate in every decision. | 67 | 100 | 33 | 50 | 0.11 |
3. I prefer that my comanager participate in every decision. | 22 | 20 | 50 | 75 | 0.02 |
4. I prefer to have the final say in every decision. | 50 | 80 | 50 | 33 | 0.38 |
5. There should be one physician leader to direct the overall management of the patients' hospital course. | 89* | 100 | 67 | 83 | 0.43 |
6. Physician consensus should always be sought in every clinical decision. | 22 | 40 | 50 | 67 | 0.11 |
7. I have a clear understanding of my role on the comanagement service. | 61 | 80 | 83 | 75 | 0.66 |
8. I have as much a sense of ownership of patients on the comanaged service as on a non‐comanaged service. | 61 | 60 | 83 | 50 | 0.60 |
9. Comanagement tends to improve patient care. | 94 | 100* | 83 | 100* | 0.47 |
B. Repeated Surveys | Hospitalists, % (n = 15) | NPPs, % (n = 5) | Hepatologists, % (n = 6) | GI Fellows, % (n = 6) | P‐value |
1. I would have liked greater influence in directing the overall management. | 40 | 60 | 0 | 17 | 0.12 |
2. I was responsible for work in clinical areas I was not comfortable managing. | 0 | 0 | 0 | 0 | NA |
3. There was one physician leader to direct the overall management of the patients' hospital course. | 60* | 80 | 67 | 83 | 0.70 |
4. Physician consensus was always sought in every clinical decision. | 40 | 40 | 50 | 67 | 0.72 |
5. I (have/had) a clear understanding of my role on the comanagement service. | 73 | 80 | 100 | 83 | 0.57 |
6. I had as much a sense of ownership of patients on the comanaged service as on a non‐comanaged service. | 53 | 80 | 100 | 67 | 0.20 |
7. Patients on my service received better care than they would have without comanagement. | 93 | 40* | 67 | 50* | 0.06 |
For the majority of surveyed areas, there was concordance between expectations and experiences of providers on comanagement. Most providers, regardless of professional role, agreed that there should be a single physician leader to direct the overall management (Table 2A, item 5). The majority perceived that a single physician directed the overall management of the patients' hospital course, although fewer hospitalists did so compared with baseline expectations (Table 2B, item 3). Many respondents felt at baseline that physician consensus should govern every management decision, and a similar proportion actually experienced consensus‐seeking on service.
We found that the proportion of providers reporting an understanding of their role increased slightly, though not significantly, from before (Table 2A, item 7) to after rotating on the comanaged service (Table 2B, item 5). Although not statistically significant, there was a trend towards hospitalists and gastrointestinal (GI) fellows reporting a lack of patient ownership, both before and after serving on the comanaged service. Finally, nearly all respondents reported that comanagement should improve care quality, although only the attending hospitalist and hepatologist felt that their experience on the comanaged service actually improved patient care (Table 2B, item 7).
DISCUSSION
In this survey of providers participating on a comanaged medical service, most reported understanding their role in the collaborative arrangement and had an initial perception that comanagement should improve patient care quality. We found that hospitalists preferred and were expected to participate in care globally, while hepatologists themselves preferred and were expected not to focus on every management decision. The prevalence of desire for ultimate authority across the professional roles suggests tensions that exist in this care model around how decisions are made. The majority of providers preferred and experienced a single physician leader under comanagement, but many also experienced consensus‐seeking for every management decision.
From these findings, we conclude that decision‐making processes are not uniform under comanagement and that some role ambiguity is present, but there appears to be a pattern of natural roles. This pattern can be defined by focus (general for hospitalists vs specialty‐specific for hepatologists), rather than by responsibilities for managing particular medical problems. The preference among both generalists and specialists for the broader involvement of hospitalist comanagers suggests an implicit recognition of the need for integrated management to overcome the silo‐effect within the comanagement structure.9 Although details about how such integration was achieved are not available in our data, we found that comanagement may be distinct from traditional consultative practice in that the consultants (hospitalists in this case) manage not only general medical problems, such as diabetes or hypertension, but hospitalizations more generally. From a mission‐based standpoint, comanagement may be seen as a collaborative management of complex patients by two or more clinical experts with distinct knowledge, skills, or focus enacted for the purpose of improving care quality.
The focus of comanagement on improving quality is in line with the founding charge of the hospital medicine specialty to raise hospital care quality.10 In fact, the distinction between comanagement and consultation may be meaningful only if comanagers can work with specialists to implement evidence‐based practice, process improvement, and address quality and cost concerns. But as seen in NPPs and fellows' skepticism of improved quality under comanagement, there is still clearly work to be done to validate this model through measurable improvement in patients' experiences and outcomes. Proving the advantages of comanagement as a platform for practice improvement remains future work.11
Collaborative arrangements create natural tensions related to team function.5 This is seen in the similar proportion of hospitalists and hepatologists indicating desire for final decision‐making authority. Although comanagement evokes assumptions about egalitarian provider interactions involving shared decision‐making and responsibility, it seems to function empirically under hierarchical as well as consensus‐seeking forms of decision‐making. Providers at the top of hierarchical teams typically experience their work as interdependent and collaborative, and report more positive interactions with other care providers.12 Based on the fact that no hepatologists wanted more influence over decision‐making, we assume that hepatologists were the physician leaders for most of the studied comanaged teams. Under situations characterized by high levels of complexity and interdependence, a team governed by a single leader may often be more effective than one governed by shared authority.8 However, even under hierarchical models, a more participatory than supervisory leadership can help avoid alienating partners through a pattern of we decide, you carry it out that is often associated with ineffective leadership styles.1314 In fact, this alienating effect on providers in subordinate roles (ie, NPPs and fellows) may have contributed to the negative perception of the team's function on improving patient care.
This study is limited in the following ways. We did not have 100% participation in the Repeated Surveys. Attitudes and experiences of participants in a single comanagement practice are not representative of all comanaging providers. However, the goal of this studyto collect unique survey data from providers themselves to inform an evolving definition of comanagementis modest enough in scope to not require a generalizable sample. Because this study unearthed differences in expectations and experiences within a single site, they may serve as a lower bound for the extent of differences across and within multiple sites. In addition, comanagement enacted for complex medical patients is not as common as the comanagement of surgical patients. Moreover, comanagement models in academic hospitals may have structural features and priorities not found in community settings. Whether or not these disparate models share enough in common to be categorized under a single rubric is a valid question.
Although the teamwork structure and provider roles within comanagement vary, the practice arrangement's preoccupation with quality can be seen as its defining feature. Limited evidence, to date,1, 1519 and the rapid proliferation of the model, suggest that quality and efficiency advantages can be obtained from an effective implementation of comanagement. As in any team‐based care model, a common understanding of roles and expectations are essential to enhancing teamwork. Our interpretation of the mission of comanagement may further enhance teamwork through an explicit articulation of shared goals.
Comanagement is common in hospital medicine practice. And yet, there is no consensus about how comanagement is different from traditional consultative practice. At its core, hospitalist comanagement is a practice arrangement wherein hospitalists and other specialists manage complex patients collaboratively. Beyond this, Huddleston et al. distinguish comanagement from traditional consultations in the comanaging hospitalists' prerogative to provide direct medical care in addition to consultative advice.1 Siegal focuses on the shared responsibility and authority among partnering providers in the comanagement model.2 Whinney and Michota see comanagement as patient care referral at the onset of a care episode, in contrast to consultations that are activated to address emergent problems.3 In a recent study that found the growing adoption of medical comanagement in Medicare beneficiaries (as much as 40% of surgical hospitalizations in 2006), comanagement was defined as an intensive form of consultation involving a claim for evaluation and management services on greater than 70% of inpatient days.4
In addition to the intensity, frequency, timing, responsibility, and authority of care, comanagement may be described by participating physicians' roles. With recent attention on multidisciplinary teams and an increasing focus on collaborative care, many of the hierarchical relations among healthcare providers are breaking down.5 Several studies of multidisciplinary teams suggest that more egalitarian, rather than hierarchical, problem‐solving and decision‐making among team members are beneficial to patients.67 However, neither the intended nor natural team structure under comanagement is known. We sought to shed some light on provider interactions by characterizing the expectations and experiences of providers of a comanaged service. The findings yielded an opportunity to generate an evolving, but conceptually supported definition of comanagement.
SETTING
We conducted a survey study of providers participating in a comanaged inpatient hepatology service at the University of Chicago Medical Center, a 572‐bed urban teaching hospital. The service was created in 2006, partly to address staffing problems related to housestaff work hour restrictions and partly to improve the care of candidates and recipients of liver transplantation. Nonsurgical floor patients with liver diseases were managed on the service by two collaborating teams of providers. The hepatology team consisted of an attending physician and a fellow, while the hospitalist team consisted of a hospitalist and one or two nonphysician providers (physician assistant or nurse practitioner). The practice model is characterized as comanagement because of the highly interdependent nature of the team's daily tasks and the norms of intensive communication, through formal joint daily rounds and informal direct exchanges of instructions and updates. Hepatologists were mainly responsible for coordinating admissions, managing issues related to liver dysfunction, communicating with transplant surgeons if necessary, and arranging postdischarge care. Hospitalists were responsible for admitting patients, managing routine (eg, ordering daily labs) and urgent issues (eg, responding to critical lab values) during hospitalizations, coordinating with ancillary and consultative staff, and discharging patients. Occasional meetings between the hepatology and hospital medicine groups were used to clarify assignment of responsibilities. Floor nurses received in‐servicing at the commencement of the service. Additional details about the service are described elsewhere.8
DATA COLLECTION AND ANALYSIS
For the purpose of our analysis, we defined interactions between any member of the hospitalist and hepatologist teams as pertinent to comanagement. The hospitalist nonphysician provider (NPP) and hepatologistfellow relationships are governed by the more traditional hierarchical dynamics based on supervision and authority according to laws and regulations. At the beginning of the study period, each participant completed nine items of a Baseline Survey that addressed respondents' expectations and preferences for the management of an ideally comanaged service. Responses were solicited using a 4‐point Likert‐type scale and were dichotomized such that agree and somewhat agree were grouped, while disagree and somewhat disagree were grouped for data analysis. Items were generated to address the salient issues of comanagement after reviewing the pertinent literature.
Subsequently, participants were asked to complete Repeated Surveys immediately before each change in membership of the comanaged team between April and October 2008. The surveys were hand delivered by one of the authors (K.H.) on the last day of each team's rotation and were often completed immediately. The seven items of the Repeated Survey reprised items from the Baseline Survey that were rephrased to allow respondents to report their direct experiences on specific teams. Because all providers rotated on the service more than once during the study period, the average value for each Likert‐type response across multiple surveys completed by a single provider was calculated before being dichotomized at the midpoint (<2.5, agree; 2.5, disagree). We reported proportions of respondents in agreement with survey item statements.
Comparison statistics across providers were generated using the chi‐square test. Differences in proportions between related items of the Baseline and Repeated Surveys were compared using the two‐sample test of proportions. All analyses were conducted using a statistics application (STATA 10.0, College Station, TX) with alpha equal to, or less than, 0.05 considered significant. The Institutional Review Board of the University of Chicago approved this project.
RESULTS
All 43 providers completed the Baseline Survey. During the study period, 32 of these participants rotated on the service and completed 177 of the 233 Repeated Surveys (79%) administered. The responses describe team interactions on the 47 unique combinations of providers comprising the comanaged teams. Details of the response rates are shown in Table 1.
