User login
Readmissions in Primary Care Clinics
Reducing hospital readmissions is a national healthcare priority. In October 2012, the Centers for Medicare and Medicaid Services (CMS) enacted financial penalties on hospitals with higher than average risk‐adjusted readmissions, offering an incentive pool of $850 million in the first year.[1] As a result, a wide range of activities to understand and reduce readmissions among patients with congestive heart failure (CHF), acute myocardial infarction (AMI), and pneumonia have emerged.[2, 3, 4, 5] Some have proposed that to effectively reduce hospital readmissions, a community of inpatient and outpatient providers and local support organizations must coordinate efforts.[6, 7] In fact, CMS has recognized the important role of community support groups and outpatient providers in safe discharge transitions in 2 ways. First, in 2011 CMS launched the Community‐based Care Transitions Program to fund community‐based organizations to assist Medicare patients with care transitions.[8] Second, CMS introduced 2 new reimbursement codes for primary care providers (PCPs) to perform care coordination immediately after hospital discharge.[9] Both of these new payment programs represent an evolving perspective that reducing hospital readmissions requires active participation among outpatient partners.
As leaders of the outpatient care team, PCPs play a significant role in reducing hospital readmissions. One way in which PCPs can begin to understand the magnitude of the issue within their clinic is to evaluate the clinic's 30‐day readmission rates. Currently, CMS calculates readmission rates at the hospital level. However, understanding these rates at the clinic level is critical for developing strategies for improvement across the care continuum. Our current understanding of effective outpatient interventions to reduce hospital readmission is limited.[3] As clinics introduce and refine strategies to reduce readmissions, tracking the impact of these strategies on readmission rates will be critical for identifying effective outpatient interventions. Clinics with similar patient case‐mix can also benchmark readmission rates, sharing best practices from clinics with lower‐than‐expected rates.
There are no available studies or proposed methodologies to guide primary care clinics in calculating their 30‐day readmission rates. A particularly difficult challenge is obtaining the admission information when patients may be admitted to 1 of several area hospitals. For large integrated delivery networks where primary care patients are relatively loyal to the network of physicians and hospitals, an opportunity exists to explore the data. Furthermore, variations in readmission rates across primary care specialties (such as internal medicine and family practice) are not well understood. In this study, we set out to develop a methodology for calculating all‐cause 30‐day hospital readmission rates at the level of individual primary care practices and to identify factors associated with variations in these rates.
METHODS
Study Design
We conducted a retrospective observational study of adult primary care patients at the University of California, San Francisco (UCSF) who were hospitalized at UCSF Medical Center between July 1, 2009 and June 30, 2012. UCSF Medical Center is comprised of Moffitt‐Long Hospital (a 600‐bed facility) and UCSF‐Mount Zion Hospital (a 90‐bed facility) located in San Francisco, CA. The patient population was limited to adults ages 18 and over with a PCP at UCSF. UCSF has 7 adult primary care clinics: General Internal Medicine (IM), Family Practice (FP), Women's Health, Geriatrics, a combined IM/FP clinic, Human Immunodeficiency Virus (HIV) Primary Care, and a Concierge Internal Medicine clinic staffed by IM physicians. Between 2009 and 2011, all clinics completed the process of enpanelment, or defining the population of patients for which each PCP and the clinic is responsible. We obtained the list of patient and PCP assignments at each of the 7 clinics across the time period of study. We then obtained UCSF Medical Center hospital claims data for this group, including dates of admission and discharge, patient age, sex, race/ethnicity, language, insurance, admitting service, diagnosis codes, information on intensive care unit stay, and discharge disposition. Hospital claims data are housed in Transition Systems International (Boston, MA) administrative databases, a cost‐accounting system that collects data abstracted from patient charts upon discharge from UCSF Medical Center.
All‐cause 30‐day hospital readmission rates were calculated for each primary care clinic, using an adaptation of the CMS definition. First, we defined index discharges as the first discharge for an individual patient in any given 30‐day interval. Only 1 index discharge is flagged for each 30‐day interval. The first admission within 30 days after the index discharge was flagged as the readmission. Consistent with CMS methodology, only the first readmission in the 30‐day period was counted. We included all inpatient and observation status admissions and excluded patients who died during the index encounter, left against medical advice, or transferred to another acute care hospital after the index encounter.
We used secondary diagnosis codes in the administrative data to classify comorbidities by the Elixhauser methodology.[10] All but 1 adult primary care clinic at UCSF are faculty‐only clinics, whereby the assigned PCP is an attending physician. In the general internal medicine clinic, an attending or a resident can serve as the PCP, and approximately 20% of IM clinic patients have a resident as their PCP. For the IM clinic, we classified patients' PCP as attending, resident, or departed. The latter category refers to patients whose PCPs were residents who had graduated or faculty who had departed and had not been assigned to a new PCP prior to the index admission or readmission.
Statistical Analysis
We built a model to predict readmissions using the demographic and clinical variables with [2] P<0.20 in an initial bivariate analysis, and then removed, with backward selection, the least significant variables until only those with P0.05 remained. Age, log‐length of stay (LOS), and intensive care unit stay were forced in the model, as studies evaluating factors related to readmissions have often included these as important covariates.[11, 12, 13, 14] Results were expressed as adjusted odds ratio (OR) with 95% confidence interval (CI). All analyses were carried out using SAS version 9.2 (SAS Institute, Inc., Cary, NC).
This study was exempt from review by the institutional review board of UCSF.
RESULTS
During the study period, there were 12,564 discharges from UCSF Medical Center for primary care patients belonging to the 7 clinics. Of these, 8685 were index discharges and 1032 were readmissions within 30 days. Table 1 shows the characteristics of the patients who had at least 1 admission during the study period. In all but 2 clinics (HIV Primary Care and Concierge Internal Medicine), there were more women hospitalized than men. Age and gender differences across clinics are consistent with the patient populations served by these clinics, with Women's Health having more female patients and younger patients hospitalized compared to Geriatrics.
General Internal Medicine | Family Practice | Women's Health | Geriatrics | Combined IM/FP | HIV Primary Care | Concierge IM | |
---|---|---|---|---|---|---|---|
| |||||||
Panel size | 26,521 | 10,495 | 8,526 | 190 | 4,109 | 1,039 | 706 |
FTE attending MD and NP providers | 18.8 | 7.3 | 4.5 | 1.7 | 5.0 | 2.3 | 2.1 |
Mean panel size per FTE | 1,311 | 1,448 | 1,895 | 112 | 822 | 452 | 336 |
Clinic visits in FY 2012 | 50,362 | 20,647 | 11,014 | 4,425 | 8,120 | 4,182 | 1,713 |
No. of index discharges | 5,388 | 1,204 | 983 | 409 | 339 | 249 | 113 |
No. of readmissions | 718 | 104 | 76 | 56 | 30 | 37 | 11 |
No. of patients discharged during study period | 4,063 | 1,003 | 818 | 289* | 290 | 185 | 84 |
% Male | 40.1% | 33.9% | 4.3% | 35.3% | 33.8% | 69.7% | 52.4% |
Average age (SD), y | 60.4 (18.6) | 52.0 (19.0) | 47.0 (15.7) | 83.2 (6.9) | 46.3 (16.9) | 49.5 (9.9) | 64.5 (15.6) |
Age range, y | 19104 | 1896 | 1997 | 6399 | 1890 | 2273 | 2092 |
Race | |||||||
% White | 42.2% | 41.3% | 58.8% | 61.9% | 49.3% | 61.0% | 91.7% |
% Black | 16.3% | 8.5% | 7.5% | 7.3% | 10.0% | 27.6% | 0.0% |
% Asian | 23.6% | 31.4% | 19.0% | 17.0% | 16.6% | 2.2% | 4.8% |
% Native America/ Alaskan Native | 0.7% | 1.6% | 0.7% | 0.7% | 0.7% | 0.5% | 0.0% |
% Other | 16.3% | 15.7% | 13.1% | 12.5% | 18.3% | 8.1% | 2.4% |
Not available | 1.0% | 1.6% | 1.0% | 0.7% | 5.2% | 0.5% | 1.2% |
Ethnicity | |||||||
% Hispanic | 8.8% | 10.6% | 5.0% | 6.2% | 8.3% | 4.9% | 0.0% |
% Non‐Hispanic | 73.8% | 74.5% | 81.8% | 73.0% | 74.5% | 84.9% | 75.0% |
Not available | 17.4% | 15.0% | 13.2% | 20.8% | 17.2% | 10.3% | 25.0% |
Language | |||||||
% English | 69.7% | 75.6% | 88.8% | 69.6% | 85.9% | 89.7% | 89.3% |
% Spanish | 3.8% | 1.9% | 0.6% | 4.2% | 1.0% | 0.5% | 0.0% |
% Chinese (Mandarin or Cantonese) | 8.0% | 6.7% | 1.2% | 4.8% | 2.1% | 0.0% | 0.0% |
% Russian | 1.7% | 0.6% | 0.1% | 0.4% | 1.0% | 0.0% | 0.0% |
% Vietnamese | 1.0% | 1.4% | 0.0% | 0.4% | 0.0% | 0.0% | 0.0% |
% Other | 11.7% | 9.3% | 5.6% | 18.0% | 6.9% | 8.1% | 4.8% |
Not available | 4.1% | 4.6% | 3.7% | 2.8% | 3.1% | 1.6% | 6.0% |
Insurance type | |||||||
% Private | 35.4% | 58.7% | 77.4% | 10.7% | 63.8% | 36.8% | 66.7% |
% Medicare | 22.0% | 17.2% | 12.0% | 67.8% | 14.8% | 8.7% | 33.3% |
% Medicaid | 15.5% | 12.5% | 3.1% | 1.4% | 12.8% | 24.3% | 0.0% |
% Dual eligible | 24.4% | 8.8% | 5.4% | 19.4% | 6.6% | 27.6% | 0.0% |
% Self‐pay/other | 2.6% | 2.9% | 2.2% | 0.7% | 2.1% | 2.7% | 0.0% |
All‐cause 30‐day readmission rates varied across practices, with HIV Primary Care being the highest at 14.9%, followed by Geriatrics at 13.7%, General Internal Medicine at 13.3%, Concierge Internal Medicine at 9.7%, combined IM/FP at 8.9%, Family Practice at 8.6%, and Women's Health at 7.7% (Figure 1). Despite HIV Primary Care having the highest readmission rate, the number of index discharges during the 3‐year period was relatively low (249) compared to General Internal Medicine (5388). For the index admission, the top 5 admitting services were medicine, obstetrics, cardiology, orthopedic surgery, and general surgery (Table 2). Medicine was the primary admitting service for patients in the following clinics: General Internal Medicine, Geriatrics, HIV Primary Care, and Concierge Internal Medicine. Obstetrics was the primary admitting service for patients in the Family Practice, Women's Health, and combined IM/FP clinics. Vaginal delivery was the top discharge diagnosis‐related group (DRG) for patients in the General Internal Medicine, Family Practice, Women's Health, and combined IM/FP clinics. The top discharge DRG was urinary tract infection for Geriatrics, HIV for the HIV clinic (13.6%), and chemotherapy (8.0%) for Concierge Internal Medicine. Average LOS varied from 4.7 days for patients in the HIV Primary Care clinic to 2.8 days for patients in the Concierge Internal Medicine clinic. Average LOS was 3.4, 3.1, and 3.2 days in the Family Practice, Women's Health, and Geriatrics clinics, respectively, and 3.8 days in the General Internal Medicine and combined IM/FP clinics. For all clinics except Geriatrics, the majority of patients were discharged home without home health. A larger proportion of patients in the Geriatrics clinic were discharged home with home health or discharged to a skilled nursing facility.
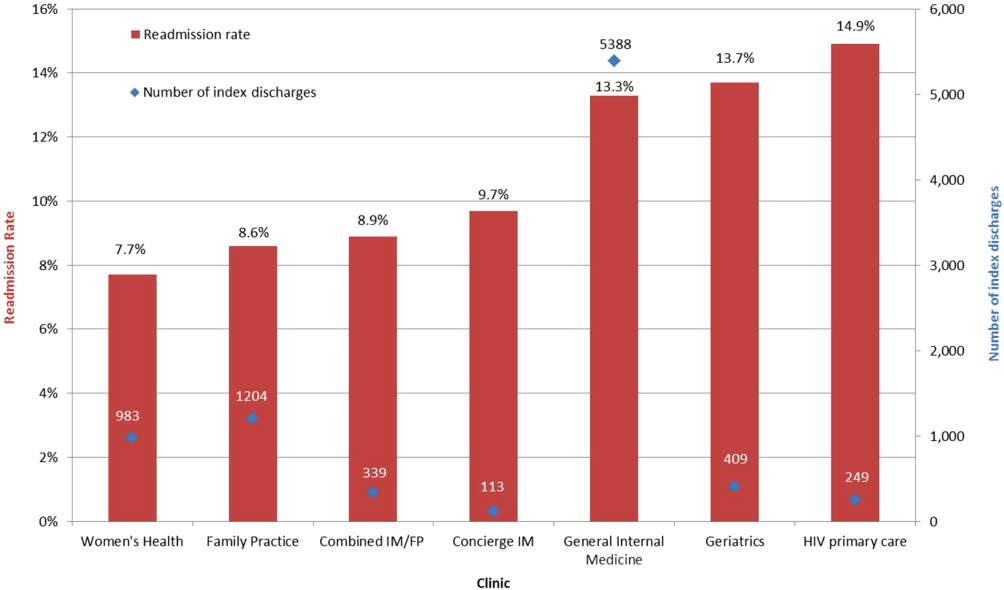
General Internal Medicine, N=5,388 | Family Practice, N=1,204 | Women's Health, N=983 | Geriatrics, N=409 | Combined IM/FP, N=339 | HIV Primary Care, N=249 | Concierge IM, N=113 | |
---|---|---|---|---|---|---|---|
| |||||||
Top 5 admitting services | |||||||
Medicine | 41.8% | 25.0% | 15.2% | 56.4% | 23.5% | 59.7% | 19.0% |
Obstetrics | 7.5% | 24.7% | 38.7% | 0.0% | 35.6% | 2.1% | 9.0% |
Cardiology | 15.1% | 10.9% | 6.2% | 16.5% | 10.3% | 6.5% | 17.0% |
Orthopedic surgery | 8.3% | 8.3% | 8.2% | 7.3% | 5.3% | 4.8% | 12.0% |
Adult general surgery | 7.2% | 9.8% | 10.0% | 6.0% | 5.0% | 5.8% | 7.0% |
Top 5 discharge diagnoses | |||||||
Vaginal delivery | 3.5% | 13.6% | 20.6% | 0.0% | 17.9% | 0.3% | 3.0% |
Major joint replacement | 3.5% | 3.4% | 4.8% | 4.3% | 3.8% | 0.7% | 5.0% |
Vaginal delivery with complications | 1.3% | 5.0% | 6.9% | 0.0% | 4.1% | 1.0% | 2.0% |
Simple pneumonia and pleurisy | 2.8% | 1.2% | 0.6% | 3.8% | 0.3% | 2.1% | 0.0% |
Urinary tract infection | 2.0% | 1.5% | 1.4% | 6.2% | 0.9% | 2.4% | 1.0% |
Discharge disposition | |||||||
% Home | 69.1% | 74.6% | 76.6% | 47.8% | 69.9% | 78.6% | 68.4% |
% Home with home health | 19.4% | 16.5% | 17.8% | 24.1% | 21.7% | 11.8% | 18.8% |
% Skilled nursing facility | 7.2% | 4.9% | 3.0% | 18.9% | 3.3% | 5.0% | 0.8% |
% Other | 4.3% | 4.0% | 2.6% | 9.2% | 5.1% | 4.6% | 12.0% |
Average length of stay (SD) | 3.8 (5.6) | 3.4 (7.5) | 3.1 (3.7) | 3.2 (3.7) | 3.8 (5.2) | 4.7 (6.5) | 2.8 (2.8) |
% Discharges with ICU stay | 11.0% | 10.6% | 5.9% | 8.5% | 9.2% | 11.1% | 15.8% |
Factors associated with variation in readmission rates included: male gender (OR: 1.21, 95% CI: 1.051.40), Medicare (OR: 1.31, 95% CI: 1.051.64; Ref=private) and dual‐eligible Medicare‐Medicaid insurance (OR: 1.26, 95% CI: 1.011.56), unknown primary language (OR: 0.06, 95% CI: 0.020.25; Ref=English), and the following comorbidities: peripheral vascular disease, renal failure, lymphoma, fluid and electrolyte disorders, and anemia. Multivariable logistic regression modeling results are listed in Table 3. Patients having a resident PCP showed no increased odds of readmission (OR: 1.13, 95% CI: 0.931.37; Ref=attending PCP). However, patients with a graduated resident PCP or departed faculty PCP awaiting transfer to a new PCP had an OR of 1.59 (95% CI: 1.162.17) compared with having a current faculty PCP. The C‐statistic for this model was 0.67.
Adjusted Odds Ratio (95% CI) | |
---|---|
| |
Age | |
<65 years | Ref |
>65 years | 0.91 (0.751.10) |
Clinic | |
General Internal Medicine | 1.24 (0.991.56) |
Family Practice | Ref |
Women's Health | 1.13 (0.821.56) |
Geriatrics | 1.20 (0.841.74) |
Combined Internal Medicine/Family Practice | 1.13 (0.731.74) |
HIV Primary Care | 1.24 (0.811.89) |
Concierge Internal Medicine | 1.06 (0.542.07) |
Sex | |
Female | Ref |
Male | 1.21 (1.051.40) |
Insurance | |
Private | Ref |
Medicaid | 1.09 (0.871.37) |
Medicare | 1.31 (1.051.64) |
Dual eligible MedicareMedicaid | 1.26 (1.011.56) |
Self/other | 1.20 (0.741.94) |
Language | |
English | Ref |
Spanish | 1.02 (0.701.47) |
Chinese | 1.13 (0.871.47) |
Other | 0.87 (0.701.08) |
Unknown | 0.06 (0.020.25) |
Comorbidities | |
Pulmonary circulation disease | 1.51 (0.982.32) |
Peripheral vascular disease | 1.50 (1.141.98) |
Renal failure | 1.31 (1.091.58) |
Lymphoma | 2.50 (1.434.36) |
Fluid and electrolyte disorders | 1.27 (1.071.52) |
Deficiency anemia | 1.25 (1.041.51) |
Physician | |
Attending | Ref |
Resident | 1.13 (0.931.37) |
Departed PCP (internal medicine only) | 1.59 (1.162.17) |
ICU stay | 1.03 (0.831.28) |
LOS (log) | 1.02 (0.981.06) |
Admitting service | |
Medicine | Ref |
Obstetrics | 0.41 (0.300.56) |
Cardiology | 1.12 (0.911.37) |
Orthopedic surgery | 0.36 (0.250.51) |
Adult general surgery | 0.64 (0.470.87) |
Other services | 0.76 (0.630.92) |
DISCUSSION
In this study we introduce a complementary way to view hospital readmissions, from the perspective of primary care practices. We found variation in readmission rates across primary care practices. After controlling for admitting service, clinic, provider, and patient factors, the specific characteristics of male gender, patients with Medicare or Medicare with Medicaid, and patients in our General Internal Medicine clinic with a departed PCP were independent risk factors for hospital readmission. Patients with specific comorbidities were also at increased risk for admissions, including those with peripheral vascular disease, renal failure, lymphoma, fluid and electrolyte disorders, and anemia. Because this is the first study viewing readmissions from the perspective of primary care practices, our findings are unique in the literature. However, hospital‐based studies have found similar relationships between readmission rates and these specific comorbidities.[11, 12, 15] Unlike other studies, our study cohort did not show CHF as an independent risk factor. We hypothesize this is because in 2008, UCSF Medical Center introduced an inpatient‐based intervention to reduce readmissions in patients with heart failure. By 2011, the readmission rates of patients with heart failure (primary or secondary diagnoses) had dropped by 30%. The success of this program, focused only on patients with heart failure, likely affected our analysis of comorbidities as risk factors for readmissions.
Models developed to predict hospital readmissions, overall and for specific disease conditions, have inconsistently identified predictive factors, and there is not a specific set of variables that dominate.[16, 17, 18, 19] A recent review of readmission risk prediction models suggested that models that take into account psychosocial factors such as social support, substance abuse, and functional status improved model performance.[16] We hypothesize that the reason why male gender was significant in our model may be related to lack of social support, especially among those who may be single or widowed. Other studies have also showed male gender as a predictor for hospital readmissions.[14, 20]
People with Medicare as the primary payor or Medicare with Medicaid also tended to have higher risk of readmission. We believe that this may be a proxy for the combined effect of age, multiple comorbidities, and psychosocial factors. In a multicenter study of general medicine inpatients, Medicare, but not age, was also found to be a predictive variable.[21] Unlike other studies, our study did not find Medicaid alone as a significant predictive variable for readmissions.[15, 21] One explanation may be that in hospital‐based studies, people with Medicaid who are at high risk for readmission may be high‐risk because they do not have a PCP or good access to outpatient care. In our study, all patients have a PCP in 1 of the UCSF clinics, and access to care is improved with this established relationship. These other studies did not examine Medicare‐Medicaid dual eligibility status. Our results are consistent with a national study on avoidable hospital admissions that showed the dual eligibility population experiencing 60% higher avoidable admission rates compared to the Medicaid‐only population.[22]
An interesting finding was that there were no statistically significant differences in readmissions among patients who report a language other than English as their primary language. Although language barriers and health literacy can affect a patient's ability to understand discharge instructions, the use of translators at UCSF Medical Center may have decreased the risk of readmissions. For a small number of patients whose primary language was not recorded (unknown language in our model), they appeared to have a lower risk of readmissions. Language preferences are recorded by our admitting staff during the process of admission. This step may have been skipped after hours or if the patient was not able to answer the question. However, we do not have a good hypothesis as to why these patients may have a reduced risk of readmissions.
Having a departed PCP in the General Internal Medicine clinic was an independent predictor of readmissions. These patients have access to primary care; they can schedule acute appointments with covering providers for new medical issues or follow‐up of chronic conditions. However, until they are transferred to a new PCP, they do not have a provider who is proactively managing their preventive and chronic disease care, including follow‐up and coordinating care after hospital discharge. Our study is not the first to suggest adverse outcomes for patients who are in transition from 1 primary care provider to the next.[23] Studies conducted in General Internal Medicine clinics have shown missed opportunities for cancer screening and overlooked test results during the transition period,[24] and as many as one‐fifth of patients whom residents identified as high‐risk were lost to follow‐up.[25] However, our study is the first to show the link between PCP transition in a teaching clinic and hospital readmissions. This finding underscores the importance of continuity of care in the optimal management of patients following hospital discharge.
There are several limitations to this study. First, our study only considers hospitalizations to UCSF Medical Center, potentially undercounting readmissions to area hospitals. Because our study population are patients with PCPs at UCSF, these patients tend to seek specialty and acute care at UCSF Medical Center as well. We obtained payor data from our medical group (Hill Physicians), which covers our largest private payors, to understand whether our results can be applied on a global basis. We found that in 87.4% of index admissions with readmissions from January 1, 2010 through May 30, 2012, the readmission occurred at UCSF Medical Center. We conducted a similar analysis with CMS data from October 1, 2008 to June 30, 2011, the latest data we have from CMS. For patients with UCSF PCPs and a diagnosis of AMI or CHF, 100% were readmitted back to UCSF Medical Center. For patients with UCSF PCPs and a diagnosis of pneumonia, 89% were readmitted back to UCSF Medical Center. Given that only a small percentage of patients with PCPs at UCSF present for readmission at other area hospitals, we believe that limiting our analysis to UCSF Medical Center is reasonable.
In our study, we did not remove vaginal deliveries or Caesarian sections prior to building the model. Primary care physicians and their clinic leadership are accustomed to taking a population‐health perspective. We anticipate they would be interested in designing interventions and addressing readmission for the entire primary care panel. Although readmissions after delivery are not frequent, they can still occur, and interventions should not necessarily exclude this population. We did run a sensitivity analysis by removing vaginal delivery and Caesarian sections from the analysis. As expected, readmission rates for all practices increased except for Geriatrics. However, the independent predictors of readmissions did not change.
Our study is based on a population of patients at 1 urban academic medical center and may not be generalizable across all delivery systems. Our population is racially and ethnically diverse, and many do not speak English as their primary language. The study also spans different types of primary care clinics, capturing a wide range of ages and case mix. As PCP assignments fluctuate over time, there may be errors with PCP attribution in the UCSF Medical Center data systems. We do not believe errors in PCP attribution would differ across primary care practices. Because the primary care practices' performance reports on quality measures are based on PCP assignment, each clinic regularly updates their clinic panels and has specific protocols to address errors with PCP attribution.
Finally, our study includes only the variables that we were able to extract from administrative claims. Other explanatory variables that have been suggested as important for evaluation, such as social support, functional assessment, access to care, hospital discharge process, and posthospitalization follow‐up, were not included. Each of these could be explored in future studies.
This study offers a unique perspective of hospital readmission by introducing a new methodology for primary care clinics to calculate and evaluate their all‐cause 30‐day readmission rates. Although this methodology is not intended to provide real‐time feedback to clinics on readmitted patients, it opens the door for benchmarking based on specific case‐mix indices. Another direction for future research is to design robust evaluations of the impact of interventions, both inpatient and outpatient, on primary care clinic readmission rates. Finally, future research should replicate this analysis across teaching clinics to identify whether provider turnover is a consistent independent predictor of hospital readmissions.
This study also has implications for inpatient interventions. For example, discharging physicians may consider proactively identifying whether the patient has a continuous primary care provider. Patients who are in between PCPs may need closer follow‐up after discharge, until they re‐establish with a new PCP. This can be accomplished through a postdischarge clinic visit, either run by inpatient providers or covering physicians in the primary care clinic. In addition, the discharging physician may work with the case manager to increase the level of care coordination. The case manager could contact the primary care clinic and proactively ask for immediate PCP re‐assignment. Once a new PCP has been identified, the discharging physician could consider a warm hand‐off. With a warm hand‐off, the new PCP may feel more comfortable managing problems that may arise after hospital discharge, and especially before the first outpatient visit with the new PCP. Future research can test whether these interventions could effectively reduce hospital readmissions across a broad primary care population.
CONCLUSION
Primary care providers and their clinics play an important role in managing population health, decreasing healthcare spending, and keeping patients out of the hospital. In this study, we introduce a tool in which primary care clinics can begin to understand their hospital readmission rates. This may be particularly valuable as primary care providers enter global payment arrangements such as accountable care organizations or bundled payments and are responsible for a population of patients across the continuum of care. We found significant variation in readmission rates between different primary care practices, but much of this variation appears to be due to differences between practices in patient demographics, comorbidities, and hospitalization factors. Our study is the first to show the association between provider transitions and higher hospital readmissions. Continuity of care is critical for the optimal management of patients following hospital discharge. More attention will need to be focused on providing good continuity outpatient care for patients at high risk for readmissions.
Acknowledgements
The authors acknowledge Janelle Lee, MBA, MHA, DrPH, for her assistance with extracting the hospital claims data at UCSF Medical Center.
Disclosure: Dr. Tang and Ms. Maselli were supported by a University of California, Center for Health Quality and Innovation grant. The authors report no conflicts of interest.
- Medicare's new hospital value‐based purchasing program is likely to have only a small impact on hospital payments. Health Aff. 2012;31:1932–1940. , .
- Contemporary evidence about hospital strategies for reducing 30‐day readmissions. J Am Coll Cardiol. 2012;60:607–614. , , , et al.
- Interventions to reduce 30‐day rehospitalization: a systematic review. Ann Intern Med. 2011;155:520–528. , , , , .
- Reducing hospital readmissions: lessons from top‐performing hospitals. The Commonwealth Fund Synthesis Report. Available at: http://www.commonwealthfund.org/publications/case‐studies/2011/apr/reducing‐hospital‐readmissions. Published April 6, 2011. Accessed February 8, 2013. , , .
- Effective Interventions to Reduce Rehospitalizations: A Survey of the Published Evidence. Cambridge, MA: Institute for Healthcare Improvement; 2009. , .
- Recasting readmissions by placing the hospital role in community context. JAMA. 2013;309:351–352. , , .
- Hospitalists and care transitions: the divorce of inpatient and outpatient care. Health Affairs. 2008;27:1315–1327. , , , .
- Center for Medicare and Medicaid Innovation. Community‐based Care Transitions Program. Available at: http://innovation.cms.gov/initiatives/CCTP/#collapse‐tableDetails. Accessed February 8, 2013.
- Medicare's transitional care payment—a step toward the medical home. N Engl J Med. 2013;368:692–694. , , .
- Volume thresholds and hospital characteristics in the United States. Health Aff (Millwood). 2003;22:167–177. , , .
- Thirty‐day rehospitalizations after acute myocardial infarction: a cohort study. Ann Intern Med. 2012;157:11–18. , , , , , .
- Rehospitalizations among patients in the Medicare fee‐for‐service program. N Engl J Med. 2009;360:1418–1428. , , .
- Associations between reduced hospital length of stay and 30‐day readmission rate and mortality: 14‐year experience in 129 Veterans Affairs hospitals. Ann Intern Med. 2012;157:837–845. , , , et al.
- Readmission after hospitalization for congestive heart failure among Medicare beneficiaries. Arch Intern Med. 1997;157:99–104. , , , et al.
- Redefining readmission risk factors for general medicine patients. J Hosp Med. 2011;6:54–60. , , , .
- Risk prediction models for hospital readmission: a systematic review. JAMA. 2011;206:1688–1698. , , , et al.
- Statistical models and patient predictors of readmission for heart failure: a systematic review. Arch Intern Med. 2008;168:1371–1386. , , , et al.
- Statistical models and patient predictors of readmission for acute myocardial infarction: a systematic review. Circ Cardiovasc Qual Outcomes. 2009;2:500–507. , , , .
- Predictors of hospital readmission after stroke: a systematic review. Stroke. 2010;41:2525–2533. , , , et al.
- Risk factors for 30‐day hospital readmission in patients ≥65 years of age. Proc (Bayl Univ Med Cent). 2008;21:363–372. , , , , .
- Hospital readmission in general medicine patients: a prediction model. J Gen Intern Med. 2010;25:211–219. , , , et al.
- Users of Medicaid home and community‐based services are especially vulnerable to costly avoidable hospital admissions. Health Affairs. 2012;31:1167–1175. , , .
- Academic year‐end transfers of outpatients from outgoing to incoming residents: an unaddressed patient safety issue. JAMA. 2009;302:1327–1329. , .
- The revolving door of resident continuity practice: identifying gaps in transitions of care. J Gen Intern Med. 2011;26:995–998. , , , , , .
- Outcomes for resident‐identified high‐risk patients and resident perspectives of year‐end continuity clinic handoffs. J Gen Intern Med. 2012;27:1438–1444. , , , et al.
