User login
Is Hospital Discharge the Rube Goldberg Machine of Academic Internal Medicine?
One of the least taught yet most complicated tasks confronting new trainees is the bewildering process of discharging a patient. On an internal medicine service, this process can often resemble a Rube Goldberg machine, in which a “simple” task is accomplished through a series of interconnected, almost comically convoluted, yet separate steps that are triggered one after another and must be executed perfectly in sequence for success. It seems easy at first; just tap out a few sentences in the discharge paperwork, do a quick medication reconciliation, and a click of a button later, voila! The patient magically falls off the list and is on their merry way home. In reality, it only takes one wrench thrown into the Rube Goldberg machine to take down the whole operation. Much to the chagrin of internal medicine interns across the country, residents quickly learn that discharge planning is usually far from straightforward and that a myriad of obstacles (often dynamic and frustratingly unpredictable) can stand in the way of a successful discharge.
While some surgical services can streamline discharge processes to target defined lengths of stay based on a particular diagnosis, general medicine patients tend to have greater numbers of comorbid conditions, complex hospital courses, and wider variation in access to posthospital healthcare. In addition, there is very little formal instruction in transitions of care, and most residents identify direct patient care (learning by doing) as the primary mode of education.1,2 Struggling through the process of finding an appropriate placement, ensuring adequate outpatient follow-up, and untangling a jumbled mess of a medication reconciliation is often the only way that housestaff learn the Sisyphean task of transitioning care out of the hospital. The unpredictability and intensity of patient care adds to the ever growing list of competing demands on the time and attention of residents. Attendings face pressure on all sides to not only provide exemplary patient care and an educational experience but also to optimize hospital throughput by discharging patients as soon as possible (and ideally before noon). No wonder that the discharge process can threaten to unravel at any time, with delays and complications in discharge metamorphosing into increased length of stay (LOS), poorer outcomes, and increased 30-day readmission rates. As on-the-ground providers, what realities do we face when challenging ourselves to discharge patients before noon, and what practical changes in our workflow can we make to reach this goal?
In this month’s Journal of Hospital Medicine, Zoucha et al. examine these questions in real time by identifying barriers preventing both “definite” and “possible” discharges at three representative time points over the course of randomly chosen weekdays. They surveyed both housestaff and attendings at five academic hospitals across the United States, and the majority of patients were cared for on teaching services.3 Reflecting the inherent differences in workflow between teaching and nonteaching services, delays in definite discharges on teaching services were most often hindered by completing rounds and the need to staff the patient with the attending, whereas nonresident services identified other patient-care-related (both urgent and nonurgent) issues to be the culprits. Late-afternoon discharges were delayed on teaching services due to outstanding paperwork and follow-up arrangements, both of which most senior residents are keenly aware of and make their best effort to complete ahead of time. Patients designated as “possible” discharges were awaiting clinical improvement and resolution of disposition issues dependent on social work and safe placement, which reasonably seemed independent of service type. These descriptive findings suggest that nonresident services are more efficient than resident teams, and we are keen to identify novel solutions, such as dedicated discharge coordinators,4 to facilitate the discharge process on resident teams without detracting from the educational value of the rotation.
Zoucha et al. also found that factors beyond our control (having a lower daily census, attending on a nonresident service) were significantly associated with both earlier discharge order entry times and the actual time of patient discharge.3 While it is tempting to foist the entirety of the blame on extrinsic factors such as discharge placement and insurance issues, the reality is there might be some workflow changes that could expedite the discharge process. The authors are correct to emphasize that rounding style, in which discharges are prioritized to be seen first, is a behavior modification worth targeting. The percentage of teams that routinely see discharges first is not well studied, as other factors, such as clinically unstable patients, new admissions from overnight, and even mundane characteristics such as geographic location in the hospital, can also compete for prioritization in rounding order. Given the authors’ findings, we are eager to see further work in this area as prioritization of discharges during rounds could conceivably be studied within the context of a randomized controlled trial. Other innovations in rounding styles such as rounding-in-flow5 (in which all tasks are completed for a single patient before rounding on the next patient) can also significantly reduce the time to discharge order placement.
With help from the Penn Medicine Center for Health Care Innovation, we are actively studying bottlenecks in the discharge process by developing an interactive platform focused on delivering real-time information to all members of the healthcare team. Rapid rounds are held every morning with the attending physician, floor nursing leadership, physical therapy, social worker, and case management to quickly identify pending tasks, anticipated disposition, and a target date of discharge. Efficiency is key, as each team is limited to approximately 5-10 minutes. Previous studies (mostly pre–post studies) have shown that this simple intervention significantly reduced LOS,6,7 increased rates of discharge before noon,8 and was improved by electronic tracking tools.9 Our multidisciplinary rounds are unique in that information is then entered into an intuitive, web-based platform, which allows consolidation and analysis that permits generation of real-time statistics. By standardizing the discharge planning process, we hope to streamline a previously fragmented process and maximize the efficiency of hospital resource utilization.
Ultimately, high-quality care of complex patients on internal medicine services from admission to discharge requires hard work, smart utilization of resources, and a little bit of luck. There may not be a secret ingredient that guarantees perfectly efficient discharges 100% of the time, but this study inspires us to ponder additional approaches to this longstanding problem. The authors are to be congratulated for a rigorous study that illuminates where we as healthcare providers are able to realistically intervene to expedite the discharge process. First, having a lower census cap may not be possible in this era of maximal hospital usage, but this work suggests that thoughtful management of time on rounds may be a way to address the underlying problem. Secondly, the superior efficiency of nonteaching services may merely reflect the increased experience of the providers, and a realistic solution could be to implement a formal curriculum to educate housestaff about the discharge process, which would simultaneously address residency competency standards for transitions of care. Finally, the role of innovative informatics tools will surely open further avenues of investigation, as we continually evolve in response to intensifying standards of modern, efficient healthcare delivery in the 21st century. It may not be possible to eliminate the complexity from this particular Rube Goldberg machine, but taking the steps above may allow us to implement as many fail-safes as we can.
Disclosures
The authors have nothing to disclose.