Baseline Survey, Completed/ Administered (%) | Repeated Surveys, Completed/ Administered (%) | Respondents Completing Repeated Surveys, n | Repeated Surveys Completed per Respondent, Median (IQR) | |
---|---|---|---|---|
| ||||
Hospitalists | 18/18 (100) | 36/43 (84) | 15 | 2 (2, 3) |
NPPs | 5/5 (100) | 92/97 (95) | 5 | 20 (18, 20) |
Hepatologists | 6/6 (100) | 26/42 (62) | 6 | 7 (3.75, 8) |
Fellows | 12/12 (100) | 23/42 (55) | 6 | 7 (5.5, 8.5) |
Total | 43/43 (100) | 177/223 (79) | 32 | 4.5 (2, 8.25) |
As shown in Table 2A, items 13, more members of the hospitalist team preferred to be informed about every management decision compared to members of the hepatologist team. Conversely, more of members of the hepatologist team than the hospitalist team preferred their comanaging partners to participate in every decision. A statistically similar proportion of respondents in each of the professional roles indicated desire for greater influence in directing management decisions (Table 2B, item 1).
A. Baseline Survey | Hospitalists, % (n = 18) | NPPs, % (n = 5) | Hepatologists, % (n = 6) | GI Fellows, % (n = 12) | P‐value |
---|---|---|---|---|---|
| |||||
1. I prefer to be informed about every decision. | 83 | 100 | 17 | 42 | <0.01 |
2. I prefer to participate in every decision. | 67 | 100 | 33 | 50 | 0.11 |
3. I prefer that my comanager participate in every decision. | 22 | 20 | 50 | 75 | 0.02 |
4. I prefer to have the final say in every decision. | 50 | 80 | 50 | 33 | 0.38 |
5. There should be one physician leader to direct the overall management of the patients' hospital course. | 89* | 100 | 67 | 83 | 0.43 |
6. Physician consensus should always be sought in every clinical decision. | 22 | 40 | 50 | 67 | 0.11 |
7. I have a clear understanding of my role on the comanagement service. | 61 | 80 | 83 | 75 | 0.66 |
8. I have as much a sense of ownership of patients on the comanaged service as on a non‐comanaged service. | 61 | 60 | 83 | 50 | 0.60 |
9. Comanagement tends to improve patient care. | 94 | 100* | 83 | 100* | 0.47 |
B. Repeated Surveys | Hospitalists, % (n = 15) | NPPs, % (n = 5) | Hepatologists, % (n = 6) | GI Fellows, % (n = 6) | P‐value |
1. I would have liked greater influence in directing the overall management. | 40 | 60 | 0 | 17 | 0.12 |
2. I was responsible for work in clinical areas I was not comfortable managing. | 0 | 0 | 0 | 0 | NA |
3. There was one physician leader to direct the overall management of the patients' hospital course. | 60* | 80 | 67 | 83 | 0.70 |
4. Physician consensus was always sought in every clinical decision. | 40 | 40 | 50 | 67 | 0.72 |
5. I (have/had) a clear understanding of my role on the comanagement service. | 73 | 80 | 100 | 83 | 0.57 |
6. I had as much a sense of ownership of patients on the comanaged service as on a non‐comanaged service. | 53 | 80 | 100 | 67 | 0.20 |
7. Patients on my service received better care than they would have without comanagement. | 93 | 40* | 67 | 50* | 0.06 |
For the majority of surveyed areas, there was concordance between expectations and experiences of providers on comanagement. Most providers, regardless of professional role, agreed that there should be a single physician leader to direct the overall management (Table 2A, item 5). The majority perceived that a single physician directed the overall management of the patients' hospital course, although fewer hospitalists did so compared with baseline expectations (Table 2B, item 3). Many respondents felt at baseline that physician consensus should govern every management decision, and a similar proportion actually experienced consensus‐seeking on service.
We found that the proportion of providers reporting an understanding of their role increased slightly, though not significantly, from before (Table 2A, item 7) to after rotating on the comanaged service (Table 2B, item 5). Although not statistically significant, there was a trend towards hospitalists and gastrointestinal (GI) fellows reporting a lack of patient ownership, both before and after serving on the comanaged service. Finally, nearly all respondents reported that comanagement should improve care quality, although only the attending hospitalist and hepatologist felt that their experience on the comanaged service actually improved patient care (Table 2B, item 7).
DISCUSSION
In this survey of providers participating on a comanaged medical service, most reported understanding their role in the collaborative arrangement and had an initial perception that comanagement should improve patient care quality. We found that hospitalists preferred and were expected to participate in care globally, while hepatologists themselves preferred and were expected not to focus on every management decision. The prevalence of desire for ultimate authority across the professional roles suggests tensions that exist in this care model around how decisions are made. The majority of providers preferred and experienced a single physician leader under comanagement, but many also experienced consensus‐seeking for every management decision.
From these findings, we conclude that decision‐making processes are not uniform under comanagement and that some role ambiguity is present, but there appears to be a pattern of natural roles. This pattern can be defined by focus (general for hospitalists vs specialty‐specific for hepatologists), rather than by responsibilities for managing particular medical problems. The preference among both generalists and specialists for the broader involvement of hospitalist comanagers suggests an implicit recognition of the need for integrated management to overcome the silo‐effect within the comanagement structure.9 Although details about how such integration was achieved are not available in our data, we found that comanagement may be distinct from traditional consultative practice in that the consultants (hospitalists in this case) manage not only general medical problems, such as diabetes or hypertension, but hospitalizations more generally. From a mission‐based standpoint, comanagement may be seen as a collaborative management of complex patients by two or more clinical experts with distinct knowledge, skills, or focus enacted for the purpose of improving care quality.
The focus of comanagement on improving quality is in line with the founding charge of the hospital medicine specialty to raise hospital care quality.10 In fact, the distinction between comanagement and consultation may be meaningful only if comanagers can work with specialists to implement evidence‐based practice, process improvement, and address quality and cost concerns. But as seen in NPPs and fellows' skepticism of improved quality under comanagement, there is still clearly work to be done to validate this model through measurable improvement in patients' experiences and outcomes. Proving the advantages of comanagement as a platform for practice improvement remains future work.11
Collaborative arrangements create natural tensions related to team function.5 This is seen in the similar proportion of hospitalists and hepatologists indicating desire for final decision‐making authority. Although comanagement evokes assumptions about egalitarian provider interactions involving shared decision‐making and responsibility, it seems to function empirically under hierarchical as well as consensus‐seeking forms of decision‐making. Providers at the top of hierarchical teams typically experience their work as interdependent and collaborative, and report more positive interactions with other care providers.12 Based on the fact that no hepatologists wanted more influence over decision‐making, we assume that hepatologists were the physician leaders for most of the studied comanaged teams. Under situations characterized by high levels of complexity and interdependence, a team governed by a single leader may often be more effective than one governed by shared authority.8 However, even under hierarchical models, a more participatory than supervisory leadership can help avoid alienating partners through a pattern of we decide, you carry it out that is often associated with ineffective leadership styles.1314 In fact, this alienating effect on providers in subordinate roles (ie, NPPs and fellows) may have contributed to the negative perception of the team's function on improving patient care.
This study is limited in the following ways. We did not have 100% participation in the Repeated Surveys. Attitudes and experiences of participants in a single comanagement practice are not representative of all comanaging providers. However, the goal of this studyto collect unique survey data from providers themselves to inform an evolving definition of comanagementis modest enough in scope to not require a generalizable sample. Because this study unearthed differences in expectations and experiences within a single site, they may serve as a lower bound for the extent of differences across and within multiple sites. In addition, comanagement enacted for complex medical patients is not as common as the comanagement of surgical patients. Moreover, comanagement models in academic hospitals may have structural features and priorities not found in community settings. Whether or not these disparate models share enough in common to be categorized under a single rubric is a valid question.
Although the teamwork structure and provider roles within comanagement vary, the practice arrangement's preoccupation with quality can be seen as its defining feature. Limited evidence, to date,1, 1519 and the rapid proliferation of the model, suggest that quality and efficiency advantages can be obtained from an effective implementation of comanagement. As in any team‐based care model, a common understanding of roles and expectations are essential to enhancing teamwork. Our interpretation of the mission of comanagement may further enhance teamwork through an explicit articulation of shared goals.
- Medical and surgical comanagement after elective hip and knee arthroplasty: A randomized, controlled trial.Ann Intern Med.2004;141(1):28–38. , , , et al.
- Just because you can, doesn't mean that you should: A call for the rational application of hospitalist comanagement.J Hosp Med.2008;3(5):398–402. .
- Surgical comanagement: A natural evolution of hospitalist practice.J Hosp Med.2008;3(5):394–397. , .
- Comanagement of hospitalized surgical patients by medicine physicians in the United States.Arch Intern Med.2010;170(4):363–368. , , , , .
- Structure and meaning in multidisciplinary teamwork.Sociol Health Illn.1998;20(6):848–873. .
- Human factors and cardiac surgery: A multicenter study.J Thorac Cardiov Surg.2000;119(4):661–670. , , , , .
- The effects of a collaborative model of primary care on the mortality and hospital use of community‐dwelling older adults.J Gerontol A‐Biol.2001;56(2):M106–M112. , , .
- Effects of provider characteristics on care coordination under comanagement.J Hosp Med.2010;5:508–513. , , , , , .
- Crossing the Quality Chasm: A New Health System for the Twenty‐First Century.Washington, DC:Institute of Medicine;2001. , , .
- The emerging role of “hospitalists” in the American health care system.N Engl J Med.1996;335(7):514–517. , .
- Internal medicine comanagement of surgical patients: Can we afford to do this?Arch Intern Med.2010;170(22):1965–1966. .
- Operating room teamwork among physicians and nurses: Teamwork in the eye of the beholder.J Am Coll Surg.2006;202(5):746–752. , , , et al.
- “We decide, you carry it out”: A social network analysis of multidisciplinary longterm care teams.Soc Sci Med.1997;45(9):1411–1421. .
- Patterns of aggressive behavior in experimentally created social climates.J Soc Psychol.1939;10:271–301. , , .
- Comanagement of surgical patients between neurosurgeons and hospitalists.Arch Intern Med.2010;170(22):2004–2010. , , , et al.
- Outcomes for older patients with hip fractures: The impact of orthopedic and geriatric medicine cocare.J Orthop Trauma.2006;20(3):172–180. , , , , , .
- Effects of a hospitalist model on elderly patients with hip fracture.Arch Intern Med.2005;165(7):796–801. , , , et al.
- Hip fractures in geriatric patients. Results of an interdisciplinary hospital care program.Clin Orthop Relat Res.1992(274):213–225. , , , .
- Impact of a comanaged Geriatric Fracture Center on short‐term hip fracture outcomes.Arch Intern Med.2009;169(18):1712–1717. , , , .
- Medical and surgical comanagement after elective hip and knee arthroplasty: A randomized, controlled trial.Ann Intern Med.2004;141(1):28–38. , , , et al.
- Just because you can, doesn't mean that you should: A call for the rational application of hospitalist comanagement.J Hosp Med.2008;3(5):398–402. .
- Surgical comanagement: A natural evolution of hospitalist practice.J Hosp Med.2008;3(5):394–397. , .
- Comanagement of hospitalized surgical patients by medicine physicians in the United States.Arch Intern Med.2010;170(4):363–368. , , , , .
- Structure and meaning in multidisciplinary teamwork.Sociol Health Illn.1998;20(6):848–873. .
- Human factors and cardiac surgery: A multicenter study.J Thorac Cardiov Surg.2000;119(4):661–670. , , , , .