Reducing hospital readmissions is a national healthcare priority. In October 2012, the Centers for Medicare and Medicaid Services (CMS) enacted financial penalties on hospitals with higher than average risk‐adjusted readmissions, offering an incentive pool of $850 million in the first year.[1] As a result, a wide range of activities to understand and reduce readmissions among patients with congestive heart failure (CHF), acute myocardial infarction (AMI), and pneumonia have emerged.[2, 3, 4, 5] Some have proposed that to effectively reduce hospital readmissions, a community of inpatient and outpatient providers and local support organizations must coordinate efforts.[6, 7] In fact, CMS has recognized the important role of community support groups and outpatient providers in safe discharge transitions in 2 ways. First, in 2011 CMS launched the Community‐based Care Transitions Program to fund community‐based organizations to assist Medicare patients with care transitions.[8] Second, CMS introduced 2 new reimbursement codes for primary care providers (PCPs) to perform care coordination immediately after hospital discharge.[9] Both of these new payment programs represent an evolving perspective that reducing hospital readmissions requires active participation among outpatient partners.
As leaders of the outpatient care team, PCPs play a significant role in reducing hospital readmissions. One way in which PCPs can begin to understand the magnitude of the issue within their clinic is to evaluate the clinic's 30‐day readmission rates. Currently, CMS calculates readmission rates at the hospital level. However, understanding these rates at the clinic level is critical for developing strategies for improvement across the care continuum. Our current understanding of effective outpatient interventions to reduce hospital readmission is limited.[3] As clinics introduce and refine strategies to reduce readmissions, tracking the impact of these strategies on readmission rates will be critical for identifying effective outpatient interventions. Clinics with similar patient case‐mix can also benchmark readmission rates, sharing best practices from clinics with lower‐than‐expected rates.
There are no available studies or proposed methodologies to guide primary care clinics in calculating their 30‐day readmission rates. A particularly difficult challenge is obtaining the admission information when patients may be admitted to 1 of several area hospitals. For large integrated delivery networks where primary care patients are relatively loyal to the network of physicians and hospitals, an opportunity exists to explore the data. Furthermore, variations in readmission rates across primary care specialties (such as internal medicine and family practice) are not well understood. In this study, we set out to develop a methodology for calculating all‐cause 30‐day hospital readmission rates at the level of individual primary care practices and to identify factors associated with variations in these rates.
METHODS
Study Design
We conducted a retrospective observational study of adult primary care patients at the University of California, San Francisco (UCSF) who were hospitalized at UCSF Medical Center between July 1, 2009 and June 30, 2012. UCSF Medical Center is comprised of Moffitt‐Long Hospital (a 600‐bed facility) and UCSF‐Mount Zion Hospital (a 90‐bed facility) located in San Francisco, CA. The patient population was limited to adults ages 18 and over with a PCP at UCSF. UCSF has 7 adult primary care clinics: General Internal Medicine (IM), Family Practice (FP), Women's Health, Geriatrics, a combined IM/FP clinic, Human Immunodeficiency Virus (HIV) Primary Care, and a Concierge Internal Medicine clinic staffed by IM physicians. Between 2009 and 2011, all clinics completed the process of enpanelment, or defining the population of patients for which each PCP and the clinic is responsible. We obtained the list of patient and PCP assignments at each of the 7 clinics across the time period of study. We then obtained UCSF Medical Center hospital claims data for this group, including dates of admission and discharge, patient age, sex, race/ethnicity, language, insurance, admitting service, diagnosis codes, information on intensive care unit stay, and discharge disposition. Hospital claims data are housed in Transition Systems International (Boston, MA) administrative databases, a cost‐accounting system that collects data abstracted from patient charts upon discharge from UCSF Medical Center.
All‐cause 30‐day hospital readmission rates were calculated for each primary care clinic, using an adaptation of the CMS definition. First, we defined index discharges as the first discharge for an individual patient in any given 30‐day interval. Only 1 index discharge is flagged for each 30‐day interval. The first admission within 30 days after the index discharge was flagged as the readmission. Consistent with CMS methodology, only the first readmission in the 30‐day period was counted. We included all inpatient and observation status admissions and excluded patients who died during the index encounter, left against medical advice, or transferred to another acute care hospital after the index encounter.
We used secondary diagnosis codes in the administrative data to classify comorbidities by the Elixhauser methodology.[10] All but 1 adult primary care clinic at UCSF are faculty‐only clinics, whereby the assigned PCP is an attending physician. In the general internal medicine clinic, an attending or a resident can serve as the PCP, and approximately 20% of IM clinic patients have a resident as their PCP. For the IM clinic, we classified patients' PCP as attending, resident, or departed. The latter category refers to patients whose PCPs were residents who had graduated or faculty who had departed and had not been assigned to a new PCP prior to the index admission or readmission.
Statistical Analysis
We built a model to predict readmissions using the demographic and clinical variables with [2] P<0.20 in an initial bivariate analysis, and then removed, with backward selection, the least significant variables until only those with P0.05 remained. Age, log‐length of stay (LOS), and intensive care unit stay were forced in the model, as studies evaluating factors related to readmissions have often included these as important covariates.[11, 12, 13, 14] Results were expressed as adjusted odds ratio (OR) with 95% confidence interval (CI). All analyses were carried out using SAS version 9.2 (SAS Institute, Inc., Cary, NC).
This study was exempt from review by the institutional review board of UCSF.
RESULTS
During the study period, there were 12,564 discharges from UCSF Medical Center for primary care patients belonging to the 7 clinics. Of these, 8685 were index discharges and 1032 were readmissions within 30 days. Table 1 shows the characteristics of the patients who had at least 1 admission during the study period. In all but 2 clinics (HIV Primary Care and Concierge Internal Medicine), there were more women hospitalized than men. Age and gender differences across clinics are consistent with the patient populations served by these clinics, with Women's Health having more female patients and younger patients hospitalized compared to Geriatrics.
General Internal Medicine | Family Practice | Women's Health | Geriatrics | Combined IM/FP | HIV Primary Care | Concierge IM | |
---|---|---|---|---|---|---|---|
| |||||||
Panel size | 26,521 | 10,495 | 8,526 | 190 | 4,109 | 1,039 | 706 |
FTE attending MD and NP providers | 18.8 | 7.3 | 4.5 | 1.7 | 5.0 | 2.3 | 2.1 |
Mean panel size per FTE | 1,311 | 1,448 | 1,895 | 112 | 822 | 452 | 336 |
Clinic visits in FY 2012 | 50,362 | 20,647 | 11,014 | 4,425 | 8,120 | 4,182 | 1,713 |
No. of index discharges | 5,388 | 1,204 | 983 | 409 | 339 | 249 | 113 |
No. of readmissions | 718 | 104 | 76 | 56 | 30 | 37 | 11 |
No. of patients discharged during study period | 4,063 | 1,003 | 818 | 289* | 290 | 185 | 84 |
% Male | 40.1% | 33.9% | 4.3% | 35.3% | 33.8% | 69.7% | 52.4% |
Average age (SD), y | 60.4 (18.6) | 52.0 (19.0) | 47.0 (15.7) | 83.2 (6.9) | 46.3 (16.9) | 49.5 (9.9) | 64.5 (15.6) |
Age range, y | 19104 | 1896 | 1997 | 6399 | 1890 | 2273 | 2092 |
Race | |||||||
% White | 42.2% | 41.3% | 58.8% | 61.9% | 49.3% | 61.0% | 91.7% |
% Black | 16.3% | 8.5% | 7.5% | 7.3% | 10.0% | 27.6% | 0.0% |
% Asian | 23.6% | 31.4% | 19.0% | 17.0% | 16.6% | 2.2% | 4.8% |
% Native America/ Alaskan Native | 0.7% | 1.6% | 0.7% | 0.7% | 0.7% | 0.5% | 0.0% |
% Other | 16.3% | 15.7% | 13.1% | 12.5% | 18.3% | 8.1% | 2.4% |
Not available | 1.0% | 1.6% | 1.0% | 0.7% | 5.2% | 0.5% | 1.2% |
Ethnicity | |||||||
% Hispanic | 8.8% | 10.6% | 5.0% | 6.2% | 8.3% | 4.9% | 0.0% |
% Non‐Hispanic | 73.8% | 74.5% | 81.8% | 73.0% | 74.5% | 84.9% | 75.0% |
Not available | 17.4% | 15.0% | 13.2% | 20.8% | 17.2% | 10.3% | 25.0% |
Language | |||||||
% English | 69.7% | 75.6% | 88.8% | 69.6% | 85.9% | 89.7% | 89.3% |
% Spanish | 3.8% | 1.9% | 0.6% | 4.2% | 1.0% | 0.5% | 0.0% |
% Chinese (Mandarin or Cantonese) | 8.0% | 6.7% | 1.2% | 4.8% | 2.1% | 0.0% | 0.0% |
% Russian | 1.7% | 0.6% | 0.1% | 0.4% | 1.0% | 0.0% | 0.0% |
% Vietnamese | 1.0% | 1.4% | 0.0% | 0.4% | 0.0% | 0.0% | 0.0% |
% Other | 11.7% | 9.3% | 5.6% | 18.0% | 6.9% | 8.1% | 4.8% |
Not available | 4.1% | 4.6% | 3.7% | 2.8% | 3.1% | 1.6% | 6.0% |
Insurance type | |||||||
% Private | 35.4% | 58.7% | 77.4% | 10.7% | 63.8% | 36.8% | 66.7% |
% Medicare | 22.0% | 17.2% | 12.0% | 67.8% | 14.8% | 8.7% | 33.3% |
% Medicaid | 15.5% | 12.5% | 3.1% | 1.4% | 12.8% | 24.3% | 0.0% |
% Dual eligible | 24.4% | 8.8% | 5.4% | 19.4% | 6.6% | 27.6% | 0.0% |
% Self‐pay/other | 2.6% | 2.9% | 2.2% | 0.7% | 2.1% | 2.7% | 0.0% |
All‐cause 30‐day readmission rates varied across practices, with HIV Primary Care being the highest at 14.9%, followed by Geriatrics at 13.7%, General Internal Medicine at 13.3%, Concierge Internal Medicine at 9.7%, combined IM/FP at 8.9%, Family Practice at 8.6%, and Women's Health at 7.7% (Figure 1). Despite HIV Primary Care having the highest readmission rate, the number of index discharges during the 3‐year period was relatively low (249) compared to General Internal Medicine (5388). For the index admission, the top 5 admitting services were medicine, obstetrics, cardiology, orthopedic surgery, and general surgery (Table 2). Medicine was the primary admitting service for patients in the following clinics: General Internal Medicine, Geriatrics, HIV Primary Care, and Concierge Internal Medicine. Obstetrics was the primary admitting service for patients in the Family Practice, Women's Health, and combined IM/FP clinics. Vaginal delivery was the top discharge diagnosis‐related group (DRG) for patients in the General Internal Medicine, Family Practice, Women's Health, and combined IM/FP clinics. The top discharge DRG was urinary tract infection for Geriatrics, HIV for the HIV clinic (13.6%), and chemotherapy (8.0%) for Concierge Internal Medicine. Average LOS varied from 4.7 days for patients in the HIV Primary Care clinic to 2.8 days for patients in the Concierge Internal Medicine clinic. Average LOS was 3.4, 3.1, and 3.2 days in the Family Practice, Women's Health, and Geriatrics clinics, respectively, and 3.8 days in the General Internal Medicine and combined IM/FP clinics. For all clinics except Geriatrics, the majority of patients were discharged home without home health. A larger proportion of patients in the Geriatrics clinic were discharged home with home health or discharged to a skilled nursing facility.
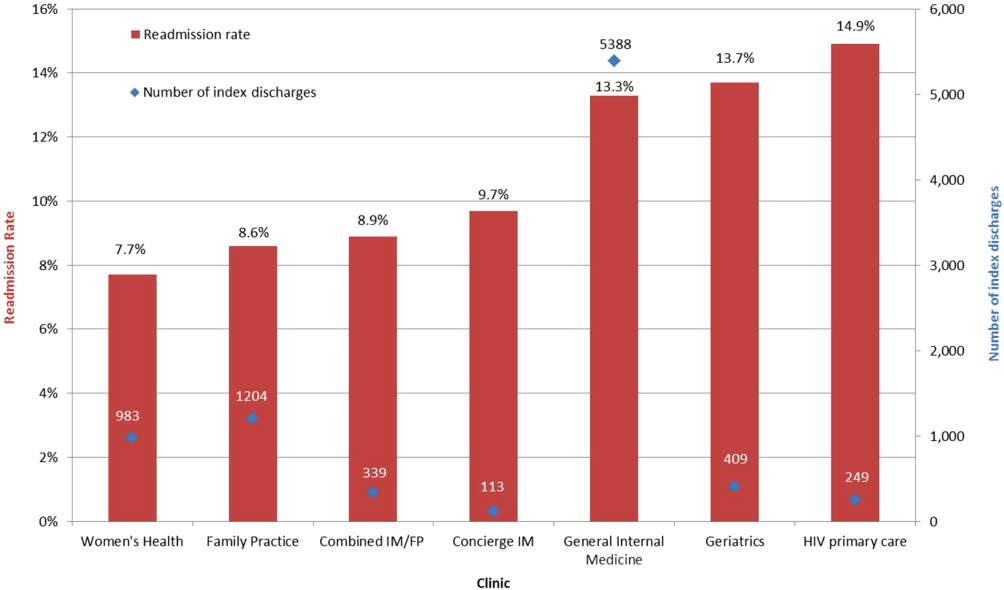
General Internal Medicine, N=5,388 | Family Practice, N=1,204 | Women's Health, N=983 | Geriatrics, N=409 | Combined IM/FP, N=339 | HIV Primary Care, N=249 | Concierge IM, N=113 | |
---|---|---|---|---|---|---|---|
| |||||||
Top 5 admitting services | |||||||
Medicine | 41.8% | 25.0% | 15.2% | 56.4% | 23.5% | 59.7% | 19.0% |
Obstetrics | 7.5% | 24.7% | 38.7% | 0.0% | 35.6% | 2.1% | 9.0% |
Cardiology | 15.1% | 10.9% | 6.2% | 16.5% | 10.3% | 6.5% | 17.0% |
Orthopedic surgery | 8.3% | 8.3% | 8.2% | 7.3% | 5.3% | 4.8% | 12.0% |
Adult general surgery | 7.2% | 9.8% | 10.0% | 6.0% | 5.0% | 5.8% | 7.0% |
Top 5 discharge diagnoses | |||||||
Vaginal delivery | 3.5% | 13.6% | 20.6% | 0.0% | 17.9% | 0.3% | 3.0% |
Major joint replacement | 3.5% | 3.4% | 4.8% | 4.3% | 3.8% | 0.7% | 5.0% |
Vaginal delivery with complications | 1.3% | 5.0% | 6.9% | 0.0% | 4.1% | 1.0% | 2.0% |
Simple pneumonia and pleurisy | 2.8% | 1.2% | 0.6% | 3.8% | 0.3% | 2.1% | 0.0% |
Urinary tract infection | 2.0% | 1.5% | 1.4% | 6.2% | 0.9% | 2.4% | 1.0% |
Discharge disposition | |||||||
% Home | 69.1% | 74.6% | 76.6% | 47.8% | 69.9% | 78.6% | 68.4% |
% Home with home health | 19.4% | 16.5% | 17.8% | 24.1% | 21.7% | 11.8% | 18.8% |
% Skilled nursing facility | 7.2% | 4.9% | 3.0% | 18.9% | 3.3% | 5.0% | 0.8% |
% Other | 4.3% | 4.0% | 2.6% | 9.2% | 5.1% | 4.6% | 12.0% |
Average length of stay (SD) | 3.8 (5.6) | 3.4 (7.5) | 3.1 (3.7) | 3.2 (3.7) | 3.8 (5.2) | 4.7 (6.5) | 2.8 (2.8) |
% Discharges with ICU stay | 11.0% | 10.6% | 5.9% | 8.5% | 9.2% | 11.1% | 15.8% |
Factors associated with variation in readmission rates included: male gender (OR: 1.21, 95% CI: 1.051.40), Medicare (OR: 1.31, 95% CI: 1.051.64; Ref=private) and dual‐eligible Medicare‐Medicaid insurance (OR: 1.26, 95% CI: 1.011.56), unknown primary language (OR: 0.06, 95% CI: 0.020.25; Ref=English), and the following comorbidities: peripheral vascular disease, renal failure, lymphoma, fluid and electrolyte disorders, and anemia. Multivariable logistic regression modeling results are listed in Table 3. Patients having a resident PCP showed no increased odds of readmission (OR: 1.13, 95% CI: 0.931.37; Ref=attending PCP). However, patients with a graduated resident PCP or departed faculty PCP awaiting transfer to a new PCP had an OR of 1.59 (95% CI: 1.162.17) compared with having a current faculty PCP. The C‐statistic for this model was 0.67.
Adjusted Odds Ratio (95% CI) | |
---|---|
| |
Age | |
<65 years | Ref |
>65 years | 0.91 (0.751.10) |
Clinic | |
General Internal Medicine | 1.24 (0.991.56) |
Family Practice | Ref |
Women's Health | 1.13 (0.821.56) |
Geriatrics | 1.20 (0.841.74) |
Combined Internal Medicine/Family Practice | 1.13 (0.731.74) |
HIV Primary Care | 1.24 (0.811.89) |
Concierge Internal Medicine | 1.06 (0.542.07) |
Sex | |
Female | Ref |
Male | 1.21 (1.051.40) |
Insurance | |
Private | Ref |
Medicaid | 1.09 (0.871.37) |
Medicare | 1.31 (1.051.64) |
Dual eligible MedicareMedicaid | 1.26 (1.011.56) |
Self/other | 1.20 (0.741.94) |
Language | |
English | Ref |
Spanish | 1.02 (0.701.47) |
Chinese | 1.13 (0.871.47) |
Other | 0.87 (0.701.08) |
Unknown | 0.06 (0.020.25) |
Comorbidities | |
Pulmonary circulation disease | 1.51 (0.982.32) |
Peripheral vascular disease | 1.50 (1.141.98) |
Renal failure | 1.31 (1.091.58) |
Lymphoma | 2.50 (1.434.36) |
Fluid and electrolyte disorders | 1.27 (1.071.52) |
Deficiency anemia | 1.25 (1.041.51) |
Physician | |
Attending | Ref |
Resident | 1.13 (0.931.37) |
Departed PCP (internal medicine only) | 1.59 (1.162.17) |
ICU stay | 1.03 (0.831.28) |
LOS (log) | 1.02 (0.981.06) |
Admitting service | |
Medicine | Ref |
Obstetrics | 0.41 (0.300.56) |
Cardiology | 1.12 (0.911.37) |
Orthopedic surgery | 0.36 (0.250.51) |
Adult general surgery | 0.64 (0.470.87) |
Other services | 0.76 (0.630.92) |
DISCUSSION
In this study we introduce a complementary way to view hospital readmissions, from the perspective of primary care practices. We found variation in readmission rates across primary care practices. After controlling for admitting service, clinic, provider, and patient factors, the specific characteristics of male gender, patients with Medicare or Medicare with Medicaid, and patients in our General Internal Medicine clinic with a departed PCP were independent risk factors for hospital readmission. Patients with specific comorbidities were also at increased risk for admissions, including those with peripheral vascular disease, renal failure, lymphoma, fluid and electrolyte disorders, and anemia. Because this is the first study viewing readmissions from the perspective of primary care practices, our findings are unique in the literature. However, hospital‐based studies have found similar relationships between readmission rates and these specific comorbidities.[11, 12, 15] Unlike other studies, our study cohort did not show CHF as an independent risk factor. We hypothesize this is because in 2008, UCSF Medical Center introduced an inpatient‐based intervention to reduce readmissions in patients with heart failure. By 2011, the readmission rates of patients with heart failure (primary or secondary diagnoses) had dropped by 30%. The success of this program, focused only on patients with heart failure, likely affected our analysis of comorbidities as risk factors for readmissions.
Models developed to predict hospital readmissions, overall and for specific disease conditions, have inconsistently identified predictive factors, and there is not a specific set of variables that dominate.[16, 17, 18, 19] A recent review of readmission risk prediction models suggested that models that take into account psychosocial factors such as social support, substance abuse, and functional status improved model performance.[16] We hypothesize that the reason why male gender was significant in our model may be related to lack of social support, especially among those who may be single or widowed. Other studies have also showed male gender as a predictor for hospital readmissions.[14, 20]
People with Medicare as the primary payor or Medicare with Medicaid also tended to have higher risk of readmission. We believe that this may be a proxy for the combined effect of age, multiple comorbidities, and psychosocial factors. In a multicenter study of general medicine inpatients, Medicare, but not age, was also found to be a predictive variable.[21] Unlike other studies, our study did not find Medicaid alone as a significant predictive variable for readmissions.[15, 21] One explanation may be that in hospital‐based studies, people with Medicaid who are at high risk for readmission may be high‐risk because they do not have a PCP or good access to outpatient care. In our study, all patients have a PCP in 1 of the UCSF clinics, and access to care is improved with this established relationship. These other studies did not examine Medicare‐Medicaid dual eligibility status. Our results are consistent with a national study on avoidable hospital admissions that showed the dual eligibility population experiencing 60% higher avoidable admission rates compared to the Medicaid‐only population.[22]
An interesting finding was that there were no statistically significant differences in readmissions among patients who report a language other than English as their primary language. Although language barriers and health literacy can affect a patient's ability to understand discharge instructions, the use of translators at UCSF Medical Center may have decreased the risk of readmissions. For a small number of patients whose primary language was not recorded (unknown language in our model), they appeared to have a lower risk of readmissions. Language preferences are recorded by our admitting staff during the process of admission. This step may have been skipped after hours or if the patient was not able to answer the question. However, we do not have a good hypothesis as to why these patients may have a reduced risk of readmissions.
Having a departed PCP in the General Internal Medicine clinic was an independent predictor of readmissions. These patients have access to primary care; they can schedule acute appointments with covering providers for new medical issues or follow‐up of chronic conditions. However, until they are transferred to a new PCP, they do not have a provider who is proactively managing their preventive and chronic disease care, including follow‐up and coordinating care after hospital discharge. Our study is not the first to suggest adverse outcomes for patients who are in transition from 1 primary care provider to the next.[23] Studies conducted in General Internal Medicine clinics have shown missed opportunities for cancer screening and overlooked test results during the transition period,[24] and as many as one‐fifth of patients whom residents identified as high‐risk were lost to follow‐up.[25] However, our study is the first to show the link between PCP transition in a teaching clinic and hospital readmissions. This finding underscores the importance of continuity of care in the optimal management of patients following hospital discharge.
There are several limitations to this study. First, our study only considers hospitalizations to UCSF Medical Center, potentially undercounting readmissions to area hospitals. Because our study population are patients with PCPs at UCSF, these patients tend to seek specialty and acute care at UCSF Medical Center as well. We obtained payor data from our medical group (Hill Physicians), which covers our largest private payors, to understand whether our results can be applied on a global basis. We found that in 87.4% of index admissions with readmissions from January 1, 2010 through May 30, 2012, the readmission occurred at UCSF Medical Center. We conducted a similar analysis with CMS data from October 1, 2008 to June 30, 2011, the latest data we have from CMS. For patients with UCSF PCPs and a diagnosis of AMI or CHF, 100% were readmitted back to UCSF Medical Center. For patients with UCSF PCPs and a diagnosis of pneumonia, 89% were readmitted back to UCSF Medical Center. Given that only a small percentage of patients with PCPs at UCSF present for readmission at other area hospitals, we believe that limiting our analysis to UCSF Medical Center is reasonable.
In our study, we did not remove vaginal deliveries or Caesarian sections prior to building the model. Primary care physicians and their clinic leadership are accustomed to taking a population‐health perspective. We anticipate they would be interested in designing interventions and addressing readmission for the entire primary care panel. Although readmissions after delivery are not frequent, they can still occur, and interventions should not necessarily exclude this population. We did run a sensitivity analysis by removing vaginal delivery and Caesarian sections from the analysis. As expected, readmission rates for all practices increased except for Geriatrics. However, the independent predictors of readmissions did not change.
Our study is based on a population of patients at 1 urban academic medical center and may not be generalizable across all delivery systems. Our population is racially and ethnically diverse, and many do not speak English as their primary language. The study also spans different types of primary care clinics, capturing a wide range of ages and case mix. As PCP assignments fluctuate over time, there may be errors with PCP attribution in the UCSF Medical Center data systems. We do not believe errors in PCP attribution would differ across primary care practices. Because the primary care practices' performance reports on quality measures are based on PCP assignment, each clinic regularly updates their clinic panels and has specific protocols to address errors with PCP attribution.
Finally, our study includes only the variables that we were able to extract from administrative claims. Other explanatory variables that have been suggested as important for evaluation, such as social support, functional assessment, access to care, hospital discharge process, and posthospitalization follow‐up, were not included. Each of these could be explored in future studies.
This study offers a unique perspective of hospital readmission by introducing a new methodology for primary care clinics to calculate and evaluate their all‐cause 30‐day readmission rates. Although this methodology is not intended to provide real‐time feedback to clinics on readmitted patients, it opens the door for benchmarking based on specific case‐mix indices. Another direction for future research is to design robust evaluations of the impact of interventions, both inpatient and outpatient, on primary care clinic readmission rates. Finally, future research should replicate this analysis across teaching clinics to identify whether provider turnover is a consistent independent predictor of hospital readmissions.
This study also has implications for inpatient interventions. For example, discharging physicians may consider proactively identifying whether the patient has a continuous primary care provider. Patients who are in between PCPs may need closer follow‐up after discharge, until they re‐establish with a new PCP. This can be accomplished through a postdischarge clinic visit, either run by inpatient providers or covering physicians in the primary care clinic. In addition, the discharging physician may work with the case manager to increase the level of care coordination. The case manager could contact the primary care clinic and proactively ask for immediate PCP re‐assignment. Once a new PCP has been identified, the discharging physician could consider a warm hand‐off. With a warm hand‐off, the new PCP may feel more comfortable managing problems that may arise after hospital discharge, and especially before the first outpatient visit with the new PCP. Future research can test whether these interventions could effectively reduce hospital readmissions across a broad primary care population.
CONCLUSION
Primary care providers and their clinics play an important role in managing population health, decreasing healthcare spending, and keeping patients out of the hospital. In this study, we introduce a tool in which primary care clinics can begin to understand their hospital readmission rates. This may be particularly valuable as primary care providers enter global payment arrangements such as accountable care organizations or bundled payments and are responsible for a population of patients across the continuum of care. We found significant variation in readmission rates between different primary care practices, but much of this variation appears to be due to differences between practices in patient demographics, comorbidities, and hospitalization factors. Our study is the first to show the association between provider transitions and higher hospital readmissions. Continuity of care is critical for the optimal management of patients following hospital discharge. More attention will need to be focused on providing good continuity outpatient care for patients at high risk for readmissions.
Acknowledgements
The authors acknowledge Janelle Lee, MBA, MHA, DrPH, for her assistance with extracting the hospital claims data at UCSF Medical Center.
Disclosure: Dr. Tang and Ms. Maselli were supported by a University of California, Center for Health Quality and Innovation grant. The authors report no conflicts of interest.
Reducing hospital readmissions is a national healthcare priority. In October 2012, the Centers for Medicare and Medicaid Services (CMS) enacted financial penalties on hospitals with higher than average risk‐adjusted readmissions, offering an incentive pool of $850 million in the first year.[1] As a result, a wide range of activities to understand and reduce readmissions among patients with congestive heart failure (CHF), acute myocardial infarction (AMI), and pneumonia have emerged.[2, 3, 4, 5] Some have proposed that to effectively reduce hospital readmissions, a community of inpatient and outpatient providers and local support organizations must coordinate efforts.[6, 7] In fact, CMS has recognized the important role of community support groups and outpatient providers in safe discharge transitions in 2 ways. First, in 2011 CMS launched the Community‐based Care Transitions Program to fund community‐based organizations to assist Medicare patients with care transitions.[8] Second, CMS introduced 2 new reimbursement codes for primary care providers (PCPs) to perform care coordination immediately after hospital discharge.[9] Both of these new payment programs represent an evolving perspective that reducing hospital readmissions requires active participation among outpatient partners.
As leaders of the outpatient care team, PCPs play a significant role in reducing hospital readmissions. One way in which PCPs can begin to understand the magnitude of the issue within their clinic is to evaluate the clinic's 30‐day readmission rates. Currently, CMS calculates readmission rates at the hospital level. However, understanding these rates at the clinic level is critical for developing strategies for improvement across the care continuum. Our current understanding of effective outpatient interventions to reduce hospital readmission is limited.[3] As clinics introduce and refine strategies to reduce readmissions, tracking the impact of these strategies on readmission rates will be critical for identifying effective outpatient interventions. Clinics with similar patient case‐mix can also benchmark readmission rates, sharing best practices from clinics with lower‐than‐expected rates.
There are no available studies or proposed methodologies to guide primary care clinics in calculating their 30‐day readmission rates. A particularly difficult challenge is obtaining the admission information when patients may be admitted to 1 of several area hospitals. For large integrated delivery networks where primary care patients are relatively loyal to the network of physicians and hospitals, an opportunity exists to explore the data. Furthermore, variations in readmission rates across primary care specialties (such as internal medicine and family practice) are not well understood. In this study, we set out to develop a methodology for calculating all‐cause 30‐day hospital readmission rates at the level of individual primary care practices and to identify factors associated with variations in these rates.
METHODS
Study Design
We conducted a retrospective observational study of adult primary care patients at the University of California, San Francisco (UCSF) who were hospitalized at UCSF Medical Center between July 1, 2009 and June 30, 2012. UCSF Medical Center is comprised of Moffitt‐Long Hospital (a 600‐bed facility) and UCSF‐Mount Zion Hospital (a 90‐bed facility) located in San Francisco, CA. The patient population was limited to adults ages 18 and over with a PCP at UCSF. UCSF has 7 adult primary care clinics: General Internal Medicine (IM), Family Practice (FP), Women's Health, Geriatrics, a combined IM/FP clinic, Human Immunodeficiency Virus (HIV) Primary Care, and a Concierge Internal Medicine clinic staffed by IM physicians. Between 2009 and 2011, all clinics completed the process of enpanelment, or defining the population of patients for which each PCP and the clinic is responsible. We obtained the list of patient and PCP assignments at each of the 7 clinics across the time period of study. We then obtained UCSF Medical Center hospital claims data for this group, including dates of admission and discharge, patient age, sex, race/ethnicity, language, insurance, admitting service, diagnosis codes, information on intensive care unit stay, and discharge disposition. Hospital claims data are housed in Transition Systems International (Boston, MA) administrative databases, a cost‐accounting system that collects data abstracted from patient charts upon discharge from UCSF Medical Center.
All‐cause 30‐day hospital readmission rates were calculated for each primary care clinic, using an adaptation of the CMS definition. First, we defined index discharges as the first discharge for an individual patient in any given 30‐day interval. Only 1 index discharge is flagged for each 30‐day interval. The first admission within 30 days after the index discharge was flagged as the readmission. Consistent with CMS methodology, only the first readmission in the 30‐day period was counted. We included all inpatient and observation status admissions and excluded patients who died during the index encounter, left against medical advice, or transferred to another acute care hospital after the index encounter.