1. Young E, Stickrath C, McNulty M, et al. Residents’ exposure to educational experiences in facilitating hospital discharges. J Grad Med Educ. 2017;9(2):184-189. doi: 10.4300/JGME-D-16-00503.1. PubMed
2. Greysen SR, Schiliro D, Curry L, et al. “Learning by doing” - Resident perspectives on developing competency in high-quality discharge care. J Gen Intern Med. 2012;27(9):1188-1194. doi: 10.1007/s11606-012-2094-5. PubMed
3. Zoucha J, Hull M, Keniston A, et al. Barriers to Early Hospital Discharge: A Cross-Sectional Study at Five Academic Hospitals. J Hosp Med. 2018;13(12):816-822. doi: 10.12788/jhm.3074. PubMed
4. Finn KM, Heffner R, Chang Y, et al. Improving the discharge process by embedding a discharge facilitator in a resident team. J Hosp Med. 2011;6(9):494-500. doi: 10.1002/jhm.924. PubMed
5. Calderon AS, Blackmore CC, Williams BL, et al. Transforming ward rounds through rounding-in-flow. J Grad Med Educ. 2014;6(4):750-755. doi: 10.4300/JGME-D-13-00324.1. PubMed
6. Kane M, Rohatgi N, Heidenreich PA, et al. Lean-based redesign of multidisciplinary rounds on general medicine service. J Hosp Med. 2018;13(7):482-485. doi: 10.12788/jhm.2908. PubMed
7. Gonçalves-Bradley D, Lannin N, Clemson L, Cameron ID, Shepperd S. Discharge planning from hospital. Cochrane Database Syst Rev. 2016;1-3. doi: 10.1002/14651858.CD000313.pub5.www.cochranelibrary.com. PubMed
8. Wertheimer B, Jacobs REA, Bailey M, et al. Discharge before noon: an achievable hospital goal. J Hosp Med. 2014;9(4):210-214. doi: 10.1002/jhm.2154. PubMed
9. Meo N, Paul E, Wilson C, Powers J, Magbual M, Miles KM. Introducing an electronic tracking tool into daily multidisciplinary discharge rounds on a medicine service: a quality improvement project to reduce length of stay. BMJ Open Qual. 2018;7(3):e000174. doi: 10.1136/bmjoq-2017-000174. PubMed
One of the least taught yet most complicated tasks confronting new trainees is the bewildering process of discharging a patient. On an internal medicine service, this process can often resemble a Rube Goldberg machine, in which a “simple” task is accomplished through a series of interconnected, almost comically convoluted, yet separate steps that are triggered one after another and must be executed perfectly in sequence for success. It seems easy at first; just tap out a few sentences in the discharge paperwork, do a quick medication reconciliation, and a click of a button later, voila! The patient magically falls off the list and is on their merry way home. In reality, it only takes one wrench thrown into the Rube Goldberg machine to take down the whole operation. Much to the chagrin of internal medicine interns across the country, residents quickly learn that discharge planning is usually far from straightforward and that a myriad of obstacles (often dynamic and frustratingly unpredictable) can stand in the way of a successful discharge.
While some surgical services can streamline discharge processes to target defined lengths of stay based on a particular diagnosis, general medicine patients tend to have greater numbers of comorbid conditions, complex hospital courses, and wider variation in access to posthospital healthcare. In addition, there is very little formal instruction in transitions of care, and most residents identify direct patient care (learning by doing) as the primary mode of education.1,2 Struggling through the process of finding an appropriate placement, ensuring adequate outpatient follow-up, and untangling a jumbled mess of a medication reconciliation is often the only way that housestaff learn the Sisyphean task of transitioning care out of the hospital. The unpredictability and intensity of patient care adds to the ever growing list of competing demands on the time and attention of residents. Attendings face pressure on all sides to not only provide exemplary patient care and an educational experience but also to optimize hospital throughput by discharging patients as soon as possible (and ideally before noon). No wonder that the discharge process can threaten to unravel at any time, with delays and complications in discharge metamorphosing into increased length of stay (LOS), poorer outcomes, and increased 30-day readmission rates. As on-the-ground providers, what realities do we face when challenging ourselves to discharge patients before noon, and what practical changes in our workflow can we make to reach this goal?
In this month’s Journal of Hospital Medicine, Zoucha et al. examine these questions in real time by identifying barriers preventing both “definite” and “possible” discharges at three representative time points over the course of randomly chosen weekdays. They surveyed both housestaff and attendings at five academic hospitals across the United States, and the majority of patients were cared for on teaching services.3 Reflecting the inherent differences in workflow between teaching and nonteaching services, delays in definite discharges on teaching services were most often hindered by completing rounds and the need to staff the patient with the attending, whereas nonresident services identified other patient-care-related (both urgent and nonurgent) issues to be the culprits. Late-afternoon discharges were delayed on teaching services due to outstanding paperwork and follow-up arrangements, both of which most senior residents are keenly aware of and make their best effort to complete ahead of time. Patients designated as “possible” discharges were awaiting clinical improvement and resolution of disposition issues dependent on social work and safe placement, which reasonably seemed independent of service type. These descriptive findings suggest that nonresident services are more efficient than resident teams, and we are keen to identify novel solutions, such as dedicated discharge coordinators,4 to facilitate the discharge process on resident teams without detracting from the educational value of the rotation.
Zoucha et al. also found that factors beyond our control (having a lower daily census, attending on a nonresident service) were significantly associated with both earlier discharge order entry times and the actual time of patient discharge.3 While it is tempting to foist the entirety of the blame on extrinsic factors such as discharge placement and insurance issues, the reality is there might be some workflow changes that could expedite the discharge process. The authors are correct to emphasize that rounding style, in which discharges are prioritized to be seen first, is a behavior modification worth targeting. The percentage of teams that routinely see discharges first is not well studied, as other factors, such as clinically unstable patients, new admissions from overnight, and even mundane characteristics such as geographic location in the hospital, can also compete for prioritization in rounding order. Given the authors’ findings, we are eager to see further work in this area as prioritization of discharges during rounds could conceivably be studied within the context of a randomized controlled trial. Other innovations in rounding styles such as rounding-in-flow5 (in which all tasks are completed for a single patient before rounding on the next patient) can also significantly reduce the time to discharge order placement.
With help from the Penn Medicine Center for Health Care Innovation, we are actively studying bottlenecks in the discharge process by developing an interactive platform focused on delivering real-time information to all members of the healthcare team. Rapid rounds are held every morning with the attending physician, floor nursing leadership, physical therapy, social worker, and case management to quickly identify pending tasks, anticipated disposition, and a target date of discharge. Efficiency is key, as each team is limited to approximately 5-10 minutes. Previous studies (mostly pre–post studies) have shown that this simple intervention significantly reduced LOS,6,7 increased rates of discharge before noon,8 and was improved by electronic tracking tools.9 Our multidisciplinary rounds are unique in that information is then entered into an intuitive, web-based platform, which allows consolidation and analysis that permits generation of real-time statistics. By standardizing the discharge planning process, we hope to streamline a previously fragmented process and maximize the efficiency of hospital resource utilization.
Ultimately, high-quality care of complex patients on internal medicine services from admission to discharge requires hard work, smart utilization of resources, and a little bit of luck. There may not be a secret ingredient that guarantees perfectly efficient discharges 100% of the time, but this study inspires us to ponder additional approaches to this longstanding problem. The authors are to be congratulated for a rigorous study that illuminates where we as healthcare providers are able to realistically intervene to expedite the discharge process. First, having a lower census cap may not be possible in this era of maximal hospital usage, but this work suggests that thoughtful management of time on rounds may be a way to address the underlying problem. Secondly, the superior efficiency of nonteaching services may merely reflect the increased experience of the providers, and a realistic solution could be to implement a formal curriculum to educate housestaff about the discharge process, which would simultaneously address residency competency standards for transitions of care. Finally, the role of innovative informatics tools will surely open further avenues of investigation, as we continually evolve in response to intensifying standards of modern, efficient healthcare delivery in the 21st century. It may not be possible to eliminate the complexity from this particular Rube Goldberg machine, but taking the steps above may allow us to implement as many fail-safes as we can.
Disclosures
The authors have nothing to disclose.
One of the least taught yet most complicated tasks confronting new trainees is the bewildering process of discharging a patient. On an internal medicine service, this process can often resemble a Rube Goldberg machine, in which a “simple” task is accomplished through a series of interconnected, almost comically convoluted, yet separate steps that are triggered one after another and must be executed perfectly in sequence for success. It seems easy at first; just tap out a few sentences in the discharge paperwork, do a quick medication reconciliation, and a click of a button later, voila! The patient magically falls off the list and is on their merry way home. In reality, it only takes one wrench thrown into the Rube Goldberg machine to take down the whole operation. Much to the chagrin of internal medicine interns across the country, residents quickly learn that discharge planning is usually far from straightforward and that a myriad of obstacles (often dynamic and frustratingly unpredictable) can stand in the way of a successful discharge.