- The effects of a collaborative model of primary care on the mortality and hospital use of community‐dwelling older adults.J Gerontol A‐Biol.2001;56(2):M106–M112. , , .
- Effects of provider characteristics on care coordination under comanagement.J Hosp Med.2010;5:508–513. , , , , , .
- Crossing the Quality Chasm: A New Health System for the Twenty‐First Century.Washington, DC:Institute of Medicine;2001. , , .
- The emerging role of “hospitalists” in the American health care system.N Engl J Med.1996;335(7):514–517. , .
- Internal medicine comanagement of surgical patients: Can we afford to do this?Arch Intern Med.2010;170(22):1965–1966. .
- Operating room teamwork among physicians and nurses: Teamwork in the eye of the beholder.J Am Coll Surg.2006;202(5):746–752. , , , et al.
- “We decide, you carry it out”: A social network analysis of multidisciplinary longterm care teams.Soc Sci Med.1997;45(9):1411–1421. .
- Patterns of aggressive behavior in experimentally created social climates.J Soc Psychol.1939;10:271–301. , , .
- Comanagement of surgical patients between neurosurgeons and hospitalists.Arch Intern Med.2010;170(22):2004–2010. , , , et al.
- Outcomes for older patients with hip fractures: The impact of orthopedic and geriatric medicine cocare.J Orthop Trauma.2006;20(3):172–180. , , , , , .
- Effects of a hospitalist model on elderly patients with hip fracture.Arch Intern Med.2005;165(7):796–801. , , , et al.
- Hip fractures in geriatric patients. Results of an interdisciplinary hospital care program.Clin Orthop Relat Res.1992(274):213–225. , , , .
- Impact of a comanaged Geriatric Fracture Center on short‐term hip fracture outcomes.Arch Intern Med.2009;169(18):1712–1717. , , , .
Copyright © 2011 Society of Hospital Medicine
Care Coordination Under Comanagement
Technological advances drive medical providers to specialize through the need for proficiency around increasingly focused areas of expertise.1 But the benefits of specialization are attained only by balancing the advantages of increasing expertise and the costs of coordinating care that must be borne as specialization increases.2 Integrating experts into modern medical delivery systems requires attention to the coordinating mechanisms that govern team‐based care.3
Coordination, defined as the management of task interdependencies,4 is a central component and a useful measure of teamwork.5 Several studies demonstrate the patient‐level impact of coordination among providers.69 Gittell et al.8 demonstrated that orthopedic hospitals whose staff had better relational coordination (RC) measures had shorter lengths of stay and better post‐operative pain control for patients undergoing surgery. In medical intensive care units (ICUs), Wheelan et al.9 showed that staff members of units with lower mortality rates perceived their teams as functioning at higher stages of group development and perceived their team members as less dependent and more trusting.
Communication is the cornerstone of effective team coordination.10, 11 As such, practice model interventions that facilitate frequent communication of higher quality are associated with lower error rates10 and better teamwork.11 The use of hospitalists, for example, is shown to capitalize on this advantage by improving coordination through physician availability that facilitates communication and relational interactions among hospital‐based staff.12 While system‐level interventions such as this have received significant attention from experts in organizations, empirical studies that explore the contribution of team member characteristics to overall coordination are lacking.13
Inpatient comanagement services offer a unique model for studying teamwork. While the label is used to describe a variety of arrangements,1416 comanagement broadly describes a practice model wherein providers of various specialties deliver direct care to patients, in contrast to the traditional generalist‐consultant model in which specialists lend expertise.17 Many recent comanagement practices involve hospitalists in partnership with surgeons in the care of patients with concurrent medical and surgical needs,18 but similar arrangements between hospitalists and medical subspecialists are being adopted in some medical centers for the care of complex patients with conditions such as heart failure, cancer, stroke, and solid organ transplantations. Coordination among providers has not been studied in this context.
The goals of this study are: (1) to measure the input of individual providers to the overall coordination of care on a highly interdependent medical comanagement service, (2) to characterize high and low coordinators, and (3) to explore the relationship between coordination and patient outcomes. The main hypothesis is that the quality of team coordination is determined partly by the attributes of its members such that their individual contributions to the coordination of care affect the outcomes of vulnerable hospitalized patients.
Materials and Methods
Setting
The study was conducted at the University of Chicago Medical Center, Chicago, IL, an urban 572‐bed tertiary care hospital. The comanaged multidisciplinary inpatient service serves hospitalized patients with complex medical needs. This study focused on providers and patients from a subset of the comanaged multidisciplinary inpatient service that involved the collaboration of medical hepatologists with hospitalists. A hepatology team, composed of an attending hepatologist and a fellow, comanaged with 2 hospitalist teams, each composed of an attending hospitalist and 1 or 2 nonphysician providers (NPPs). Attending physicians rotated on the service in 1‐week to 3‐week rotations, while fellows rotated in 4‐week stretches. NPPs worked nonuniform 3‐day or 4‐day weeks excluding weekends and holidays. The hepatology team was responsible for arranging admissions, developing a care plan with a specialty focus, coordinating care with transplant surgeons when necessary, and managing post‐discharge care. The hospitalist teams were responsible for admitting patients, managing routine and emergent inpatient issues, coordinating care with ancillary and consultative staff, and discharging patients. Dedicated evening and night hospitalists, who were not part of the comanaging day‐time teams, provided after‐hours care. Outside of these areas, there was no instruction or education about how responsibilities should be shared among providers on the service.
Subjects and Study Design
Baseline Survey of Providers
All hospitalists, NPPs, hepatologists, and fellows scheduled to rotate on the comanaged multidisciplinary inpatient service signed a written consent to participate. In April 2008 a nonanonymous baseline 17‐item paper survey was administered.
Items of the Baseline Survey (supporting information Appendix A) were generated from a consideration of the most salient issues around the management structure of comanagement models from a comprehensive review the literature. Two items addressed the respondents' experience and intent to leave their role. Twelve items addressed their preferences about the provider management structure of an ideally comanaged inpatient service, specifically soliciting their preferences about a single physician leader, consensus seeking, and their preferred degree of information, participation, and decision making under the model. Included in this set of items was a single item assessment of the provider's sense of patient ownership on an ideally comanaged service. The final 3 items addressed the perceived assignment of responsibilities. Each of these items presented a clinical objective followed by up to 7 contingent tasks on whose completion the successful execution of the objective depended. Each respondent was asked to indicate one or more of the 4 provider types that should be responsible for completing each task.
Repeated Survey of Providers
From April to October 2008, providers who rotated on the comanaged liver service were surveyed repeatedly to give information about the actual management structure and coordination within teams, which consisted of combinations of randomly assigned providers. Physicians were surveyed on the day when any 1 of the 3 physician types ended his or her rotation. NPPs were surveyed every Wednesday except on the weeks when none of the physicians had changed since the previous survey. One investigator (KH) hand‐delivered the surveys, usually during the first minutes of the joint daily rounds and collected them immediately upon completion. Surveys that could not be completed immediately were collected on daily rounds on subsequent days within 1 week. The primary reason for nonresponse was lost surveys that were not immediately completed.
The 14‐item Repeated Survey (supporting information Appendix B) consisted of 2 parts. The first 7 items reprised items from the Baseline Survey that addressed management structures, but were rephrased to allow respondents to report their experiences on their immediate rotation. The second part of the Repeated Survey addressed RC, which is described below.
The study protocols, consents, and data collection mechanisms were approved by the institutional review board of the University of Chicago Medical Center. Collection of patient information was designed to comply with the Health Insurance Portability and Accountability Act of 1996.
Patients
Patients were admitted to 1 of the 2 hospitalist teams on the comanaged service on alternating days, which allowed patients to be assigned to providers pseudo‐randomly. Consent to use clinical data was obtained during their stay or by telephone after discharge. If patients were unable to provide consent due to cognitive impairment, consent was sought through designated proxies.19
Main Measurements
Relational Care Coordination
The survey instrument used to measure individual contributions to overall coordination was adapted from the Relational Coordination tool developed by Gittell.20 This instrument was chosen because it has already been validated in various clinical contexts8, 12, 21 and the theoretical assumptions about the independent relational and communication components of coordination are applicable to our context. RC is characterized by the 7 domains of frequent, timely, accurate, and problem‐solving communications; shared goals, shared knowledge, and mutual respect. Respondents rated, on a 5‐point scale (1 = negative, 5 = positive), team members of the other 3 provider types during each rotation on all of the 7 domains. The mean across the domains yielded the RC score. Although the instrument was originally developed to measure the coordination in groups of individuals, the RC for a single provider was calculated by taking the mean of all the RC directed at that individual across team members who worked with him or her during the study period. Because some providers worked more rotations than others, a nonuniform number of observations contributed to the calculation of individual RC (Table 1). For each provider type, individuals were ranked on their RC and categorized in tertiles representing high, middle, and low coordinators.
Baseline Survey (%) | Repeated Surveys (%) | % Female | Years Experience Median (range) | # RC Evaluations of Each Provider Median (range) | RC Mean (range) | |
---|---|---|---|---|---|---|
| ||||||
Hospitalists | 15/15 (100) | 36/43 (84) | 42 | 1 (0‐10) | 6 (3‐21) | 4.71 (4.33‐4.94) |
NPPs | 5/5 (100) | 92/97 (95) | 100 | 4 (2‐15) | 30 (23‐34) | 4.60 (4.48‐4.71) |
Hepatologists | 6/6 (100) | 26/42 (62) | 33 | 7 (1‐25) | 16 (5‐51) | 4.37 (4.03‐4.59) |
GI fellows | 6/6 (100) | 23/42 (55) | 48 | 1 (0‐1) | 19 (8‐37) | 4.28 (3.88‐4.53) |
Total | 32/32 (100) | 177/223 (79) | 55 | 2 (0‐25) | 12.5 (3‐51) | 4.57 (3.88‐4.94) |
Statistical Analysis
The discriminating ability of the RC for individuals was assessed by comparing the highest and lowest RC of each provider type using the 2‐tailed t‐test. The difference in responses to items from the Baseline and Repeated Surveys by individual RC tertiles was assessed with the Chi‐squared test for categorical data and the 2‐tailed t‐test for comparing means. For each physician type, the frequency of the composite bad outcomes between the highest and lowest RC tertile categories were compared using a 2‐sample Wilcoxon rank‐sum (Mann‐Whitney) test for nonparametric data.
Results
All 32 providers (100%) completed the Baseline Survey and participated in the Repeated Surveys of which 177/224 (79%) were completed. The median number of surveys that contributed to the calculation of individual RC and the mean RC by provider type are summarized in Table 1.
Of the 119 patients managed on the service, the mean age (standard deviation [SD]) was 55 (14) years and 48% were women. Of the 201 hospitalizations, there were 13 floor‐to‐ICU transfers and 5 in‐hospital deaths, however, we excluded from the analysis 1 death of a patient who was admitted under inpatient hospice status.
RC Measures
Individual provider RC ranges were 4.33 to 4.94 (p = 0.05) for hospitalists; 4.48 to 4.71 (p = 0.10) for NP/PAs; 4.03 to 4.59 (p < 0.01) for hepatologists; and 3.88 to 4.52 (p = 0.02) for fellows. The high, middle, and low coordinator categories for each provider type were shown to be durable through time by demonstrating that the coordination ranking of individuals was essentially preserved even when using partial data from each half of the study period. Thus, RC appears to reflect a stable attribute of the provider as opposed to specific circumstances of the rotation. The categories were shown to be durable to the influences of bad outcomes (inpatient deaths and ICU transfers) by demonstrating that the placement of individuals into 1 of the 3 coordination categories were preserved even when data from rotations involving a bad outcome were removed. Nonetheless, in order to address the possibility of bad outcomes negatively affecting perception of coordination, all analysis involving RC used the values that excluded data from these rotations.