We used secondary diagnosis codes in the administrative data to classify comorbidities by the Elixhauser methodology.[10] All but 1 adult primary care clinic at UCSF are faculty‐only clinics, whereby the assigned PCP is an attending physician. In the general internal medicine clinic, an attending or a resident can serve as the PCP, and approximately 20% of IM clinic patients have a resident as their PCP. For the IM clinic, we classified patients' PCP as attending, resident, or departed. The latter category refers to patients whose PCPs were residents who had graduated or faculty who had departed and had not been assigned to a new PCP prior to the index admission or readmission.
Statistical Analysis
We built a model to predict readmissions using the demographic and clinical variables with [2] P<0.20 in an initial bivariate analysis, and then removed, with backward selection, the least significant variables until only those with P0.05 remained. Age, log‐length of stay (LOS), and intensive care unit stay were forced in the model, as studies evaluating factors related to readmissions have often included these as important covariates.[11, 12, 13, 14] Results were expressed as adjusted odds ratio (OR) with 95% confidence interval (CI). All analyses were carried out using SAS version 9.2 (SAS Institute, Inc., Cary, NC).
This study was exempt from review by the institutional review board of UCSF.
RESULTS
During the study period, there were 12,564 discharges from UCSF Medical Center for primary care patients belonging to the 7 clinics. Of these, 8685 were index discharges and 1032 were readmissions within 30 days. Table 1 shows the characteristics of the patients who had at least 1 admission during the study period. In all but 2 clinics (HIV Primary Care and Concierge Internal Medicine), there were more women hospitalized than men. Age and gender differences across clinics are consistent with the patient populations served by these clinics, with Women's Health having more female patients and younger patients hospitalized compared to Geriatrics.
General Internal Medicine | Family Practice | Women's Health | Geriatrics | Combined IM/FP | HIV Primary Care | Concierge IM | |
---|---|---|---|---|---|---|---|
| |||||||
Panel size | 26,521 | 10,495 | 8,526 | 190 | 4,109 | 1,039 | 706 |
FTE attending MD and NP providers | 18.8 | 7.3 | 4.5 | 1.7 | 5.0 | 2.3 | 2.1 |
Mean panel size per FTE | 1,311 | 1,448 | 1,895 | 112 | 822 | 452 | 336 |
Clinic visits in FY 2012 | 50,362 | 20,647 | 11,014 | 4,425 | 8,120 | 4,182 | 1,713 |
No. of index discharges | 5,388 | 1,204 | 983 | 409 | 339 | 249 | 113 |
No. of readmissions | 718 | 104 | 76 | 56 | 30 | 37 | 11 |
No. of patients discharged during study period | 4,063 | 1,003 | 818 | 289* | 290 | 185 | 84 |
% Male | 40.1% | 33.9% | 4.3% | 35.3% | 33.8% | 69.7% | 52.4% |
Average age (SD), y | 60.4 (18.6) | 52.0 (19.0) | 47.0 (15.7) | 83.2 (6.9) | 46.3 (16.9) | 49.5 (9.9) | 64.5 (15.6) |
Age range, y | 19104 | 1896 | 1997 | 6399 | 1890 | 2273 | 2092 |
Race | |||||||
% White | 42.2% | 41.3% | 58.8% | 61.9% | 49.3% | 61.0% | 91.7% |
% Black | 16.3% | 8.5% | 7.5% | 7.3% | 10.0% | 27.6% | 0.0% |
% Asian | 23.6% | 31.4% | 19.0% | 17.0% | 16.6% | 2.2% | 4.8% |
% Native America/ Alaskan Native | 0.7% | 1.6% | 0.7% | 0.7% | 0.7% | 0.5% | 0.0% |
% Other | 16.3% | 15.7% | 13.1% | 12.5% | 18.3% | 8.1% | 2.4% |
Not available | 1.0% | 1.6% | 1.0% | 0.7% | 5.2% | 0.5% | 1.2% |
Ethnicity | |||||||
% Hispanic | 8.8% | 10.6% | 5.0% | 6.2% | 8.3% | 4.9% | 0.0% |
% Non‐Hispanic | 73.8% | 74.5% | 81.8% | 73.0% | 74.5% | 84.9% | 75.0% |
Not available | 17.4% | 15.0% | 13.2% | 20.8% | 17.2% | 10.3% | 25.0% |
Language | |||||||
% English | 69.7% | 75.6% | 88.8% | 69.6% | 85.9% | 89.7% | 89.3% |
% Spanish | 3.8% | 1.9% | 0.6% | 4.2% | 1.0% | 0.5% | 0.0% |
% Chinese (Mandarin or Cantonese) | 8.0% | 6.7% | 1.2% | 4.8% | 2.1% | 0.0% | 0.0% |
% Russian | 1.7% | 0.6% | 0.1% | 0.4% | 1.0% | 0.0% | 0.0% |
% Vietnamese | 1.0% | 1.4% | 0.0% | 0.4% | 0.0% | 0.0% | 0.0% |
% Other | 11.7% | 9.3% | 5.6% | 18.0% | 6.9% | 8.1% | 4.8% |
Not available | 4.1% | 4.6% | 3.7% | 2.8% | 3.1% | 1.6% | 6.0% |
Insurance type | |||||||
% Private | 35.4% | 58.7% | 77.4% | 10.7% | 63.8% | 36.8% | 66.7% |
% Medicare | 22.0% | 17.2% | 12.0% | 67.8% | 14.8% | 8.7% | 33.3% |
% Medicaid | 15.5% | 12.5% | 3.1% | 1.4% | 12.8% | 24.3% | 0.0% |
% Dual eligible | 24.4% | 8.8% | 5.4% | 19.4% | 6.6% | 27.6% | 0.0% |
% Self‐pay/other | 2.6% | 2.9% | 2.2% | 0.7% | 2.1% | 2.7% | 0.0% |
All‐cause 30‐day readmission rates varied across practices, with HIV Primary Care being the highest at 14.9%, followed by Geriatrics at 13.7%, General Internal Medicine at 13.3%, Concierge Internal Medicine at 9.7%, combined IM/FP at 8.9%, Family Practice at 8.6%, and Women's Health at 7.7% (Figure 1). Despite HIV Primary Care having the highest readmission rate, the number of index discharges during the 3‐year period was relatively low (249) compared to General Internal Medicine (5388). For the index admission, the top 5 admitting services were medicine, obstetrics, cardiology, orthopedic surgery, and general surgery (Table 2). Medicine was the primary admitting service for patients in the following clinics: General Internal Medicine, Geriatrics, HIV Primary Care, and Concierge Internal Medicine. Obstetrics was the primary admitting service for patients in the Family Practice, Women's Health, and combined IM/FP clinics. Vaginal delivery was the top discharge diagnosis‐related group (DRG) for patients in the General Internal Medicine, Family Practice, Women's Health, and combined IM/FP clinics. The top discharge DRG was urinary tract infection for Geriatrics, HIV for the HIV clinic (13.6%), and chemotherapy (8.0%) for Concierge Internal Medicine. Average LOS varied from 4.7 days for patients in the HIV Primary Care clinic to 2.8 days for patients in the Concierge Internal Medicine clinic. Average LOS was 3.4, 3.1, and 3.2 days in the Family Practice, Women's Health, and Geriatrics clinics, respectively, and 3.8 days in the General Internal Medicine and combined IM/FP clinics. For all clinics except Geriatrics, the majority of patients were discharged home without home health. A larger proportion of patients in the Geriatrics clinic were discharged home with home health or discharged to a skilled nursing facility.
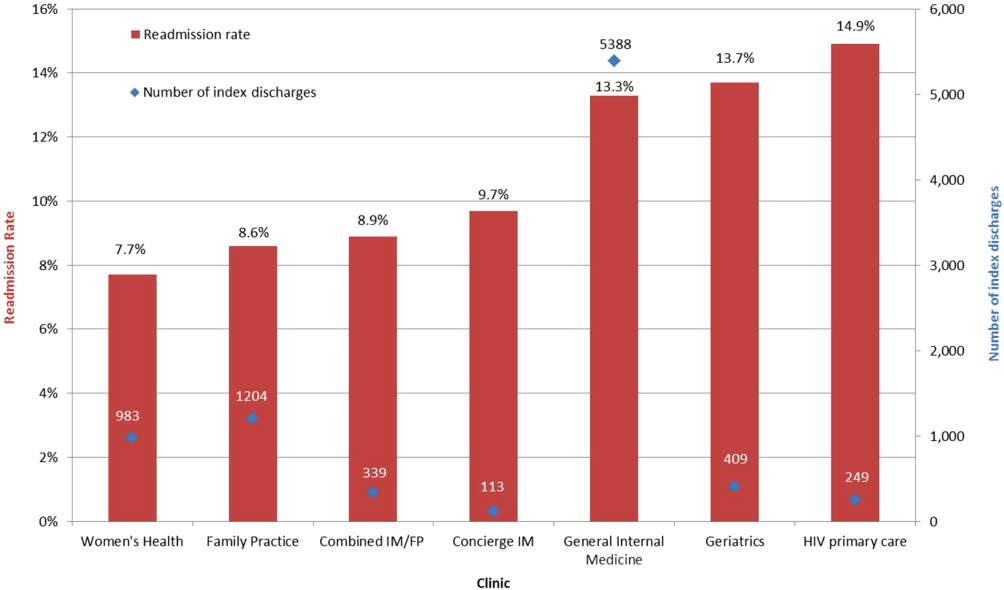
General Internal Medicine, N=5,388 | Family Practice, N=1,204 | Women's Health, N=983 | Geriatrics, N=409 | Combined IM/FP, N=339 | HIV Primary Care, N=249 | Concierge IM, N=113 | |
---|---|---|---|---|---|---|---|
| |||||||
Top 5 admitting services | |||||||
Medicine | 41.8% | 25.0% | 15.2% | 56.4% | 23.5% | 59.7% | 19.0% |
Obstetrics | 7.5% | 24.7% | 38.7% | 0.0% | 35.6% | 2.1% | 9.0% |
Cardiology | 15.1% | 10.9% | 6.2% | 16.5% | 10.3% | 6.5% | 17.0% |
Orthopedic surgery | 8.3% | 8.3% | 8.2% | 7.3% | 5.3% | 4.8% | 12.0% |
Adult general surgery | 7.2% | 9.8% | 10.0% | 6.0% | 5.0% | 5.8% | 7.0% |
Top 5 discharge diagnoses | |||||||
Vaginal delivery | 3.5% | 13.6% | 20.6% | 0.0% | 17.9% | 0.3% | 3.0% |
Major joint replacement | 3.5% | 3.4% | 4.8% | 4.3% | 3.8% | 0.7% | 5.0% |
Vaginal delivery with complications | 1.3% | 5.0% | 6.9% | 0.0% | 4.1% | 1.0% | 2.0% |
Simple pneumonia and pleurisy | 2.8% | 1.2% | 0.6% | 3.8% | 0.3% | 2.1% | 0.0% |
Urinary tract infection | 2.0% | 1.5% | 1.4% | 6.2% | 0.9% | 2.4% | 1.0% |
Discharge disposition | |||||||
% Home | 69.1% | 74.6% | 76.6% | 47.8% | 69.9% | 78.6% | 68.4% |
% Home with home health | 19.4% | 16.5% | 17.8% | 24.1% | 21.7% | 11.8% | 18.8% |
% Skilled nursing facility | 7.2% | 4.9% | 3.0% | 18.9% | 3.3% | 5.0% | 0.8% |
% Other | 4.3% | 4.0% | 2.6% | 9.2% | 5.1% | 4.6% | 12.0% |
Average length of stay (SD) | 3.8 (5.6) | 3.4 (7.5) | 3.1 (3.7) | 3.2 (3.7) | 3.8 (5.2) | 4.7 (6.5) | 2.8 (2.8) |
% Discharges with ICU stay | 11.0% | 10.6% | 5.9% | 8.5% | 9.2% | 11.1% | 15.8% |
Factors associated with variation in readmission rates included: male gender (OR: 1.21, 95% CI: 1.051.40), Medicare (OR: 1.31, 95% CI: 1.051.64; Ref=private) and dual‐eligible Medicare‐Medicaid insurance (OR: 1.26, 95% CI: 1.011.56), unknown primary language (OR: 0.06, 95% CI: 0.020.25; Ref=English), and the following comorbidities: peripheral vascular disease, renal failure, lymphoma, fluid and electrolyte disorders, and anemia. Multivariable logistic regression modeling results are listed in Table 3. Patients having a resident PCP showed no increased odds of readmission (OR: 1.13, 95% CI: 0.931.37; Ref=attending PCP). However, patients with a graduated resident PCP or departed faculty PCP awaiting transfer to a new PCP had an OR of 1.59 (95% CI: 1.162.17) compared with having a current faculty PCP. The C‐statistic for this model was 0.67.
Adjusted Odds Ratio (95% CI) | |
---|---|
| |
Age | |
<65 years | Ref |
>65 years | 0.91 (0.751.10) |
Clinic | |
General Internal Medicine | 1.24 (0.991.56) |
Family Practice | Ref |
Women's Health | 1.13 (0.821.56) |
Geriatrics | 1.20 (0.841.74) |
Combined Internal Medicine/Family Practice | 1.13 (0.731.74) |
HIV Primary Care | 1.24 (0.811.89) |
Concierge Internal Medicine | 1.06 (0.542.07) |
Sex | |
Female | Ref |
Male | 1.21 (1.051.40) |
Insurance | |
Private | Ref |
Medicaid | 1.09 (0.871.37) |
Medicare | 1.31 (1.051.64) |
Dual eligible MedicareMedicaid | 1.26 (1.011.56) |
Self/other | 1.20 (0.741.94) |
Language | |
English | Ref |
Spanish | 1.02 (0.701.47) |
Chinese | 1.13 (0.871.47) |
Other | 0.87 (0.701.08) |
Unknown | 0.06 (0.020.25) |
Comorbidities | |
Pulmonary circulation disease | 1.51 (0.982.32) |
Peripheral vascular disease | 1.50 (1.141.98) |
Renal failure | 1.31 (1.091.58) |
Lymphoma | 2.50 (1.434.36) |
Fluid and electrolyte disorders | 1.27 (1.071.52) |
Deficiency anemia | 1.25 (1.041.51) |
Physician | |
Attending | Ref |
Resident | 1.13 (0.931.37) |
Departed PCP (internal medicine only) | 1.59 (1.162.17) |
ICU stay | 1.03 (0.831.28) |
LOS (log) | 1.02 (0.981.06) |
Admitting service | |
Medicine | Ref |
Obstetrics | 0.41 (0.300.56) |
Cardiology | 1.12 (0.911.37) |
Orthopedic surgery | 0.36 (0.250.51) |
Adult general surgery | 0.64 (0.470.87) |
Other services | 0.76 (0.630.92) |
DISCUSSION
In this study we introduce a complementary way to view hospital readmissions, from the perspective of primary care practices. We found variation in readmission rates across primary care practices. After controlling for admitting service, clinic, provider, and patient factors, the specific characteristics of male gender, patients with Medicare or Medicare with Medicaid, and patients in our General Internal Medicine clinic with a departed PCP were independent risk factors for hospital readmission. Patients with specific comorbidities were also at increased risk for admissions, including those with peripheral vascular disease, renal failure, lymphoma, fluid and electrolyte disorders, and anemia. Because this is the first study viewing readmissions from the perspective of primary care practices, our findings are unique in the literature. However, hospital‐based studies have found similar relationships between readmission rates and these specific comorbidities.[11, 12, 15] Unlike other studies, our study cohort did not show CHF as an independent risk factor. We hypothesize this is because in 2008, UCSF Medical Center introduced an inpatient‐based intervention to reduce readmissions in patients with heart failure. By 2011, the readmission rates of patients with heart failure (primary or secondary diagnoses) had dropped by 30%. The success of this program, focused only on patients with heart failure, likely affected our analysis of comorbidities as risk factors for readmissions.
Models developed to predict hospital readmissions, overall and for specific disease conditions, have inconsistently identified predictive factors, and there is not a specific set of variables that dominate.[16, 17, 18, 19] A recent review of readmission risk prediction models suggested that models that take into account psychosocial factors such as social support, substance abuse, and functional status improved model performance.[16] We hypothesize that the reason why male gender was significant in our model may be related to lack of social support, especially among those who may be single or widowed. Other studies have also showed male gender as a predictor for hospital readmissions.[14, 20]
People with Medicare as the primary payor or Medicare with Medicaid also tended to have higher risk of readmission. We believe that this may be a proxy for the combined effect of age, multiple comorbidities, and psychosocial factors. In a multicenter study of general medicine inpatients, Medicare, but not age, was also found to be a predictive variable.[21] Unlike other studies, our study did not find Medicaid alone as a significant predictive variable for readmissions.[15, 21] One explanation may be that in hospital‐based studies, people with Medicaid who are at high risk for readmission may be high‐risk because they do not have a PCP or good access to outpatient care. In our study, all patients have a PCP in 1 of the UCSF clinics, and access to care is improved with this established relationship. These other studies did not examine Medicare‐Medicaid dual eligibility status. Our results are consistent with a national study on avoidable hospital admissions that showed the dual eligibility population experiencing 60% higher avoidable admission rates compared to the Medicaid‐only population.[22]
An interesting finding was that there were no statistically significant differences in readmissions among patients who report a language other than English as their primary language. Although language barriers and health literacy can affect a patient's ability to understand discharge instructions, the use of translators at UCSF Medical Center may have decreased the risk of readmissions. For a small number of patients whose primary language was not recorded (unknown language in our model), they appeared to have a lower risk of readmissions. Language preferences are recorded by our admitting staff during the process of admission. This step may have been skipped after hours or if the patient was not able to answer the question. However, we do not have a good hypothesis as to why these patients may have a reduced risk of readmissions.
Having a departed PCP in the General Internal Medicine clinic was an independent predictor of readmissions. These patients have access to primary care; they can schedule acute appointments with covering providers for new medical issues or follow‐up of chronic conditions. However, until they are transferred to a new PCP, they do not have a provider who is proactively managing their preventive and chronic disease care, including follow‐up and coordinating care after hospital discharge. Our study is not the first to suggest adverse outcomes for patients who are in transition from 1 primary care provider to the next.[23] Studies conducted in General Internal Medicine clinics have shown missed opportunities for cancer screening and overlooked test results during the transition period,[24] and as many as one‐fifth of patients whom residents identified as high‐risk were lost to follow‐up.[25] However, our study is the first to show the link between PCP transition in a teaching clinic and hospital readmissions. This finding underscores the importance of continuity of care in the optimal management of patients following hospital discharge.
There are several limitations to this study. First, our study only considers hospitalizations to UCSF Medical Center, potentially undercounting readmissions to area hospitals. Because our study population are patients with PCPs at UCSF, these patients tend to seek specialty and acute care at UCSF Medical Center as well. We obtained payor data from our medical group (Hill Physicians), which covers our largest private payors, to understand whether our results can be applied on a global basis. We found that in 87.4% of index admissions with readmissions from January 1, 2010 through May 30, 2012, the readmission occurred at UCSF Medical Center. We conducted a similar analysis with CMS data from October 1, 2008 to June 30, 2011, the latest data we have from CMS. For patients with UCSF PCPs and a diagnosis of AMI or CHF, 100% were readmitted back to UCSF Medical Center. For patients with UCSF PCPs and a diagnosis of pneumonia, 89% were readmitted back to UCSF Medical Center. Given that only a small percentage of patients with PCPs at UCSF present for readmission at other area hospitals, we believe that limiting our analysis to UCSF Medical Center is reasonable.
In our study, we did not remove vaginal deliveries or Caesarian sections prior to building the model. Primary care physicians and their clinic leadership are accustomed to taking a population‐health perspective. We anticipate they would be interested in designing interventions and addressing readmission for the entire primary care panel. Although readmissions after delivery are not frequent, they can still occur, and interventions should not necessarily exclude this population. We did run a sensitivity analysis by removing vaginal delivery and Caesarian sections from the analysis. As expected, readmission rates for all practices increased except for Geriatrics. However, the independent predictors of readmissions did not change.
Our study is based on a population of patients at 1 urban academic medical center and may not be generalizable across all delivery systems. Our population is racially and ethnically diverse, and many do not speak English as their primary language. The study also spans different types of primary care clinics, capturing a wide range of ages and case mix. As PCP assignments fluctuate over time, there may be errors with PCP attribution in the UCSF Medical Center data systems. We do not believe errors in PCP attribution would differ across primary care practices. Because the primary care practices' performance reports on quality measures are based on PCP assignment, each clinic regularly updates their clinic panels and has specific protocols to address errors with PCP attribution.
Finally, our study includes only the variables that we were able to extract from administrative claims. Other explanatory variables that have been suggested as important for evaluation, such as social support, functional assessment, access to care, hospital discharge process, and posthospitalization follow‐up, were not included. Each of these could be explored in future studies.
This study offers a unique perspective of hospital readmission by introducing a new methodology for primary care clinics to calculate and evaluate their all‐cause 30‐day readmission rates. Although this methodology is not intended to provide real‐time feedback to clinics on readmitted patients, it opens the door for benchmarking based on specific case‐mix indices. Another direction for future research is to design robust evaluations of the impact of interventions, both inpatient and outpatient, on primary care clinic readmission rates. Finally, future research should replicate this analysis across teaching clinics to identify whether provider turnover is a consistent independent predictor of hospital readmissions.
This study also has implications for inpatient interventions. For example, discharging physicians may consider proactively identifying whether the patient has a continuous primary care provider. Patients who are in between PCPs may need closer follow‐up after discharge, until they re‐establish with a new PCP. This can be accomplished through a postdischarge clinic visit, either run by inpatient providers or covering physicians in the primary care clinic. In addition, the discharging physician may work with the case manager to increase the level of care coordination. The case manager could contact the primary care clinic and proactively ask for immediate PCP re‐assignment. Once a new PCP has been identified, the discharging physician could consider a warm hand‐off. With a warm hand‐off, the new PCP may feel more comfortable managing problems that may arise after hospital discharge, and especially before the first outpatient visit with the new PCP. Future research can test whether these interventions could effectively reduce hospital readmissions across a broad primary care population.
CONCLUSION
Primary care providers and their clinics play an important role in managing population health, decreasing healthcare spending, and keeping patients out of the hospital. In this study, we introduce a tool in which primary care clinics can begin to understand their hospital readmission rates. This may be particularly valuable as primary care providers enter global payment arrangements such as accountable care organizations or bundled payments and are responsible for a population of patients across the continuum of care. We found significant variation in readmission rates between different primary care practices, but much of this variation appears to be due to differences between practices in patient demographics, comorbidities, and hospitalization factors. Our study is the first to show the association between provider transitions and higher hospital readmissions. Continuity of care is critical for the optimal management of patients following hospital discharge. More attention will need to be focused on providing good continuity outpatient care for patients at high risk for readmissions.
Acknowledgements
The authors acknowledge Janelle Lee, MBA, MHA, DrPH, for her assistance with extracting the hospital claims data at UCSF Medical Center.
Disclosure: Dr. Tang and Ms. Maselli were supported by a University of California, Center for Health Quality and Innovation grant. The authors report no conflicts of interest.
- Medicare's new hospital value‐based purchasing program is likely to have only a small impact on hospital payments. Health Aff. 2012;31:1932–1940. , .
- Contemporary evidence about hospital strategies for reducing 30‐day readmissions. J Am Coll Cardiol. 2012;60:607–614. , , , et al.
- Interventions to reduce 30‐day rehospitalization: a systematic review. Ann Intern Med. 2011;155:520–528. , , , , .
- Reducing hospital readmissions: lessons from top‐performing hospitals. The Commonwealth Fund Synthesis Report. Available at: http://www.commonwealthfund.org/publications/case‐studies/2011/apr/reducing‐hospital‐readmissions. Published April 6, 2011. Accessed February 8, 2013. , , .
- Effective Interventions to Reduce Rehospitalizations: A Survey of the Published Evidence. Cambridge, MA: Institute for Healthcare Improvement; 2009. , .
- Recasting readmissions by placing the hospital role in community context. JAMA. 2013;309:351–352. , , .
- Hospitalists and care transitions: the divorce of inpatient and outpatient care. Health Affairs. 2008;27:1315–1327. , , , .
- Center for Medicare and Medicaid Innovation. Community‐based Care Transitions Program. Available at: http://innovation.cms.gov/initiatives/CCTP/#collapse‐tableDetails. Accessed February 8, 2013.
- Medicare's transitional care payment—a step toward the medical home. N Engl J Med. 2013;368:692–694. , , .
- Volume thresholds and hospital characteristics in the United States. Health Aff (Millwood). 2003;22:167–177. , , .
- Thirty‐day rehospitalizations after acute myocardial infarction: a cohort study. Ann Intern Med. 2012;157:11–18. , , , , , .
- Rehospitalizations among patients in the Medicare fee‐for‐service program. N Engl J Med. 2009;360:1418–1428. , , .
- Associations between reduced hospital length of stay and 30‐day readmission rate and mortality: 14‐year experience in 129 Veterans Affairs hospitals. Ann Intern Med. 2012;157:837–845. , , , et al.
- Readmission after hospitalization for congestive heart failure among Medicare beneficiaries. Arch Intern Med. 1997;157:99–104. , , , et al.
- Redefining readmission risk factors for general medicine patients. J Hosp Med. 2011;6:54–60. , , , .
- Risk prediction models for hospital readmission: a systematic review. JAMA. 2011;206:1688–1698. , , , et al.
- Statistical models and patient predictors of readmission for heart failure: a systematic review. Arch Intern Med. 2008;168:1371–1386. , , , et al.
- Statistical models and patient predictors of readmission for acute myocardial infarction: a systematic review. Circ Cardiovasc Qual Outcomes. 2009;2:500–507. , , , .
- Predictors of hospital readmission after stroke: a systematic review. Stroke. 2010;41:2525–2533. , , , et al.
- Risk factors for 30‐day hospital readmission in patients ≥65 years of age. Proc (Bayl Univ Med Cent). 2008;21:363–372. , , , , .
- Hospital readmission in general medicine patients: a prediction model. J Gen Intern Med. 2010;25:211–219. , , , et al.
- Users of Medicaid home and community‐based services are especially vulnerable to costly avoidable hospital admissions. Health Affairs. 2012;31:1167–1175. , , .
- Academic year‐end transfers of outpatients from outgoing to incoming residents: an unaddressed patient safety issue. JAMA. 2009;302:1327–1329. , .
- The revolving door of resident continuity practice: identifying gaps in transitions of care. J Gen Intern Med. 2011;26:995–998. , , , , , .
- Outcomes for resident‐identified high‐risk patients and resident perspectives of year‐end continuity clinic handoffs. J Gen Intern Med. 2012;27:1438–1444. , , , et al.
- Medicare's new hospital value‐based purchasing program is likely to have only a small impact on hospital payments. Health Aff. 2012;31:1932–1940. , .
- Contemporary evidence about hospital strategies for reducing 30‐day readmissions. J Am Coll Cardiol. 2012;60:607–614. , , , et al.
- Interventions to reduce 30‐day rehospitalization: a systematic review. Ann Intern Med. 2011;155:520–528. , , , , .
- Reducing hospital readmissions: lessons from top‐performing hospitals. The Commonwealth Fund Synthesis Report. Available at: http://www.commonwealthfund.org/publications/case‐studies/2011/apr/reducing‐hospital‐readmissions. Published April 6, 2011. Accessed February 8, 2013. , , .
- Effective Interventions to Reduce Rehospitalizations: A Survey of the Published Evidence. Cambridge, MA: Institute for Healthcare Improvement; 2009. , .
- Recasting readmissions by placing the hospital role in community context. JAMA. 2013;309:351–352. , , .
- Hospitalists and care transitions: the divorce of inpatient and outpatient care. Health Affairs. 2008;27:1315–1327. , , , .
- Center for Medicare and Medicaid Innovation. Community‐based Care Transitions Program. Available at: http://innovation.cms.gov/initiatives/CCTP/#collapse‐tableDetails. Accessed February 8, 2013.
- Medicare's transitional care payment—a step toward the medical home. N Engl J Med. 2013;368:692–694. , , .
- Volume thresholds and hospital characteristics in the United States. Health Aff (Millwood). 2003;22:167–177. , , .
- Thirty‐day rehospitalizations after acute myocardial infarction: a cohort study. Ann Intern Med. 2012;157:11–18. , , , , , .
- Rehospitalizations among patients in the Medicare fee‐for‐service program. N Engl J Med. 2009;360:1418–1428. , , .
- Associations between reduced hospital length of stay and 30‐day readmission rate and mortality: 14‐year experience in 129 Veterans Affairs hospitals. Ann Intern Med. 2012;157:837–845. , , , et al.
- Readmission after hospitalization for congestive heart failure among Medicare beneficiaries. Arch Intern Med. 1997;157:99–104. , , , et al.
- Redefining readmission risk factors for general medicine patients. J Hosp Med. 2011;6:54–60. , , , .
- Risk prediction models for hospital readmission: a systematic review. JAMA. 2011;206:1688–1698. , , , et al.
- Statistical models and patient predictors of readmission for heart failure: a systematic review. Arch Intern Med. 2008;168:1371–1386. , , , et al.
- Statistical models and patient predictors of readmission for acute myocardial infarction: a systematic review. Circ Cardiovasc Qual Outcomes. 2009;2:500–507. , , , .
- Predictors of hospital readmission after stroke: a systematic review. Stroke. 2010;41:2525–2533. , , , et al.
- Risk factors for 30‐day hospital readmission in patients ≥65 years of age. Proc (Bayl Univ Med Cent). 2008;21:363–372. , , , , .
- Hospital readmission in general medicine patients: a prediction model. J Gen Intern Med. 2010;25:211–219. , , , et al.
- Users of Medicaid home and community‐based services are especially vulnerable to costly avoidable hospital admissions. Health Affairs. 2012;31:1167–1175. , , .
- Academic year‐end transfers of outpatients from outgoing to incoming residents: an unaddressed patient safety issue. JAMA. 2009;302:1327–1329. , .
- The revolving door of resident continuity practice: identifying gaps in transitions of care. J Gen Intern Med. 2011;26:995–998. , , , , , .
- Outcomes for resident‐identified high‐risk patients and resident perspectives of year‐end continuity clinic handoffs. J Gen Intern Med. 2012;27:1438–1444. , , , et al.