While some surgical services can streamline discharge processes to target defined lengths of stay based on a particular diagnosis, general medicine patients tend to have greater numbers of comorbid conditions, complex hospital courses, and wider variation in access to posthospital healthcare. In addition, there is very little formal instruction in transitions of care, and most residents identify direct patient care (learning by doing) as the primary mode of education.1,2 Struggling through the process of finding an appropriate placement, ensuring adequate outpatient follow-up, and untangling a jumbled mess of a medication reconciliation is often the only way that housestaff learn the Sisyphean task of transitioning care out of the hospital. The unpredictability and intensity of patient care adds to the ever growing list of competing demands on the time and attention of residents. Attendings face pressure on all sides to not only provide exemplary patient care and an educational experience but also to optimize hospital throughput by discharging patients as soon as possible (and ideally before noon). No wonder that the discharge process can threaten to unravel at any time, with delays and complications in discharge metamorphosing into increased length of stay (LOS), poorer outcomes, and increased 30-day readmission rates. As on-the-ground providers, what realities do we face when challenging ourselves to discharge patients before noon, and what practical changes in our workflow can we make to reach this goal?
In this month’s Journal of Hospital Medicine, Zoucha et al. examine these questions in real time by identifying barriers preventing both “definite” and “possible” discharges at three representative time points over the course of randomly chosen weekdays. They surveyed both housestaff and attendings at five academic hospitals across the United States, and the majority of patients were cared for on teaching services.3 Reflecting the inherent differences in workflow between teaching and nonteaching services, delays in definite discharges on teaching services were most often hindered by completing rounds and the need to staff the patient with the attending, whereas nonresident services identified other patient-care-related (both urgent and nonurgent) issues to be the culprits. Late-afternoon discharges were delayed on teaching services due to outstanding paperwork and follow-up arrangements, both of which most senior residents are keenly aware of and make their best effort to complete ahead of time. Patients designated as “possible” discharges were awaiting clinical improvement and resolution of disposition issues dependent on social work and safe placement, which reasonably seemed independent of service type. These descriptive findings suggest that nonresident services are more efficient than resident teams, and we are keen to identify novel solutions, such as dedicated discharge coordinators,4 to facilitate the discharge process on resident teams without detracting from the educational value of the rotation.
Zoucha et al. also found that factors beyond our control (having a lower daily census, attending on a nonresident service) were significantly associated with both earlier discharge order entry times and the actual time of patient discharge.3 While it is tempting to foist the entirety of the blame on extrinsic factors such as discharge placement and insurance issues, the reality is there might be some workflow changes that could expedite the discharge process. The authors are correct to emphasize that rounding style, in which discharges are prioritized to be seen first, is a behavior modification worth targeting. The percentage of teams that routinely see discharges first is not well studied, as other factors, such as clinically unstable patients, new admissions from overnight, and even mundane characteristics such as geographic location in the hospital, can also compete for prioritization in rounding order. Given the authors’ findings, we are eager to see further work in this area as prioritization of discharges during rounds could conceivably be studied within the context of a randomized controlled trial. Other innovations in rounding styles such as rounding-in-flow5 (in which all tasks are completed for a single patient before rounding on the next patient) can also significantly reduce the time to discharge order placement.
With help from the Penn Medicine Center for Health Care Innovation, we are actively studying bottlenecks in the discharge process by developing an interactive platform focused on delivering real-time information to all members of the healthcare team. Rapid rounds are held every morning with the attending physician, floor nursing leadership, physical therapy, social worker, and case management to quickly identify pending tasks, anticipated disposition, and a target date of discharge. Efficiency is key, as each team is limited to approximately 5-10 minutes. Previous studies (mostly pre–post studies) have shown that this simple intervention significantly reduced LOS,6,7 increased rates of discharge before noon,8 and was improved by electronic tracking tools.9 Our multidisciplinary rounds are unique in that information is then entered into an intuitive, web-based platform, which allows consolidation and analysis that permits generation of real-time statistics. By standardizing the discharge planning process, we hope to streamline a previously fragmented process and maximize the efficiency of hospital resource utilization.
Ultimately, high-quality care of complex patients on internal medicine services from admission to discharge requires hard work, smart utilization of resources, and a little bit of luck. There may not be a secret ingredient that guarantees perfectly efficient discharges 100% of the time, but this study inspires us to ponder additional approaches to this longstanding problem. The authors are to be congratulated for a rigorous study that illuminates where we as healthcare providers are able to realistically intervene to expedite the discharge process. First, having a lower census cap may not be possible in this era of maximal hospital usage, but this work suggests that thoughtful management of time on rounds may be a way to address the underlying problem. Secondly, the superior efficiency of nonteaching services may merely reflect the increased experience of the providers, and a realistic solution could be to implement a formal curriculum to educate housestaff about the discharge process, which would simultaneously address residency competency standards for transitions of care. Finally, the role of innovative informatics tools will surely open further avenues of investigation, as we continually evolve in response to intensifying standards of modern, efficient healthcare delivery in the 21st century. It may not be possible to eliminate the complexity from this particular Rube Goldberg machine, but taking the steps above may allow us to implement as many fail-safes as we can.
Disclosures
The authors have nothing to disclose.