Characteristics of Good and Poor Coordinators
Patient Ownership
The single‐item measure of patient ownership in the Baseline Survey reads: I have as much a sense of ownership of my patients on the comanaged service as on a non‐comanaged service. The majority of providers of every type in the high and middle coordinator categories agreed, while providers in the low coordinator category generally disagreed with the statement. The aggregated responses of all the provider types are shown in Table 2.
Agree | Somewhat Agree | Somewhat Disagree | Disagree | |
---|---|---|---|---|
High | 4 | 6 | 0 | 1 |
Middle | 5 | 4 | 0 | 2 |
Low | 2 | 0 | 4 | 4 |
p < 0.01 |
Leadership
Hepatologists are the potential leader of the comanaged team because of their content expertise in liver diseases. Their responses to the 3 items in the Baseline Survey that addressed perceived assignment of responsibilities are shown in Table 3. The high compared to the low coordinator hepatologists delegated the responsibility of completing necessary tasks to more providers, overall, such that an average of 3 providers were redundantly held responsible for the completion of each task by the high coordinators while only 1 provider was held responsible by the low coordinators. Furthermore, the high coordinators delegated the responsibility of completing more tasks to themselves compared to the low coordinators.
Hepatologists | Mean # of Tasks Delegated Overall, n (SD) | Mean # of Providers Delegated to Each Task, n (SD) | Mean # of Tasks Delegated to Self, n (SD) |
---|---|---|---|
| |||
High (n = 2) | 56 (0.0) | 2.9 (0.0) | 11.5 (2.1) |
Middle (n = 2) | 35 (2.8) | 1.8 (0.2) | 9.5 (3.5) |
Low (n = 2) | 19 (1.4) | 1.0 (0.1) | 4.5 (2.1) |
p value(high vs. low) | <0.01 | <0.01 | 0.08 |
According to responses to the management structure items of the Repeated Surveys, more providers of every type indicated that a single physician leader directed the overall management of every patient when a high or middle coordinator hospitalist was on service as opposed to a service with a low coordinator hospitalist (high 76% vs. middle 73% vs. low 58%, P = 0.06). Furthermore, a low coordinator hospitalist on service was more likely to indicate a desire for greater influence in directing the management of patients (desire influence 93% vs. not 7%, P < 0.01). This pattern was also seen with low coordinator NPPs, who more often indicated a desire for greater influence in directing patient management (desire influence 100% vs. not 0%, P < 0.01).
Experience
Age, years in practice, years at the institution, and time spent on the comanaged service were not associated with RC in our small sample of providers.
Outcomes by Provider Coordination
The unit of analysis in this section is the team‐patient encounter, which is the consecutive days during which a unique assortment of physicians managed a patient's hospitalization. NPPs could not be associated with any single team due to their nonuniform work patterns. The 201 hospitalizations in this study were composed of 351 team‐patient encounters. Table 4 displays the unadjusted frequency of inpatient deaths and ICU transfers that occurred during these encounters by RC tertiles. In each of the 3 physician types, composite bad outcomes are most frequent among the lowest coordinators. The pattern is statistically significant for hospitalists.
Team‐Patient Encounters, n | Mean Length of Encounter, n (days) | ICU Transfer, n (%) | Hospital Death, n (%) | Bad Outcome, n (%) | |
---|---|---|---|---|---|
| |||||
Hospitalists | |||||
High (n = 5) | 92 | 3.1 | 1 (1.1) | 1 (1.1) | 1 (1.1) |
Middle (n = 5) | 119 | 3.2 | 1 (0.8) | 1 (0.8) | 1 (0.8) |
Low (n = 5) | 140 | 3.2 | 11 (7.9) | 2 (1.4) | 12 (8.6) |
p value (high vs. low) | NA | 0.70 | 0.02 | 0.82 | 0.02 |
Hepatologists | |||||
High (n = 2) | 99 | 3.2 | (2.0) | 0 (0.0) | 2 (2.0) |
Middle (n = 2) | 79 | 3.4 | 3 (3.0) | 1 (1.3) | 3 (3.0) |
Low (n = 2) | 173 | 3.0 | 8 (4.6) | 3 (1.7) | 9 (5.2) |
p value (high vs. low) | NA | 0.52 | 0.27 | 0.19 | 0.20 |
GI fellows | |||||
High (n = 2) | 111 | 3.1 | 2 (1.8) | 0 (0.0) | 2 (1.8) |
Middle (n = 2) | 67 | 3.3 | 2 (3.0) | 1 (1.5) | 2 (3.0) |
Low (n = 2) | 173 | 3.2 | 9 (5.2) | 3 (1.7) | 10 (5.8) |
p value (high vs. low) | NA | 0.74 | 0.15 | 0.16 | 0.10 |
Another interesting observation is the largest number of encounters in the lowest coordination tertile of each physician type. While the reason for this finding is not clear, associations between work‐load and poor coordination evoke issues related to burnout. In order to address the possibility of an artifactually elevated probability of a bad outcome among providers who rotated through the service more often, we calculated the correlation between the number of encounter‐days and the frequency of bad outcomes for the 15 providers who were associated with at last one such event. If these events occurred by chance, we should find a positive correlation between its frequency and the number of encounters. The Pearson's correlation coefficient of 0.38 suggests that bad outcomes do not occur more frequently with providers who work more rotations by chance alone.
Discussion
By adapting Gittell's RC instrument to focus on individual providers, we found that their characteristic attributes such as preference for particular management styles, leadership quality, and patient ownership are associated with their externally perceived contributions to the overall coordination of care. In an unadjusted analysis, we also observed an intriguing trend towards more frequent major hospital complications when the worst coordinators of each physician type were on service.
Existing evidence22, 23 mostly summarized in a recent RAND Health report shows a weak association between clinical teamwork quality and patient mortality. While our data also support this association, it does so with limitations. Most importantly, the small sample size limited our ability to rigorously account for potential confounders that may have contributed to this apparent association. Further studies may better address whether or not bad outcomes are indeed associated with poor coordinators in highly interdependent clinical teams. In addition to confounding, the small sample size of providers makes the analysis vulnerable to type 1 errors. We addressed this issue by intensively surveying providers repeatedly to achieve a high resolution of the coordination and management structure measures from each comanaged team. The potential for omitted variables and reverse causality in that the coordination scores may be negatively influenced by particularly complex patients and bad outcomes remains a valid concern. We addressed this by confirming the stability of provider RC over time and excluding the RC data from rotations with a bad outcome, but the negative perception of an individual tied to past bad outcomes may persist beyond a particular rotation. Survey responses are subject to recall and hindsight biases, which we attempted to minimize by surveying respondents immediately after each team rotation. Finally, all of our findings may be not be generalizable to other comanagement settings. However, the important correlations between coordination and quality have been observed in other contexts.24, 25
In our study, in‐hospital deaths and ICU transfers are treated as consequences of uncoordinated care. This interpretation may be problematic for circumstances when death is inevitable no matter how well coordinated the care, or when transfer to a higher level of care is appropriate. The rationale for grouping the 2 events into 1 composite bad outcome is based on the assumption that both death and the escalation of care can be delayed to an extent, if not wholly prevented, with the coordinated utilization of a modern hospital's resources. The attribution of these events to poor coordinators may indicate the unraveling of coordination that normally must be maintained to help patients overcome decompensating events that are particularly common in the course of patients with severe liver diseases. Due to the exploratory nature of this analysis, additional studies are necessary to fully characterize the relationship between care coordination and care transfers.
An important implication of this study is that the communication skill and ethical disposition of each individual provider is relevant to the coordination that is sought in multi‐provider teams. Training medical professionals to be better team members may have direct impact on the patients they serve. Our finding about patient ownership suggests that commitment to patients in the framework of care is not merely tradition but a characteristic of competent physicians. Moreover, physicians' commitment to patients is a possible factor, not just in achieving patients' satisfaction, but in securing better outcomes. To that end, the teaching of this and other humanistic principles must remain a vital part of medical education at all levels of training.
Several implications about team leadership and hierarchy are apparent from the data. Findings around the perceived assignment of responsibilities show that high coordinator hepatologists acknowledge the advantages of overlapping task boundaries to prevent critical tasks from being missed and risking bad outcomes. High RC hepatologists in our study adopted a more participatory than supervisory role which presumably facilitated better coordination by transmitting organizational goals to other team members. The function of a comanaged team is likely to be enhanced by a fluid assignment of roles to better handle tasks with high uncertainty. Accordingly, comanagement models of care may not be appropriate in settings where tasks are not interdependent.26 Inherent hierarchy appears to be a feature of well coordinated teams. One possible interpretation of our data is that hospitalists who yield the leadership role to the hepatologist are perceived to be better coordinators and that those who insist on exerting more influence in team decisions are perceived to be poor coordinators.
Existing evidence around care coordination predicts that comanagement designs improve provider coordination through stage‐based and site‐based specialization.12 However, the mechanisms that mediate coordination and patient outcomes are not clear. Moreover, the mechanisms of coordinating multi‐disciplinary teams may be specific to each clinical setting. The role of individual provider characteristics on coordination deserves more attention. Similarly, the impact of organizational culture under which favorable provider characteristics thrive is unknown. Finally, a detailed exposition of patient ownership and the role patients play in affecting the coordination of healthcare resources needs further exploration.
- Technology, specialization, and the allied health professions.J Allied Health.1983;12(3):177–182. .
- Hospitalists and the doctor‐patient relationship.J Legal Stud.2001;30:589–606. .
- From Chaos to Care: The Promise of Team‐Based medicine.Cambridge, MA:Perseus;2002. .
- Determinants of coordination modes within organizations.Am Sociol Rev.1976;41:322–338. , , .
- Teamwork and patient safety in dynamic domains of healthcare: a review of the literature.Acta Anaesthesiol Scand.2009;53(2):143–151. .
- The association between interdisciplinary collaboration and patient outcomes in a medical intensive care unit.Heart Lung.1992;21:18–24. , , , , .
- Patterns of coordination and clinical outcomes: a study of surgical services.Health Serv Res.1998;22:1211–1236. , , , et al.
- Impact of relational coordination on quality of care, postoperative pain and functioning, and length of stay: a nine‐hospital study of surgical patients.Med Care.2000;38(8):807–819. , , , et al.
- The link between teamwork and patients' outcomes in intensive care units.Am J Crit Care.2003;12(6):527–534. , , .
- Assessing system failures in operating rooms and intensive care units.Qual Saf health Care.2007;16:45–50. , , .
- Patient handover from surgery to intensive care: using Formula 1 pit‐stop and aviation models to improve safety and quality.Paediatr Anaesth.2007;17(5):470–478. , , , et al.
- Is the doctor in? A relational approach to job design and the coordination of work.Hum Resour Manage.2008;47(4):729–755. , , , .
- Developing a team performance framework for the intensive care unit.Crit Care Med.2009;37(5):1787–1793. , , , .
- Medical and surgical comanagement after elective hip and knee arthroplasty: a randomized, controlled trial.Ann Intern Med.2004;141(1):28–38. , , , et al.
- Medical/psychiatric comanagement by nurse practitioners in chronic hepatitis C treatment: a case study.Arch Psychiatr Nurs.2007;21(2):87–90. , , .
- Perioperative medicine for the hospitalized patient.Med Clin North Am.2008;92(2):325–348. , .