© 2014 Society of Hospital Medicine
High Users of Hospital Care
Despite signs of a slowing trend,[1, 2, 3] US healthcare costs continue to rise, and cost containment remains a major area of concern. Hospital costs are the largest single category of national healthcare expenditures,[4] and the burden of cost containment is increasingly being shifted to hospitals.[5] As such, hospitals are increasingly focusing on implementing interventions to reduce rates of hospitalizations and readmissions as a mechanism to reduce overall healthcare costs.[5, 6, 7, 8, 9]
Multiple factors potentially contribute to patients being high cost, including acute care utilization,[10, 11, 12] pharmaceuticals,[13, 14] procedures,[15] catastrophic illness,[16] and high‐risk chronic conditions.[11, 17, 18] However, many hospitals are implementing interventions focused on a single subset of these high cost patientshigh users of inpatient services. Care management interventions have received particular attention, due to their perceived potential to improve quality of care while reducing costs through the mechanism of reducing hospital admissions.[19, 20, 21] Despite their increasing prevalence, there is limited evidence demonstrating the effectiveness of these programs. Among interventions targeting high cost individuals, the Medicare Care Management for High Cost Beneficiaries showed no effect on hospital admissions.[19] Another high‐profile intervention, the Citywide Care Management System led by the Camden Coalition, showed promising preliminary results, but data from a systematic evaluation are lacking.[22] Conversely, interventions targeting individuals with frequent hospitalizations have similarly shown mixed results in reducing costs.[6, 7, 9, 23]
Taken together, these data suggest that the relationship between high costs and frequent hospital use is complicated, and the population of individuals with frequent hospitalizations may not represent the entire population of high cost individuals. Thus, focusing on reduction of hospitalizations alone may be inadequate to reduce costs. For these reasons, we sought to determine how many high cost individuals would be captured by focusing only on frequently hospitalized (high admit) individuals by examining the overlap between these populations. We also sought to describe the characteristics and distinctions between the resulting subgroups of high users to better inform the design of future interventions.
METHODS
We examined the cross‐sectional relationship between high cost and high admit populations among adult patients 18 years of age hospitalized at the University of California, San Francisco (UCSF) Medical Center, a 660‐bed urban academic medical center from July 1, 2010 to June 30, 2011.
This study was conducted as part of a quality improvement project to identify high user primary care patients for complex care management. Individuals were included in the study if: (1) they had an assigned UCSF primary care provider (PCP), and (2) they had at least 1 hospitalization at UCSF during the study period. PCP assignments were ascertained from panel lists maintained by clinics; lists included individuals with at least 1 visit at any of the 8 primary care clinics at UCSF in the 2 years prior to the end of the study period. Because individuals are dropped from PCP panels at death, we were unable to ascertain or include individuals who died during the study period.
From the initial study population, we defined the high cost group as those who were in the top decile of total hospital costs, and the high admit group as those who were in the top decile of total hospitalizations during the study period. We elected to use the top decile as a cutoff given that it is a common operational definition used to identify high users to target for intervention.[24]
To examine the relationship between high cost and high admits we defined 3 further subgroups: high costlow admits, high costhigh admits, and low costhigh admits (Figure 1). To explore the face validity of these descriptors and classification scheme, we subsequently examined the proportion of total hospital costs and total hospitalizations each subgroup accounted for with respect to the study population.
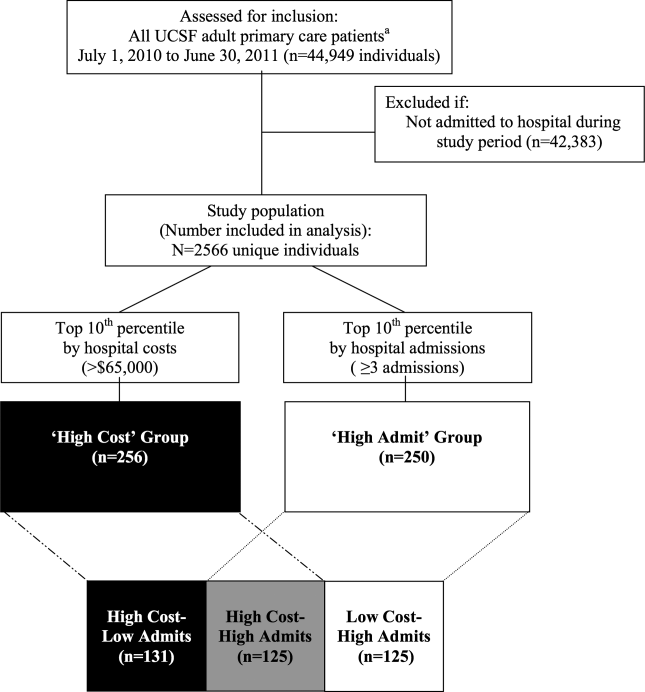
Data Sources
Hospital costs, demographic data, and encounter diagnoses were obtained from the hospital's Transition Systems Incorporated system (TSI, also known as Eclipsys or Allscripts), a commercially available automated cost accounting system that integrates multiple data sources to calculate total hospital costs on a per patient basis. Several studies have previously used the TSI system to estimate the costs of healthcare services at individual hospitals, and this approach is generally considered the most accurate method to estimate cost.[25, 26] Hospital costs included the sum of actual total costs (not billed charges) for all hospital episodes including lab costs, drug costs, surgical supplies, nurse salaries and benefits, utilities, housekeeping, and allocated administrative overhead. This cost total does not capture the cost of physician labor (pro‐fees), preadmission costs (e.g., outpatient care), or postadmission costs (e.g., home health, nursing home, or other postdischarge care). Preadmission lab, diagnostic tests, and imaging were included in hospital costs if these were ordered within 72 hours of hospital admission. Emergency department (ED) costs were included if an individual was admitted to the hospital via the ED. Hospitalizations were defined as inpatient admissions only to UCSF because we were unable to reliably ascertain hospitalizations outside of UCSF. PCP assignments were ascertained from administrative panel lists maintained by clinics.
Study Variables
We analyzed factors previously shown[13, 27, 28, 29] to be associated with high healthcare cost and utilization. We examined demographic characteristics and hospitalization characteristics, including admission source, length of stay (LOS), cost per hospitalization, whether the episode was a 30‐day readmission, days in the intensive care unit (ICU), and encounter diagnoses.
To ascertain whether a hospitalization was for a medical versus surgical condition, we used discharge diagnosis codes and designations as per the Medicare Severity Diagnosis‐Related Groups (MS‐DRG) versions 27 and 28 definitions manuals. We subsequently grouped medical and surgical conditions by Major Diagnostic Categories as per the MS‐DRG definitions manuals.
Using MS‐DRG codes, we also classified whether hospitalizations were for pneumonia, acute myocardial infarction (AMI), and congestive heart failure (CHF), as these 3 conditions have specific payment penalties under the Centers for Medicare & Medicaid Services (CMS) reimbursement policies. For these CMS core conditions, we included hospitalizations with MS‐DRG codes 193195, 280282, and 291293 (codes 283285 were not included for AMI because individuals who died during the study period were excluded.)
Analysis
We used descriptive statistics to compare patient and hospitalization characteristics between subgroups. Non‐normally distributed variables including LOS and cost per hospitalization were log transformed. Because a single individual could account for multiple hospitalizations, we performed a companion analysis of hospitalization characteristics using generalized estimating equations with an independent correlation structure to account for clustering of hospitalizations within individuals. Our findings were robust using either approach. For ease of interpretation, P values from the former analysis are presented.
To determine whether the overall distribution and characteristics we observed for high user subgroups were a single‐year anomaly or representative of temporally stable trends, we compared non‐high users and high user subgroup characteristics over the 3 years preceding the study period using linear regression for trend.
The institutional review board at UCSF approved this study protocol.
RESULTS
Of the 2566 unique individuals included in the analysis (Figure 1), 256 individuals were identified as high cost (top decile, $65,000). This group accounted for 45% of all costs and 22% of all hospitalizations (Figure 2). Two hundred fifty individuals were identified as high admits (top decile, 3 hospitalizations). This group accounted for 32% of all costs and 28% of all hospitalizations.
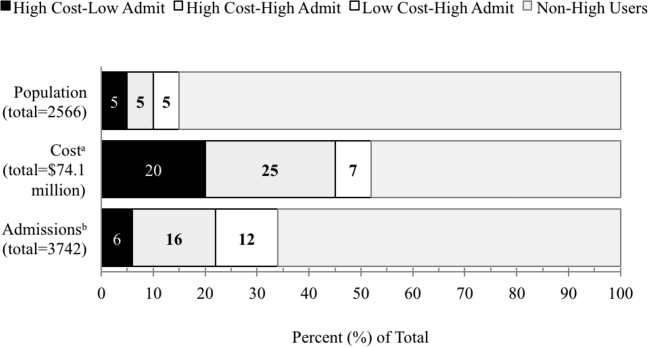
Only 48% of high cost individuals were also high admit ($65,000 and 3 hospitalizations; n=125, Figure 1). Among high users, we subsequently defined 3 subgroups based on the relationship between cost and hospitalizations (high costlow admits, high costhigh admits, and low costhigh admits). Each subgroup accounted for approximately 5% of the overall study population (Figure 2). The high costlow admits subgroup incurred a proportionate share of hospitalizations (6%) but a disproportionate share of costs (20%). The high costhigh admits subgroup had a disproportionate share of both costs (25%) and hospitalizations (16%). The low costhigh admits subgroup had a proportionate share of costs (7%) but a disproportionate share of hospitalizations (12%).
Patient and Hospitalization Characteristics
Compared to non‐high users, all high user subgroups were more likely to have public insurance (Medicare or Medicaid) or have dualeligible status, and the two high cost subgroups were more likely to be male and African American (P<0.05 for all). Compared to each other, subgroups were similar with respect to race/ethnicity, payer, and age (Table 1).
Non‐High Users,n=2145 | High User Subgroups | ||||||
---|---|---|---|---|---|---|---|
1 | 2 | 3 | P Value for Comparison | ||||
High CostLow Admit, n=131 | High CostHigh Admit, n=125 | Low CostHigh Admit, n=125 | 1 vs 2 | 2 vs 3 | 1 vs 3 | ||
| |||||||
Male, % | 34 | 57 | 42 | 38 | 0.02 | 0.52 | 0.003 |
Race/ethnicity, % | 0.15 | 0.76 | 0.18 | ||||
White | 45 | 46 | 36 | 38 | |||
Black | 14 | 21 | 26 | 19 | |||
Hispanic | 9 | 5 | 10 | 10 | |||
Asian | 22 | 18 | 22 | 26 | |||
Other | 10 | 10 | 6 | 6 | |||
Primary payer, % | 0.23 | 0.44 | 0.51 | ||||
Commercial | 42 | 24 | 20 | 17 | |||
Medicare | 42 | 58 | 55 | 61 | |||
Medicaid | 15 | 17 | 25 | 21 | |||
Other | <1 | 2 | 2 | ||||
Dual eligible, %* | 18 | 27 | 26 | 31 | 0.85 | 0.38 | 0.48 |
Age, mean yearsSD | 5720 | 6017 | 5817 | 6320 | 0.40 | 0.04 | 0.19 |
No. of hospitalizations per individual, median (interquartile range) | 1 (11) | 2 (12) | 4 (36) | 3 (34) | <0.001 | <0.001 | <0.001 |
Hospital costs per individual, median $1000 (interquartile range) | 12 (722) | 93 (75122) | 113 (85174) | 37 (3051) | <0.001 | <0.001 | <0.001 |
Regarding hospitalization characteristics, each high user subgroup was distinct and significantly different from each of the other subgroups with respect to admission source, proportion of 30‐day readmissions, LOS, and cost per hospitalization (Table 2, P<0.001 for all). The low costhigh admit subgroup had the highest proportion of admissions from the ED (73%), a moderate proportion of 30‐day readmissions (32%), the shortest LOS (median, 3 days; interquartile range [IQR], 24 days) and the lowest cost per hospitalization (median, $12,000; IQR, $8,000$15,000). In contrast, the high costlow admit subgroup had the highest proportion of admissions from clinic or physician referrals (45%), lowest proportion of 30‐day readmissions (17%), the longest LOS (median, 10; IQR, 417), the most ICU days per hospitalization (median, 1; range, 049) and the highest cost per hospitalization (median, $68,000; IQR, $43,000$95,000). High costhigh admit individuals had the highest proportion of 30‐day readmissions (47%) and a moderate cost per hospitalization (median, $28,000; IQR, $23,000$38,000), but the highest median cost per individual over 1 year ($113,000; IQR, $85,000$174,000, Table 1). Hospitalizations classified as 30‐day readmissions accounted for 42% of costs incurred by this subgroup; 30‐day readmissions specifically associated with CMS core conditions accounted for <1% of costs.
Non‐High Users | High User Subgroups | ||||||
---|---|---|---|---|---|---|---|
1 | 2 | 3 | P Value for Comparison | ||||
High CostLow Admit | High CostHigh Admit | Low CostHigh Admit | 1 vs 2 | 2 vs 3 | 1 vs 3 | ||
| |||||||
No. of admissions | 2500 | 206 | 605 | 431 | |||
Admission source, % | <0.001 | <0.001 | <0.001 | ||||
Emergency department | 53 | 50 | 65 | 73 | |||
Clinic or physician referral | 44 | 45 | 30 | 20 | |||
Transfer from outside facility | 2 | 5 | 4 | 4 | |||
Self‐referral | 1 | <1 | 1 | 3 | |||
Other | <1 | ||||||
30‐day readmission, % | 5 | 17 | 47 | 32 | <0.001 | <0.001 | <0.001 |
LOS, median days (IQR) | 3 (24) | 10 (417) | 5 (310) | 3 (24) | <0.001 | <0.001 | <0.001 |
ICU days, median (range)* | 0 (08) | 1 (049) | 0 (021) | 0 (03) | <0.001 | <0.001 | <0.001 |
Cost per hospitalization, median $1,000 (IQR) | 11 (719) | 68 (4395) | 28 (2338) | 12 (815) | <0.001 | <0.001 | <0.001 |
Encounter diagnoses | <0.001 | 0.002 | <0.001 | ||||
Surgical MS‐DRGs, % | 30 | 58 | 22 | 13 | |||
Most common MDCs | |||||||
Cardiovascular | 4 | 15 | 8 | 6 | |||
Orthopedic | 10 | 13 | 6 | 4 | |||
Transplant | <1 | 7 | 1 | 1 | |||
Medical MS‐DRGs, % | 70 | 42 | 78 | 87 | |||
Most common MDCs | |||||||
Pregnancy related | 17 | 2 | 2 | 2 | |||
Cardiovascular | 10 | 10 | 7 | 13 | |||
Respiratory | 9 | 4 | 14 | 17 | |||
Gastrointestinal | 7 | 3 | 10 | 14 | |||
Hematologic | 1 | 2 | 9 | 6 | |||
Myeloproliferative | <1 | 4 | 9 | 6 | |||
CMS core condition | 7 | 3 | 6 | 12 | 0.174 | 0.01 | 0.004 |
Encounter diagnoses associated with hospitalizations were also significantly different between each of the high user subgroups (Table 2, P<0.001 for all). The high costlow admit subgroup was predominantly hospitalized for surgical conditions (58% vs 42% for medical MS‐DRGs) and had the lowest proportion of hospitalizations for CMS core conditions (3%). The most common types of surgical hospitalizations in this subgroup were for cardiovascular procedures (15%; including coronary artery bypass grafting and cardiac valve replacement) and orthopedic procedures (13%; including hip, knee, and other joint replacements). Most surgical hospitalizations were from referrals (67%) rather than admissions through the ED. In contrast, the low costhigh admit group was predominantly hospitalized for medical conditions (87% vs 13% for surgical MS‐DRGs) and had the highest proportion of hospitalizations for CMS core conditions (12%). The most common types of medical hospitalizations in this subgroup were for respiratory conditions (17%; including chronic obstructive pulmonary disease and pneumonia), gastrointestinal conditions (14%), and cardiovascular conditions (13%; including CHF, AMI, arrhythmia, and chest pain). High costhigh admit individuals were also hospitalized primarily for medical rather than surgical conditions (78% vs 22% medical vs surgical MS‐DRGs). Only 6% of hospitalizations in this subgroup were for CMS core conditions, and only 2% of hospitalizations were 30‐day readmissions for CMS core conditions.
The overlap between the high cost and high admit groups was persistently 48% or less for the 3 years prior to the study period (Table 3). Although the extent of overlap was similar across years, the absolute dollar value for the cutoff to define the top decile by hospital costs gradually increased over time from $47,000 in 2008 to $65,000 in 2011 (P<0.001 for trend). Among the high costlow admit subgroup, there was a trend toward a decrease in the proportion of surgical hospitalizations from 67% in 2008 to 58% in 2011 (P=0.09).
2008 | 2009 | 2010 | 2011 | P Value (For Linear Trend) | |
---|---|---|---|---|---|
| |||||
Study population | 2408 | 2518 | 2647 | 2566 | |
Characteristics, n | |||||
Cutoff for high cost (top decile), nearest $1000 | >47 | >51 | >54 | >65 | <0.001 |
Proportion of total hospital costs incurred by high cost group, % | 46 | 47 | 47 | 48 | |
Cutoff for high admit (top decile), no. of admissions | 3 | 3 | 3 | 3 | |
High cost who are also high admit, % | 42 | 48 | 48 | 48 | |
Discharge diagnoses by subgroup* | |||||
Non‐high user population | |||||
Surgical MS‐DRG | 32 (751) | 33 (842) | 36 (932) | 30 (751) | 0.51 |
Medical MS‐DRG | 68 (1598) | 67 (1676) | 64 (1673) | 70 (1749) | |
High costlow admit | |||||
Surgical MS‐DRG | 67 (138) | 68 (132) | 61 (120) | 58 (119) | 0.09 |
Medical MS‐DRG | 33(67) | 32 (63) | 39 (78) | 42 (87) | |
High costhigh admit | |||||
Surgical MS‐DRG | 23 (104) | 25 (133) | 24 (150) | 22 (134) | 0.60 |
Medical MS‐DRG | 77 (341) | 75 (392) | 76 (464) | 78 (471) | |
Low costhigh admit | |||||
Surgical MS‐DRG | 11 (35) | 17 (44) | 13 (40) | 13 (54) | 0.90 |
Medical MS‐DRG | 89 (277) | 83 (219) | 87 (269) | 87 (377) |
DISCUSSION
In this study, we found that only half of high cost individuals were also high admit. Further categorizing high users into high costlow admit versus high costhigh admit versus low costhigh admit identified distinct patterns between each group. High costhigh admit individuals were more likely to be hospitalized for medical conditions, whereas high costlow admit individuals were more likely to be hospitalized for surgical conditions. CMS core conditions accounted for a low proportion of overall hospitalizations across all groups.
Our findings suggest several distinct types of high users with different clinical characteristics, utilization, and cost patterns. From a hospital perspective, one implication is that a multifaceted approach to cost containment, rather than the one‐size‐fits‐all strategy of reducing hospitalizations, may be more effective in reducing costs. For example, our findings show that high costlow admit individuals have a disproportionate number of hospitalizations for surgical conditions, longer LOS, and more ICU days. Costs incurred by this subgroup may be more responsive to in‐hospital interventions aimed at reducing procedural costs, LOS, unnecessary use of the ICU, and minimizing postoperative infections and complications rather than to a care management approach.
In contrast, care management strategies such as improving postdischarge care and chronic disease management, which aim to achieve cost savings through reducing hospitalizations, may be more effective in reducing costs among high costhigh admit individuals, who have a high proportion of hospitalizations for medical conditions and the highest proportion of 30‐day readmissions. Such strategies may also be important in optimizing the quality of care for low costhigh admit individuals, who have the highest proportion of medical hospitalizations among all high users, though the potential for cost savings may be more limited in this subgroup.
Our results suggest that current hospital‐based approachesdriven by readmissions penalties for CMS core conditionsmay have less than the expected impact on costs. For example, although high costhigh admit individuals had the highest proportion of 30‐day readmissions, readmissions specifically for CMS core conditions accounted for <1% of costs in this subgroup. Thus, the potential return on an expensive investment in a care management intervention is unclear, given the small number of readmissions for these select conditions. From a broader perspective, the focus on readmissions for CMS core conditions, which overall contribute relatively little to high hospital costs, may not be a comprehensive enough strategy for cost containment. To date, there have been limited policies targeting factors contributing to high hospital costs outside of frequent medical hospitalizations. Medicare's nonpayment policy for treatment of preventable hospital conditions, including surgical site infections, translates prevention of these conditions into cost savings for hospitals.[30] However, this rule has been criticized for not going far enough to drive substantial savings.[31] A new CMS rule authorizes states to identify other provider‐preventable conditions for which Medicaid payment will be prohibited.[32] Future policy efforts should further emphasize a comprehensive, multipronged approach beyond readmissions penalties for select conditions if healthcare cost containment remains a policy priority.
Our results should be interpreted in light of several limitations. First, this was a single‐site study at an academic medical center; the generalizability of our results to other settings is unclear. Our cost data likely reflect local market factors, including the highest wage rates for skilled healthcare labor in the United States.[33] Although the explicit distribution of high user subgroups may be institution‐specific due to variations in our cost structure, we anticipate that the general classification paradigm will be similar in other health systems. Second, we captured utilization and costs only at a single hospital. However, our study population includes only individuals with PCPs at UCSF, and internal data from both Medicare and UCSF's largest private payer show that over 85% of hospitalizations among this population are to UCSF Medical Center. Third, we were able to capture only hospital costs rather than overall healthcare costs. Given that hospital costs account for the single largest category of total national health costs,[4] we expect that future studies examining total health costs will show similar findings. Fourth, our data did not include measures of health status, socioeconomic status, housing, or mental health comorbidities to permit an analysis of these factors, which have been previously related to frequent hospitalizations and high costs.[34, 35, 36, 37, 38, 39] Fifth, due to resource constraints, we were unable to conduct a longitudinal analysis to examine the extent to which individuals are consistently high users over time. Previous studies have described that 20% to 30% of individuals are consistently high users; the remainder have discrete periods of high utilization.[34, 40] This may be an important consideration in the design of future interventions.
Finally, our analysis was limited to individuals with a PCP to allow identification of an accessible cohort for care management. Thus, we did not capture individuals without a PCP and individuals who died during the study period, because these individuals no longer had an assigned PCP following death. Although this approach is consistent with that of many care management programs,[19] these populations are likely to incur higher than average utilization and healthcare costs, and represent important areas for future investigation.
In summary, our study identifies three types of high‐user populations that differ in the proportion of costs attributable to frequent hospitalizations, clinical conditions associated with hospital use, and frequency of 30‐day readmissions. Stratifying high users by both costs and hospitalizations may help identify tailored strategies to more effectively reduce costs and utilization.
Acknowledgments
The authors acknowledge Diana Patterson, Leanna Zaporozhets, and Andre Devito for their assistance in data collection.
Disclosures: Dr. Nguyen had full access to all of the data in the study and takes responsibility for the integrity of the data and the accuracy of the data analysis. Dr. Nguyen's work on this project was completed as a primary care research fellow at the University of California, San Francisco funded by a federal training grant from the National Research Service Award (NRSA T32HP19025‐07‐00).
- National Health Expenditure Accounts Team. Recession contributes to slowest annual rate of increase in health spending in five decades. Health Aff (Millwood). 2011;30(1):11–22. , , , ;
- Growth in US health spending remained slow in 2010; health share of gross domestic product was unchanged from 2009. Health Aff (Millwood). 2012;31(1):208–219. , , , .
- the National Health Expenditure Accounts Team. National health spending in 2011: overall growth remains low, but some payers and services show signs of acceleration. Health Aff (Millwood). 2013;32(1):87–99. , , , ;
- Centers for Medicare and Medicaid Services; Office of the Actuary; National Health Statistics Group. National healthcare expenditures data. 2012. Available at: https://www.cms.gov/Research‐Statistics‐Data‐and‐Systems/Statistics‐Trends‐and‐Reports/NationalHealthExpendData/downloads/tables.pdf.
- Medicare Payment Advisory Commission. Report to the Congress: Promoting Greater Efficiency in Medicare. Washington, DC: Medicare Payment Advisory Commission; 2007.
- The care transitions intervention: results of a randomized controlled trial. Arch Intern Med. 2006;166(17):1822–1828. , , , .
- A reengineered hospital discharge program to decrease rehospitalization: a randomized trial. Ann Intern Med. 2009;150(3):178–187. , , , et al.
- How‐to Guide: Improving Transitions from the Hospital to the Clinical Office Practice to Reduce Avoidable Rehospitalizations. Cambridge, MA: Institute for Healthcare Improvement; 2012. , , , .
- Comprehensive discharge planning and home follow‐up of hospitalized elders: a randomized clinical trial. JAMA. 1999;281(7):613–620. , , , et al.
- High‐cost users of medical care. N Engl J Med. 1980;302(18):996–1002. , .
- Frequency and clinical description of high‐cost patients in 17 acute‐care hospitals. N Engl J Med. 1979;300(23):1306–1309. , , .
- Effectiveness of interventions targeting frequent users of emergency departments: a systematic review. Ann Emerg Med. 2011;58(1):41–52.e42. , , , et al.
- Medication, diagnostic, and cost information as predictors of high‐risk patients in need of care management. Am J Manag Care. 2009;15(1):41–48. , , , .
- Tracking health care costs: trends stabilize but remain high in 2002. Health Aff (Millwood). 2003;Suppl Web Exclusives:W3–266–274. , .
- An iconoclastic view of health cost containment. Health Aff (Millwood). 1993;12(suppl):152–171. .
- Comparisons of medical utilizations and categorical diagnoses of emergency visits between the elderly with catastrophic illness certificates and those without. BMC Health Serv Res. 2013;13:152. , , , et al.
- Finding future high‐cost cases: comparing prior cost versus diagnosis‐based methods. Health Serv Res. 2001;36(6 pt 2):194–206. , , , .
- Spending and service use among people with the fifteen most costly medical conditions, 1997. Health Aff (Millwood). 2003;22(2):129–138. , .
- Lessons from Medicare's Demonstration Projects on Disease Management and Care Coordination. Washington, D.C.: Congressional Budget Office; 2012. .
- The hot spotters. The New Yorker. January 24, 2011. .
- Collaboration Across the Disciplines in Health Care. Burlington, MA: Jones and Bartlett; 2010. , , .
- Hope for New Jersey's city hospitals: the Camden Initiative. Perspect Health Inf Manag. 2010:7:1d. , , .
- Hospital‐initiated transitional care interventions as a patient safety strategy: a systematic review. Ann Intern Med. 2013;158(5 pt 2):433–440. , , , , , .
- Agency for Healthcare Research and Quality. The concentration and persistence in the level of health expenditures over time: estimates for the U.S. population, 2008–2009. 2012. Available at: http://meps.ahrq.gov/mepsweb/data_files/publications/st354/stat354.shtml.
- The use of the transition cost accounting system in health services research. Cost Eff Resour Alloc. 2007;5:11. , , , , , .
- Cost reduction and quality improvement: it takes two to tango. Crit Care Med. 2000;28(2):581–583. , .
- Case finding for patients at risk of readmission to hospital: development of algorithm to identify high risk patients. BMJ. 2006;333(7563):327. , , , .
- Risk prediction models for hospital readmission: a systematic review. JAMA. 2011;306(15):1688–1698. , , , et al.
- Chronic disease score as a predictor of hospitalization. Epidemiology. 2002;13(3):340–346. , , , et al.
- Centers for Medicare and Medicaid Services. Hospital‐acquired conditions (HAC) in acute inpatient prospective payment system (IPPS) hospitals fact sheet. 2011. Available at: https://www.cms.gov/Medicare/Medicare‐Fee‐for‐Service‐Payment/HospitalAcqCond/downloads/HACFactsheet.pdf. Accessed August 31, 2013.
- Medicare's policy not to pay for treating hospital‐acquired conditions: the impact. Health Aff (Millwood). 2009;28(5):1485–1493. , , .
- Department of Health and Human Services, Centers for Medicare 2013.
- Consistently high users of medical care among the elderly. Med Care. 1990;28(6):527–540. , , , .
- Utilization patterns among long‐term enrollees in a prepaid group practice health maintenance organization. Med Care. 1985;23(11):1221–1233. , , , .
- Depressive symptoms and health service utilization among the community elderly. J Am Geriatr Soc. 1983;31(7):417–420. , , .
- The influence of socio‐economic status and multimorbidity patterns on healthcare costs: a six‐year follow‐up under a universal healthcare system. Int J Equity Health. 2013;12(1):69. , .
- Association between the level of housing instability, economic standing and health care access: a meta‐regression. J Health Care Poor Underserved. 2008;19(4):1212–1228. , , .
- High health care utilization and costs associated with lower socio‐economic status: results from a linked dataset. Can J Public Health. 2009;100(3):180–183. , , , .
- An analysis of the use of Medicare services by the continuously enrolled aged. Med Care. 1983;21(6):567–585. , .
Despite signs of a slowing trend,[1, 2, 3] US healthcare costs continue to rise, and cost containment remains a major area of concern. Hospital costs are the largest single category of national healthcare expenditures,[4] and the burden of cost containment is increasingly being shifted to hospitals.[5] As such, hospitals are increasingly focusing on implementing interventions to reduce rates of hospitalizations and readmissions as a mechanism to reduce overall healthcare costs.[5, 6, 7, 8, 9]
Multiple factors potentially contribute to patients being high cost, including acute care utilization,[10, 11, 12] pharmaceuticals,[13, 14] procedures,[15] catastrophic illness,[16] and high‐risk chronic conditions.[11, 17, 18] However, many hospitals are implementing interventions focused on a single subset of these high cost patientshigh users of inpatient services. Care management interventions have received particular attention, due to their perceived potential to improve quality of care while reducing costs through the mechanism of reducing hospital admissions.[19, 20, 21] Despite their increasing prevalence, there is limited evidence demonstrating the effectiveness of these programs. Among interventions targeting high cost individuals, the Medicare Care Management for High Cost Beneficiaries showed no effect on hospital admissions.[19] Another high‐profile intervention, the Citywide Care Management System led by the Camden Coalition, showed promising preliminary results, but data from a systematic evaluation are lacking.[22] Conversely, interventions targeting individuals with frequent hospitalizations have similarly shown mixed results in reducing costs.[6, 7, 9, 23]
Taken together, these data suggest that the relationship between high costs and frequent hospital use is complicated, and the population of individuals with frequent hospitalizations may not represent the entire population of high cost individuals. Thus, focusing on reduction of hospitalizations alone may be inadequate to reduce costs. For these reasons, we sought to determine how many high cost individuals would be captured by focusing only on frequently hospitalized (high admit) individuals by examining the overlap between these populations. We also sought to describe the characteristics and distinctions between the resulting subgroups of high users to better inform the design of future interventions.
METHODS
We examined the cross‐sectional relationship between high cost and high admit populations among adult patients 18 years of age hospitalized at the University of California, San Francisco (UCSF) Medical Center, a 660‐bed urban academic medical center from July 1, 2010 to June 30, 2011.
This study was conducted as part of a quality improvement project to identify high user primary care patients for complex care management. Individuals were included in the study if: (1) they had an assigned UCSF primary care provider (PCP), and (2) they had at least 1 hospitalization at UCSF during the study period. PCP assignments were ascertained from panel lists maintained by clinics; lists included individuals with at least 1 visit at any of the 8 primary care clinics at UCSF in the 2 years prior to the end of the study period. Because individuals are dropped from PCP panels at death, we were unable to ascertain or include individuals who died during the study period.
From the initial study population, we defined the high cost group as those who were in the top decile of total hospital costs, and the high admit group as those who were in the top decile of total hospitalizations during the study period. We elected to use the top decile as a cutoff given that it is a common operational definition used to identify high users to target for intervention.[24]
To examine the relationship between high cost and high admits we defined 3 further subgroups: high costlow admits, high costhigh admits, and low costhigh admits (Figure 1). To explore the face validity of these descriptors and classification scheme, we subsequently examined the proportion of total hospital costs and total hospitalizations each subgroup accounted for with respect to the study population.