1. Young E, Stickrath C, McNulty M, et al. Residents’ exposure to educational experiences in facilitating hospital discharges. J Grad Med Educ. 2017;9(2):184-189. doi: 10.4300/JGME-D-16-00503.1. PubMed
2. Greysen SR, Schiliro D, Curry L, et al. “Learning by doing” - Resident perspectives on developing competency in high-quality discharge care. J Gen Intern Med. 2012;27(9):1188-1194. doi: 10.1007/s11606-012-2094-5. PubMed
3. Zoucha J, Hull M, Keniston A, et al. Barriers to Early Hospital Discharge: A Cross-Sectional Study at Five Academic Hospitals. J Hosp Med. 2018;13(12):816-822. doi: 10.12788/jhm.3074. PubMed
4. Finn KM, Heffner R, Chang Y, et al. Improving the discharge process by embedding a discharge facilitator in a resident team. J Hosp Med. 2011;6(9):494-500. doi: 10.1002/jhm.924. PubMed
5. Calderon AS, Blackmore CC, Williams BL, et al. Transforming ward rounds through rounding-in-flow. J Grad Med Educ. 2014;6(4):750-755. doi: 10.4300/JGME-D-13-00324.1. PubMed
6. Kane M, Rohatgi N, Heidenreich PA, et al. Lean-based redesign of multidisciplinary rounds on general medicine service. J Hosp Med. 2018;13(7):482-485. doi: 10.12788/jhm.2908. PubMed
7. Gonçalves-Bradley D, Lannin N, Clemson L, Cameron ID, Shepperd S. Discharge planning from hospital. Cochrane Database Syst Rev. 2016;1-3. doi: 10.1002/14651858.CD000313.pub5.www.cochranelibrary.com. PubMed
8. Wertheimer B, Jacobs REA, Bailey M, et al. Discharge before noon: an achievable hospital goal. J Hosp Med. 2014;9(4):210-214. doi: 10.1002/jhm.2154. PubMed
9. Meo N, Paul E, Wilson C, Powers J, Magbual M, Miles KM. Introducing an electronic tracking tool into daily multidisciplinary discharge rounds on a medicine service: a quality improvement project to reduce length of stay. BMJ Open Qual. 2018;7(3):e000174. doi: 10.1136/bmjoq-2017-000174. PubMed
1. Young E, Stickrath C, McNulty M, et al. Residents’ exposure to educational experiences in facilitating hospital discharges. J Grad Med Educ. 2017;9(2):184-189. doi: 10.4300/JGME-D-16-00503.1. PubMed
2. Greysen SR, Schiliro D, Curry L, et al. “Learning by doing” - Resident perspectives on developing competency in high-quality discharge care. J Gen Intern Med. 2012;27(9):1188-1194. doi: 10.1007/s11606-012-2094-5. PubMed
3. Zoucha J, Hull M, Keniston A, et al. Barriers to Early Hospital Discharge: A Cross-Sectional Study at Five Academic Hospitals. J Hosp Med. 2018;13(12):816-822. doi: 10.12788/jhm.3074. PubMed
4. Finn KM, Heffner R, Chang Y, et al. Improving the discharge process by embedding a discharge facilitator in a resident team. J Hosp Med. 2011;6(9):494-500. doi: 10.1002/jhm.924. PubMed
5. Calderon AS, Blackmore CC, Williams BL, et al. Transforming ward rounds through rounding-in-flow. J Grad Med Educ. 2014;6(4):750-755. doi: 10.4300/JGME-D-13-00324.1. PubMed
6. Kane M, Rohatgi N, Heidenreich PA, et al. Lean-based redesign of multidisciplinary rounds on general medicine service. J Hosp Med. 2018;13(7):482-485. doi: 10.12788/jhm.2908. PubMed
7. Gonçalves-Bradley D, Lannin N, Clemson L, Cameron ID, Shepperd S. Discharge planning from hospital. Cochrane Database Syst Rev. 2016;1-3. doi: 10.1002/14651858.CD000313.pub5.www.cochranelibrary.com. PubMed
8. Wertheimer B, Jacobs REA, Bailey M, et al. Discharge before noon: an achievable hospital goal. J Hosp Med. 2014;9(4):210-214. doi: 10.1002/jhm.2154. PubMed
9. Meo N, Paul E, Wilson C, Powers J, Magbual M, Miles KM. Introducing an electronic tracking tool into daily multidisciplinary discharge rounds on a medicine service: a quality improvement project to reduce length of stay. BMJ Open Qual. 2018;7(3):e000174. doi: 10.1136/bmjoq-2017-000174. PubMed
© 2018 Society of Hospital Medicine
Patient-Centered, Payer-Centered, or Both? The 30-Day Readmission Metric
There is little doubt that preventing 30-day readmissions to the hospital results in lower costs for payers. However, reducing costs alone does not make this metric a measure of “high value” care.1 Rather, it is the improvement in the effectiveness of the discharge process that occurs alongside lower costs that makes readmission reduction efforts “high value” – or a “win-win” for patients and payers.
However, the article by Nuckols and colleagues in this month’s issue of the Journal of Hospital Medicine (JHM) suggests that it might not be that simple and adds nuance to the ongoing discussion about the 30-day readmission metric.2 The study used data collected by the federal government to examine changes not only in 30-day readmission rates between 2009-2010 and 2013-2014 but also changes in emergency department (ED) and observation unit visits. What they found is important. In general, despite reductions in 30-day readmissions for patients served by Medicare and private insurance, there were increases in observation unit and ED visits across all payer types (including Medicare and private insurance). These increases in observation unit and ED visits resulted in statistically higher overall “revisit” rates for the uninsured and those insured by Medicaid and offset any improvements in the “revisit” rates resulting from reductions in 30-day readmissions for those with private insurance. Those insured by Medicare—representing about 300,000 of the 420,000 visits analyzed—still had a statistically lower “revisit” rate, but it was only marginally lower (25.0% in 2013-2014 versus 25.3% in 2009-2010).2
The generalizability of the Nuckols’ study was limited in that it examined only index admissions for acute myocardial infarction (AMI), heart failure (HF), and pneumonia and used data from only Georgia, Nebraska, South Carolina, and Tennessee—the four states where observation and ED visit data were available in the federal database.2 The study also did not examine hospital-level revisit data; hence, it was not able to determine if hospitals with greater reductions in readmission rates had greater increases in observation or ED visits, as one might predict. Despite these limitations, the rigor of the study was noteworthy. The authors used matching techniques to ensure that the populations examined in the two time periods were comparable. Unlike previous research,3,4 they also used a comprehensive definition of a hospital “revisit” (including both observation and ED visits) and measured “revisit” rates across several payer types, rather than focusing exclusively on those covered by fee for service Medicare, as in past studies.4,5
What the study by Nuckols and colleagues suggests is that even though patients may be readmitted less, they may be coming back to the ED or getting admitted to the observation unit more, resulting in overall “revisit” rates that are marginally lower for Medicare patients, but often the same or even higher for other payer groups, particularly disadvantaged payer groups who are uninsured or insured by Medicaid.2 Although the authors do not assert causality for these trends, it is worth noting that the much-discussed Hospital Readmission Reduction Program (or “readmission penalty”) applies only to Medicare patients aged more than 65 years. It is likely that this program influenced the differences identified between payer groups in this article.
Beyond the policy implications of these findings, the experience of patients cared for in these different settings is of paramount importance. Unfortunately, there are limited data comparing patient perceptions, preferences, or outcomes resulting from readmission to an inpatient service versus an observation unit or ED visit within 30 days of discharge. However, there is reason to believe that costs could be higher for some patients treated in the ED or an observation unit as compared to those in the inpatient setting,6 and that care continuity and quality may be different across these settings. In a recent white paper on observation care published by the Society of Hospital Medicine (SHM) Public Policy Committee,7 the SHM reported the results of a 2017 survey of its members about observation care. The results were concerning. An overwhelming majority of respondents (87%) believed that the rules for observation are unclear for patients, and 68% of respondents believed that policy changes mandating informing patients of their observation status have created conflict between the provider and the patient.7 As shared by one respondent, “the observation issue can severely damage the therapeutic bond with patient/family, who may conclude that the hospitalist has more interest in saving someone money at the expense of patient care.”7 Thus, there is significant concern about the nature of observation stays and the experience for patients and providers. We should take care to better understand these experiences given that readmission reduction efforts may funnel more patients into observation care.
As a next step, we recommend further examination of how “revisit” rates have changed over time for patients with any discharge diagnosis, and not just those with pneumonia, AMI, or HF.8 Such examinations should be stratified by payer to identify differential impacts on those with lower socioeconomic status. Analyses should also examine changes in “revisit” types at the hospital level to better understand if hospitals with reductions in readmission rates are simply shifting revisits to the observation unit or ED. It is possible that inpatient readmissions for any given hospital are decreasing without concomitant increases in observation visits, as there are forces independent of the readmission penalty, such as the Recovery Audit Contractor program, that are driving hospitals to more frequently code patients as observation visits rather than inpatient admissions.9 Thus, readmissions could decrease and observation unit visits could increase independent of one another. We also recommend further research to examine differences in care quality, clinical outcomes, and costs for those readmitted to the hospital within 30 days of discharge versus those cared for in observation units or the ED. The challenge of such studies will be to identify and examine comparable populations of patients across these three settings. Examining patient perceptions and preferences across these settings is also critical. Finally, when assessing interventions to reduce inpatient readmissions, we need to consider “revisits” as a whole, not simply readmissions.10 Otherwise, we may simply be promoting the use of interventions that shift inpatient readmissions to observation unit or ED revisits, and there is little that is patient-centered or high value about that.9
Disclosures
The authors have nothing to disclose.