- The place and training of the general practitioner.Calif Med.1949;70(4):265–268. .
- Surgical comanagement: a natural evolution of hospitalist practice.J Hosp Med.2008;3(5):394–397. , .
- Validation of a telephone version of the mini‐mental state examination.J Am Geriatr Soc.1992;40(7):697–702. , , , .
- Organizing work to support relational co‐ordination.Int J of Human Resource Management.2000;11(3):517–539. .
- Beyond our walls: impact of patient and provider coordination across the continuum on outcomes for surgical patients.Health Serv Res.2007;42:7–24. , , , , .
- The effect of multidisciplinary care teams on intensive care unit mortality.Arch Intern Med.2010;170(4):369–376. , , , , .
- Outcome measures for effective teamwork in inpatient care: final report.Santa Monica:RAND Health;2008. , , , .
- The performance of intensive care units: does good management make a difference?Med Care.1994;32(5):508–525. , , , et al.
- Observational assessment of surgical teamwork: a feasibility study.World J Surg.2006;30(10):1774–1783. , , , .
- Just because you can, doesn't mean that you should: A call for the rational application of hospitalist comanagement.J Hosp Med.2008;3(5):398–3402. .
Technological advances drive medical providers to specialize through the need for proficiency around increasingly focused areas of expertise.1 But the benefits of specialization are attained only by balancing the advantages of increasing expertise and the costs of coordinating care that must be borne as specialization increases.2 Integrating experts into modern medical delivery systems requires attention to the coordinating mechanisms that govern team‐based care.3
Coordination, defined as the management of task interdependencies,4 is a central component and a useful measure of teamwork.5 Several studies demonstrate the patient‐level impact of coordination among providers.69 Gittell et al.8 demonstrated that orthopedic hospitals whose staff had better relational coordination (RC) measures had shorter lengths of stay and better post‐operative pain control for patients undergoing surgery. In medical intensive care units (ICUs), Wheelan et al.9 showed that staff members of units with lower mortality rates perceived their teams as functioning at higher stages of group development and perceived their team members as less dependent and more trusting.
Communication is the cornerstone of effective team coordination.10, 11 As such, practice model interventions that facilitate frequent communication of higher quality are associated with lower error rates10 and better teamwork.11 The use of hospitalists, for example, is shown to capitalize on this advantage by improving coordination through physician availability that facilitates communication and relational interactions among hospital‐based staff.12 While system‐level interventions such as this have received significant attention from experts in organizations, empirical studies that explore the contribution of team member characteristics to overall coordination are lacking.13
Inpatient comanagement services offer a unique model for studying teamwork. While the label is used to describe a variety of arrangements,1416 comanagement broadly describes a practice model wherein providers of various specialties deliver direct care to patients, in contrast to the traditional generalist‐consultant model in which specialists lend expertise.17 Many recent comanagement practices involve hospitalists in partnership with surgeons in the care of patients with concurrent medical and surgical needs,18 but similar arrangements between hospitalists and medical subspecialists are being adopted in some medical centers for the care of complex patients with conditions such as heart failure, cancer, stroke, and solid organ transplantations. Coordination among providers has not been studied in this context.
The goals of this study are: (1) to measure the input of individual providers to the overall coordination of care on a highly interdependent medical comanagement service, (2) to characterize high and low coordinators, and (3) to explore the relationship between coordination and patient outcomes. The main hypothesis is that the quality of team coordination is determined partly by the attributes of its members such that their individual contributions to the coordination of care affect the outcomes of vulnerable hospitalized patients.
Materials and Methods
Setting
The study was conducted at the University of Chicago Medical Center, Chicago, IL, an urban 572‐bed tertiary care hospital. The comanaged multidisciplinary inpatient service serves hospitalized patients with complex medical needs. This study focused on providers and patients from a subset of the comanaged multidisciplinary inpatient service that involved the collaboration of medical hepatologists with hospitalists. A hepatology team, composed of an attending hepatologist and a fellow, comanaged with 2 hospitalist teams, each composed of an attending hospitalist and 1 or 2 nonphysician providers (NPPs). Attending physicians rotated on the service in 1‐week to 3‐week rotations, while fellows rotated in 4‐week stretches. NPPs worked nonuniform 3‐day or 4‐day weeks excluding weekends and holidays. The hepatology team was responsible for arranging admissions, developing a care plan with a specialty focus, coordinating care with transplant surgeons when necessary, and managing post‐discharge care. The hospitalist teams were responsible for admitting patients, managing routine and emergent inpatient issues, coordinating care with ancillary and consultative staff, and discharging patients. Dedicated evening and night hospitalists, who were not part of the comanaging day‐time teams, provided after‐hours care. Outside of these areas, there was no instruction or education about how responsibilities should be shared among providers on the service.
Subjects and Study Design
Baseline Survey of Providers
All hospitalists, NPPs, hepatologists, and fellows scheduled to rotate on the comanaged multidisciplinary inpatient service signed a written consent to participate. In April 2008 a nonanonymous baseline 17‐item paper survey was administered.
Items of the Baseline Survey (supporting information Appendix A) were generated from a consideration of the most salient issues around the management structure of comanagement models from a comprehensive review the literature. Two items addressed the respondents' experience and intent to leave their role. Twelve items addressed their preferences about the provider management structure of an ideally comanaged inpatient service, specifically soliciting their preferences about a single physician leader, consensus seeking, and their preferred degree of information, participation, and decision making under the model. Included in this set of items was a single item assessment of the provider's sense of patient ownership on an ideally comanaged service. The final 3 items addressed the perceived assignment of responsibilities. Each of these items presented a clinical objective followed by up to 7 contingent tasks on whose completion the successful execution of the objective depended. Each respondent was asked to indicate one or more of the 4 provider types that should be responsible for completing each task.
Repeated Survey of Providers
From April to October 2008, providers who rotated on the comanaged liver service were surveyed repeatedly to give information about the actual management structure and coordination within teams, which consisted of combinations of randomly assigned providers. Physicians were surveyed on the day when any 1 of the 3 physician types ended his or her rotation. NPPs were surveyed every Wednesday except on the weeks when none of the physicians had changed since the previous survey. One investigator (KH) hand‐delivered the surveys, usually during the first minutes of the joint daily rounds and collected them immediately upon completion. Surveys that could not be completed immediately were collected on daily rounds on subsequent days within 1 week. The primary reason for nonresponse was lost surveys that were not immediately completed.
The 14‐item Repeated Survey (supporting information Appendix B) consisted of 2 parts. The first 7 items reprised items from the Baseline Survey that addressed management structures, but were rephrased to allow respondents to report their experiences on their immediate rotation. The second part of the Repeated Survey addressed RC, which is described below.
The study protocols, consents, and data collection mechanisms were approved by the institutional review board of the University of Chicago Medical Center. Collection of patient information was designed to comply with the Health Insurance Portability and Accountability Act of 1996.
Patients
Patients were admitted to 1 of the 2 hospitalist teams on the comanaged service on alternating days, which allowed patients to be assigned to providers pseudo‐randomly. Consent to use clinical data was obtained during their stay or by telephone after discharge. If patients were unable to provide consent due to cognitive impairment, consent was sought through designated proxies.19
Main Measurements
Relational Care Coordination
The survey instrument used to measure individual contributions to overall coordination was adapted from the Relational Coordination tool developed by Gittell.20 This instrument was chosen because it has already been validated in various clinical contexts8, 12, 21 and the theoretical assumptions about the independent relational and communication components of coordination are applicable to our context. RC is characterized by the 7 domains of frequent, timely, accurate, and problem‐solving communications; shared goals, shared knowledge, and mutual respect. Respondents rated, on a 5‐point scale (1 = negative, 5 = positive), team members of the other 3 provider types during each rotation on all of the 7 domains. The mean across the domains yielded the RC score. Although the instrument was originally developed to measure the coordination in groups of individuals, the RC for a single provider was calculated by taking the mean of all the RC directed at that individual across team members who worked with him or her during the study period. Because some providers worked more rotations than others, a nonuniform number of observations contributed to the calculation of individual RC (Table 1). For each provider type, individuals were ranked on their RC and categorized in tertiles representing high, middle, and low coordinators.
Baseline Survey (%) | Repeated Surveys (%) | % Female | Years Experience Median (range) | # RC Evaluations of Each Provider Median (range) | RC Mean (range) | |
---|---|---|---|---|---|---|
| ||||||
Hospitalists | 15/15 (100) | 36/43 (84) | 42 | 1 (0‐10) | 6 (3‐21) | 4.71 (4.33‐4.94) |
NPPs | 5/5 (100) | 92/97 (95) | 100 | 4 (2‐15) | 30 (23‐34) | 4.60 (4.48‐4.71) |
Hepatologists | 6/6 (100) | 26/42 (62) | 33 | 7 (1‐25) | 16 (5‐51) | 4.37 (4.03‐4.59) |
GI fellows | 6/6 (100) | 23/42 (55) | 48 | 1 (0‐1) | 19 (8‐37) | 4.28 (3.88‐4.53) |
Total | 32/32 (100) | 177/223 (79) | 55 | 2 (0‐25) | 12.5 (3‐51) | 4.57 (3.88‐4.94) |
Statistical Analysis
The discriminating ability of the RC for individuals was assessed by comparing the highest and lowest RC of each provider type using the 2‐tailed t‐test. The difference in responses to items from the Baseline and Repeated Surveys by individual RC tertiles was assessed with the Chi‐squared test for categorical data and the 2‐tailed t‐test for comparing means. For each physician type, the frequency of the composite bad outcomes between the highest and lowest RC tertile categories were compared using a 2‐sample Wilcoxon rank‐sum (Mann‐Whitney) test for nonparametric data.
Results
All 32 providers (100%) completed the Baseline Survey and participated in the Repeated Surveys of which 177/224 (79%) were completed. The median number of surveys that contributed to the calculation of individual RC and the mean RC by provider type are summarized in Table 1.
Of the 119 patients managed on the service, the mean age (standard deviation [SD]) was 55 (14) years and 48% were women. Of the 201 hospitalizations, there were 13 floor‐to‐ICU transfers and 5 in‐hospital deaths, however, we excluded from the analysis 1 death of a patient who was admitted under inpatient hospice status.
RC Measures
Individual provider RC ranges were 4.33 to 4.94 (p = 0.05) for hospitalists; 4.48 to 4.71 (p = 0.10) for NP/PAs; 4.03 to 4.59 (p < 0.01) for hepatologists; and 3.88 to 4.52 (p = 0.02) for fellows. The high, middle, and low coordinator categories for each provider type were shown to be durable through time by demonstrating that the coordination ranking of individuals was essentially preserved even when using partial data from each half of the study period. Thus, RC appears to reflect a stable attribute of the provider as opposed to specific circumstances of the rotation. The categories were shown to be durable to the influences of bad outcomes (inpatient deaths and ICU transfers) by demonstrating that the placement of individuals into 1 of the 3 coordination categories were preserved even when data from rotations involving a bad outcome were removed. Nonetheless, in order to address the possibility of bad outcomes negatively affecting perception of coordination, all analysis involving RC used the values that excluded data from these rotations.
Characteristics of Good and Poor Coordinators
Patient Ownership
The single‐item measure of patient ownership in the Baseline Survey reads: I have as much a sense of ownership of my patients on the comanaged service as on a non‐comanaged service. The majority of providers of every type in the high and middle coordinator categories agreed, while providers in the low coordinator category generally disagreed with the statement. The aggregated responses of all the provider types are shown in Table 2.