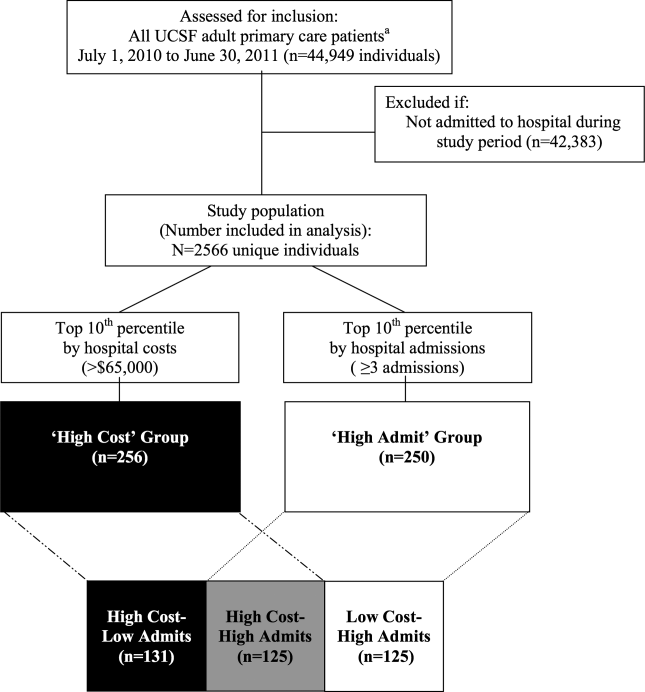
Data Sources
Hospital costs, demographic data, and encounter diagnoses were obtained from the hospital's Transition Systems Incorporated system (TSI, also known as Eclipsys or Allscripts), a commercially available automated cost accounting system that integrates multiple data sources to calculate total hospital costs on a per patient basis. Several studies have previously used the TSI system to estimate the costs of healthcare services at individual hospitals, and this approach is generally considered the most accurate method to estimate cost.[25, 26] Hospital costs included the sum of actual total costs (not billed charges) for all hospital episodes including lab costs, drug costs, surgical supplies, nurse salaries and benefits, utilities, housekeeping, and allocated administrative overhead. This cost total does not capture the cost of physician labor (pro‐fees), preadmission costs (e.g., outpatient care), or postadmission costs (e.g., home health, nursing home, or other postdischarge care). Preadmission lab, diagnostic tests, and imaging were included in hospital costs if these were ordered within 72 hours of hospital admission. Emergency department (ED) costs were included if an individual was admitted to the hospital via the ED. Hospitalizations were defined as inpatient admissions only to UCSF because we were unable to reliably ascertain hospitalizations outside of UCSF. PCP assignments were ascertained from administrative panel lists maintained by clinics.
Study Variables
We analyzed factors previously shown[13, 27, 28, 29] to be associated with high healthcare cost and utilization. We examined demographic characteristics and hospitalization characteristics, including admission source, length of stay (LOS), cost per hospitalization, whether the episode was a 30‐day readmission, days in the intensive care unit (ICU), and encounter diagnoses.
To ascertain whether a hospitalization was for a medical versus surgical condition, we used discharge diagnosis codes and designations as per the Medicare Severity Diagnosis‐Related Groups (MS‐DRG) versions 27 and 28 definitions manuals. We subsequently grouped medical and surgical conditions by Major Diagnostic Categories as per the MS‐DRG definitions manuals.
Using MS‐DRG codes, we also classified whether hospitalizations were for pneumonia, acute myocardial infarction (AMI), and congestive heart failure (CHF), as these 3 conditions have specific payment penalties under the Centers for Medicare & Medicaid Services (CMS) reimbursement policies. For these CMS core conditions, we included hospitalizations with MS‐DRG codes 193195, 280282, and 291293 (codes 283285 were not included for AMI because individuals who died during the study period were excluded.)
Analysis
We used descriptive statistics to compare patient and hospitalization characteristics between subgroups. Non‐normally distributed variables including LOS and cost per hospitalization were log transformed. Because a single individual could account for multiple hospitalizations, we performed a companion analysis of hospitalization characteristics using generalized estimating equations with an independent correlation structure to account for clustering of hospitalizations within individuals. Our findings were robust using either approach. For ease of interpretation, P values from the former analysis are presented.
To determine whether the overall distribution and characteristics we observed for high user subgroups were a single‐year anomaly or representative of temporally stable trends, we compared non‐high users and high user subgroup characteristics over the 3 years preceding the study period using linear regression for trend.
The institutional review board at UCSF approved this study protocol.
RESULTS
Of the 2566 unique individuals included in the analysis (Figure 1), 256 individuals were identified as high cost (top decile, $65,000). This group accounted for 45% of all costs and 22% of all hospitalizations (Figure 2). Two hundred fifty individuals were identified as high admits (top decile, 3 hospitalizations). This group accounted for 32% of all costs and 28% of all hospitalizations.
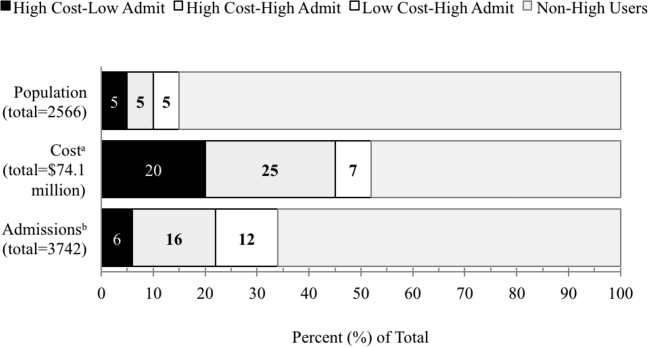
Only 48% of high cost individuals were also high admit ($65,000 and 3 hospitalizations; n=125, Figure 1). Among high users, we subsequently defined 3 subgroups based on the relationship between cost and hospitalizations (high costlow admits, high costhigh admits, and low costhigh admits). Each subgroup accounted for approximately 5% of the overall study population (Figure 2). The high costlow admits subgroup incurred a proportionate share of hospitalizations (6%) but a disproportionate share of costs (20%). The high costhigh admits subgroup had a disproportionate share of both costs (25%) and hospitalizations (16%). The low costhigh admits subgroup had a proportionate share of costs (7%) but a disproportionate share of hospitalizations (12%).
Patient and Hospitalization Characteristics
Compared to non‐high users, all high user subgroups were more likely to have public insurance (Medicare or Medicaid) or have dualeligible status, and the two high cost subgroups were more likely to be male and African American (P<0.05 for all). Compared to each other, subgroups were similar with respect to race/ethnicity, payer, and age (Table 1).
Non‐High Users,n=2145 | High User Subgroups | ||||||
---|---|---|---|---|---|---|---|
1 | 2 | 3 | P Value for Comparison | ||||
High CostLow Admit, n=131 | High CostHigh Admit, n=125 | Low CostHigh Admit, n=125 | 1 vs 2 | 2 vs 3 | 1 vs 3 | ||
| |||||||
Male, % | 34 | 57 | 42 | 38 | 0.02 | 0.52 | 0.003 |
Race/ethnicity, % | 0.15 | 0.76 | 0.18 | ||||
White | 45 | 46 | 36 | 38 | |||
Black | 14 | 21 | 26 | 19 | |||
Hispanic | 9 | 5 | 10 | 10 | |||
Asian | 22 | 18 | 22 | 26 | |||
Other | 10 | 10 | 6 | 6 | |||
Primary payer, % | 0.23 | 0.44 | 0.51 | ||||
Commercial | 42 | 24 | 20 | 17 | |||
Medicare | 42 | 58 | 55 | 61 | |||
Medicaid | 15 | 17 | 25 | 21 | |||
Other | <1 | 2 | 2 | ||||
Dual eligible, %* | 18 | 27 | 26 | 31 | 0.85 | 0.38 | 0.48 |
Age, mean yearsSD | 5720 | 6017 | 5817 | 6320 | 0.40 | 0.04 | 0.19 |
No. of hospitalizations per individual, median (interquartile range) | 1 (11) | 2 (12) | 4 (36) | 3 (34) | <0.001 | <0.001 | <0.001 |
Hospital costs per individual, median $1000 (interquartile range) | 12 (722) | 93 (75122) | 113 (85174) | 37 (3051) | <0.001 | <0.001 | <0.001 |
Regarding hospitalization characteristics, each high user subgroup was distinct and significantly different from each of the other subgroups with respect to admission source, proportion of 30‐day readmissions, LOS, and cost per hospitalization (Table 2, P<0.001 for all). The low costhigh admit subgroup had the highest proportion of admissions from the ED (73%), a moderate proportion of 30‐day readmissions (32%), the shortest LOS (median, 3 days; interquartile range [IQR], 24 days) and the lowest cost per hospitalization (median, $12,000; IQR, $8,000$15,000). In contrast, the high costlow admit subgroup had the highest proportion of admissions from clinic or physician referrals (45%), lowest proportion of 30‐day readmissions (17%), the longest LOS (median, 10; IQR, 417), the most ICU days per hospitalization (median, 1; range, 049) and the highest cost per hospitalization (median, $68,000; IQR, $43,000$95,000). High costhigh admit individuals had the highest proportion of 30‐day readmissions (47%) and a moderate cost per hospitalization (median, $28,000; IQR, $23,000$38,000), but the highest median cost per individual over 1 year ($113,000; IQR, $85,000$174,000, Table 1). Hospitalizations classified as 30‐day readmissions accounted for 42% of costs incurred by this subgroup; 30‐day readmissions specifically associated with CMS core conditions accounted for <1% of costs.
Non‐High Users | High User Subgroups | ||||||
---|---|---|---|---|---|---|---|
1 | 2 | 3 | P Value for Comparison | ||||
High CostLow Admit | High CostHigh Admit | Low CostHigh Admit | 1 vs 2 | 2 vs 3 | 1 vs 3 | ||
| |||||||
No. of admissions | 2500 | 206 | 605 | 431 | |||
Admission source, % | <0.001 | <0.001 | <0.001 | ||||
Emergency department | 53 | 50 | 65 | 73 | |||
Clinic or physician referral | 44 | 45 | 30 | 20 | |||
Transfer from outside facility | 2 | 5 | 4 | 4 | |||
Self‐referral | 1 | <1 | 1 | 3 | |||
Other | <1 | ||||||
30‐day readmission, % | 5 | 17 | 47 | 32 | <0.001 | <0.001 | <0.001 |
LOS, median days (IQR) | 3 (24) | 10 (417) | 5 (310) | 3 (24) | <0.001 | <0.001 | <0.001 |
ICU days, median (range)* | 0 (08) | 1 (049) | 0 (021) | 0 (03) | <0.001 | <0.001 | <0.001 |
Cost per hospitalization, median $1,000 (IQR) | 11 (719) | 68 (4395) | 28 (2338) | 12 (815) | <0.001 | <0.001 | <0.001 |
Encounter diagnoses | <0.001 | 0.002 | <0.001 | ||||
Surgical MS‐DRGs, % | 30 | 58 | 22 | 13 | |||
Most common MDCs | |||||||
Cardiovascular | 4 | 15 | 8 | 6 | |||
Orthopedic | 10 | 13 | 6 | 4 | |||
Transplant | <1 | 7 | 1 | 1 | |||
Medical MS‐DRGs, % | 70 | 42 | 78 | 87 | |||
Most common MDCs | |||||||
Pregnancy related | 17 | 2 | 2 | 2 | |||
Cardiovascular | 10 | 10 | 7 | 13 | |||
Respiratory | 9 | 4 | 14 | 17 | |||
Gastrointestinal | 7 | 3 | 10 | 14 | |||
Hematologic | 1 | 2 | 9 | 6 | |||
Myeloproliferative | <1 | 4 | 9 | 6 | |||
CMS core condition | 7 | 3 | 6 | 12 | 0.174 | 0.01 | 0.004 |
Encounter diagnoses associated with hospitalizations were also significantly different between each of the high user subgroups (Table 2, P<0.001 for all). The high costlow admit subgroup was predominantly hospitalized for surgical conditions (58% vs 42% for medical MS‐DRGs) and had the lowest proportion of hospitalizations for CMS core conditions (3%). The most common types of surgical hospitalizations in this subgroup were for cardiovascular procedures (15%; including coronary artery bypass grafting and cardiac valve replacement) and orthopedic procedures (13%; including hip, knee, and other joint replacements). Most surgical hospitalizations were from referrals (67%) rather than admissions through the ED. In contrast, the low costhigh admit group was predominantly hospitalized for medical conditions (87% vs 13% for surgical MS‐DRGs) and had the highest proportion of hospitalizations for CMS core conditions (12%). The most common types of medical hospitalizations in this subgroup were for respiratory conditions (17%; including chronic obstructive pulmonary disease and pneumonia), gastrointestinal conditions (14%), and cardiovascular conditions (13%; including CHF, AMI, arrhythmia, and chest pain). High costhigh admit individuals were also hospitalized primarily for medical rather than surgical conditions (78% vs 22% medical vs surgical MS‐DRGs). Only 6% of hospitalizations in this subgroup were for CMS core conditions, and only 2% of hospitalizations were 30‐day readmissions for CMS core conditions.
The overlap between the high cost and high admit groups was persistently 48% or less for the 3 years prior to the study period (Table 3). Although the extent of overlap was similar across years, the absolute dollar value for the cutoff to define the top decile by hospital costs gradually increased over time from $47,000 in 2008 to $65,000 in 2011 (P<0.001 for trend). Among the high costlow admit subgroup, there was a trend toward a decrease in the proportion of surgical hospitalizations from 67% in 2008 to 58% in 2011 (P=0.09).
2008 | 2009 | 2010 | 2011 | P Value (For Linear Trend) | |
---|---|---|---|---|---|
| |||||
Study population | 2408 | 2518 | 2647 | 2566 | |
Characteristics, n | |||||
Cutoff for high cost (top decile), nearest $1000 | >47 | >51 | >54 | >65 | <0.001 |
Proportion of total hospital costs incurred by high cost group, % | 46 | 47 | 47 | 48 | |
Cutoff for high admit (top decile), no. of admissions | 3 | 3 | 3 | 3 | |
High cost who are also high admit, % | 42 | 48 | 48 | 48 | |
Discharge diagnoses by subgroup* | |||||
Non‐high user population | |||||
Surgical MS‐DRG | 32 (751) | 33 (842) | 36 (932) | 30 (751) | 0.51 |
Medical MS‐DRG | 68 (1598) | 67 (1676) | 64 (1673) | 70 (1749) | |
High costlow admit | |||||
Surgical MS‐DRG | 67 (138) | 68 (132) | 61 (120) | 58 (119) | 0.09 |
Medical MS‐DRG | 33(67) | 32 (63) | 39 (78) | 42 (87) | |
High costhigh admit | |||||
Surgical MS‐DRG | 23 (104) | 25 (133) | 24 (150) | 22 (134) | 0.60 |
Medical MS‐DRG | 77 (341) | 75 (392) | 76 (464) | 78 (471) | |
Low costhigh admit | |||||
Surgical MS‐DRG | 11 (35) | 17 (44) | 13 (40) | 13 (54) | 0.90 |
Medical MS‐DRG | 89 (277) | 83 (219) | 87 (269) | 87 (377) |
DISCUSSION
In this study, we found that only half of high cost individuals were also high admit. Further categorizing high users into high costlow admit versus high costhigh admit versus low costhigh admit identified distinct patterns between each group. High costhigh admit individuals were more likely to be hospitalized for medical conditions, whereas high costlow admit individuals were more likely to be hospitalized for surgical conditions. CMS core conditions accounted for a low proportion of overall hospitalizations across all groups.
Our findings suggest several distinct types of high users with different clinical characteristics, utilization, and cost patterns. From a hospital perspective, one implication is that a multifaceted approach to cost containment, rather than the one‐size‐fits‐all strategy of reducing hospitalizations, may be more effective in reducing costs. For example, our findings show that high costlow admit individuals have a disproportionate number of hospitalizations for surgical conditions, longer LOS, and more ICU days. Costs incurred by this subgroup may be more responsive to in‐hospital interventions aimed at reducing procedural costs, LOS, unnecessary use of the ICU, and minimizing postoperative infections and complications rather than to a care management approach.
In contrast, care management strategies such as improving postdischarge care and chronic disease management, which aim to achieve cost savings through reducing hospitalizations, may be more effective in reducing costs among high costhigh admit individuals, who have a high proportion of hospitalizations for medical conditions and the highest proportion of 30‐day readmissions. Such strategies may also be important in optimizing the quality of care for low costhigh admit individuals, who have the highest proportion of medical hospitalizations among all high users, though the potential for cost savings may be more limited in this subgroup.
Our results suggest that current hospital‐based approachesdriven by readmissions penalties for CMS core conditionsmay have less than the expected impact on costs. For example, although high costhigh admit individuals had the highest proportion of 30‐day readmissions, readmissions specifically for CMS core conditions accounted for <1% of costs in this subgroup. Thus, the potential return on an expensive investment in a care management intervention is unclear, given the small number of readmissions for these select conditions. From a broader perspective, the focus on readmissions for CMS core conditions, which overall contribute relatively little to high hospital costs, may not be a comprehensive enough strategy for cost containment. To date, there have been limited policies targeting factors contributing to high hospital costs outside of frequent medical hospitalizations. Medicare's nonpayment policy for treatment of preventable hospital conditions, including surgical site infections, translates prevention of these conditions into cost savings for hospitals.[30] However, this rule has been criticized for not going far enough to drive substantial savings.[31] A new CMS rule authorizes states to identify other provider‐preventable conditions for which Medicaid payment will be prohibited.[32] Future policy efforts should further emphasize a comprehensive, multipronged approach beyond readmissions penalties for select conditions if healthcare cost containment remains a policy priority.
Our results should be interpreted in light of several limitations. First, this was a single‐site study at an academic medical center; the generalizability of our results to other settings is unclear. Our cost data likely reflect local market factors, including the highest wage rates for skilled healthcare labor in the United States.[33] Although the explicit distribution of high user subgroups may be institution‐specific due to variations in our cost structure, we anticipate that the general classification paradigm will be similar in other health systems. Second, we captured utilization and costs only at a single hospital. However, our study population includes only individuals with PCPs at UCSF, and internal data from both Medicare and UCSF's largest private payer show that over 85% of hospitalizations among this population are to UCSF Medical Center. Third, we were able to capture only hospital costs rather than overall healthcare costs. Given that hospital costs account for the single largest category of total national health costs,[4] we expect that future studies examining total health costs will show similar findings. Fourth, our data did not include measures of health status, socioeconomic status, housing, or mental health comorbidities to permit an analysis of these factors, which have been previously related to frequent hospitalizations and high costs.[34, 35, 36, 37, 38, 39] Fifth, due to resource constraints, we were unable to conduct a longitudinal analysis to examine the extent to which individuals are consistently high users over time. Previous studies have described that 20% to 30% of individuals are consistently high users; the remainder have discrete periods of high utilization.[34, 40] This may be an important consideration in the design of future interventions.
Finally, our analysis was limited to individuals with a PCP to allow identification of an accessible cohort for care management. Thus, we did not capture individuals without a PCP and individuals who died during the study period, because these individuals no longer had an assigned PCP following death. Although this approach is consistent with that of many care management programs,[19] these populations are likely to incur higher than average utilization and healthcare costs, and represent important areas for future investigation.
In summary, our study identifies three types of high‐user populations that differ in the proportion of costs attributable to frequent hospitalizations, clinical conditions associated with hospital use, and frequency of 30‐day readmissions. Stratifying high users by both costs and hospitalizations may help identify tailored strategies to more effectively reduce costs and utilization.
Acknowledgments
The authors acknowledge Diana Patterson, Leanna Zaporozhets, and Andre Devito for their assistance in data collection.
Disclosures: Dr. Nguyen had full access to all of the data in the study and takes responsibility for the integrity of the data and the accuracy of the data analysis. Dr. Nguyen's work on this project was completed as a primary care research fellow at the University of California, San Francisco funded by a federal training grant from the National Research Service Award (NRSA T32HP19025‐07‐00).
Despite signs of a slowing trend,[1, 2, 3] US healthcare costs continue to rise, and cost containment remains a major area of concern. Hospital costs are the largest single category of national healthcare expenditures,[4] and the burden of cost containment is increasingly being shifted to hospitals.[5] As such, hospitals are increasingly focusing on implementing interventions to reduce rates of hospitalizations and readmissions as a mechanism to reduce overall healthcare costs.[5, 6, 7, 8, 9]
Multiple factors potentially contribute to patients being high cost, including acute care utilization,[10, 11, 12] pharmaceuticals,[13, 14] procedures,[15] catastrophic illness,[16] and high‐risk chronic conditions.[11, 17, 18] However, many hospitals are implementing interventions focused on a single subset of these high cost patientshigh users of inpatient services. Care management interventions have received particular attention, due to their perceived potential to improve quality of care while reducing costs through the mechanism of reducing hospital admissions.[19, 20, 21] Despite their increasing prevalence, there is limited evidence demonstrating the effectiveness of these programs. Among interventions targeting high cost individuals, the Medicare Care Management for High Cost Beneficiaries showed no effect on hospital admissions.[19] Another high‐profile intervention, the Citywide Care Management System led by the Camden Coalition, showed promising preliminary results, but data from a systematic evaluation are lacking.[22] Conversely, interventions targeting individuals with frequent hospitalizations have similarly shown mixed results in reducing costs.[6, 7, 9, 23]
Taken together, these data suggest that the relationship between high costs and frequent hospital use is complicated, and the population of individuals with frequent hospitalizations may not represent the entire population of high cost individuals. Thus, focusing on reduction of hospitalizations alone may be inadequate to reduce costs. For these reasons, we sought to determine how many high cost individuals would be captured by focusing only on frequently hospitalized (high admit) individuals by examining the overlap between these populations. We also sought to describe the characteristics and distinctions between the resulting subgroups of high users to better inform the design of future interventions.
METHODS
We examined the cross‐sectional relationship between high cost and high admit populations among adult patients 18 years of age hospitalized at the University of California, San Francisco (UCSF) Medical Center, a 660‐bed urban academic medical center from July 1, 2010 to June 30, 2011.
This study was conducted as part of a quality improvement project to identify high user primary care patients for complex care management. Individuals were included in the study if: (1) they had an assigned UCSF primary care provider (PCP), and (2) they had at least 1 hospitalization at UCSF during the study period. PCP assignments were ascertained from panel lists maintained by clinics; lists included individuals with at least 1 visit at any of the 8 primary care clinics at UCSF in the 2 years prior to the end of the study period. Because individuals are dropped from PCP panels at death, we were unable to ascertain or include individuals who died during the study period.
From the initial study population, we defined the high cost group as those who were in the top decile of total hospital costs, and the high admit group as those who were in the top decile of total hospitalizations during the study period. We elected to use the top decile as a cutoff given that it is a common operational definition used to identify high users to target for intervention.[24]
To examine the relationship between high cost and high admits we defined 3 further subgroups: high costlow admits, high costhigh admits, and low costhigh admits (Figure 1). To explore the face validity of these descriptors and classification scheme, we subsequently examined the proportion of total hospital costs and total hospitalizations each subgroup accounted for with respect to the study population.
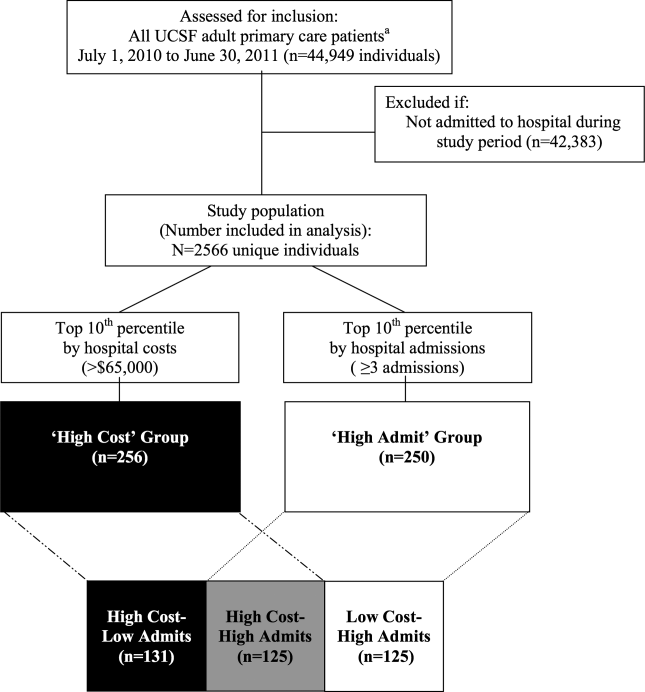
Data Sources
Hospital costs, demographic data, and encounter diagnoses were obtained from the hospital's Transition Systems Incorporated system (TSI, also known as Eclipsys or Allscripts), a commercially available automated cost accounting system that integrates multiple data sources to calculate total hospital costs on a per patient basis. Several studies have previously used the TSI system to estimate the costs of healthcare services at individual hospitals, and this approach is generally considered the most accurate method to estimate cost.[25, 26] Hospital costs included the sum of actual total costs (not billed charges) for all hospital episodes including lab costs, drug costs, surgical supplies, nurse salaries and benefits, utilities, housekeeping, and allocated administrative overhead. This cost total does not capture the cost of physician labor (pro‐fees), preadmission costs (e.g., outpatient care), or postadmission costs (e.g., home health, nursing home, or other postdischarge care). Preadmission lab, diagnostic tests, and imaging were included in hospital costs if these were ordered within 72 hours of hospital admission. Emergency department (ED) costs were included if an individual was admitted to the hospital via the ED. Hospitalizations were defined as inpatient admissions only to UCSF because we were unable to reliably ascertain hospitalizations outside of UCSF. PCP assignments were ascertained from administrative panel lists maintained by clinics.
Study Variables
We analyzed factors previously shown[13, 27, 28, 29] to be associated with high healthcare cost and utilization. We examined demographic characteristics and hospitalization characteristics, including admission source, length of stay (LOS), cost per hospitalization, whether the episode was a 30‐day readmission, days in the intensive care unit (ICU), and encounter diagnoses.
To ascertain whether a hospitalization was for a medical versus surgical condition, we used discharge diagnosis codes and designations as per the Medicare Severity Diagnosis‐Related Groups (MS‐DRG) versions 27 and 28 definitions manuals. We subsequently grouped medical and surgical conditions by Major Diagnostic Categories as per the MS‐DRG definitions manuals.
Using MS‐DRG codes, we also classified whether hospitalizations were for pneumonia, acute myocardial infarction (AMI), and congestive heart failure (CHF), as these 3 conditions have specific payment penalties under the Centers for Medicare & Medicaid Services (CMS) reimbursement policies. For these CMS core conditions, we included hospitalizations with MS‐DRG codes 193195, 280282, and 291293 (codes 283285 were not included for AMI because individuals who died during the study period were excluded.)
Analysis
We used descriptive statistics to compare patient and hospitalization characteristics between subgroups. Non‐normally distributed variables including LOS and cost per hospitalization were log transformed. Because a single individual could account for multiple hospitalizations, we performed a companion analysis of hospitalization characteristics using generalized estimating equations with an independent correlation structure to account for clustering of hospitalizations within individuals. Our findings were robust using either approach. For ease of interpretation, P values from the former analysis are presented.
To determine whether the overall distribution and characteristics we observed for high user subgroups were a single‐year anomaly or representative of temporally stable trends, we compared non‐high users and high user subgroup characteristics over the 3 years preceding the study period using linear regression for trend.
The institutional review board at UCSF approved this study protocol.
RESULTS
Of the 2566 unique individuals included in the analysis (Figure 1), 256 individuals were identified as high cost (top decile, $65,000). This group accounted for 45% of all costs and 22% of all hospitalizations (Figure 2). Two hundred fifty individuals were identified as high admits (top decile, 3 hospitalizations). This group accounted for 32% of all costs and 28% of all hospitalizations.
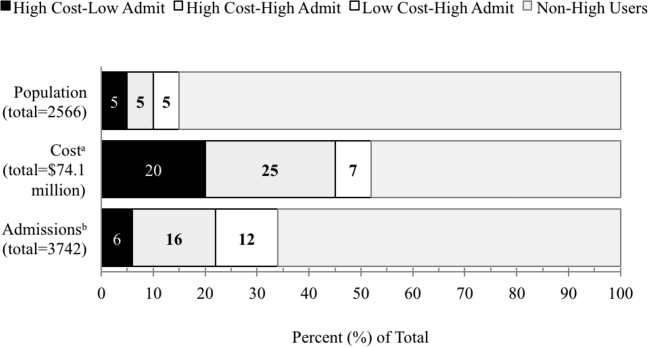
Only 48% of high cost individuals were also high admit ($65,000 and 3 hospitalizations; n=125, Figure 1). Among high users, we subsequently defined 3 subgroups based on the relationship between cost and hospitalizations (high costlow admits, high costhigh admits, and low costhigh admits). Each subgroup accounted for approximately 5% of the overall study population (Figure 2). The high costlow admits subgroup incurred a proportionate share of hospitalizations (6%) but a disproportionate share of costs (20%). The high costhigh admits subgroup had a disproportionate share of both costs (25%) and hospitalizations (16%). The low costhigh admits subgroup had a proportionate share of costs (7%) but a disproportionate share of hospitalizations (12%).
Patient and Hospitalization Characteristics
Compared to non‐high users, all high user subgroups were more likely to have public insurance (Medicare or Medicaid) or have dualeligible status, and the two high cost subgroups were more likely to be male and African American (P<0.05 for all). Compared to each other, subgroups were similar with respect to race/ethnicity, payer, and age (Table 1).