1. Smith M, Saunders R, Stuckhardt L, McGinnis JM, eds. Best care at lower cost: the path to continuously learning health care in America. Washington, DC: National Academies Press; 2013. PubMed
2. Nuckols TK, Fingar KR, Barrett ML, et al. Returns to emergency department, observation, or inpatient care within 30 days after hospitalization in 4 states, 2009 and 2010 versus 2013 and 2014. J Hosp Med. 2018;13(5):296-303. PubMed
3. Fingar KR, Washington R. Trends in Hospital Readmissions for Four High-Volume Conditions, 2009–2013. Statistical Brief No. 196. https://www.hcup-us.ahrq.gov/reports/statbriefs/sb196-Readmissions-Trends-High-Volume-Conditions.pdf. Accessed March 5, 2018.
4. Zuckerman RB, Sheingold SH, Orav EJ, Ruhter J, Epstein AM. Readmissions, observation, and the Hospital Readmissions Reduction Program. N Engl J Med. 2016;374(16):1543-1551. DOI: 10.1056/NEJMsa1513024. PubMed
5. Gerhardt G, Yemane A, Apostle K, Oelschlaeger A, Rollins E, Brennan N. Evaluating whether changes in utilization of hospital outpatient services contributed to lower Medicare readmission rate. Medicare Medicaid Res Rev. 2014;4(1). DOI: 10.5600/mmrr2014-004-01-b03 PubMed
6. Kangovi S, Cafardi SG, Smith RA, Kulkarni R, Grande D. Patient financial responsibility for observation care. J Hosp Med. 2015;10(11):718-723. DOI: 10.1002/jhm.2436. PubMed
7. The Hospital Observation Care Problem: Perspectives and Solutions from the Society of Hospital Medicine. Society of Hospital Medicine Public Policy Committee. https://www.hospitalmedicine.org/globalassets/policy-and-advocacy/advocacy-pdf/shms-observation-white-paper-2017. Accessed February 12, 2018.
8. Rosen AK, Chen Q, Shwartz M, et al. Does use of a hospital-wide readmission measure versus condition-specific readmission measures make a difference for hospital profiling and payment penalties? Medical Care. 2016;54(2):155-161. DOI: 10.1097/MLR.0000000000000455. PubMed
9. Baugh CW, Schuur JD. Observation care-high-value care or a cost-shifting loophole? N Engl J Med. 2013;369(4):302-305. DOI: 10.1056/NEJMp1304493. PubMed
10. Cassel CK, Conway PH, Delbanco SF, Jha AK, Saunders RS, Lee TH. Getting more performance from performance measurement. N Engl J Med. 2014;371(23):2145-2147. DOI: 10.1056/NEJMp1408345. PubMed
There is little doubt that preventing 30-day readmissions to the hospital results in lower costs for payers. However, reducing costs alone does not make this metric a measure of “high value” care.1 Rather, it is the improvement in the effectiveness of the discharge process that occurs alongside lower costs that makes readmission reduction efforts “high value” – or a “win-win” for patients and payers.
However, the article by Nuckols and colleagues in this month’s issue of the Journal of Hospital Medicine (JHM) suggests that it might not be that simple and adds nuance to the ongoing discussion about the 30-day readmission metric.2 The study used data collected by the federal government to examine changes not only in 30-day readmission rates between 2009-2010 and 2013-2014 but also changes in emergency department (ED) and observation unit visits. What they found is important. In general, despite reductions in 30-day readmissions for patients served by Medicare and private insurance, there were increases in observation unit and ED visits across all payer types (including Medicare and private insurance). These increases in observation unit and ED visits resulted in statistically higher overall “revisit” rates for the uninsured and those insured by Medicaid and offset any improvements in the “revisit” rates resulting from reductions in 30-day readmissions for those with private insurance. Those insured by Medicare—representing about 300,000 of the 420,000 visits analyzed—still had a statistically lower “revisit” rate, but it was only marginally lower (25.0% in 2013-2014 versus 25.3% in 2009-2010).2
The generalizability of the Nuckols’ study was limited in that it examined only index admissions for acute myocardial infarction (AMI), heart failure (HF), and pneumonia and used data from only Georgia, Nebraska, South Carolina, and Tennessee—the four states where observation and ED visit data were available in the federal database.2 The study also did not examine hospital-level revisit data; hence, it was not able to determine if hospitals with greater reductions in readmission rates had greater increases in observation or ED visits, as one might predict. Despite these limitations, the rigor of the study was noteworthy. The authors used matching techniques to ensure that the populations examined in the two time periods were comparable. Unlike previous research,3,4 they also used a comprehensive definition of a hospital “revisit” (including both observation and ED visits) and measured “revisit” rates across several payer types, rather than focusing exclusively on those covered by fee for service Medicare, as in past studies.4,5
What the study by Nuckols and colleagues suggests is that even though patients may be readmitted less, they may be coming back to the ED or getting admitted to the observation unit more, resulting in overall “revisit” rates that are marginally lower for Medicare patients, but often the same or even higher for other payer groups, particularly disadvantaged payer groups who are uninsured or insured by Medicaid.2 Although the authors do not assert causality for these trends, it is worth noting that the much-discussed Hospital Readmission Reduction Program (or “readmission penalty”) applies only to Medicare patients aged more than 65 years. It is likely that this program influenced the differences identified between payer groups in this article.
Beyond the policy implications of these findings, the experience of patients cared for in these different settings is of paramount importance. Unfortunately, there are limited data comparing patient perceptions, preferences, or outcomes resulting from readmission to an inpatient service versus an observation unit or ED visit within 30 days of discharge. However, there is reason to believe that costs could be higher for some patients treated in the ED or an observation unit as compared to those in the inpatient setting,6 and that care continuity and quality may be different across these settings. In a recent white paper on observation care published by the Society of Hospital Medicine (SHM) Public Policy Committee,7 the SHM reported the results of a 2017 survey of its members about observation care. The results were concerning. An overwhelming majority of respondents (87%) believed that the rules for observation are unclear for patients, and 68% of respondents believed that policy changes mandating informing patients of their observation status have created conflict between the provider and the patient.7 As shared by one respondent, “the observation issue can severely damage the therapeutic bond with patient/family, who may conclude that the hospitalist has more interest in saving someone money at the expense of patient care.”7 Thus, there is significant concern about the nature of observation stays and the experience for patients and providers. We should take care to better understand these experiences given that readmission reduction efforts may funnel more patients into observation care.