Agree | Somewhat Agree | Somewhat Disagree | Disagree | |
---|---|---|---|---|
High | 4 | 6 | 0 | 1 |
Middle | 5 | 4 | 0 | 2 |
Low | 2 | 0 | 4 | 4 |
p < 0.01 |
Leadership
Hepatologists are the potential leader of the comanaged team because of their content expertise in liver diseases. Their responses to the 3 items in the Baseline Survey that addressed perceived assignment of responsibilities are shown in Table 3. The high compared to the low coordinator hepatologists delegated the responsibility of completing necessary tasks to more providers, overall, such that an average of 3 providers were redundantly held responsible for the completion of each task by the high coordinators while only 1 provider was held responsible by the low coordinators. Furthermore, the high coordinators delegated the responsibility of completing more tasks to themselves compared to the low coordinators.
Hepatologists | Mean # of Tasks Delegated Overall, n (SD) | Mean # of Providers Delegated to Each Task, n (SD) | Mean # of Tasks Delegated to Self, n (SD) |
---|---|---|---|
| |||
High (n = 2) | 56 (0.0) | 2.9 (0.0) | 11.5 (2.1) |
Middle (n = 2) | 35 (2.8) | 1.8 (0.2) | 9.5 (3.5) |
Low (n = 2) | 19 (1.4) | 1.0 (0.1) | 4.5 (2.1) |
p value(high vs. low) | <0.01 | <0.01 | 0.08 |
According to responses to the management structure items of the Repeated Surveys, more providers of every type indicated that a single physician leader directed the overall management of every patient when a high or middle coordinator hospitalist was on service as opposed to a service with a low coordinator hospitalist (high 76% vs. middle 73% vs. low 58%, P = 0.06). Furthermore, a low coordinator hospitalist on service was more likely to indicate a desire for greater influence in directing the management of patients (desire influence 93% vs. not 7%, P < 0.01). This pattern was also seen with low coordinator NPPs, who more often indicated a desire for greater influence in directing patient management (desire influence 100% vs. not 0%, P < 0.01).
Experience
Age, years in practice, years at the institution, and time spent on the comanaged service were not associated with RC in our small sample of providers.
Outcomes by Provider Coordination
The unit of analysis in this section is the team‐patient encounter, which is the consecutive days during which a unique assortment of physicians managed a patient's hospitalization. NPPs could not be associated with any single team due to their nonuniform work patterns. The 201 hospitalizations in this study were composed of 351 team‐patient encounters. Table 4 displays the unadjusted frequency of inpatient deaths and ICU transfers that occurred during these encounters by RC tertiles. In each of the 3 physician types, composite bad outcomes are most frequent among the lowest coordinators. The pattern is statistically significant for hospitalists.
Team‐Patient Encounters, n | Mean Length of Encounter, n (days) | ICU Transfer, n (%) | Hospital Death, n (%) | Bad Outcome, n (%) | |
---|---|---|---|---|---|
| |||||
Hospitalists | |||||
High (n = 5) | 92 | 3.1 | 1 (1.1) | 1 (1.1) | 1 (1.1) |
Middle (n = 5) | 119 | 3.2 | 1 (0.8) | 1 (0.8) | 1 (0.8) |
Low (n = 5) | 140 | 3.2 | 11 (7.9) | 2 (1.4) | 12 (8.6) |
p value (high vs. low) | NA | 0.70 | 0.02 | 0.82 | 0.02 |
Hepatologists | |||||
High (n = 2) | 99 | 3.2 | (2.0) | 0 (0.0) | 2 (2.0) |
Middle (n = 2) | 79 | 3.4 | 3 (3.0) | 1 (1.3) | 3 (3.0) |
Low (n = 2) | 173 | 3.0 | 8 (4.6) | 3 (1.7) | 9 (5.2) |
p value (high vs. low) | NA | 0.52 | 0.27 | 0.19 | 0.20 |
GI fellows | |||||
High (n = 2) | 111 | 3.1 | 2 (1.8) | 0 (0.0) | 2 (1.8) |
Middle (n = 2) | 67 | 3.3 | 2 (3.0) | 1 (1.5) | 2 (3.0) |
Low (n = 2) | 173 | 3.2 | 9 (5.2) | 3 (1.7) | 10 (5.8) |
p value (high vs. low) | NA | 0.74 | 0.15 | 0.16 | 0.10 |
Another interesting observation is the largest number of encounters in the lowest coordination tertile of each physician type. While the reason for this finding is not clear, associations between work‐load and poor coordination evoke issues related to burnout. In order to address the possibility of an artifactually elevated probability of a bad outcome among providers who rotated through the service more often, we calculated the correlation between the number of encounter‐days and the frequency of bad outcomes for the 15 providers who were associated with at last one such event. If these events occurred by chance, we should find a positive correlation between its frequency and the number of encounters. The Pearson's correlation coefficient of 0.38 suggests that bad outcomes do not occur more frequently with providers who work more rotations by chance alone.
Discussion
By adapting Gittell's RC instrument to focus on individual providers, we found that their characteristic attributes such as preference for particular management styles, leadership quality, and patient ownership are associated with their externally perceived contributions to the overall coordination of care. In an unadjusted analysis, we also observed an intriguing trend towards more frequent major hospital complications when the worst coordinators of each physician type were on service.
Existing evidence22, 23 mostly summarized in a recent RAND Health report shows a weak association between clinical teamwork quality and patient mortality. While our data also support this association, it does so with limitations. Most importantly, the small sample size limited our ability to rigorously account for potential confounders that may have contributed to this apparent association. Further studies may better address whether or not bad outcomes are indeed associated with poor coordinators in highly interdependent clinical teams. In addition to confounding, the small sample size of providers makes the analysis vulnerable to type 1 errors. We addressed this issue by intensively surveying providers repeatedly to achieve a high resolution of the coordination and management structure measures from each comanaged team. The potential for omitted variables and reverse causality in that the coordination scores may be negatively influenced by particularly complex patients and bad outcomes remains a valid concern. We addressed this by confirming the stability of provider RC over time and excluding the RC data from rotations with a bad outcome, but the negative perception of an individual tied to past bad outcomes may persist beyond a particular rotation. Survey responses are subject to recall and hindsight biases, which we attempted to minimize by surveying respondents immediately after each team rotation. Finally, all of our findings may be not be generalizable to other comanagement settings. However, the important correlations between coordination and quality have been observed in other contexts.24, 25
In our study, in‐hospital deaths and ICU transfers are treated as consequences of uncoordinated care. This interpretation may be problematic for circumstances when death is inevitable no matter how well coordinated the care, or when transfer to a higher level of care is appropriate. The rationale for grouping the 2 events into 1 composite bad outcome is based on the assumption that both death and the escalation of care can be delayed to an extent, if not wholly prevented, with the coordinated utilization of a modern hospital's resources. The attribution of these events to poor coordinators may indicate the unraveling of coordination that normally must be maintained to help patients overcome decompensating events that are particularly common in the course of patients with severe liver diseases. Due to the exploratory nature of this analysis, additional studies are necessary to fully characterize the relationship between care coordination and care transfers.
An important implication of this study is that the communication skill and ethical disposition of each individual provider is relevant to the coordination that is sought in multi‐provider teams. Training medical professionals to be better team members may have direct impact on the patients they serve. Our finding about patient ownership suggests that commitment to patients in the framework of care is not merely tradition but a characteristic of competent physicians. Moreover, physicians' commitment to patients is a possible factor, not just in achieving patients' satisfaction, but in securing better outcomes. To that end, the teaching of this and other humanistic principles must remain a vital part of medical education at all levels of training.
Several implications about team leadership and hierarchy are apparent from the data. Findings around the perceived assignment of responsibilities show that high coordinator hepatologists acknowledge the advantages of overlapping task boundaries to prevent critical tasks from being missed and risking bad outcomes. High RC hepatologists in our study adopted a more participatory than supervisory role which presumably facilitated better coordination by transmitting organizational goals to other team members. The function of a comanaged team is likely to be enhanced by a fluid assignment of roles to better handle tasks with high uncertainty. Accordingly, comanagement models of care may not be appropriate in settings where tasks are not interdependent.26 Inherent hierarchy appears to be a feature of well coordinated teams. One possible interpretation of our data is that hospitalists who yield the leadership role to the hepatologist are perceived to be better coordinators and that those who insist on exerting more influence in team decisions are perceived to be poor coordinators.
Existing evidence around care coordination predicts that comanagement designs improve provider coordination through stage‐based and site‐based specialization.12 However, the mechanisms that mediate coordination and patient outcomes are not clear. Moreover, the mechanisms of coordinating multi‐disciplinary teams may be specific to each clinical setting. The role of individual provider characteristics on coordination deserves more attention. Similarly, the impact of organizational culture under which favorable provider characteristics thrive is unknown. Finally, a detailed exposition of patient ownership and the role patients play in affecting the coordination of healthcare resources needs further exploration.
Technological advances drive medical providers to specialize through the need for proficiency around increasingly focused areas of expertise.1 But the benefits of specialization are attained only by balancing the advantages of increasing expertise and the costs of coordinating care that must be borne as specialization increases.2 Integrating experts into modern medical delivery systems requires attention to the coordinating mechanisms that govern team‐based care.3
Coordination, defined as the management of task interdependencies,4 is a central component and a useful measure of teamwork.5 Several studies demonstrate the patient‐level impact of coordination among providers.69 Gittell et al.8 demonstrated that orthopedic hospitals whose staff had better relational coordination (RC) measures had shorter lengths of stay and better post‐operative pain control for patients undergoing surgery. In medical intensive care units (ICUs), Wheelan et al.9 showed that staff members of units with lower mortality rates perceived their teams as functioning at higher stages of group development and perceived their team members as less dependent and more trusting.
Communication is the cornerstone of effective team coordination.10, 11 As such, practice model interventions that facilitate frequent communication of higher quality are associated with lower error rates10 and better teamwork.11 The use of hospitalists, for example, is shown to capitalize on this advantage by improving coordination through physician availability that facilitates communication and relational interactions among hospital‐based staff.12 While system‐level interventions such as this have received significant attention from experts in organizations, empirical studies that explore the contribution of team member characteristics to overall coordination are lacking.13
Inpatient comanagement services offer a unique model for studying teamwork. While the label is used to describe a variety of arrangements,1416 comanagement broadly describes a practice model wherein providers of various specialties deliver direct care to patients, in contrast to the traditional generalist‐consultant model in which specialists lend expertise.17 Many recent comanagement practices involve hospitalists in partnership with surgeons in the care of patients with concurrent medical and surgical needs,18 but similar arrangements between hospitalists and medical subspecialists are being adopted in some medical centers for the care of complex patients with conditions such as heart failure, cancer, stroke, and solid organ transplantations. Coordination among providers has not been studied in this context.
The goals of this study are: (1) to measure the input of individual providers to the overall coordination of care on a highly interdependent medical comanagement service, (2) to characterize high and low coordinators, and (3) to explore the relationship between coordination and patient outcomes. The main hypothesis is that the quality of team coordination is determined partly by the attributes of its members such that their individual contributions to the coordination of care affect the outcomes of vulnerable hospitalized patients.