Non‐High Users,n=2145 | High User Subgroups | ||||||
---|---|---|---|---|---|---|---|
1 | 2 | 3 | P Value for Comparison | ||||
High CostLow Admit, n=131 | High CostHigh Admit, n=125 | Low CostHigh Admit, n=125 | 1 vs 2 | 2 vs 3 | 1 vs 3 | ||
| |||||||
Male, % | 34 | 57 | 42 | 38 | 0.02 | 0.52 | 0.003 |
Race/ethnicity, % | 0.15 | 0.76 | 0.18 | ||||
White | 45 | 46 | 36 | 38 | |||
Black | 14 | 21 | 26 | 19 | |||
Hispanic | 9 | 5 | 10 | 10 | |||
Asian | 22 | 18 | 22 | 26 | |||
Other | 10 | 10 | 6 | 6 | |||
Primary payer, % | 0.23 | 0.44 | 0.51 | ||||
Commercial | 42 | 24 | 20 | 17 | |||
Medicare | 42 | 58 | 55 | 61 | |||
Medicaid | 15 | 17 | 25 | 21 | |||
Other | <1 | 2 | 2 | ||||
Dual eligible, %* | 18 | 27 | 26 | 31 | 0.85 | 0.38 | 0.48 |
Age, mean yearsSD | 5720 | 6017 | 5817 | 6320 | 0.40 | 0.04 | 0.19 |
No. of hospitalizations per individual, median (interquartile range) | 1 (11) | 2 (12) | 4 (36) | 3 (34) | <0.001 | <0.001 | <0.001 |
Hospital costs per individual, median $1000 (interquartile range) | 12 (722) | 93 (75122) | 113 (85174) | 37 (3051) | <0.001 | <0.001 | <0.001 |
Regarding hospitalization characteristics, each high user subgroup was distinct and significantly different from each of the other subgroups with respect to admission source, proportion of 30‐day readmissions, LOS, and cost per hospitalization (Table 2, P<0.001 for all). The low costhigh admit subgroup had the highest proportion of admissions from the ED (73%), a moderate proportion of 30‐day readmissions (32%), the shortest LOS (median, 3 days; interquartile range [IQR], 24 days) and the lowest cost per hospitalization (median, $12,000; IQR, $8,000$15,000). In contrast, the high costlow admit subgroup had the highest proportion of admissions from clinic or physician referrals (45%), lowest proportion of 30‐day readmissions (17%), the longest LOS (median, 10; IQR, 417), the most ICU days per hospitalization (median, 1; range, 049) and the highest cost per hospitalization (median, $68,000; IQR, $43,000$95,000). High costhigh admit individuals had the highest proportion of 30‐day readmissions (47%) and a moderate cost per hospitalization (median, $28,000; IQR, $23,000$38,000), but the highest median cost per individual over 1 year ($113,000; IQR, $85,000$174,000, Table 1). Hospitalizations classified as 30‐day readmissions accounted for 42% of costs incurred by this subgroup; 30‐day readmissions specifically associated with CMS core conditions accounted for <1% of costs.
Non‐High Users | High User Subgroups | ||||||
---|---|---|---|---|---|---|---|
1 | 2 | 3 | P Value for Comparison | ||||
High CostLow Admit | High CostHigh Admit | Low CostHigh Admit | 1 vs 2 | 2 vs 3 | 1 vs 3 | ||
| |||||||
No. of admissions | 2500 | 206 | 605 | 431 | |||
Admission source, % | <0.001 | <0.001 | <0.001 | ||||
Emergency department | 53 | 50 | 65 | 73 | |||
Clinic or physician referral | 44 | 45 | 30 | 20 | |||
Transfer from outside facility | 2 | 5 | 4 | 4 | |||
Self‐referral | 1 | <1 | 1 | 3 | |||
Other | <1 | ||||||
30‐day readmission, % | 5 | 17 | 47 | 32 | <0.001 | <0.001 | <0.001 |
LOS, median days (IQR) | 3 (24) | 10 (417) | 5 (310) | 3 (24) | <0.001 | <0.001 | <0.001 |
ICU days, median (range)* | 0 (08) | 1 (049) | 0 (021) | 0 (03) | <0.001 | <0.001 | <0.001 |
Cost per hospitalization, median $1,000 (IQR) | 11 (719) | 68 (4395) | 28 (2338) | 12 (815) | <0.001 | <0.001 | <0.001 |
Encounter diagnoses | <0.001 | 0.002 | <0.001 | ||||
Surgical MS‐DRGs, % | 30 | 58 | 22 | 13 | |||
Most common MDCs | |||||||
Cardiovascular | 4 | 15 | 8 | 6 | |||
Orthopedic | 10 | 13 | 6 | 4 | |||
Transplant | <1 | 7 | 1 | 1 | |||
Medical MS‐DRGs, % | 70 | 42 | 78 | 87 | |||
Most common MDCs | |||||||
Pregnancy related | 17 | 2 | 2 | 2 | |||
Cardiovascular | 10 | 10 | 7 | 13 | |||
Respiratory | 9 | 4 | 14 | 17 | |||
Gastrointestinal | 7 | 3 | 10 | 14 | |||
Hematologic | 1 | 2 | 9 | 6 | |||
Myeloproliferative | <1 | 4 | 9 | 6 | |||
CMS core condition | 7 | 3 | 6 | 12 | 0.174 | 0.01 | 0.004 |
Encounter diagnoses associated with hospitalizations were also significantly different between each of the high user subgroups (Table 2, P<0.001 for all). The high costlow admit subgroup was predominantly hospitalized for surgical conditions (58% vs 42% for medical MS‐DRGs) and had the lowest proportion of hospitalizations for CMS core conditions (3%). The most common types of surgical hospitalizations in this subgroup were for cardiovascular procedures (15%; including coronary artery bypass grafting and cardiac valve replacement) and orthopedic procedures (13%; including hip, knee, and other joint replacements). Most surgical hospitalizations were from referrals (67%) rather than admissions through the ED. In contrast, the low costhigh admit group was predominantly hospitalized for medical conditions (87% vs 13% for surgical MS‐DRGs) and had the highest proportion of hospitalizations for CMS core conditions (12%). The most common types of medical hospitalizations in this subgroup were for respiratory conditions (17%; including chronic obstructive pulmonary disease and pneumonia), gastrointestinal conditions (14%), and cardiovascular conditions (13%; including CHF, AMI, arrhythmia, and chest pain). High costhigh admit individuals were also hospitalized primarily for medical rather than surgical conditions (78% vs 22% medical vs surgical MS‐DRGs). Only 6% of hospitalizations in this subgroup were for CMS core conditions, and only 2% of hospitalizations were 30‐day readmissions for CMS core conditions.
The overlap between the high cost and high admit groups was persistently 48% or less for the 3 years prior to the study period (Table 3). Although the extent of overlap was similar across years, the absolute dollar value for the cutoff to define the top decile by hospital costs gradually increased over time from $47,000 in 2008 to $65,000 in 2011 (P<0.001 for trend). Among the high costlow admit subgroup, there was a trend toward a decrease in the proportion of surgical hospitalizations from 67% in 2008 to 58% in 2011 (P=0.09).
2008 | 2009 | 2010 | 2011 | P Value (For Linear Trend) | |
---|---|---|---|---|---|
| |||||
Study population | 2408 | 2518 | 2647 | 2566 | |
Characteristics, n | |||||
Cutoff for high cost (top decile), nearest $1000 | >47 | >51 | >54 | >65 | <0.001 |
Proportion of total hospital costs incurred by high cost group, % | 46 | 47 | 47 | 48 | |
Cutoff for high admit (top decile), no. of admissions | 3 | 3 | 3 | 3 | |
High cost who are also high admit, % | 42 | 48 | 48 | 48 | |
Discharge diagnoses by subgroup* | |||||
Non‐high user population | |||||
Surgical MS‐DRG | 32 (751) | 33 (842) | 36 (932) | 30 (751) | 0.51 |
Medical MS‐DRG | 68 (1598) | 67 (1676) | 64 (1673) | 70 (1749) | |
High costlow admit | |||||
Surgical MS‐DRG | 67 (138) | 68 (132) | 61 (120) | 58 (119) | 0.09 |
Medical MS‐DRG | 33(67) | 32 (63) | 39 (78) | 42 (87) | |
High costhigh admit | |||||
Surgical MS‐DRG | 23 (104) | 25 (133) | 24 (150) | 22 (134) | 0.60 |
Medical MS‐DRG | 77 (341) | 75 (392) | 76 (464) | 78 (471) | |
Low costhigh admit | |||||
Surgical MS‐DRG | 11 (35) | 17 (44) | 13 (40) | 13 (54) | 0.90 |
Medical MS‐DRG | 89 (277) | 83 (219) | 87 (269) | 87 (377) |
DISCUSSION
In this study, we found that only half of high cost individuals were also high admit. Further categorizing high users into high costlow admit versus high costhigh admit versus low costhigh admit identified distinct patterns between each group. High costhigh admit individuals were more likely to be hospitalized for medical conditions, whereas high costlow admit individuals were more likely to be hospitalized for surgical conditions. CMS core conditions accounted for a low proportion of overall hospitalizations across all groups.
Our findings suggest several distinct types of high users with different clinical characteristics, utilization, and cost patterns. From a hospital perspective, one implication is that a multifaceted approach to cost containment, rather than the one‐size‐fits‐all strategy of reducing hospitalizations, may be more effective in reducing costs. For example, our findings show that high costlow admit individuals have a disproportionate number of hospitalizations for surgical conditions, longer LOS, and more ICU days. Costs incurred by this subgroup may be more responsive to in‐hospital interventions aimed at reducing procedural costs, LOS, unnecessary use of the ICU, and minimizing postoperative infections and complications rather than to a care management approach.
In contrast, care management strategies such as improving postdischarge care and chronic disease management, which aim to achieve cost savings through reducing hospitalizations, may be more effective in reducing costs among high costhigh admit individuals, who have a high proportion of hospitalizations for medical conditions and the highest proportion of 30‐day readmissions. Such strategies may also be important in optimizing the quality of care for low costhigh admit individuals, who have the highest proportion of medical hospitalizations among all high users, though the potential for cost savings may be more limited in this subgroup.
Our results suggest that current hospital‐based approachesdriven by readmissions penalties for CMS core conditionsmay have less than the expected impact on costs. For example, although high costhigh admit individuals had the highest proportion of 30‐day readmissions, readmissions specifically for CMS core conditions accounted for <1% of costs in this subgroup. Thus, the potential return on an expensive investment in a care management intervention is unclear, given the small number of readmissions for these select conditions. From a broader perspective, the focus on readmissions for CMS core conditions, which overall contribute relatively little to high hospital costs, may not be a comprehensive enough strategy for cost containment. To date, there have been limited policies targeting factors contributing to high hospital costs outside of frequent medical hospitalizations. Medicare's nonpayment policy for treatment of preventable hospital conditions, including surgical site infections, translates prevention of these conditions into cost savings for hospitals.[30] However, this rule has been criticized for not going far enough to drive substantial savings.[31] A new CMS rule authorizes states to identify other provider‐preventable conditions for which Medicaid payment will be prohibited.[32] Future policy efforts should further emphasize a comprehensive, multipronged approach beyond readmissions penalties for select conditions if healthcare cost containment remains a policy priority.
Our results should be interpreted in light of several limitations. First, this was a single‐site study at an academic medical center; the generalizability of our results to other settings is unclear. Our cost data likely reflect local market factors, including the highest wage rates for skilled healthcare labor in the United States.[33] Although the explicit distribution of high user subgroups may be institution‐specific due to variations in our cost structure, we anticipate that the general classification paradigm will be similar in other health systems. Second, we captured utilization and costs only at a single hospital. However, our study population includes only individuals with PCPs at UCSF, and internal data from both Medicare and UCSF's largest private payer show that over 85% of hospitalizations among this population are to UCSF Medical Center. Third, we were able to capture only hospital costs rather than overall healthcare costs. Given that hospital costs account for the single largest category of total national health costs,[4] we expect that future studies examining total health costs will show similar findings. Fourth, our data did not include measures of health status, socioeconomic status, housing, or mental health comorbidities to permit an analysis of these factors, which have been previously related to frequent hospitalizations and high costs.[34, 35, 36, 37, 38, 39] Fifth, due to resource constraints, we were unable to conduct a longitudinal analysis to examine the extent to which individuals are consistently high users over time. Previous studies have described that 20% to 30% of individuals are consistently high users; the remainder have discrete periods of high utilization.[34, 40] This may be an important consideration in the design of future interventions.
Finally, our analysis was limited to individuals with a PCP to allow identification of an accessible cohort for care management. Thus, we did not capture individuals without a PCP and individuals who died during the study period, because these individuals no longer had an assigned PCP following death. Although this approach is consistent with that of many care management programs,[19] these populations are likely to incur higher than average utilization and healthcare costs, and represent important areas for future investigation.
In summary, our study identifies three types of high‐user populations that differ in the proportion of costs attributable to frequent hospitalizations, clinical conditions associated with hospital use, and frequency of 30‐day readmissions. Stratifying high users by both costs and hospitalizations may help identify tailored strategies to more effectively reduce costs and utilization.
Acknowledgments
The authors acknowledge Diana Patterson, Leanna Zaporozhets, and Andre Devito for their assistance in data collection.
Disclosures: Dr. Nguyen had full access to all of the data in the study and takes responsibility for the integrity of the data and the accuracy of the data analysis. Dr. Nguyen's work on this project was completed as a primary care research fellow at the University of California, San Francisco funded by a federal training grant from the National Research Service Award (NRSA T32HP19025‐07‐00).
- National Health Expenditure Accounts Team. Recession contributes to slowest annual rate of increase in health spending in five decades. Health Aff (Millwood). 2011;30(1):11–22. , , , ;
- Growth in US health spending remained slow in 2010; health share of gross domestic product was unchanged from 2009. Health Aff (Millwood). 2012;31(1):208–219. , , , .
- the National Health Expenditure Accounts Team. National health spending in 2011: overall growth remains low, but some payers and services show signs of acceleration. Health Aff (Millwood). 2013;32(1):87–99. , , , ;
- Centers for Medicare and Medicaid Services; Office of the Actuary; National Health Statistics Group. National healthcare expenditures data. 2012. Available at: https://www.cms.gov/Research‐Statistics‐Data‐and‐Systems/Statistics‐Trends‐and‐Reports/NationalHealthExpendData/downloads/tables.pdf.
- Medicare Payment Advisory Commission. Report to the Congress: Promoting Greater Efficiency in Medicare. Washington, DC: Medicare Payment Advisory Commission; 2007.
- The care transitions intervention: results of a randomized controlled trial. Arch Intern Med. 2006;166(17):1822–1828. , , , .
- A reengineered hospital discharge program to decrease rehospitalization: a randomized trial. Ann Intern Med. 2009;150(3):178–187. , , , et al.
- How‐to Guide: Improving Transitions from the Hospital to the Clinical Office Practice to Reduce Avoidable Rehospitalizations. Cambridge, MA: Institute for Healthcare Improvement; 2012. , , , .
- Comprehensive discharge planning and home follow‐up of hospitalized elders: a randomized clinical trial. JAMA. 1999;281(7):613–620. , , , et al.
- High‐cost users of medical care. N Engl J Med. 1980;302(18):996–1002. , .
- Frequency and clinical description of high‐cost patients in 17 acute‐care hospitals. N Engl J Med. 1979;300(23):1306–1309. , , .
- Effectiveness of interventions targeting frequent users of emergency departments: a systematic review. Ann Emerg Med. 2011;58(1):41–52.e42. , , , et al.
- Medication, diagnostic, and cost information as predictors of high‐risk patients in need of care management. Am J Manag Care. 2009;15(1):41–48. , , , .
- Tracking health care costs: trends stabilize but remain high in 2002. Health Aff (Millwood). 2003;Suppl Web Exclusives:W3–266–274. , .
- An iconoclastic view of health cost containment. Health Aff (Millwood). 1993;12(suppl):152–171. .
- Comparisons of medical utilizations and categorical diagnoses of emergency visits between the elderly with catastrophic illness certificates and those without. BMC Health Serv Res. 2013;13:152. , , , et al.
- Finding future high‐cost cases: comparing prior cost versus diagnosis‐based methods. Health Serv Res. 2001;36(6 pt 2):194–206. , , , .
- Spending and service use among people with the fifteen most costly medical conditions, 1997. Health Aff (Millwood). 2003;22(2):129–138. , .
- Lessons from Medicare's Demonstration Projects on Disease Management and Care Coordination. Washington, D.C.: Congressional Budget Office; 2012. .
- The hot spotters. The New Yorker. January 24, 2011. .
- Collaboration Across the Disciplines in Health Care. Burlington, MA: Jones and Bartlett; 2010. , , .
- Hope for New Jersey's city hospitals: the Camden Initiative. Perspect Health Inf Manag. 2010:7:1d. , , .
- Hospital‐initiated transitional care interventions as a patient safety strategy: a systematic review. Ann Intern Med. 2013;158(5 pt 2):433–440. , , , , , .
- Agency for Healthcare Research and Quality. The concentration and persistence in the level of health expenditures over time: estimates for the U.S. population, 2008–2009. 2012. Available at: http://meps.ahrq.gov/mepsweb/data_files/publications/st354/stat354.shtml.
- The use of the transition cost accounting system in health services research. Cost Eff Resour Alloc. 2007;5:11. , , , , , .
- Cost reduction and quality improvement: it takes two to tango. Crit Care Med. 2000;28(2):581–583. , .
- Case finding for patients at risk of readmission to hospital: development of algorithm to identify high risk patients. BMJ. 2006;333(7563):327. , , , .
- Risk prediction models for hospital readmission: a systematic review. JAMA. 2011;306(15):1688–1698. , , , et al.
- Chronic disease score as a predictor of hospitalization. Epidemiology. 2002;13(3):340–346. , , , et al.
- Centers for Medicare and Medicaid Services. Hospital‐acquired conditions (HAC) in acute inpatient prospective payment system (IPPS) hospitals fact sheet. 2011. Available at: https://www.cms.gov/Medicare/Medicare‐Fee‐for‐Service‐Payment/HospitalAcqCond/downloads/HACFactsheet.pdf. Accessed August 31, 2013.
- Medicare's policy not to pay for treating hospital‐acquired conditions: the impact. Health Aff (Millwood). 2009;28(5):1485–1493. , , .
- Department of Health and Human Services, Centers for Medicare 2013.
- Consistently high users of medical care among the elderly. Med Care. 1990;28(6):527–540. , , , .
- Utilization patterns among long‐term enrollees in a prepaid group practice health maintenance organization. Med Care. 1985;23(11):1221–1233. , , , .
- Depressive symptoms and health service utilization among the community elderly. J Am Geriatr Soc. 1983;31(7):417–420. , , .
- The influence of socio‐economic status and multimorbidity patterns on healthcare costs: a six‐year follow‐up under a universal healthcare system. Int J Equity Health. 2013;12(1):69. , .
- Association between the level of housing instability, economic standing and health care access: a meta‐regression. J Health Care Poor Underserved. 2008;19(4):1212–1228. , , .
- High health care utilization and costs associated with lower socio‐economic status: results from a linked dataset. Can J Public Health. 2009;100(3):180–183. , , , .
- An analysis of the use of Medicare services by the continuously enrolled aged. Med Care. 1983;21(6):567–585. , .
- National Health Expenditure Accounts Team. Recession contributes to slowest annual rate of increase in health spending in five decades. Health Aff (Millwood). 2011;30(1):11–22. , , , ;
- Growth in US health spending remained slow in 2010; health share of gross domestic product was unchanged from 2009. Health Aff (Millwood). 2012;31(1):208–219. , , , .
- the National Health Expenditure Accounts Team. National health spending in 2011: overall growth remains low, but some payers and services show signs of acceleration. Health Aff (Millwood). 2013;32(1):87–99. , , , ;
- Centers for Medicare and Medicaid Services; Office of the Actuary; National Health Statistics Group. National healthcare expenditures data. 2012. Available at: https://www.cms.gov/Research‐Statistics‐Data‐and‐Systems/Statistics‐Trends‐and‐Reports/NationalHealthExpendData/downloads/tables.pdf.
- Medicare Payment Advisory Commission. Report to the Congress: Promoting Greater Efficiency in Medicare. Washington, DC: Medicare Payment Advisory Commission; 2007.
- The care transitions intervention: results of a randomized controlled trial. Arch Intern Med. 2006;166(17):1822–1828. , , , .
- A reengineered hospital discharge program to decrease rehospitalization: a randomized trial. Ann Intern Med. 2009;150(3):178–187. , , , et al.
- How‐to Guide: Improving Transitions from the Hospital to the Clinical Office Practice to Reduce Avoidable Rehospitalizations. Cambridge, MA: Institute for Healthcare Improvement; 2012. , , , .
- Comprehensive discharge planning and home follow‐up of hospitalized elders: a randomized clinical trial. JAMA. 1999;281(7):613–620. , , , et al.
- High‐cost users of medical care. N Engl J Med. 1980;302(18):996–1002. , .
- Frequency and clinical description of high‐cost patients in 17 acute‐care hospitals. N Engl J Med. 1979;300(23):1306–1309. , , .
- Effectiveness of interventions targeting frequent users of emergency departments: a systematic review. Ann Emerg Med. 2011;58(1):41–52.e42. , , , et al.
- Medication, diagnostic, and cost information as predictors of high‐risk patients in need of care management. Am J Manag Care. 2009;15(1):41–48. , , , .
- Tracking health care costs: trends stabilize but remain high in 2002. Health Aff (Millwood). 2003;Suppl Web Exclusives:W3–266–274. , .
- An iconoclastic view of health cost containment. Health Aff (Millwood). 1993;12(suppl):152–171. .
- Comparisons of medical utilizations and categorical diagnoses of emergency visits between the elderly with catastrophic illness certificates and those without. BMC Health Serv Res. 2013;13:152. , , , et al.
- Finding future high‐cost cases: comparing prior cost versus diagnosis‐based methods. Health Serv Res. 2001;36(6 pt 2):194–206. , , , .
- Spending and service use among people with the fifteen most costly medical conditions, 1997. Health Aff (Millwood). 2003;22(2):129–138. , .
- Lessons from Medicare's Demonstration Projects on Disease Management and Care Coordination. Washington, D.C.: Congressional Budget Office; 2012. .
- The hot spotters. The New Yorker. January 24, 2011. .
- Collaboration Across the Disciplines in Health Care. Burlington, MA: Jones and Bartlett; 2010. , , .
- Hope for New Jersey's city hospitals: the Camden Initiative. Perspect Health Inf Manag. 2010:7:1d. , , .
- Hospital‐initiated transitional care interventions as a patient safety strategy: a systematic review. Ann Intern Med. 2013;158(5 pt 2):433–440. , , , , , .
- Agency for Healthcare Research and Quality. The concentration and persistence in the level of health expenditures over time: estimates for the U.S. population, 2008–2009. 2012. Available at: http://meps.ahrq.gov/mepsweb/data_files/publications/st354/stat354.shtml.
- The use of the transition cost accounting system in health services research. Cost Eff Resour Alloc. 2007;5:11. , , , , , .
- Cost reduction and quality improvement: it takes two to tango. Crit Care Med. 2000;28(2):581–583. , .
- Case finding for patients at risk of readmission to hospital: development of algorithm to identify high risk patients. BMJ. 2006;333(7563):327. , , , .
- Risk prediction models for hospital readmission: a systematic review. JAMA. 2011;306(15):1688–1698. , , , et al.
- Chronic disease score as a predictor of hospitalization. Epidemiology. 2002;13(3):340–346. , , , et al.
- Centers for Medicare and Medicaid Services. Hospital‐acquired conditions (HAC) in acute inpatient prospective payment system (IPPS) hospitals fact sheet. 2011. Available at: https://www.cms.gov/Medicare/Medicare‐Fee‐for‐Service‐Payment/HospitalAcqCond/downloads/HACFactsheet.pdf. Accessed August 31, 2013.
- Medicare's policy not to pay for treating hospital‐acquired conditions: the impact. Health Aff (Millwood). 2009;28(5):1485–1493. , , .
- Department of Health and Human Services, Centers for Medicare 2013.
- Consistently high users of medical care among the elderly. Med Care. 1990;28(6):527–540. , , , .
- Utilization patterns among long‐term enrollees in a prepaid group practice health maintenance organization. Med Care. 1985;23(11):1221–1233. , , , .
- Depressive symptoms and health service utilization among the community elderly. J Am Geriatr Soc. 1983;31(7):417–420. , , .
- The influence of socio‐economic status and multimorbidity patterns on healthcare costs: a six‐year follow‐up under a universal healthcare system. Int J Equity Health. 2013;12(1):69. , .
- Association between the level of housing instability, economic standing and health care access: a meta‐regression. J Health Care Poor Underserved. 2008;19(4):1212–1228. , , .
- High health care utilization and costs associated with lower socio‐economic status: results from a linked dataset. Can J Public Health. 2009;100(3):180–183. , , , .
- An analysis of the use of Medicare services by the continuously enrolled aged. Med Care. 1983;21(6):567–585. , .
© 2013 Society of Hospital Medicine
Ideal Transitions of Care
Across the country, hospitals are rolling out programs to reduce readmissions. These range from patient education around their disease process and medications, improvements in discharge planning, medication reconciliation, outpatient appointments scheduled prior to discharge, and follow‐up phone calls, among others.[1, 2] Several collaboratives such as Project Better Outcomes by Optimizing Safe Transitions[3] and Hospital Medicine Reengineering Network[4] have formed to test, study, and share lessons learned from these inpatient‐based interventions. Because financial penalties thus far have focused on decreases in inpatient reimbursement by the Centers for Medicare and Medicaid Services (CMS), most of the interventions to reduce hospital readmissions have been concentrated in the inpatient domain. It is unclear whether new payment arrangements with CMS or commercial insurers, such as bundled payments and accountable care organizations (ACOs), will pressure primary care physicians (PCPs) to further develop outpatient‐based interventions.
In this article, I provide a PCP's perspective of how inpatient and outpatient providers can partner to create the ideal care transition from hospital to home. Although others have conducted systematic reviews or surveys of interventions to reduce hospital readmissions,[2, 5, 6, 7] I will start from a vision of an ideal transition, and then evaluate evidence supporting each step. I will also highlight areas where new reimbursement codes can help support an idealized transitions‐of‐care program.
ON HOSPITAL ADMISSION
Many PCPs consider the beginning of the care continuum to rest in the primary care practice and relationship. Over time, PCPs develop relationships with their patients, understanding the patients' values toward health and healthcare, learning their social support system and home environment, and documenting a clinical course of chronic diseases, including which therapies have and have not worked well. From a PCP perspective, it seems natural to be involved in care at the point of hospital admission. Some emergency departments (EDs) and hospital admissions offices have automated systems to email or fax PCPs admission notifications. Others rely on providers to make this connection.
Ideally, PCP communication would occur early in the hospitalization, especially for medically or socially complex patients, for 3 main reasons: (1) The PCP can offer insights on goals of care or therapies attempted in the past that may reduce unnecessary procedures, decrease length of stay, and improve patient satisfaction; (2) Reconciling medications that the patient should be taking and the list the patient reports may highlight noncompliance and trigger education around medication compliance prior to discharge; (3) Early PCP involvement may improve discharge planning efficiency, whereby the inpatient and outpatient teams agree on medical and social issues to be addressed for a safe discharge. Although there are no published studies that show communication early in the hospitalization impacts clinical outcomes, a national survey of hospitalists highlighted concerns about poor information exchange, particularly around medical history and outpatient medications, at the time of admission.[8]
As new financial models push healthcare providers to manage a population of patients under a global budget, inpatient and outpatient providers will need to communicate and collaborate at a level that is new for most institutions and providers.[9] Because early markers of success are based on financial savings, this means further reducing length of stay and transferring more care to the outpatient arena, tapping into community and home care resources. Involving PCPs early in the admission may help inpatient providers meet these goals.
DURING HOSPITALIZATION
Several publications from hospitalists have evaluated inpatient‐based interventions to reduce readmissions and improve care transitions.[10, 11, 12] Table 1 summarizes 6 steps that can improve communication and collaboration.
|
1. Involve the PCP in discharge planning early in the hospitalization.[1] |
2. Notify the PCP on hospital discharge.[44] |
3. Ensure the discharge summary is available at the time of discharge.[44] Several elements should be included in all discharge summaries:[45] |
a. Home services ordered, home agency, timing of initiation of services. |
b. Medication changes.[21] |
c. Status of active problems at time of discharge.[11] |
d. Follow‐up appointments, especially specialty follow‐up. |
e. Tests pending at discharge or follow‐up required after discharge (eg, follow‐up CT scan in 6 months for incidental lung nodule).[11, 46, 47] |
f. Equipment ordered. |
4. Schedule follow‐up appointment with appropriate outpatient provider by discharge.[11, 29] |
5. Ensure new prescriptions or changes to prescriptions are available at patient's pharmacy and any needed insurance preauthorization has been approved. |
6. Educate patient about disease process, medication adherence, lifestyle changes, and symptoms to monitor for after discharge.[22, 48, 49] |
AFTER HOSPITAL DISCHARGE
Immediately after hospital discharge, there are 7 steps that PCPs and their clinic staff can follow to support a safe transition from hospital to home. The literature supports several individual steps, but not the full package. I am proposing that primary care clinics adopt all 7 steps in an ideal transitions‐of‐care program.
Step 1: Telephone Call Within 72 Hours of Discharge
Many hospitals ask nurses or customer service staff to call patients immediately after hospital discharge. Call content ranges from reviewing discharge instructions and symptoms to satisfaction with hospital care. Even though a 2006 Cochrane review did not find a positive impact of hospital‐based postdischarge phone calls on readmission rates,[13] recent studies among select populations found small but significant reductions.[14, 15] Others have looked at fulfilling this role in the outpatient setting.[16, 17] A recent systematic review of primary care clinic‐based postdischarge phone calls showed no impact on readmission rates, but only 3 studies were included.[18] Health plan‐initiated telephone calls to plan members after hospital discharge reported a 22% reduction in readmissions.[19, 20] Because there is no standardization in telephone call content, reviews of inpatient‐based and clinic‐based interventions cited methodological challenges in drawing conclusions about impact.
Although education around disease process, lifestyle changes, and medication adherence can be effectively provided by staff from the hospital, clinic, or health plan, the outpatient clinic should assume primary responsibility for some components of the postdischarge call. First, if a patient does not have a follow‐up appointment after discharge, the clinic nurse can schedule the appointment directly. Second, medication discrepancies after hospital discharge pose safety risks.[21, 22, 23] Although inpatient nurses may review discharge medications, it is the primary care nurse who can reconcile the discharge medication list with the prehospitalization medication list and identify discrepancies. The outpatient nurse has easier access to the PCP to address discrepancies. Third, the primary care nurse can provide education around red‐flag symptoms for which to call the clinic and information on after‐hours clinic access, an area that patients have specifically requested as standard after discharge.[24] If the patient reports new symptoms, the clinic nurse has easy access to the PCP for management advice, as well as the clinic schedule for an urgent appointment. Having the primary care practice house posthospitalization phone calls allows for more efficient troubleshooting of postdischarge issues.
In January 2013, CMS introduced new codes for primary care‐based care coordination after hospitalization. Current procedural terminology (CPT) codes 99495 and 99496 can be used by PCPs who complete 2 steps: (1) document discussion with a patient or caregiver about care transitions within 2 days of discharge, and (2) have a face‐to‐face visit with the patient within 2 weeks or 1 week, respectively.[25] Reimbursement for these codes is substantial3.96 work relative value units (RVUs) for 99495 and 5.81 work RVUs for 99496considerably more than a level IV visit for complex follow‐up care (2.43 work RVUs). Primary care practices may find that reimbursement for these care coordination codes helps cover additional costs of nurses, case managers, or social workers assisting with posthospital care. The financial impact on primary care practices may increase if commercial insurers accept these CPT codes and reimburse at levels comparable to the CMS.
CMS approved reimbursement for posthospitalization phone calls despite mixed evidence on the impact of the intervention, presumably because it is perceived that early follow‐up may lead to benefits that cannot be easily captured in research studies, and simply represents good patient care. Two challenges in showing an impact of these phone calls are lack of standardization and small sample size. However, implementation of the care‐coordination CPT codes will require more standardization and potentially a much larger number of patients who receive posthospitalization phone calls. This allows for a much more robust evaluation of the intervention.