As a next step, we recommend further examination of how “revisit” rates have changed over time for patients with any discharge diagnosis, and not just those with pneumonia, AMI, or HF.8 Such examinations should be stratified by payer to identify differential impacts on those with lower socioeconomic status. Analyses should also examine changes in “revisit” types at the hospital level to better understand if hospitals with reductions in readmission rates are simply shifting revisits to the observation unit or ED. It is possible that inpatient readmissions for any given hospital are decreasing without concomitant increases in observation visits, as there are forces independent of the readmission penalty, such as the Recovery Audit Contractor program, that are driving hospitals to more frequently code patients as observation visits rather than inpatient admissions.9 Thus, readmissions could decrease and observation unit visits could increase independent of one another. We also recommend further research to examine differences in care quality, clinical outcomes, and costs for those readmitted to the hospital within 30 days of discharge versus those cared for in observation units or the ED. The challenge of such studies will be to identify and examine comparable populations of patients across these three settings. Examining patient perceptions and preferences across these settings is also critical. Finally, when assessing interventions to reduce inpatient readmissions, we need to consider “revisits” as a whole, not simply readmissions.10 Otherwise, we may simply be promoting the use of interventions that shift inpatient readmissions to observation unit or ED revisits, and there is little that is patient-centered or high value about that.9
Disclosures
The authors have nothing to disclose.
There is little doubt that preventing 30-day readmissions to the hospital results in lower costs for payers. However, reducing costs alone does not make this metric a measure of “high value” care.1 Rather, it is the improvement in the effectiveness of the discharge process that occurs alongside lower costs that makes readmission reduction efforts “high value” – or a “win-win” for patients and payers.
However, the article by Nuckols and colleagues in this month’s issue of the Journal of Hospital Medicine (JHM) suggests that it might not be that simple and adds nuance to the ongoing discussion about the 30-day readmission metric.2 The study used data collected by the federal government to examine changes not only in 30-day readmission rates between 2009-2010 and 2013-2014 but also changes in emergency department (ED) and observation unit visits. What they found is important. In general, despite reductions in 30-day readmissions for patients served by Medicare and private insurance, there were increases in observation unit and ED visits across all payer types (including Medicare and private insurance). These increases in observation unit and ED visits resulted in statistically higher overall “revisit” rates for the uninsured and those insured by Medicaid and offset any improvements in the “revisit” rates resulting from reductions in 30-day readmissions for those with private insurance. Those insured by Medicare—representing about 300,000 of the 420,000 visits analyzed—still had a statistically lower “revisit” rate, but it was only marginally lower (25.0% in 2013-2014 versus 25.3% in 2009-2010).2
The generalizability of the Nuckols’ study was limited in that it examined only index admissions for acute myocardial infarction (AMI), heart failure (HF), and pneumonia and used data from only Georgia, Nebraska, South Carolina, and Tennessee—the four states where observation and ED visit data were available in the federal database.2 The study also did not examine hospital-level revisit data; hence, it was not able to determine if hospitals with greater reductions in readmission rates had greater increases in observation or ED visits, as one might predict. Despite these limitations, the rigor of the study was noteworthy. The authors used matching techniques to ensure that the populations examined in the two time periods were comparable. Unlike previous research,3,4 they also used a comprehensive definition of a hospital “revisit” (including both observation and ED visits) and measured “revisit” rates across several payer types, rather than focusing exclusively on those covered by fee for service Medicare, as in past studies.4,5
What the study by Nuckols and colleagues suggests is that even though patients may be readmitted less, they may be coming back to the ED or getting admitted to the observation unit more, resulting in overall “revisit” rates that are marginally lower for Medicare patients, but often the same or even higher for other payer groups, particularly disadvantaged payer groups who are uninsured or insured by Medicaid.2 Although the authors do not assert causality for these trends, it is worth noting that the much-discussed Hospital Readmission Reduction Program (or “readmission penalty”) applies only to Medicare patients aged more than 65 years. It is likely that this program influenced the differences identified between payer groups in this article.
Beyond the policy implications of these findings, the experience of patients cared for in these different settings is of paramount importance. Unfortunately, there are limited data comparing patient perceptions, preferences, or outcomes resulting from readmission to an inpatient service versus an observation unit or ED visit within 30 days of discharge. However, there is reason to believe that costs could be higher for some patients treated in the ED or an observation unit as compared to those in the inpatient setting,6 and that care continuity and quality may be different across these settings. In a recent white paper on observation care published by the Society of Hospital Medicine (SHM) Public Policy Committee,7 the SHM reported the results of a 2017 survey of its members about observation care. The results were concerning. An overwhelming majority of respondents (87%) believed that the rules for observation are unclear for patients, and 68% of respondents believed that policy changes mandating informing patients of their observation status have created conflict between the provider and the patient.7 As shared by one respondent, “the observation issue can severely damage the therapeutic bond with patient/family, who may conclude that the hospitalist has more interest in saving someone money at the expense of patient care.”7 Thus, there is significant concern about the nature of observation stays and the experience for patients and providers. We should take care to better understand these experiences given that readmission reduction efforts may funnel more patients into observation care.
As a next step, we recommend further examination of how “revisit” rates have changed over time for patients with any discharge diagnosis, and not just those with pneumonia, AMI, or HF.8 Such examinations should be stratified by payer to identify differential impacts on those with lower socioeconomic status. Analyses should also examine changes in “revisit” types at the hospital level to better understand if hospitals with reductions in readmission rates are simply shifting revisits to the observation unit or ED. It is possible that inpatient readmissions for any given hospital are decreasing without concomitant increases in observation visits, as there are forces independent of the readmission penalty, such as the Recovery Audit Contractor program, that are driving hospitals to more frequently code patients as observation visits rather than inpatient admissions.9 Thus, readmissions could decrease and observation unit visits could increase independent of one another. We also recommend further research to examine differences in care quality, clinical outcomes, and costs for those readmitted to the hospital within 30 days of discharge versus those cared for in observation units or the ED. The challenge of such studies will be to identify and examine comparable populations of patients across these three settings. Examining patient perceptions and preferences across these settings is also critical. Finally, when assessing interventions to reduce inpatient readmissions, we need to consider “revisits” as a whole, not simply readmissions.10 Otherwise, we may simply be promoting the use of interventions that shift inpatient readmissions to observation unit or ED revisits, and there is little that is patient-centered or high value about that.9
Disclosures
The authors have nothing to disclose.
1. Smith M, Saunders R, Stuckhardt L, McGinnis JM, eds. Best care at lower cost: the path to continuously learning health care in America. Washington, DC: National Academies Press; 2013. PubMed
2. Nuckols TK, Fingar KR, Barrett ML, et al. Returns to emergency department, observation, or inpatient care within 30 days after hospitalization in 4 states, 2009 and 2010 versus 2013 and 2014. J Hosp Med. 2018;13(5):296-303. PubMed
3. Fingar KR, Washington R. Trends in Hospital Readmissions for Four High-Volume Conditions, 2009–2013. Statistical Brief No. 196. https://www.hcup-us.ahrq.gov/reports/statbriefs/sb196-Readmissions-Trends-High-Volume-Conditions.pdf. Accessed March 5, 2018.
4. Zuckerman RB, Sheingold SH, Orav EJ, Ruhter J, Epstein AM. Readmissions, observation, and the Hospital Readmissions Reduction Program. N Engl J Med. 2016;374(16):1543-1551. DOI: 10.1056/NEJMsa1513024. PubMed
5. Gerhardt G, Yemane A, Apostle K, Oelschlaeger A, Rollins E, Brennan N. Evaluating whether changes in utilization of hospital outpatient services contributed to lower Medicare readmission rate. Medicare Medicaid Res Rev. 2014;4(1). DOI: 10.5600/mmrr2014-004-01-b03 PubMed
6. Kangovi S, Cafardi SG, Smith RA, Kulkarni R, Grande D. Patient financial responsibility for observation care. J Hosp Med. 2015;10(11):718-723. DOI: 10.1002/jhm.2436. PubMed
7. The Hospital Observation Care Problem: Perspectives and Solutions from the Society of Hospital Medicine. Society of Hospital Medicine Public Policy Committee. https://www.hospitalmedicine.org/globalassets/policy-and-advocacy/advocacy-pdf/shms-observation-white-paper-2017. Accessed February 12, 2018.