Materials and Methods
Setting
The study was conducted at the University of Chicago Medical Center, Chicago, IL, an urban 572‐bed tertiary care hospital. The comanaged multidisciplinary inpatient service serves hospitalized patients with complex medical needs. This study focused on providers and patients from a subset of the comanaged multidisciplinary inpatient service that involved the collaboration of medical hepatologists with hospitalists. A hepatology team, composed of an attending hepatologist and a fellow, comanaged with 2 hospitalist teams, each composed of an attending hospitalist and 1 or 2 nonphysician providers (NPPs). Attending physicians rotated on the service in 1‐week to 3‐week rotations, while fellows rotated in 4‐week stretches. NPPs worked nonuniform 3‐day or 4‐day weeks excluding weekends and holidays. The hepatology team was responsible for arranging admissions, developing a care plan with a specialty focus, coordinating care with transplant surgeons when necessary, and managing post‐discharge care. The hospitalist teams were responsible for admitting patients, managing routine and emergent inpatient issues, coordinating care with ancillary and consultative staff, and discharging patients. Dedicated evening and night hospitalists, who were not part of the comanaging day‐time teams, provided after‐hours care. Outside of these areas, there was no instruction or education about how responsibilities should be shared among providers on the service.
Subjects and Study Design
Baseline Survey of Providers
All hospitalists, NPPs, hepatologists, and fellows scheduled to rotate on the comanaged multidisciplinary inpatient service signed a written consent to participate. In April 2008 a nonanonymous baseline 17‐item paper survey was administered.
Items of the Baseline Survey (supporting information Appendix A) were generated from a consideration of the most salient issues around the management structure of comanagement models from a comprehensive review the literature. Two items addressed the respondents' experience and intent to leave their role. Twelve items addressed their preferences about the provider management structure of an ideally comanaged inpatient service, specifically soliciting their preferences about a single physician leader, consensus seeking, and their preferred degree of information, participation, and decision making under the model. Included in this set of items was a single item assessment of the provider's sense of patient ownership on an ideally comanaged service. The final 3 items addressed the perceived assignment of responsibilities. Each of these items presented a clinical objective followed by up to 7 contingent tasks on whose completion the successful execution of the objective depended. Each respondent was asked to indicate one or more of the 4 provider types that should be responsible for completing each task.
Repeated Survey of Providers
From April to October 2008, providers who rotated on the comanaged liver service were surveyed repeatedly to give information about the actual management structure and coordination within teams, which consisted of combinations of randomly assigned providers. Physicians were surveyed on the day when any 1 of the 3 physician types ended his or her rotation. NPPs were surveyed every Wednesday except on the weeks when none of the physicians had changed since the previous survey. One investigator (KH) hand‐delivered the surveys, usually during the first minutes of the joint daily rounds and collected them immediately upon completion. Surveys that could not be completed immediately were collected on daily rounds on subsequent days within 1 week. The primary reason for nonresponse was lost surveys that were not immediately completed.
The 14‐item Repeated Survey (supporting information Appendix B) consisted of 2 parts. The first 7 items reprised items from the Baseline Survey that addressed management structures, but were rephrased to allow respondents to report their experiences on their immediate rotation. The second part of the Repeated Survey addressed RC, which is described below.
The study protocols, consents, and data collection mechanisms were approved by the institutional review board of the University of Chicago Medical Center. Collection of patient information was designed to comply with the Health Insurance Portability and Accountability Act of 1996.
Patients
Patients were admitted to 1 of the 2 hospitalist teams on the comanaged service on alternating days, which allowed patients to be assigned to providers pseudo‐randomly. Consent to use clinical data was obtained during their stay or by telephone after discharge. If patients were unable to provide consent due to cognitive impairment, consent was sought through designated proxies.19
Main Measurements
Relational Care Coordination
The survey instrument used to measure individual contributions to overall coordination was adapted from the Relational Coordination tool developed by Gittell.20 This instrument was chosen because it has already been validated in various clinical contexts8, 12, 21 and the theoretical assumptions about the independent relational and communication components of coordination are applicable to our context. RC is characterized by the 7 domains of frequent, timely, accurate, and problem‐solving communications; shared goals, shared knowledge, and mutual respect. Respondents rated, on a 5‐point scale (1 = negative, 5 = positive), team members of the other 3 provider types during each rotation on all of the 7 domains. The mean across the domains yielded the RC score. Although the instrument was originally developed to measure the coordination in groups of individuals, the RC for a single provider was calculated by taking the mean of all the RC directed at that individual across team members who worked with him or her during the study period. Because some providers worked more rotations than others, a nonuniform number of observations contributed to the calculation of individual RC (Table 1). For each provider type, individuals were ranked on their RC and categorized in tertiles representing high, middle, and low coordinators.
Baseline Survey (%) | Repeated Surveys (%) | % Female | Years Experience Median (range) | # RC Evaluations of Each Provider Median (range) | RC Mean (range) | |
---|---|---|---|---|---|---|
| ||||||
Hospitalists | 15/15 (100) | 36/43 (84) | 42 | 1 (0‐10) | 6 (3‐21) | 4.71 (4.33‐4.94) |
NPPs | 5/5 (100) | 92/97 (95) | 100 | 4 (2‐15) | 30 (23‐34) | 4.60 (4.48‐4.71) |
Hepatologists | 6/6 (100) | 26/42 (62) | 33 | 7 (1‐25) | 16 (5‐51) | 4.37 (4.03‐4.59) |
GI fellows | 6/6 (100) | 23/42 (55) | 48 | 1 (0‐1) | 19 (8‐37) | 4.28 (3.88‐4.53) |
Total | 32/32 (100) | 177/223 (79) | 55 | 2 (0‐25) | 12.5 (3‐51) | 4.57 (3.88‐4.94) |
Statistical Analysis
The discriminating ability of the RC for individuals was assessed by comparing the highest and lowest RC of each provider type using the 2‐tailed t‐test. The difference in responses to items from the Baseline and Repeated Surveys by individual RC tertiles was assessed with the Chi‐squared test for categorical data and the 2‐tailed t‐test for comparing means. For each physician type, the frequency of the composite bad outcomes between the highest and lowest RC tertile categories were compared using a 2‐sample Wilcoxon rank‐sum (Mann‐Whitney) test for nonparametric data.
Results
All 32 providers (100%) completed the Baseline Survey and participated in the Repeated Surveys of which 177/224 (79%) were completed. The median number of surveys that contributed to the calculation of individual RC and the mean RC by provider type are summarized in Table 1.
Of the 119 patients managed on the service, the mean age (standard deviation [SD]) was 55 (14) years and 48% were women. Of the 201 hospitalizations, there were 13 floor‐to‐ICU transfers and 5 in‐hospital deaths, however, we excluded from the analysis 1 death of a patient who was admitted under inpatient hospice status.
RC Measures
Individual provider RC ranges were 4.33 to 4.94 (p = 0.05) for hospitalists; 4.48 to 4.71 (p = 0.10) for NP/PAs; 4.03 to 4.59 (p < 0.01) for hepatologists; and 3.88 to 4.52 (p = 0.02) for fellows. The high, middle, and low coordinator categories for each provider type were shown to be durable through time by demonstrating that the coordination ranking of individuals was essentially preserved even when using partial data from each half of the study period. Thus, RC appears to reflect a stable attribute of the provider as opposed to specific circumstances of the rotation. The categories were shown to be durable to the influences of bad outcomes (inpatient deaths and ICU transfers) by demonstrating that the placement of individuals into 1 of the 3 coordination categories were preserved even when data from rotations involving a bad outcome were removed. Nonetheless, in order to address the possibility of bad outcomes negatively affecting perception of coordination, all analysis involving RC used the values that excluded data from these rotations.
Characteristics of Good and Poor Coordinators
Patient Ownership
The single‐item measure of patient ownership in the Baseline Survey reads: I have as much a sense of ownership of my patients on the comanaged service as on a non‐comanaged service. The majority of providers of every type in the high and middle coordinator categories agreed, while providers in the low coordinator category generally disagreed with the statement. The aggregated responses of all the provider types are shown in Table 2.
Agree | Somewhat Agree | Somewhat Disagree | Disagree | |
---|---|---|---|---|
High | 4 | 6 | 0 | 1 |
Middle | 5 | 4 | 0 | 2 |
Low | 2 | 0 | 4 | 4 |
p < 0.01 |
Leadership
Hepatologists are the potential leader of the comanaged team because of their content expertise in liver diseases. Their responses to the 3 items in the Baseline Survey that addressed perceived assignment of responsibilities are shown in Table 3. The high compared to the low coordinator hepatologists delegated the responsibility of completing necessary tasks to more providers, overall, such that an average of 3 providers were redundantly held responsible for the completion of each task by the high coordinators while only 1 provider was held responsible by the low coordinators. Furthermore, the high coordinators delegated the responsibility of completing more tasks to themselves compared to the low coordinators.
Hepatologists | Mean # of Tasks Delegated Overall, n (SD) | Mean # of Providers Delegated to Each Task, n (SD) | Mean # of Tasks Delegated to Self, n (SD) |
---|---|---|---|
| |||
High (n = 2) | 56 (0.0) | 2.9 (0.0) | 11.5 (2.1) |
Middle (n = 2) | 35 (2.8) | 1.8 (0.2) | 9.5 (3.5) |
Low (n = 2) | 19 (1.4) | 1.0 (0.1) | 4.5 (2.1) |
p value(high vs. low) | <0.01 | <0.01 | 0.08 |
According to responses to the management structure items of the Repeated Surveys, more providers of every type indicated that a single physician leader directed the overall management of every patient when a high or middle coordinator hospitalist was on service as opposed to a service with a low coordinator hospitalist (high 76% vs. middle 73% vs. low 58%, P = 0.06). Furthermore, a low coordinator hospitalist on service was more likely to indicate a desire for greater influence in directing the management of patients (desire influence 93% vs. not 7%, P < 0.01). This pattern was also seen with low coordinator NPPs, who more often indicated a desire for greater influence in directing patient management (desire influence 100% vs. not 0%, P < 0.01).
Experience
Age, years in practice, years at the institution, and time spent on the comanaged service were not associated with RC in our small sample of providers.
Outcomes by Provider Coordination
The unit of analysis in this section is the team‐patient encounter, which is the consecutive days during which a unique assortment of physicians managed a patient's hospitalization. NPPs could not be associated with any single team due to their nonuniform work patterns. The 201 hospitalizations in this study were composed of 351 team‐patient encounters. Table 4 displays the unadjusted frequency of inpatient deaths and ICU transfers that occurred during these encounters by RC tertiles. In each of the 3 physician types, composite bad outcomes are most frequent among the lowest coordinators. The pattern is statistically significant for hospitalists.
Team‐Patient Encounters, n | Mean Length of Encounter, n (days) | ICU Transfer, n (%) | Hospital Death, n (%) | Bad Outcome, n (%) | |
---|---|---|---|---|---|
| |||||
Hospitalists | |||||
High (n = 5) | 92 | 3.1 | 1 (1.1) | 1 (1.1) | 1 (1.1) |
Middle (n = 5) | 119 | 3.2 | 1 (0.8) | 1 (0.8) | 1 (0.8) |
Low (n = 5) | 140 | 3.2 | 11 (7.9) | 2 (1.4) | 12 (8.6) |
p value (high vs. low) | NA | 0.70 | 0.02 | 0.82 | 0.02 |
Hepatologists | |||||
High (n = 2) | 99 | 3.2 | (2.0) | 0 (0.0) | 2 (2.0) |
Middle (n = 2) | 79 | 3.4 | 3 (3.0) | 1 (1.3) | 3 (3.0) |
Low (n = 2) | 173 | 3.0 | 8 (4.6) | 3 (1.7) | 9 (5.2) |
p value (high vs. low) | NA | 0.52 | 0.27 | 0.19 | 0.20 |
GI fellows | |||||
High (n = 2) | 111 | 3.1 | 2 (1.8) | 0 (0.0) | 2 (1.8) |
Middle (n = 2) | 67 | 3.3 | 2 (3.0) | 1 (1.5) | 2 (3.0) |
Low (n = 2) | 173 | 3.2 | 9 (5.2) | 3 (1.7) | 10 (5.8) |
p value (high vs. low) | NA | 0.74 | 0.15 | 0.16 | 0.10 |
Another interesting observation is the largest number of encounters in the lowest coordination tertile of each physician type. While the reason for this finding is not clear, associations between work‐load and poor coordination evoke issues related to burnout. In order to address the possibility of an artifactually elevated probability of a bad outcome among providers who rotated through the service more often, we calculated the correlation between the number of encounter‐days and the frequency of bad outcomes for the 15 providers who were associated with at last one such event. If these events occurred by chance, we should find a positive correlation between its frequency and the number of encounters. The Pearson's correlation coefficient of 0.38 suggests that bad outcomes do not occur more frequently with providers who work more rotations by chance alone.