Step 2: Follow‐up Appointment With PCP or Most Appropriate Continuity Provider
Early follow‐up with an appropriate outpatient provider has been associated with reduced hospital readmissions for patients with congestive heart failure, chronic obstructive pulmonary disease, and psychiatric illnesses,[26, 27, 28, 29] but this finding has not been consistent across all patient populations.[5, 30] It is not well understood if the follow‐up appointment needs to be within a specific time frame, especially if the patient is already being touched once by the system through the posthospitalization call. General consensus falls within 7 days for patients at moderate to high risk for readmissions.[31, 32] Regardless of risk, follow‐up visits must occur within 2 weeks of discharge to claim the CMS reimbursement for posthospitalization care coordination, and higher reimbursement is offered if it occurs within 1 week.
Step 3: Care Coordination
A nurse, social worker, or case manager partnering with the PCP on care coordination may improve the patient experience and outcomes.[17, 24] Although the inpatient social worker or case manager may have helped address some housing, financial, home care, and durable medical equipment needs, often these issues are not completely resolved at discharge. There should be a seamless handoff between inpatient and outpatient care coordinators.
Although some primary care practices include social workers, case managers, or health coaches, many have general clinic nurses functioning in these roles. One way to help fund these roles is through the care coordination CPT codes as previously described. Another consideration, as the financial model for funding care across the care continuum changes, is to have inpatient social workers and case managers work jointly with inpatient and outpatient providers, following patients to the outpatient setting until their social needs are met. This arrangement is more feasible for integrated delivery systems or primary care clinics with contractual agreements with local hospitals, an emerging trend in markets across the United States.[33] Other resources for care coordination include health plan case managers and local community nonprofits. In 2011, CMS launched the Community‐Based Care Transitions Program (CCTP), which will award up to $500 million in funding over 5 years to community‐based organizations to assist Medicare patients with care transitions.[34]
One way of operationalizing care coordination, especially in primary care clinics that do not have an embedded social worker or case manager, is to offer a team‐based appointment in conjunction with the physician postdischarge visit. A healthcare team member (nurse, experienced medical assistant, pharmacist) reviews hospital discharge records, educates the patient about the reasons for hospitalization and how to prevent readmission, performs detailed review of medications, follows up on any pending test results, reviews home care orders or durable medical equipment orders, and identifies any psychosocial issues that need to be addressed. All findings are documented in the patient chart and available for review at the beginning of the physician visit. With the team previsit in place, the physician can focus on the medical problems.
Step 4: Repeat Process Above Until Active Issues Are Stabilized
For some patients, steps 1 through 4 may need to be repeated until active medical and psychosocial issues are stabilized. Creating clinic infrastructure to support patients who may need to return weekly for titration of medications or monitoring of lab values until they normalize can prevent unnecessary ED visits. Patients with psychosocial issues will likely need longitudinal support, as these issues often take months to resolve.
Step 5: Create Access in Clinic for Patients With New Symptoms
Even after the first posthospitalization visit, patients may need to return to their PCP because of new symptoms or for active monitoring. In many parts of the country, PCP access is limited.[33] To meet patient demand for timely appointments, many primary care practices have piloted advanced access scheduling, reserving the majority of appointments for same‐day patient requests. However, evaluations show that the same‐day appointment goals of advanced access are difficult to achieve for most practices.[35] Despite challenges to same‐day access for the general clinic population, it is critical to create access for patients recently hospitalized, as many are at high risk for an ED visit or another hospital admission.
Step 6: Know Your Numbers
A basic tenet of quality improvement is measuring baseline performance and performance at intermediate time points during an intervention.[36] A recent Cochrane review found that feeding back performance to physicians can lead to potentially important improvements in practice.[37] In an Institute for Healthcare Improvement how‐to guide for improving care transitions, measuring readmission rates is 1 step in their Model for Improvement.[32] However, few primary care clinics are actively monitoring their readmission rates. One basic challenge is data availability. Primary care clinics affiliated with a hospital can obtain discharge and readmissions data from the hospital, but patients may also be hospitalized at other facilities. Insurers would be the best source of hospital discharge data, and some payors supply PCPs with risk‐adjusted performance metrics.[38, 39] As ACOs mature, primary care clinics can partner with payors to obtain data and begin trending their hospital discharge and readmission rates. In the interim, trending readmission rates at a single affiliated institution and filtering by service, discharge diagnosis, or payor may reveal areas for intervention.
Step 7: Know Your Readmitted Patients
Similar to knowing the primary care clinic's overall discharge and readmission numbers, it is also important to know the population of frequently readmitted patients. Even though some PCPs may be able to recall these patients by memory, it is important to review these patients' charts and identify preventable factors related to readmission, especially system‐related factors. Conducting reviews can be time intensive and add new demands for busy PCPs. However, many clinics already conduct morbidity and mortality conferences and case reviews as part of improving patient satisfaction, service, and outcomes. Case reviews of frequently admitted patients can fall under these established activities.
IMPLICATIONS
In this vision of the ideal care transition, I am suggesting a shift in culture from a predominantly hospital‐based program to a program that spans the care continuum and requires active participation and ownership from the PCP's team. It will require inpatient and outpatient providers to communicate early and frequently during the hospitalization, sharing patient information efficiently and working collaboratively as part of a larger team to meet the medical and psychosocial needs of the patient. This concept is not new, but has not been supported financially from payors.[1, 9, 40] Most PCPs operate on margins that cannot support additional PCP time to coordinate care for patients or staff to assist (although many PCPs believe this is the role of the primary care medical home).[33] Some payors agree that stipends to support infrastructure change are needed to improve patient outcomes.[17, 38, 39]
Even though every envisioned step does not require additional funding, new payment arrangements under ACOs and bundled payments may offer opportunities for PCPs to assume a larger role in care transitions and secure funding to pay for interventions. However, primary care practices must be positioned to negotiate favorable global payment agreements, be willing to assume risks associated with global payments, and prioritize management of medically and socially complex patients who are at risk for preventable ED visits and hospitalizations. PCPs who are not participating in ACOs or bundled payments, or those who are risk adverse, may be able to finance pieces of this vision with the new care coordination CPT codes supported by Medicare (and possibly commercial payors in the future). They may also partner with community groups participating in CCTP for additional support. Others focus on the long‐term benefits of ACO‐like structures rather than the short‐term investments needed.[41]
Are all 14 steps proposed above essential? Without doubt, this vision will be difficult to fully operationalize and requires coordination and support from many distinct groups. Should all patients be offered a basic package of interventions, reserving the full package for those who are identified as highest risk for poor outcomes after hospital discharge? There is already some support around specialized interventions for patients at high risk for readmissions,[32, 41] and risk prediction models have been introduced to identify these individuals.[42] Or should we approach this as a menu of interventions from which to choose, tailoring interventions to individual patient needs? These questions should be tested, as our experience in coordinating care across the continuum matures. With over 100 ACOs formed in Medicare alone[43] and many more with commercial insurers, our understanding in this area will grow in the next 5 years.
CONCLUSIONS
As cost containment measures in healthcare target preventable readmissions, hospitals and primary care physicians are increasingly encouraged to improve transitions along the care continuum. In this article, I offer 1 PCP's vision of the ideal transitions‐of‐care program from hospital to home. This article focuses on steps that can be taken by PCPs and their clinic staff; it does not address the role of outpatient specialists, home care agencies, or community support groups in care transitions. Operationalizing this vision requires commitment from the hospital and clinic leadership, as well as buy‐in from front‐line providers. More research is required to understand the marginal impact of each component of this vision, as well as the comprehensive package of interventions proposed, on patient outcomes. New financial models with payors and hospitals may make it easier for primary care clinics to test this vision. Current financial incentives are likely still inadequate to fully align care along the continuum, but they offer some support for more PCPs to take an active role. The time has come to shift our traditional view of transitions of care from a hospital‐centric set of interventions toward one that spans the entire care continuum and includes primary care physicians and their clinic staff as key partners.
Acknowledgments
The author thanks Jeffrey Fujimoto for his assistance with the literature review.
Disclosures: Ning Tang, MD, is supported by a University of California, Center for Health Quality and Innovation grant. Dr. Tang has no financial conflicts of interests.
- Reducing hospital readmissions: lessons from top‐performing hospitals. The Commonwealth Fund Synthesis Report. April 2011. Available at: http://www.commonwealthfund.org/Publications/Case‐Studies/2011/Apr/Reducing‐Hospital‐Readmissions.aspx. Accessed January 30, 2013. , , .
- Effective Interventions to Reduce Rehospitalizations: A Survey of the Published Evidence. Cambridge, MA: Institute for Healthcare Improvement; 2009. , .
- Society of Hospital Medicine. Project BOOST. Available at: www.hospitalmedicine.org/BOOST/. Accessed January 30, 2013.
- American Association of Medical Colleges. HOMERUN Executive Summary. Available at: https://members.aamc.org/eweb/upload/HOMERUN%20summary%202012.pdf. Accessed January 30, 2013.
- Interventions to reduce 30‐day rehospitalization: a systematic review. Ann Intern Med. 2011;155:520–528. , , , , .
- Contemporary evidence about hospital strategies for reducing 30‐day readmissions. J Am Coll Cardiol. 2012;60:607–614. , , , et al.
- Improving patient handovers from hospital to primary care: a systematic review. Ann Intern Med. 2012;157:417–428. , , , et al.
- Hospitalists and care transitions: the divorce of inpatient and outpatient care. Health Aff. 2008;27:1315–1327. , , , .
- Recasting readmissions by placing the hospital role in community context. JAMA. 2013;309:351–352. , , .
- Promoting effective transitions of care at hospital discharge: a review of key issues for hospitalists. J Hosp Med. 2007;2:314–323. , , , .
- Transition of care for hospitalized elderly patients—development of a discharge checklist for hospitalists. J Hosp Med. 2006;1:354–360. , , , et al.
- Transitioning the patient with acute coronary syndrome from inpatient to primary care. J Hosp Med. 2010;5(suppl):S8–S14. .
- Telephone follow‐up, initiated by a hospital‐based health professional, for postdischarge problems in patients discharged from hospital to home. Cochrane Database Syst Rev. 2006;(4):CD004510. , .
- Post‐discharge intervention in vulnerable, chronically ill patients. J Hosp Med. 2012;7:124–130. , , , .
- Low‐cost transitional care with nurse managers making mostly phone contact with patients cut rehospitalization at a VA hospital. Health Aff. 2012;31:2659–2668. , , , et al.
- Redefining and redesigning hospital discharge to enhance patient care: a randomized control study. J Gen Intern Med. 2008;23:1228–1233. , , , .
- How Geisinger's advanced medical home model argues the case for rapid‐cycle innovation. Health Aff. 2010;29:2047–2053. . , , , et al.
- Telephone follow‐up as a primary care intervention for postdischarge outcomes improvement: a systematic review. Am J Med. 2012;125:915–921. , , .
- The impact of postdischarge telephonic follow‐up on hospital readmissions. Popul Health Manag. 2011;14:27–32. , , , , .
- Prioritized post‐discharge telephonic outreach reduces hospital readmissions for select high‐risk patients. Am J Manag Care. 2012;18:838–844. , , , , .
- Association of ICU or hospital admission with unintentional discontinuation of medications for chronic diseases. JAMA. 2011;306:840–847. , , , et al.
- Effect of a pharmacist intervention on clinically important medication errors after hospital discharge. Ann Intern Med. 2012;157:1–10. , , , et al.
- Relationship of health literacy to intentional and unintentional non‐adherence of hospital discharge medications. J Gen Intern Med. 2012;27:173–178. , , , , , .
- Patient experiences of transitioning from hospital to home: an ethnographic quality improvement project. J Hosp Med. 2012;7:382–387. , , , , .
- Medicare finalizes physician pay for new care coordination benefit. American Medical News. November 12, 2012. Available at: http://www.ama‐assn.org/amednews/2012/11/12/gvl11112.htm. Accessed February 8, 2013. .
- Outpatient follow‐up visit and 30‐day emergency department visit and readmission in patients hospitalized for chronic obstructive pulmonary disease. Arch Intern Med. 2010;170:1664–1670. , , , , .
- Post‐hospitalization transitions: examining the effects of timing of primary care provider follow‐up. J Hosp Med. 2010;5:392–397. , , .
- Relationship between early physician follow‐up and 30‐day readmission among Medicare beneficiaries hospitalized for heart failure. JAMA. 2010;303:1716–1722. , , , et al.
- Effects of discharge planning and compliance with outpatient appointments on readmission rates. Psychiatr Serv. 2000;51:885–889. , , .
- Do timely outpatient follow‐up visits decrease hospital readmission rates? Am J Med Qual. 2012;27:11–15. , , , , .
- Project BOOST. Tool for addressing risk: a geriatric evaluation for transitions. Available at: http://www.hospitalmedicine.org/ResourceRoomRedesign/RR_CareTransitions/PDFs/TARGET.pdf. Accessed February 3, 2013.
- How‐to Guide: Improving Transitions from the Hospital to Community Settings to Reduce Avoidable Rehospitalizations. Cambridge, MA: Institute for Healthcare Improvement; 2012. , , , , .
- Primary care: current problems and proposed solutions. Health Aff. 2010;29:799–805. , .
- Center for Medicare and Medicaid Innovation. Community‐based Care Transitions Program. Available at: http://innovation.cms.gov/initiatives/CCTP/#collapse‐tableDetails. Accessed February 8, 2013.
- Advanced access scheduling outcomes: a systematic review. Arch Intern Med. 2011;171:1150–1159. , , .
- The Improvement Guide: A Practical Approach to Enhancing Organizational Performance. 2nd ed. San Francisco, CA: Jossey‐Bass Publishers; 2009. , , , .
- Audit and feedback: effects on professional practice and healthcare outcomes. Cochrane Database Syst Rev. 2012;(6):CD000259. , , , et al.
- Agency for Healthcare Research and Quality. Policy Innovation Profile. Insurer provides financial incentives, infrastructure, and other support to stimulate provider participation in quality improvement collaborations. June 6, 2012. Available at: http://www.innovations.ahrq.gov/content.aspx?id=3641. Accessed February 8, 2013.
- Private‐payer innovation in Massachusetts: The “Alternative Quality Contract.” Health Aff. 2011;30:51–61. , , , .
- AMA Expert Panel on Care Transitions. There and home again, safely: five responsibilities of ambulatory practices in high quality care transitions. American Medical Association; Chicago, IL; 2013. Available at: www.ama‐assn.org/go/patientsafety. Accessed February 22, 2013. , ;
- Agency for Healthcare Research and Quality. Policy Innovation Profile. Medical center establishes infrastructure to manage care under capitated contracts, leading to better chronic care management and lower utilization and costs. October 3, 2012. Available at: http://www.innovations.ahrq.gov/content.aspx?id=3651. Accessed February 8, 2013.
- Risk prediction models for hospital readmission: a systematic review. JAMA. 2011;306:1688–1698. , , , et al.
- Centers for Medicare and Medicaid Services. More doctors, hospitals partner to coordinate care for people with Medicare: providers form 106 new accountable care organizations. Press release January 10, 2013. Available at: http://www.cms.gov/apps/media/press/release.asp?Counter=4501297:831–841.
- Transitions of care consensus policy statement American College of Physicians‐Society of General Internal Medicine‐Society of Hospital Medicine‐American Geriatrics Society‐American College of Emergency Physicians‐Society of Academic Emergency Medicine. J Gen Intern Med. 2009;24:971–976. , , , et al.
- Patient safety concerns arising from test results that return after hospital discharge. Ann Intern Med. 2005;143(2):121–128. , , , et al.
- Tying up loose ends: discharging patients with unresolved medical issues. Arch Intern Med. 2007;167:1305–1311. , , .
- Perceptions of readmitted patients on the transition from hospital to home. J Hosp Med. 2012;7:709–712. , , , , , .
- Patients' understanding of their treatment plans and diagnosis at discharge. Mayo Clin Proc. 2005;80:991–994. , .
Across the country, hospitals are rolling out programs to reduce readmissions. These range from patient education around their disease process and medications, improvements in discharge planning, medication reconciliation, outpatient appointments scheduled prior to discharge, and follow‐up phone calls, among others.[1, 2] Several collaboratives such as Project Better Outcomes by Optimizing Safe Transitions[3] and Hospital Medicine Reengineering Network[4] have formed to test, study, and share lessons learned from these inpatient‐based interventions. Because financial penalties thus far have focused on decreases in inpatient reimbursement by the Centers for Medicare and Medicaid Services (CMS), most of the interventions to reduce hospital readmissions have been concentrated in the inpatient domain. It is unclear whether new payment arrangements with CMS or commercial insurers, such as bundled payments and accountable care organizations (ACOs), will pressure primary care physicians (PCPs) to further develop outpatient‐based interventions.
In this article, I provide a PCP's perspective of how inpatient and outpatient providers can partner to create the ideal care transition from hospital to home. Although others have conducted systematic reviews or surveys of interventions to reduce hospital readmissions,[2, 5, 6, 7] I will start from a vision of an ideal transition, and then evaluate evidence supporting each step. I will also highlight areas where new reimbursement codes can help support an idealized transitions‐of‐care program.
ON HOSPITAL ADMISSION
Many PCPs consider the beginning of the care continuum to rest in the primary care practice and relationship. Over time, PCPs develop relationships with their patients, understanding the patients' values toward health and healthcare, learning their social support system and home environment, and documenting a clinical course of chronic diseases, including which therapies have and have not worked well. From a PCP perspective, it seems natural to be involved in care at the point of hospital admission. Some emergency departments (EDs) and hospital admissions offices have automated systems to email or fax PCPs admission notifications. Others rely on providers to make this connection.
Ideally, PCP communication would occur early in the hospitalization, especially for medically or socially complex patients, for 3 main reasons: (1) The PCP can offer insights on goals of care or therapies attempted in the past that may reduce unnecessary procedures, decrease length of stay, and improve patient satisfaction; (2) Reconciling medications that the patient should be taking and the list the patient reports may highlight noncompliance and trigger education around medication compliance prior to discharge; (3) Early PCP involvement may improve discharge planning efficiency, whereby the inpatient and outpatient teams agree on medical and social issues to be addressed for a safe discharge. Although there are no published studies that show communication early in the hospitalization impacts clinical outcomes, a national survey of hospitalists highlighted concerns about poor information exchange, particularly around medical history and outpatient medications, at the time of admission.[8]
As new financial models push healthcare providers to manage a population of patients under a global budget, inpatient and outpatient providers will need to communicate and collaborate at a level that is new for most institutions and providers.[9] Because early markers of success are based on financial savings, this means further reducing length of stay and transferring more care to the outpatient arena, tapping into community and home care resources. Involving PCPs early in the admission may help inpatient providers meet these goals.
DURING HOSPITALIZATION
Several publications from hospitalists have evaluated inpatient‐based interventions to reduce readmissions and improve care transitions.[10, 11, 12] Table 1 summarizes 6 steps that can improve communication and collaboration.
|
1. Involve the PCP in discharge planning early in the hospitalization.[1] |
2. Notify the PCP on hospital discharge.[44] |
3. Ensure the discharge summary is available at the time of discharge.[44] Several elements should be included in all discharge summaries:[45] |
a. Home services ordered, home agency, timing of initiation of services. |
b. Medication changes.[21] |
c. Status of active problems at time of discharge.[11] |
d. Follow‐up appointments, especially specialty follow‐up. |
e. Tests pending at discharge or follow‐up required after discharge (eg, follow‐up CT scan in 6 months for incidental lung nodule).[11, 46, 47] |
f. Equipment ordered. |
4. Schedule follow‐up appointment with appropriate outpatient provider by discharge.[11, 29] |
5. Ensure new prescriptions or changes to prescriptions are available at patient's pharmacy and any needed insurance preauthorization has been approved. |
6. Educate patient about disease process, medication adherence, lifestyle changes, and symptoms to monitor for after discharge.[22, 48, 49] |
AFTER HOSPITAL DISCHARGE
Immediately after hospital discharge, there are 7 steps that PCPs and their clinic staff can follow to support a safe transition from hospital to home. The literature supports several individual steps, but not the full package. I am proposing that primary care clinics adopt all 7 steps in an ideal transitions‐of‐care program.
Step 1: Telephone Call Within 72 Hours of Discharge
Many hospitals ask nurses or customer service staff to call patients immediately after hospital discharge. Call content ranges from reviewing discharge instructions and symptoms to satisfaction with hospital care. Even though a 2006 Cochrane review did not find a positive impact of hospital‐based postdischarge phone calls on readmission rates,[13] recent studies among select populations found small but significant reductions.[14, 15] Others have looked at fulfilling this role in the outpatient setting.[16, 17] A recent systematic review of primary care clinic‐based postdischarge phone calls showed no impact on readmission rates, but only 3 studies were included.[18] Health plan‐initiated telephone calls to plan members after hospital discharge reported a 22% reduction in readmissions.[19, 20] Because there is no standardization in telephone call content, reviews of inpatient‐based and clinic‐based interventions cited methodological challenges in drawing conclusions about impact.
Although education around disease process, lifestyle changes, and medication adherence can be effectively provided by staff from the hospital, clinic, or health plan, the outpatient clinic should assume primary responsibility for some components of the postdischarge call. First, if a patient does not have a follow‐up appointment after discharge, the clinic nurse can schedule the appointment directly. Second, medication discrepancies after hospital discharge pose safety risks.[21, 22, 23] Although inpatient nurses may review discharge medications, it is the primary care nurse who can reconcile the discharge medication list with the prehospitalization medication list and identify discrepancies. The outpatient nurse has easier access to the PCP to address discrepancies. Third, the primary care nurse can provide education around red‐flag symptoms for which to call the clinic and information on after‐hours clinic access, an area that patients have specifically requested as standard after discharge.[24] If the patient reports new symptoms, the clinic nurse has easy access to the PCP for management advice, as well as the clinic schedule for an urgent appointment. Having the primary care practice house posthospitalization phone calls allows for more efficient troubleshooting of postdischarge issues.
In January 2013, CMS introduced new codes for primary care‐based care coordination after hospitalization. Current procedural terminology (CPT) codes 99495 and 99496 can be used by PCPs who complete 2 steps: (1) document discussion with a patient or caregiver about care transitions within 2 days of discharge, and (2) have a face‐to‐face visit with the patient within 2 weeks or 1 week, respectively.[25] Reimbursement for these codes is substantial3.96 work relative value units (RVUs) for 99495 and 5.81 work RVUs for 99496considerably more than a level IV visit for complex follow‐up care (2.43 work RVUs). Primary care practices may find that reimbursement for these care coordination codes helps cover additional costs of nurses, case managers, or social workers assisting with posthospital care. The financial impact on primary care practices may increase if commercial insurers accept these CPT codes and reimburse at levels comparable to the CMS.
CMS approved reimbursement for posthospitalization phone calls despite mixed evidence on the impact of the intervention, presumably because it is perceived that early follow‐up may lead to benefits that cannot be easily captured in research studies, and simply represents good patient care. Two challenges in showing an impact of these phone calls are lack of standardization and small sample size. However, implementation of the care‐coordination CPT codes will require more standardization and potentially a much larger number of patients who receive posthospitalization phone calls. This allows for a much more robust evaluation of the intervention.
Step 2: Follow‐up Appointment With PCP or Most Appropriate Continuity Provider
Early follow‐up with an appropriate outpatient provider has been associated with reduced hospital readmissions for patients with congestive heart failure, chronic obstructive pulmonary disease, and psychiatric illnesses,[26, 27, 28, 29] but this finding has not been consistent across all patient populations.[5, 30] It is not well understood if the follow‐up appointment needs to be within a specific time frame, especially if the patient is already being touched once by the system through the posthospitalization call. General consensus falls within 7 days for patients at moderate to high risk for readmissions.[31, 32] Regardless of risk, follow‐up visits must occur within 2 weeks of discharge to claim the CMS reimbursement for posthospitalization care coordination, and higher reimbursement is offered if it occurs within 1 week.
Step 3: Care Coordination
A nurse, social worker, or case manager partnering with the PCP on care coordination may improve the patient experience and outcomes.[17, 24] Although the inpatient social worker or case manager may have helped address some housing, financial, home care, and durable medical equipment needs, often these issues are not completely resolved at discharge. There should be a seamless handoff between inpatient and outpatient care coordinators.
Although some primary care practices include social workers, case managers, or health coaches, many have general clinic nurses functioning in these roles. One way to help fund these roles is through the care coordination CPT codes as previously described. Another consideration, as the financial model for funding care across the care continuum changes, is to have inpatient social workers and case managers work jointly with inpatient and outpatient providers, following patients to the outpatient setting until their social needs are met. This arrangement is more feasible for integrated delivery systems or primary care clinics with contractual agreements with local hospitals, an emerging trend in markets across the United States.[33] Other resources for care coordination include health plan case managers and local community nonprofits. In 2011, CMS launched the Community‐Based Care Transitions Program (CCTP), which will award up to $500 million in funding over 5 years to community‐based organizations to assist Medicare patients with care transitions.[34]
One way of operationalizing care coordination, especially in primary care clinics that do not have an embedded social worker or case manager, is to offer a team‐based appointment in conjunction with the physician postdischarge visit. A healthcare team member (nurse, experienced medical assistant, pharmacist) reviews hospital discharge records, educates the patient about the reasons for hospitalization and how to prevent readmission, performs detailed review of medications, follows up on any pending test results, reviews home care orders or durable medical equipment orders, and identifies any psychosocial issues that need to be addressed. All findings are documented in the patient chart and available for review at the beginning of the physician visit. With the team previsit in place, the physician can focus on the medical problems.
Step 4: Repeat Process Above Until Active Issues Are Stabilized
For some patients, steps 1 through 4 may need to be repeated until active medical and psychosocial issues are stabilized. Creating clinic infrastructure to support patients who may need to return weekly for titration of medications or monitoring of lab values until they normalize can prevent unnecessary ED visits. Patients with psychosocial issues will likely need longitudinal support, as these issues often take months to resolve.
Step 5: Create Access in Clinic for Patients With New Symptoms
Even after the first posthospitalization visit, patients may need to return to their PCP because of new symptoms or for active monitoring. In many parts of the country, PCP access is limited.[33] To meet patient demand for timely appointments, many primary care practices have piloted advanced access scheduling, reserving the majority of appointments for same‐day patient requests. However, evaluations show that the same‐day appointment goals of advanced access are difficult to achieve for most practices.[35] Despite challenges to same‐day access for the general clinic population, it is critical to create access for patients recently hospitalized, as many are at high risk for an ED visit or another hospital admission.
Step 6: Know Your Numbers
A basic tenet of quality improvement is measuring baseline performance and performance at intermediate time points during an intervention.[36] A recent Cochrane review found that feeding back performance to physicians can lead to potentially important improvements in practice.[37] In an Institute for Healthcare Improvement how‐to guide for improving care transitions, measuring readmission rates is 1 step in their Model for Improvement.[32] However, few primary care clinics are actively monitoring their readmission rates. One basic challenge is data availability. Primary care clinics affiliated with a hospital can obtain discharge and readmissions data from the hospital, but patients may also be hospitalized at other facilities. Insurers would be the best source of hospital discharge data, and some payors supply PCPs with risk‐adjusted performance metrics.[38, 39] As ACOs mature, primary care clinics can partner with payors to obtain data and begin trending their hospital discharge and readmission rates. In the interim, trending readmission rates at a single affiliated institution and filtering by service, discharge diagnosis, or payor may reveal areas for intervention.
Step 7: Know Your Readmitted Patients
Similar to knowing the primary care clinic's overall discharge and readmission numbers, it is also important to know the population of frequently readmitted patients. Even though some PCPs may be able to recall these patients by memory, it is important to review these patients' charts and identify preventable factors related to readmission, especially system‐related factors. Conducting reviews can be time intensive and add new demands for busy PCPs. However, many clinics already conduct morbidity and mortality conferences and case reviews as part of improving patient satisfaction, service, and outcomes. Case reviews of frequently admitted patients can fall under these established activities.
IMPLICATIONS
In this vision of the ideal care transition, I am suggesting a shift in culture from a predominantly hospital‐based program to a program that spans the care continuum and requires active participation and ownership from the PCP's team. It will require inpatient and outpatient providers to communicate early and frequently during the hospitalization, sharing patient information efficiently and working collaboratively as part of a larger team to meet the medical and psychosocial needs of the patient. This concept is not new, but has not been supported financially from payors.[1, 9, 40] Most PCPs operate on margins that cannot support additional PCP time to coordinate care for patients or staff to assist (although many PCPs believe this is the role of the primary care medical home).[33] Some payors agree that stipends to support infrastructure change are needed to improve patient outcomes.[17, 38, 39]
Even though every envisioned step does not require additional funding, new payment arrangements under ACOs and bundled payments may offer opportunities for PCPs to assume a larger role in care transitions and secure funding to pay for interventions. However, primary care practices must be positioned to negotiate favorable global payment agreements, be willing to assume risks associated with global payments, and prioritize management of medically and socially complex patients who are at risk for preventable ED visits and hospitalizations. PCPs who are not participating in ACOs or bundled payments, or those who are risk adverse, may be able to finance pieces of this vision with the new care coordination CPT codes supported by Medicare (and possibly commercial payors in the future). They may also partner with community groups participating in CCTP for additional support. Others focus on the long‐term benefits of ACO‐like structures rather than the short‐term investments needed.[41]
Are all 14 steps proposed above essential? Without doubt, this vision will be difficult to fully operationalize and requires coordination and support from many distinct groups. Should all patients be offered a basic package of interventions, reserving the full package for those who are identified as highest risk for poor outcomes after hospital discharge? There is already some support around specialized interventions for patients at high risk for readmissions,[32, 41] and risk prediction models have been introduced to identify these individuals.[42] Or should we approach this as a menu of interventions from which to choose, tailoring interventions to individual patient needs? These questions should be tested, as our experience in coordinating care across the continuum matures. With over 100 ACOs formed in Medicare alone[43] and many more with commercial insurers, our understanding in this area will grow in the next 5 years.
CONCLUSIONS
As cost containment measures in healthcare target preventable readmissions, hospitals and primary care physicians are increasingly encouraged to improve transitions along the care continuum. In this article, I offer 1 PCP's vision of the ideal transitions‐of‐care program from hospital to home. This article focuses on steps that can be taken by PCPs and their clinic staff; it does not address the role of outpatient specialists, home care agencies, or community support groups in care transitions. Operationalizing this vision requires commitment from the hospital and clinic leadership, as well as buy‐in from front‐line providers. More research is required to understand the marginal impact of each component of this vision, as well as the comprehensive package of interventions proposed, on patient outcomes. New financial models with payors and hospitals may make it easier for primary care clinics to test this vision. Current financial incentives are likely still inadequate to fully align care along the continuum, but they offer some support for more PCPs to take an active role. The time has come to shift our traditional view of transitions of care from a hospital‐centric set of interventions toward one that spans the entire care continuum and includes primary care physicians and their clinic staff as key partners.
Acknowledgments
The author thanks Jeffrey Fujimoto for his assistance with the literature review.