8. Rosen AK, Chen Q, Shwartz M, et al. Does use of a hospital-wide readmission measure versus condition-specific readmission measures make a difference for hospital profiling and payment penalties? Medical Care. 2016;54(2):155-161. DOI: 10.1097/MLR.0000000000000455. PubMed
9. Baugh CW, Schuur JD. Observation care-high-value care or a cost-shifting loophole? N Engl J Med. 2013;369(4):302-305. DOI: 10.1056/NEJMp1304493. PubMed
10. Cassel CK, Conway PH, Delbanco SF, Jha AK, Saunders RS, Lee TH. Getting more performance from performance measurement. N Engl J Med. 2014;371(23):2145-2147. DOI: 10.1056/NEJMp1408345. PubMed
1. Smith M, Saunders R, Stuckhardt L, McGinnis JM, eds. Best care at lower cost: the path to continuously learning health care in America. Washington, DC: National Academies Press; 2013. PubMed
2. Nuckols TK, Fingar KR, Barrett ML, et al. Returns to emergency department, observation, or inpatient care within 30 days after hospitalization in 4 states, 2009 and 2010 versus 2013 and 2014. J Hosp Med. 2018;13(5):296-303. PubMed
3. Fingar KR, Washington R. Trends in Hospital Readmissions for Four High-Volume Conditions, 2009–2013. Statistical Brief No. 196. https://www.hcup-us.ahrq.gov/reports/statbriefs/sb196-Readmissions-Trends-High-Volume-Conditions.pdf. Accessed March 5, 2018.
4. Zuckerman RB, Sheingold SH, Orav EJ, Ruhter J, Epstein AM. Readmissions, observation, and the Hospital Readmissions Reduction Program. N Engl J Med. 2016;374(16):1543-1551. DOI: 10.1056/NEJMsa1513024. PubMed
5. Gerhardt G, Yemane A, Apostle K, Oelschlaeger A, Rollins E, Brennan N. Evaluating whether changes in utilization of hospital outpatient services contributed to lower Medicare readmission rate. Medicare Medicaid Res Rev. 2014;4(1). DOI: 10.5600/mmrr2014-004-01-b03 PubMed
6. Kangovi S, Cafardi SG, Smith RA, Kulkarni R, Grande D. Patient financial responsibility for observation care. J Hosp Med. 2015;10(11):718-723. DOI: 10.1002/jhm.2436. PubMed
7. The Hospital Observation Care Problem: Perspectives and Solutions from the Society of Hospital Medicine. Society of Hospital Medicine Public Policy Committee. https://www.hospitalmedicine.org/globalassets/policy-and-advocacy/advocacy-pdf/shms-observation-white-paper-2017. Accessed February 12, 2018.
8. Rosen AK, Chen Q, Shwartz M, et al. Does use of a hospital-wide readmission measure versus condition-specific readmission measures make a difference for hospital profiling and payment penalties? Medical Care. 2016;54(2):155-161. DOI: 10.1097/MLR.0000000000000455. PubMed
9. Baugh CW, Schuur JD. Observation care-high-value care or a cost-shifting loophole? N Engl J Med. 2013;369(4):302-305. DOI: 10.1056/NEJMp1304493. PubMed
10. Cassel CK, Conway PH, Delbanco SF, Jha AK, Saunders RS, Lee TH. Getting more performance from performance measurement. N Engl J Med. 2014;371(23):2145-2147. DOI: 10.1056/NEJMp1408345. PubMed
© 2018 Society of Hospital Medicine
Reducing SNF Readmissions: At What Cost?
The landscape of postacute care in skilled nursing facilities (SNFs) in the United States is evolving. As the population ages, a growing number of elderly persons are being discharged to SNFs at an enormous cost and with clear evidence of disappointing outcomes. The reaction to these trends includes payment reforms that “bundle” hospital and postacute care, act as incentives to discourage SNFs, or penalize SNFs for undesired patient outcomes. Hospitalists are expected to increasingly feel the effect of these reforms.1
Thus, hospitals are demonstrating renewed interest in reducing readmissions from SNFs. In this issue of Journal of Hospital Medicine, Rosen and colleagues present the results of the Enhanced Care Program (ECP), a multicomponent intervention consisting of 9 nurse practitioners (NPs), a pharmacist, a pharmacy technician, a nurse educator, a program administrator, and a medical director.2 These providers are deployed to 8 SNFs around a large teaching hospital, providing direct clinical care as well as 24/7 call availability for enrolled patients, robust medication reconciliation, and monthly education for SNF nursing staff. A unique aspect of this model was that individual attending physicians in the associated SNFs could decide whether to enroll their patients in the model; patients not enrolled represented a contemporaneous control cohort. The authors found a nearly 30% reduction in the odds of 30-day readmission (OR 0.71 [0.60–0.85] after adjustment), which was robust to multiple sensitivity analyses, including a propensity-matched cohort comparison. The authors should be commended for working to mitigate these potential confounders, thereby strengthening their conclusions. Such a large reduction in readmissions reflects their high underlying prevalence (23% in the nonintervention cohort).
This report closely follows the evaluation of a similar program at the Cleveland Clinic called Connected Care Model (CCM), in which 4 physicians and 5 NPs or physician assistants provided care, including 24/7 call availability, in 7 associated SNFs.3 In a retrospective pre-post analysis comparing the 30-day readmission rates of these SNFs with those of others in the network, similar reductions in readmissions were observed. ECP and CCM represent important extensions of a much larger body of evidence, from the Evercare model4 to the Initiative to Reduce Avoidable Hospitalizations demonstration project, which suggests that adding NPs to nursing homes reduces hospitalizations.5
However, several factors have to be considered before disseminating ECP or CCM. First, other promising “proof of concept” quality improvement studies were not efficacious when rigorously tested in nursing homes.6 Second, these programs are representative of large academic medical centers, which may establish different relationships with different SNFs compared with smaller or less well-resourced hospitals. As the Initiative to Reduce Hospitalizations demonstrated, even a fundamentally similar intervention can have extremely different results depending on the nursing homes involved,5 and the science behind establishing effective hospital–SNF partnerships is still in its infancy.7 Third, both studies have significant methodological limitations, including most importantly that they are conducted within SNFs selected to be part of their hospitals’ network.
These significant early efforts also present an opportunity to reconsider the underlying assumption of these models: that adding more supervisory clinicians to SNFs is the right approach to reduce hospitalizations. Although adding resources is an attractive “plug and play” solution for many problems in healthcare delivery, placing only 1 NP in each of the 15,583 certified nursing facilities in the United States would employ fully 10% of the entire NP workforce. Amid rising concerns about costs related to our aging population, these interventions face substantial headwinds toward becoming the standard of care without demonstrating cost effectiveness. Furthermore, many SNF directors might suggest that hospitals and hospitalists working with them to address fundamental (but much more intransigent) problems in SNFs, such as high staff turnover, low concentration of highly skilled staff (RNs and MDs), regulatory burden, and hospitals using SNFs like stepdown units, could represent a generalizable and sustainable solution.