Discussion
By adapting Gittell's RC instrument to focus on individual providers, we found that their characteristic attributes such as preference for particular management styles, leadership quality, and patient ownership are associated with their externally perceived contributions to the overall coordination of care. In an unadjusted analysis, we also observed an intriguing trend towards more frequent major hospital complications when the worst coordinators of each physician type were on service.
Existing evidence22, 23 mostly summarized in a recent RAND Health report shows a weak association between clinical teamwork quality and patient mortality. While our data also support this association, it does so with limitations. Most importantly, the small sample size limited our ability to rigorously account for potential confounders that may have contributed to this apparent association. Further studies may better address whether or not bad outcomes are indeed associated with poor coordinators in highly interdependent clinical teams. In addition to confounding, the small sample size of providers makes the analysis vulnerable to type 1 errors. We addressed this issue by intensively surveying providers repeatedly to achieve a high resolution of the coordination and management structure measures from each comanaged team. The potential for omitted variables and reverse causality in that the coordination scores may be negatively influenced by particularly complex patients and bad outcomes remains a valid concern. We addressed this by confirming the stability of provider RC over time and excluding the RC data from rotations with a bad outcome, but the negative perception of an individual tied to past bad outcomes may persist beyond a particular rotation. Survey responses are subject to recall and hindsight biases, which we attempted to minimize by surveying respondents immediately after each team rotation. Finally, all of our findings may be not be generalizable to other comanagement settings. However, the important correlations between coordination and quality have been observed in other contexts.24, 25
In our study, in‐hospital deaths and ICU transfers are treated as consequences of uncoordinated care. This interpretation may be problematic for circumstances when death is inevitable no matter how well coordinated the care, or when transfer to a higher level of care is appropriate. The rationale for grouping the 2 events into 1 composite bad outcome is based on the assumption that both death and the escalation of care can be delayed to an extent, if not wholly prevented, with the coordinated utilization of a modern hospital's resources. The attribution of these events to poor coordinators may indicate the unraveling of coordination that normally must be maintained to help patients overcome decompensating events that are particularly common in the course of patients with severe liver diseases. Due to the exploratory nature of this analysis, additional studies are necessary to fully characterize the relationship between care coordination and care transfers.
An important implication of this study is that the communication skill and ethical disposition of each individual provider is relevant to the coordination that is sought in multi‐provider teams. Training medical professionals to be better team members may have direct impact on the patients they serve. Our finding about patient ownership suggests that commitment to patients in the framework of care is not merely tradition but a characteristic of competent physicians. Moreover, physicians' commitment to patients is a possible factor, not just in achieving patients' satisfaction, but in securing better outcomes. To that end, the teaching of this and other humanistic principles must remain a vital part of medical education at all levels of training.
Several implications about team leadership and hierarchy are apparent from the data. Findings around the perceived assignment of responsibilities show that high coordinator hepatologists acknowledge the advantages of overlapping task boundaries to prevent critical tasks from being missed and risking bad outcomes. High RC hepatologists in our study adopted a more participatory than supervisory role which presumably facilitated better coordination by transmitting organizational goals to other team members. The function of a comanaged team is likely to be enhanced by a fluid assignment of roles to better handle tasks with high uncertainty. Accordingly, comanagement models of care may not be appropriate in settings where tasks are not interdependent.26 Inherent hierarchy appears to be a feature of well coordinated teams. One possible interpretation of our data is that hospitalists who yield the leadership role to the hepatologist are perceived to be better coordinators and that those who insist on exerting more influence in team decisions are perceived to be poor coordinators.
Existing evidence around care coordination predicts that comanagement designs improve provider coordination through stage‐based and site‐based specialization.12 However, the mechanisms that mediate coordination and patient outcomes are not clear. Moreover, the mechanisms of coordinating multi‐disciplinary teams may be specific to each clinical setting. The role of individual provider characteristics on coordination deserves more attention. Similarly, the impact of organizational culture under which favorable provider characteristics thrive is unknown. Finally, a detailed exposition of patient ownership and the role patients play in affecting the coordination of healthcare resources needs further exploration.
- Technology, specialization, and the allied health professions.J Allied Health.1983;12(3):177–182. .
- Hospitalists and the doctor‐patient relationship.J Legal Stud.2001;30:589–606. .
- From Chaos to Care: The Promise of Team‐Based medicine.Cambridge, MA:Perseus;2002. .
- Determinants of coordination modes within organizations.Am Sociol Rev.1976;41:322–338. , , .
- Teamwork and patient safety in dynamic domains of healthcare: a review of the literature.Acta Anaesthesiol Scand.2009;53(2):143–151. .
- The association between interdisciplinary collaboration and patient outcomes in a medical intensive care unit.Heart Lung.1992;21:18–24. , , , , .
- Patterns of coordination and clinical outcomes: a study of surgical services.Health Serv Res.1998;22:1211–1236. , , , et al.
- Impact of relational coordination on quality of care, postoperative pain and functioning, and length of stay: a nine‐hospital study of surgical patients.Med Care.2000;38(8):807–819. , , , et al.
- The link between teamwork and patients' outcomes in intensive care units.Am J Crit Care.2003;12(6):527–534. , , .
- Assessing system failures in operating rooms and intensive care units.Qual Saf health Care.2007;16:45–50. , , .
- Patient handover from surgery to intensive care: using Formula 1 pit‐stop and aviation models to improve safety and quality.Paediatr Anaesth.2007;17(5):470–478. , , , et al.
- Is the doctor in? A relational approach to job design and the coordination of work.Hum Resour Manage.2008;47(4):729–755. , , , .
- Developing a team performance framework for the intensive care unit.Crit Care Med.2009;37(5):1787–1793. , , , .
- Medical and surgical comanagement after elective hip and knee arthroplasty: a randomized, controlled trial.Ann Intern Med.2004;141(1):28–38. , , , et al.
- Medical/psychiatric comanagement by nurse practitioners in chronic hepatitis C treatment: a case study.Arch Psychiatr Nurs.2007;21(2):87–90. , , .
- Perioperative medicine for the hospitalized patient.Med Clin North Am.2008;92(2):325–348. , .
- The place and training of the general practitioner.Calif Med.1949;70(4):265–268. .
- Surgical comanagement: a natural evolution of hospitalist practice.J Hosp Med.2008;3(5):394–397. , .
- Validation of a telephone version of the mini‐mental state examination.J Am Geriatr Soc.1992;40(7):697–702. , , , .
- Organizing work to support relational co‐ordination.Int J of Human Resource Management.2000;11(3):517–539. .
- Beyond our walls: impact of patient and provider coordination across the continuum on outcomes for surgical patients.Health Serv Res.2007;42:7–24. , , , , .
- The effect of multidisciplinary care teams on intensive care unit mortality.Arch Intern Med.2010;170(4):369–376. , , , , .
- Outcome measures for effective teamwork in inpatient care: final report.Santa Monica:RAND Health;2008. , , , .
- The performance of intensive care units: does good management make a difference?Med Care.1994;32(5):508–525. , , , et al.
- Observational assessment of surgical teamwork: a feasibility study.World J Surg.2006;30(10):1774–1783. , , , .
- Just because you can, doesn't mean that you should: A call for the rational application of hospitalist comanagement.J Hosp Med.2008;3(5):398–3402. .
- Technology, specialization, and the allied health professions.J Allied Health.1983;12(3):177–182. .
- Hospitalists and the doctor‐patient relationship.J Legal Stud.2001;30:589–606. .
- From Chaos to Care: The Promise of Team‐Based medicine.Cambridge, MA:Perseus;2002. .
- Determinants of coordination modes within organizations.Am Sociol Rev.1976;41:322–338. , , .
- Teamwork and patient safety in dynamic domains of healthcare: a review of the literature.Acta Anaesthesiol Scand.2009;53(2):143–151. .
- The association between interdisciplinary collaboration and patient outcomes in a medical intensive care unit.Heart Lung.1992;21:18–24. , , , , .
- Patterns of coordination and clinical outcomes: a study of surgical services.Health Serv Res.1998;22:1211–1236. , , , et al.
- Impact of relational coordination on quality of care, postoperative pain and functioning, and length of stay: a nine‐hospital study of surgical patients.Med Care.2000;38(8):807–819. , , , et al.
- The link between teamwork and patients' outcomes in intensive care units.Am J Crit Care.2003;12(6):527–534. , , .
- Assessing system failures in operating rooms and intensive care units.Qual Saf health Care.2007;16:45–50. , , .
- Patient handover from surgery to intensive care: using Formula 1 pit‐stop and aviation models to improve safety and quality.Paediatr Anaesth.2007;17(5):470–478. , , , et al.
- Is the doctor in? A relational approach to job design and the coordination of work.Hum Resour Manage.2008;47(4):729–755. , , , .
- Developing a team performance framework for the intensive care unit.Crit Care Med.2009;37(5):1787–1793. , , , .
- Medical and surgical comanagement after elective hip and knee arthroplasty: a randomized, controlled trial.Ann Intern Med.2004;141(1):28–38. , , , et al.
- Medical/psychiatric comanagement by nurse practitioners in chronic hepatitis C treatment: a case study.Arch Psychiatr Nurs.2007;21(2):87–90. , , .
- Perioperative medicine for the hospitalized patient.Med Clin North Am.2008;92(2):325–348. , .
- The place and training of the general practitioner.Calif Med.1949;70(4):265–268. .
- Surgical comanagement: a natural evolution of hospitalist practice.J Hosp Med.2008;3(5):394–397. , .
- Validation of a telephone version of the mini‐mental state examination.J Am Geriatr Soc.1992;40(7):697–702. , , , .
- Organizing work to support relational co‐ordination.Int J of Human Resource Management.2000;11(3):517–539. .
- Beyond our walls: impact of patient and provider coordination across the continuum on outcomes for surgical patients.Health Serv Res.2007;42:7–24. , , , , .
- The effect of multidisciplinary care teams on intensive care unit mortality.Arch Intern Med.2010;170(4):369–376. , , , , .
- Outcome measures for effective teamwork in inpatient care: final report.Santa Monica:RAND Health;2008. , , , .
- The performance of intensive care units: does good management make a difference?Med Care.1994;32(5):508–525. , , , et al.
- Observational assessment of surgical teamwork: a feasibility study.World J Surg.2006;30(10):1774–1783. , , , .
- Just because you can, doesn't mean that you should: A call for the rational application of hospitalist comanagement.J Hosp Med.2008;3(5):398–3402. .
Copyright © 2010 Society of Hospital Medicine