Disclosures: Ning Tang, MD, is supported by a University of California, Center for Health Quality and Innovation grant. Dr. Tang has no financial conflicts of interests.
Across the country, hospitals are rolling out programs to reduce readmissions. These range from patient education around their disease process and medications, improvements in discharge planning, medication reconciliation, outpatient appointments scheduled prior to discharge, and follow‐up phone calls, among others.[1, 2] Several collaboratives such as Project Better Outcomes by Optimizing Safe Transitions[3] and Hospital Medicine Reengineering Network[4] have formed to test, study, and share lessons learned from these inpatient‐based interventions. Because financial penalties thus far have focused on decreases in inpatient reimbursement by the Centers for Medicare and Medicaid Services (CMS), most of the interventions to reduce hospital readmissions have been concentrated in the inpatient domain. It is unclear whether new payment arrangements with CMS or commercial insurers, such as bundled payments and accountable care organizations (ACOs), will pressure primary care physicians (PCPs) to further develop outpatient‐based interventions.
In this article, I provide a PCP's perspective of how inpatient and outpatient providers can partner to create the ideal care transition from hospital to home. Although others have conducted systematic reviews or surveys of interventions to reduce hospital readmissions,[2, 5, 6, 7] I will start from a vision of an ideal transition, and then evaluate evidence supporting each step. I will also highlight areas where new reimbursement codes can help support an idealized transitions‐of‐care program.
ON HOSPITAL ADMISSION
Many PCPs consider the beginning of the care continuum to rest in the primary care practice and relationship. Over time, PCPs develop relationships with their patients, understanding the patients' values toward health and healthcare, learning their social support system and home environment, and documenting a clinical course of chronic diseases, including which therapies have and have not worked well. From a PCP perspective, it seems natural to be involved in care at the point of hospital admission. Some emergency departments (EDs) and hospital admissions offices have automated systems to email or fax PCPs admission notifications. Others rely on providers to make this connection.
Ideally, PCP communication would occur early in the hospitalization, especially for medically or socially complex patients, for 3 main reasons: (1) The PCP can offer insights on goals of care or therapies attempted in the past that may reduce unnecessary procedures, decrease length of stay, and improve patient satisfaction; (2) Reconciling medications that the patient should be taking and the list the patient reports may highlight noncompliance and trigger education around medication compliance prior to discharge; (3) Early PCP involvement may improve discharge planning efficiency, whereby the inpatient and outpatient teams agree on medical and social issues to be addressed for a safe discharge. Although there are no published studies that show communication early in the hospitalization impacts clinical outcomes, a national survey of hospitalists highlighted concerns about poor information exchange, particularly around medical history and outpatient medications, at the time of admission.[8]
As new financial models push healthcare providers to manage a population of patients under a global budget, inpatient and outpatient providers will need to communicate and collaborate at a level that is new for most institutions and providers.[9] Because early markers of success are based on financial savings, this means further reducing length of stay and transferring more care to the outpatient arena, tapping into community and home care resources. Involving PCPs early in the admission may help inpatient providers meet these goals.
DURING HOSPITALIZATION
Several publications from hospitalists have evaluated inpatient‐based interventions to reduce readmissions and improve care transitions.[10, 11, 12] Table 1 summarizes 6 steps that can improve communication and collaboration.
|
1. Involve the PCP in discharge planning early in the hospitalization.[1] |
2. Notify the PCP on hospital discharge.[44] |
3. Ensure the discharge summary is available at the time of discharge.[44] Several elements should be included in all discharge summaries:[45] |
a. Home services ordered, home agency, timing of initiation of services. |
b. Medication changes.[21] |
c. Status of active problems at time of discharge.[11] |
d. Follow‐up appointments, especially specialty follow‐up. |
e. Tests pending at discharge or follow‐up required after discharge (eg, follow‐up CT scan in 6 months for incidental lung nodule).[11, 46, 47] |
f. Equipment ordered. |
4. Schedule follow‐up appointment with appropriate outpatient provider by discharge.[11, 29] |
5. Ensure new prescriptions or changes to prescriptions are available at patient's pharmacy and any needed insurance preauthorization has been approved. |
6. Educate patient about disease process, medication adherence, lifestyle changes, and symptoms to monitor for after discharge.[22, 48, 49] |
AFTER HOSPITAL DISCHARGE
Immediately after hospital discharge, there are 7 steps that PCPs and their clinic staff can follow to support a safe transition from hospital to home. The literature supports several individual steps, but not the full package. I am proposing that primary care clinics adopt all 7 steps in an ideal transitions‐of‐care program.
Step 1: Telephone Call Within 72 Hours of Discharge
Many hospitals ask nurses or customer service staff to call patients immediately after hospital discharge. Call content ranges from reviewing discharge instructions and symptoms to satisfaction with hospital care. Even though a 2006 Cochrane review did not find a positive impact of hospital‐based postdischarge phone calls on readmission rates,[13] recent studies among select populations found small but significant reductions.[14, 15] Others have looked at fulfilling this role in the outpatient setting.[16, 17] A recent systematic review of primary care clinic‐based postdischarge phone calls showed no impact on readmission rates, but only 3 studies were included.[18] Health plan‐initiated telephone calls to plan members after hospital discharge reported a 22% reduction in readmissions.[19, 20] Because there is no standardization in telephone call content, reviews of inpatient‐based and clinic‐based interventions cited methodological challenges in drawing conclusions about impact.
Although education around disease process, lifestyle changes, and medication adherence can be effectively provided by staff from the hospital, clinic, or health plan, the outpatient clinic should assume primary responsibility for some components of the postdischarge call. First, if a patient does not have a follow‐up appointment after discharge, the clinic nurse can schedule the appointment directly. Second, medication discrepancies after hospital discharge pose safety risks.[21, 22, 23] Although inpatient nurses may review discharge medications, it is the primary care nurse who can reconcile the discharge medication list with the prehospitalization medication list and identify discrepancies. The outpatient nurse has easier access to the PCP to address discrepancies. Third, the primary care nurse can provide education around red‐flag symptoms for which to call the clinic and information on after‐hours clinic access, an area that patients have specifically requested as standard after discharge.[24] If the patient reports new symptoms, the clinic nurse has easy access to the PCP for management advice, as well as the clinic schedule for an urgent appointment. Having the primary care practice house posthospitalization phone calls allows for more efficient troubleshooting of postdischarge issues.
In January 2013, CMS introduced new codes for primary care‐based care coordination after hospitalization. Current procedural terminology (CPT) codes 99495 and 99496 can be used by PCPs who complete 2 steps: (1) document discussion with a patient or caregiver about care transitions within 2 days of discharge, and (2) have a face‐to‐face visit with the patient within 2 weeks or 1 week, respectively.[25] Reimbursement for these codes is substantial3.96 work relative value units (RVUs) for 99495 and 5.81 work RVUs for 99496considerably more than a level IV visit for complex follow‐up care (2.43 work RVUs). Primary care practices may find that reimbursement for these care coordination codes helps cover additional costs of nurses, case managers, or social workers assisting with posthospital care. The financial impact on primary care practices may increase if commercial insurers accept these CPT codes and reimburse at levels comparable to the CMS.
CMS approved reimbursement for posthospitalization phone calls despite mixed evidence on the impact of the intervention, presumably because it is perceived that early follow‐up may lead to benefits that cannot be easily captured in research studies, and simply represents good patient care. Two challenges in showing an impact of these phone calls are lack of standardization and small sample size. However, implementation of the care‐coordination CPT codes will require more standardization and potentially a much larger number of patients who receive posthospitalization phone calls. This allows for a much more robust evaluation of the intervention.
Step 2: Follow‐up Appointment With PCP or Most Appropriate Continuity Provider
Early follow‐up with an appropriate outpatient provider has been associated with reduced hospital readmissions for patients with congestive heart failure, chronic obstructive pulmonary disease, and psychiatric illnesses,[26, 27, 28, 29] but this finding has not been consistent across all patient populations.[5, 30] It is not well understood if the follow‐up appointment needs to be within a specific time frame, especially if the patient is already being touched once by the system through the posthospitalization call. General consensus falls within 7 days for patients at moderate to high risk for readmissions.[31, 32] Regardless of risk, follow‐up visits must occur within 2 weeks of discharge to claim the CMS reimbursement for posthospitalization care coordination, and higher reimbursement is offered if it occurs within 1 week.
Step 3: Care Coordination
A nurse, social worker, or case manager partnering with the PCP on care coordination may improve the patient experience and outcomes.[17, 24] Although the inpatient social worker or case manager may have helped address some housing, financial, home care, and durable medical equipment needs, often these issues are not completely resolved at discharge. There should be a seamless handoff between inpatient and outpatient care coordinators.
Although some primary care practices include social workers, case managers, or health coaches, many have general clinic nurses functioning in these roles. One way to help fund these roles is through the care coordination CPT codes as previously described. Another consideration, as the financial model for funding care across the care continuum changes, is to have inpatient social workers and case managers work jointly with inpatient and outpatient providers, following patients to the outpatient setting until their social needs are met. This arrangement is more feasible for integrated delivery systems or primary care clinics with contractual agreements with local hospitals, an emerging trend in markets across the United States.[33] Other resources for care coordination include health plan case managers and local community nonprofits. In 2011, CMS launched the Community‐Based Care Transitions Program (CCTP), which will award up to $500 million in funding over 5 years to community‐based organizations to assist Medicare patients with care transitions.[34]
One way of operationalizing care coordination, especially in primary care clinics that do not have an embedded social worker or case manager, is to offer a team‐based appointment in conjunction with the physician postdischarge visit. A healthcare team member (nurse, experienced medical assistant, pharmacist) reviews hospital discharge records, educates the patient about the reasons for hospitalization and how to prevent readmission, performs detailed review of medications, follows up on any pending test results, reviews home care orders or durable medical equipment orders, and identifies any psychosocial issues that need to be addressed. All findings are documented in the patient chart and available for review at the beginning of the physician visit. With the team previsit in place, the physician can focus on the medical problems.
Step 4: Repeat Process Above Until Active Issues Are Stabilized
For some patients, steps 1 through 4 may need to be repeated until active medical and psychosocial issues are stabilized. Creating clinic infrastructure to support patients who may need to return weekly for titration of medications or monitoring of lab values until they normalize can prevent unnecessary ED visits. Patients with psychosocial issues will likely need longitudinal support, as these issues often take months to resolve.
Step 5: Create Access in Clinic for Patients With New Symptoms
Even after the first posthospitalization visit, patients may need to return to their PCP because of new symptoms or for active monitoring. In many parts of the country, PCP access is limited.[33] To meet patient demand for timely appointments, many primary care practices have piloted advanced access scheduling, reserving the majority of appointments for same‐day patient requests. However, evaluations show that the same‐day appointment goals of advanced access are difficult to achieve for most practices.[35] Despite challenges to same‐day access for the general clinic population, it is critical to create access for patients recently hospitalized, as many are at high risk for an ED visit or another hospital admission.
Step 6: Know Your Numbers
A basic tenet of quality improvement is measuring baseline performance and performance at intermediate time points during an intervention.[36] A recent Cochrane review found that feeding back performance to physicians can lead to potentially important improvements in practice.[37] In an Institute for Healthcare Improvement how‐to guide for improving care transitions, measuring readmission rates is 1 step in their Model for Improvement.[32] However, few primary care clinics are actively monitoring their readmission rates. One basic challenge is data availability. Primary care clinics affiliated with a hospital can obtain discharge and readmissions data from the hospital, but patients may also be hospitalized at other facilities. Insurers would be the best source of hospital discharge data, and some payors supply PCPs with risk‐adjusted performance metrics.[38, 39] As ACOs mature, primary care clinics can partner with payors to obtain data and begin trending their hospital discharge and readmission rates. In the interim, trending readmission rates at a single affiliated institution and filtering by service, discharge diagnosis, or payor may reveal areas for intervention.
Step 7: Know Your Readmitted Patients
Similar to knowing the primary care clinic's overall discharge and readmission numbers, it is also important to know the population of frequently readmitted patients. Even though some PCPs may be able to recall these patients by memory, it is important to review these patients' charts and identify preventable factors related to readmission, especially system‐related factors. Conducting reviews can be time intensive and add new demands for busy PCPs. However, many clinics already conduct morbidity and mortality conferences and case reviews as part of improving patient satisfaction, service, and outcomes. Case reviews of frequently admitted patients can fall under these established activities.
IMPLICATIONS
In this vision of the ideal care transition, I am suggesting a shift in culture from a predominantly hospital‐based program to a program that spans the care continuum and requires active participation and ownership from the PCP's team. It will require inpatient and outpatient providers to communicate early and frequently during the hospitalization, sharing patient information efficiently and working collaboratively as part of a larger team to meet the medical and psychosocial needs of the patient. This concept is not new, but has not been supported financially from payors.[1, 9, 40] Most PCPs operate on margins that cannot support additional PCP time to coordinate care for patients or staff to assist (although many PCPs believe this is the role of the primary care medical home).[33] Some payors agree that stipends to support infrastructure change are needed to improve patient outcomes.[17, 38, 39]
Even though every envisioned step does not require additional funding, new payment arrangements under ACOs and bundled payments may offer opportunities for PCPs to assume a larger role in care transitions and secure funding to pay for interventions. However, primary care practices must be positioned to negotiate favorable global payment agreements, be willing to assume risks associated with global payments, and prioritize management of medically and socially complex patients who are at risk for preventable ED visits and hospitalizations. PCPs who are not participating in ACOs or bundled payments, or those who are risk adverse, may be able to finance pieces of this vision with the new care coordination CPT codes supported by Medicare (and possibly commercial payors in the future). They may also partner with community groups participating in CCTP for additional support. Others focus on the long‐term benefits of ACO‐like structures rather than the short‐term investments needed.[41]
Are all 14 steps proposed above essential? Without doubt, this vision will be difficult to fully operationalize and requires coordination and support from many distinct groups. Should all patients be offered a basic package of interventions, reserving the full package for those who are identified as highest risk for poor outcomes after hospital discharge? There is already some support around specialized interventions for patients at high risk for readmissions,[32, 41] and risk prediction models have been introduced to identify these individuals.[42] Or should we approach this as a menu of interventions from which to choose, tailoring interventions to individual patient needs? These questions should be tested, as our experience in coordinating care across the continuum matures. With over 100 ACOs formed in Medicare alone[43] and many more with commercial insurers, our understanding in this area will grow in the next 5 years.
CONCLUSIONS
As cost containment measures in healthcare target preventable readmissions, hospitals and primary care physicians are increasingly encouraged to improve transitions along the care continuum. In this article, I offer 1 PCP's vision of the ideal transitions‐of‐care program from hospital to home. This article focuses on steps that can be taken by PCPs and their clinic staff; it does not address the role of outpatient specialists, home care agencies, or community support groups in care transitions. Operationalizing this vision requires commitment from the hospital and clinic leadership, as well as buy‐in from front‐line providers. More research is required to understand the marginal impact of each component of this vision, as well as the comprehensive package of interventions proposed, on patient outcomes. New financial models with payors and hospitals may make it easier for primary care clinics to test this vision. Current financial incentives are likely still inadequate to fully align care along the continuum, but they offer some support for more PCPs to take an active role. The time has come to shift our traditional view of transitions of care from a hospital‐centric set of interventions toward one that spans the entire care continuum and includes primary care physicians and their clinic staff as key partners.
Acknowledgments
The author thanks Jeffrey Fujimoto for his assistance with the literature review.
Disclosures: Ning Tang, MD, is supported by a University of California, Center for Health Quality and Innovation grant. Dr. Tang has no financial conflicts of interests.
- Reducing hospital readmissions: lessons from top‐performing hospitals. The Commonwealth Fund Synthesis Report. April 2011. Available at: http://www.commonwealthfund.org/Publications/Case‐Studies/2011/Apr/Reducing‐Hospital‐Readmissions.aspx. Accessed January 30, 2013. , , .
- Effective Interventions to Reduce Rehospitalizations: A Survey of the Published Evidence. Cambridge, MA: Institute for Healthcare Improvement; 2009. , .
- Society of Hospital Medicine. Project BOOST. Available at: www.hospitalmedicine.org/BOOST/. Accessed January 30, 2013.
- American Association of Medical Colleges. HOMERUN Executive Summary. Available at: https://members.aamc.org/eweb/upload/HOMERUN%20summary%202012.pdf. Accessed January 30, 2013.
- Interventions to reduce 30‐day rehospitalization: a systematic review. Ann Intern Med. 2011;155:520–528. , , , , .
- Contemporary evidence about hospital strategies for reducing 30‐day readmissions. J Am Coll Cardiol. 2012;60:607–614. , , , et al.
- Improving patient handovers from hospital to primary care: a systematic review. Ann Intern Med. 2012;157:417–428. , , , et al.
- Hospitalists and care transitions: the divorce of inpatient and outpatient care. Health Aff. 2008;27:1315–1327. , , , .
- Recasting readmissions by placing the hospital role in community context. JAMA. 2013;309:351–352. , , .
- Promoting effective transitions of care at hospital discharge: a review of key issues for hospitalists. J Hosp Med. 2007;2:314–323. , , , .
- Transition of care for hospitalized elderly patients—development of a discharge checklist for hospitalists. J Hosp Med. 2006;1:354–360. , , , et al.
- Transitioning the patient with acute coronary syndrome from inpatient to primary care. J Hosp Med. 2010;5(suppl):S8–S14. .
- Telephone follow‐up, initiated by a hospital‐based health professional, for postdischarge problems in patients discharged from hospital to home. Cochrane Database Syst Rev. 2006;(4):CD004510. , .
- Post‐discharge intervention in vulnerable, chronically ill patients. J Hosp Med. 2012;7:124–130. , , , .
- Low‐cost transitional care with nurse managers making mostly phone contact with patients cut rehospitalization at a VA hospital. Health Aff. 2012;31:2659–2668. , , , et al.
- Redefining and redesigning hospital discharge to enhance patient care: a randomized control study. J Gen Intern Med. 2008;23:1228–1233. , , , .
- How Geisinger's advanced medical home model argues the case for rapid‐cycle innovation. Health Aff. 2010;29:2047–2053. . , , , et al.
- Telephone follow‐up as a primary care intervention for postdischarge outcomes improvement: a systematic review. Am J Med. 2012;125:915–921. , , .
- The impact of postdischarge telephonic follow‐up on hospital readmissions. Popul Health Manag. 2011;14:27–32. , , , , .
- Prioritized post‐discharge telephonic outreach reduces hospital readmissions for select high‐risk patients. Am J Manag Care. 2012;18:838–844. , , , , .
- Association of ICU or hospital admission with unintentional discontinuation of medications for chronic diseases. JAMA. 2011;306:840–847. , , , et al.
- Effect of a pharmacist intervention on clinically important medication errors after hospital discharge. Ann Intern Med. 2012;157:1–10. , , , et al.
- Relationship of health literacy to intentional and unintentional non‐adherence of hospital discharge medications. J Gen Intern Med. 2012;27:173–178. , , , , , .
- Patient experiences of transitioning from hospital to home: an ethnographic quality improvement project. J Hosp Med. 2012;7:382–387. , , , , .
- Medicare finalizes physician pay for new care coordination benefit. American Medical News. November 12, 2012. Available at: http://www.ama‐assn.org/amednews/2012/11/12/gvl11112.htm. Accessed February 8, 2013. .
- Outpatient follow‐up visit and 30‐day emergency department visit and readmission in patients hospitalized for chronic obstructive pulmonary disease. Arch Intern Med. 2010;170:1664–1670. , , , , .
- Post‐hospitalization transitions: examining the effects of timing of primary care provider follow‐up. J Hosp Med. 2010;5:392–397. , , .
- Relationship between early physician follow‐up and 30‐day readmission among Medicare beneficiaries hospitalized for heart failure. JAMA. 2010;303:1716–1722. , , , et al.
- Effects of discharge planning and compliance with outpatient appointments on readmission rates. Psychiatr Serv. 2000;51:885–889. , , .
- Do timely outpatient follow‐up visits decrease hospital readmission rates? Am J Med Qual. 2012;27:11–15. , , , , .
- Project BOOST. Tool for addressing risk: a geriatric evaluation for transitions. Available at: http://www.hospitalmedicine.org/ResourceRoomRedesign/RR_CareTransitions/PDFs/TARGET.pdf. Accessed February 3, 2013.
- How‐to Guide: Improving Transitions from the Hospital to Community Settings to Reduce Avoidable Rehospitalizations. Cambridge, MA: Institute for Healthcare Improvement; 2012. , , , , .
- Primary care: current problems and proposed solutions. Health Aff. 2010;29:799–805. , .
- Center for Medicare and Medicaid Innovation. Community‐based Care Transitions Program. Available at: http://innovation.cms.gov/initiatives/CCTP/#collapse‐tableDetails. Accessed February 8, 2013.
- Advanced access scheduling outcomes: a systematic review. Arch Intern Med. 2011;171:1150–1159. , , .
- The Improvement Guide: A Practical Approach to Enhancing Organizational Performance. 2nd ed. San Francisco, CA: Jossey‐Bass Publishers; 2009. , , , .
- Audit and feedback: effects on professional practice and healthcare outcomes. Cochrane Database Syst Rev. 2012;(6):CD000259. , , , et al.
- Agency for Healthcare Research and Quality. Policy Innovation Profile. Insurer provides financial incentives, infrastructure, and other support to stimulate provider participation in quality improvement collaborations. June 6, 2012. Available at: http://www.innovations.ahrq.gov/content.aspx?id=3641. Accessed February 8, 2013.
- Private‐payer innovation in Massachusetts: The “Alternative Quality Contract.” Health Aff. 2011;30:51–61. , , , .
- AMA Expert Panel on Care Transitions. There and home again, safely: five responsibilities of ambulatory practices in high quality care transitions. American Medical Association; Chicago, IL; 2013. Available at: www.ama‐assn.org/go/patientsafety. Accessed February 22, 2013. , ;
- Agency for Healthcare Research and Quality. Policy Innovation Profile. Medical center establishes infrastructure to manage care under capitated contracts, leading to better chronic care management and lower utilization and costs. October 3, 2012. Available at: http://www.innovations.ahrq.gov/content.aspx?id=3651. Accessed February 8, 2013.
- Risk prediction models for hospital readmission: a systematic review. JAMA. 2011;306:1688–1698. , , , et al.
- Centers for Medicare and Medicaid Services. More doctors, hospitals partner to coordinate care for people with Medicare: providers form 106 new accountable care organizations. Press release January 10, 2013. Available at: http://www.cms.gov/apps/media/press/release.asp?Counter=4501297:831–841.
- Transitions of care consensus policy statement American College of Physicians‐Society of General Internal Medicine‐Society of Hospital Medicine‐American Geriatrics Society‐American College of Emergency Physicians‐Society of Academic Emergency Medicine. J Gen Intern Med. 2009;24:971–976. , , , et al.
- Patient safety concerns arising from test results that return after hospital discharge. Ann Intern Med. 2005;143(2):121–128. , , , et al.
- Tying up loose ends: discharging patients with unresolved medical issues. Arch Intern Med. 2007;167:1305–1311. , , .
- Perceptions of readmitted patients on the transition from hospital to home. J Hosp Med. 2012;7:709–712. , , , , , .
- Patients' understanding of their treatment plans and diagnosis at discharge. Mayo Clin Proc. 2005;80:991–994. , .
- Reducing hospital readmissions: lessons from top‐performing hospitals. The Commonwealth Fund Synthesis Report. April 2011. Available at: http://www.commonwealthfund.org/Publications/Case‐Studies/2011/Apr/Reducing‐Hospital‐Readmissions.aspx. Accessed January 30, 2013. , , .
- Effective Interventions to Reduce Rehospitalizations: A Survey of the Published Evidence. Cambridge, MA: Institute for Healthcare Improvement; 2009. , .
- Society of Hospital Medicine. Project BOOST. Available at: www.hospitalmedicine.org/BOOST/. Accessed January 30, 2013.
- American Association of Medical Colleges. HOMERUN Executive Summary. Available at: https://members.aamc.org/eweb/upload/HOMERUN%20summary%202012.pdf. Accessed January 30, 2013.
- Interventions to reduce 30‐day rehospitalization: a systematic review. Ann Intern Med. 2011;155:520–528. , , , , .
- Contemporary evidence about hospital strategies for reducing 30‐day readmissions. J Am Coll Cardiol. 2012;60:607–614. , , , et al.
- Improving patient handovers from hospital to primary care: a systematic review. Ann Intern Med. 2012;157:417–428. , , , et al.
- Hospitalists and care transitions: the divorce of inpatient and outpatient care. Health Aff. 2008;27:1315–1327. , , , .
- Recasting readmissions by placing the hospital role in community context. JAMA. 2013;309:351–352. , , .
- Promoting effective transitions of care at hospital discharge: a review of key issues for hospitalists. J Hosp Med. 2007;2:314–323. , , , .
- Transition of care for hospitalized elderly patients—development of a discharge checklist for hospitalists. J Hosp Med. 2006;1:354–360. , , , et al.
- Transitioning the patient with acute coronary syndrome from inpatient to primary care. J Hosp Med. 2010;5(suppl):S8–S14. .
- Telephone follow‐up, initiated by a hospital‐based health professional, for postdischarge problems in patients discharged from hospital to home. Cochrane Database Syst Rev. 2006;(4):CD004510. , .
- Post‐discharge intervention in vulnerable, chronically ill patients. J Hosp Med. 2012;7:124–130. , , , .
- Low‐cost transitional care with nurse managers making mostly phone contact with patients cut rehospitalization at a VA hospital. Health Aff. 2012;31:2659–2668. , , , et al.
- Redefining and redesigning hospital discharge to enhance patient care: a randomized control study. J Gen Intern Med. 2008;23:1228–1233. , , , .
- How Geisinger's advanced medical home model argues the case for rapid‐cycle innovation. Health Aff. 2010;29:2047–2053. . , , , et al.
- Telephone follow‐up as a primary care intervention for postdischarge outcomes improvement: a systematic review. Am J Med. 2012;125:915–921. , , .
- The impact of postdischarge telephonic follow‐up on hospital readmissions. Popul Health Manag. 2011;14:27–32. , , , , .
- Prioritized post‐discharge telephonic outreach reduces hospital readmissions for select high‐risk patients. Am J Manag Care. 2012;18:838–844. , , , , .
- Association of ICU or hospital admission with unintentional discontinuation of medications for chronic diseases. JAMA. 2011;306:840–847. , , , et al.
- Effect of a pharmacist intervention on clinically important medication errors after hospital discharge. Ann Intern Med. 2012;157:1–10. , , , et al.
- Relationship of health literacy to intentional and unintentional non‐adherence of hospital discharge medications. J Gen Intern Med. 2012;27:173–178. , , , , , .
- Patient experiences of transitioning from hospital to home: an ethnographic quality improvement project. J Hosp Med. 2012;7:382–387. , , , , .
- Medicare finalizes physician pay for new care coordination benefit. American Medical News. November 12, 2012. Available at: http://www.ama‐assn.org/amednews/2012/11/12/gvl11112.htm. Accessed February 8, 2013. .
- Outpatient follow‐up visit and 30‐day emergency department visit and readmission in patients hospitalized for chronic obstructive pulmonary disease. Arch Intern Med. 2010;170:1664–1670. , , , , .
- Post‐hospitalization transitions: examining the effects of timing of primary care provider follow‐up. J Hosp Med. 2010;5:392–397. , , .
- Relationship between early physician follow‐up and 30‐day readmission among Medicare beneficiaries hospitalized for heart failure. JAMA. 2010;303:1716–1722. , , , et al.
- Effects of discharge planning and compliance with outpatient appointments on readmission rates. Psychiatr Serv. 2000;51:885–889. , , .
- Do timely outpatient follow‐up visits decrease hospital readmission rates? Am J Med Qual. 2012;27:11–15. , , , , .
- Project BOOST. Tool for addressing risk: a geriatric evaluation for transitions. Available at: http://www.hospitalmedicine.org/ResourceRoomRedesign/RR_CareTransitions/PDFs/TARGET.pdf. Accessed February 3, 2013.
- How‐to Guide: Improving Transitions from the Hospital to Community Settings to Reduce Avoidable Rehospitalizations. Cambridge, MA: Institute for Healthcare Improvement; 2012. , , , , .
- Primary care: current problems and proposed solutions. Health Aff. 2010;29:799–805. , .
- Center for Medicare and Medicaid Innovation. Community‐based Care Transitions Program. Available at: http://innovation.cms.gov/initiatives/CCTP/#collapse‐tableDetails. Accessed February 8, 2013.
- Advanced access scheduling outcomes: a systematic review. Arch Intern Med. 2011;171:1150–1159. , , .
- The Improvement Guide: A Practical Approach to Enhancing Organizational Performance. 2nd ed. San Francisco, CA: Jossey‐Bass Publishers; 2009. , , , .
- Audit and feedback: effects on professional practice and healthcare outcomes. Cochrane Database Syst Rev. 2012;(6):CD000259. , , , et al.
- Agency for Healthcare Research and Quality. Policy Innovation Profile. Insurer provides financial incentives, infrastructure, and other support to stimulate provider participation in quality improvement collaborations. June 6, 2012. Available at: http://www.innovations.ahrq.gov/content.aspx?id=3641. Accessed February 8, 2013.
- Private‐payer innovation in Massachusetts: The “Alternative Quality Contract.” Health Aff. 2011;30:51–61. , , , .
- AMA Expert Panel on Care Transitions. There and home again, safely: five responsibilities of ambulatory practices in high quality care transitions. American Medical Association; Chicago, IL; 2013. Available at: www.ama‐assn.org/go/patientsafety. Accessed February 22, 2013. , ;
- Agency for Healthcare Research and Quality. Policy Innovation Profile. Medical center establishes infrastructure to manage care under capitated contracts, leading to better chronic care management and lower utilization and costs. October 3, 2012. Available at: http://www.innovations.ahrq.gov/content.aspx?id=3651. Accessed February 8, 2013.
- Risk prediction models for hospital readmission: a systematic review. JAMA. 2011;306:1688–1698. , , , et al.
- Centers for Medicare and Medicaid Services. More doctors, hospitals partner to coordinate care for people with Medicare: providers form 106 new accountable care organizations. Press release January 10, 2013. Available at: http://www.cms.gov/apps/media/press/release.asp?Counter=4501297:831–841.
- Transitions of care consensus policy statement American College of Physicians‐Society of General Internal Medicine‐Society of Hospital Medicine‐American Geriatrics Society‐American College of Emergency Physicians‐Society of Academic Emergency Medicine. J Gen Intern Med. 2009;24:971–976. , , , et al.
- Patient safety concerns arising from test results that return after hospital discharge. Ann Intern Med. 2005;143(2):121–128. , , , et al.
- Tying up loose ends: discharging patients with unresolved medical issues. Arch Intern Med. 2007;167:1305–1311. , , .
- Perceptions of readmitted patients on the transition from hospital to home. J Hosp Med. 2012;7:709–712. , , , , , .
- Patients' understanding of their treatment plans and diagnosis at discharge. Mayo Clin Proc. 2005;80:991–994. , .