We realize that this argument is tricky for hospitalists because its underlying logic (care has become too complex, patients are too sick, and dedicated personnel are needed) also played a major role in establishing our existence. One possibility is that like hospitalists, NPs and a growing cadre of “SNFists” will become major drivers of quality improvement, education, and leadership locally at these facilities, thereby leading to sustainable change.8 Similarly, current conditions may drive recognition that a specific set of skills is required to function effectively in the SNF environment,9 just as we believe hospitalists need unique skills to excel in today’s hospital environment.
Studies such as that of Rosen et al. are valuable for JHM because they prompt us to recognize that we as hospitalists have much to share and learn from nursing homes and the dedicated practitioners who work there. In fact, we argue that few places in the healthcare system are more in need of innovation than hospital–nursing home relationships, and hospitalists do not just have a vested clinical interest; in many ways, we see a mirror of our own development as a “specialty.” We encourage hospitals and hospitalists to take up this challenge on behalf of some of the most vulnerable patients in our system during critical times in their care trajectory. As the Commission for Long-Term Care (www.ltccommission.org) wrote in its final report to Congress: “The need is great. The time to act is now.”
Disclosures
Dr. Burke is supported by a VA Health Services Research and Development (HSR&D) Career Development Award. All opinions are those of the authors and do not necessarily represent those of the Department of Veterans Affairs. Dr. Greysen has nothing to disclose.
1. Burke RE, Cumbler E, Coleman EA, Levy C. Post-acute care reform: Implications and opportunities for hospitalists. J Hosp Med. 2017;12(1):46-51. 10.3810/hp.2012.02.958. PubMed
2. Rosen BT, Halbert RJ, Hart K, Diniz MA, Isonaka S, Black JT. The enhanced care program: Impact of a care transitions program on 30-day hospital readmissions for patients discharged from an acute care facility to skilled nursing facilities. J Hosp Med. 2018;13(4):229-235.
3. Rothberg MB. Impact of a connected care model on 30-day readmission rates from skilled nursing facilities. J Hosp Med. 2017;12(4):238-244. 10.12788/jhm.2710. PubMed
4. Kane RL, Keckhafer G, Flood S, Bershadsky B, Siadaty MS. The effect of Evercare on hospital use. J Am Geriatr Soc. 2003;51(10):1427-1434. 10.1046/j.1532-5415.2003.51461.x. PubMed
5. Ingber MJ, Feng Z, Khatutsky G, et al. Initiative to reduce avoidable hospitalizations among nursing facility residents shows promising results. Health Aff Proj Hope. 2017;36(3):441-450. 10.1377/hlthaff.2016.1310. PubMed
6. Kane RL, Huckfeldt P, Tappen R, et al. Effects of an intervention to reduce hospitalizations from nursing homes: A randomized implementation trial of the INTERACT Program. JAMA Intern Med. 2017;177(9):1257-1264. 10.1001/jamainternmed.2017.2657. PubMed
7. Lage DE, Rusinak D, Carr D, Grabowski DC, Ackerly DC. Creating a network of high-quality skilled nursing facilities: preliminary data on the postacute care quality improvement experiences of an accountable care organization. J Am Geriatr Soc. 2015;63(4):804-808. 10.1111/jgs.13351. PubMed
8. Ryskina KL, Polsky D, Werner RM. Physicians and advanced practitioners specializing in nursing home care. JAMA. 2017;318(20):2040-2042. 10.1001/jama.2017.13378. PubMed
9. Gillespie SM, Levy CR, Katz PR. What exactly is an “SNF-ist?” JAMA Intern Med. 2018;178(1):153-154. 10.1001/jamainternmed.2017.7212. PubMed
The landscape of postacute care in skilled nursing facilities (SNFs) in the United States is evolving. As the population ages, a growing number of elderly persons are being discharged to SNFs at an enormous cost and with clear evidence of disappointing outcomes. The reaction to these trends includes payment reforms that “bundle” hospital and postacute care, act as incentives to discourage SNFs, or penalize SNFs for undesired patient outcomes. Hospitalists are expected to increasingly feel the effect of these reforms.1
Thus, hospitals are demonstrating renewed interest in reducing readmissions from SNFs. In this issue of Journal of Hospital Medicine, Rosen and colleagues present the results of the Enhanced Care Program (ECP), a multicomponent intervention consisting of 9 nurse practitioners (NPs), a pharmacist, a pharmacy technician, a nurse educator, a program administrator, and a medical director.2 These providers are deployed to 8 SNFs around a large teaching hospital, providing direct clinical care as well as 24/7 call availability for enrolled patients, robust medication reconciliation, and monthly education for SNF nursing staff. A unique aspect of this model was that individual attending physicians in the associated SNFs could decide whether to enroll their patients in the model; patients not enrolled represented a contemporaneous control cohort. The authors found a nearly 30% reduction in the odds of 30-day readmission (OR 0.71 [0.60–0.85] after adjustment), which was robust to multiple sensitivity analyses, including a propensity-matched cohort comparison. The authors should be commended for working to mitigate these potential confounders, thereby strengthening their conclusions. Such a large reduction in readmissions reflects their high underlying prevalence (23% in the nonintervention cohort).
This report closely follows the evaluation of a similar program at the Cleveland Clinic called Connected Care Model (CCM), in which 4 physicians and 5 NPs or physician assistants provided care, including 24/7 call availability, in 7 associated SNFs.3 In a retrospective pre-post analysis comparing the 30-day readmission rates of these SNFs with those of others in the network, similar reductions in readmissions were observed. ECP and CCM represent important extensions of a much larger body of evidence, from the Evercare model4 to the Initiative to Reduce Avoidable Hospitalizations demonstration project, which suggests that adding NPs to nursing homes reduces hospitalizations.5
However, several factors have to be considered before disseminating ECP or CCM. First, other promising “proof of concept” quality improvement studies were not efficacious when rigorously tested in nursing homes.6 Second, these programs are representative of large academic medical centers, which may establish different relationships with different SNFs compared with smaller or less well-resourced hospitals. As the Initiative to Reduce Hospitalizations demonstrated, even a fundamentally similar intervention can have extremely different results depending on the nursing homes involved,5 and the science behind establishing effective hospital–SNF partnerships is still in its infancy.7 Third, both studies have significant methodological limitations, including most importantly that they are conducted within SNFs selected to be part of their hospitals’ network.
These significant early efforts also present an opportunity to reconsider the underlying assumption of these models: that adding more supervisory clinicians to SNFs is the right approach to reduce hospitalizations. Although adding resources is an attractive “plug and play” solution for many problems in healthcare delivery, placing only 1 NP in each of the 15,583 certified nursing facilities in the United States would employ fully 10% of the entire NP workforce. Amid rising concerns about costs related to our aging population, these interventions face substantial headwinds toward becoming the standard of care without demonstrating cost effectiveness. Furthermore, many SNF directors might suggest that hospitals and hospitalists working with them to address fundamental (but much more intransigent) problems in SNFs, such as high staff turnover, low concentration of highly skilled staff (RNs and MDs), regulatory burden, and hospitals using SNFs like stepdown units, could represent a generalizable and sustainable solution.
We realize that this argument is tricky for hospitalists because its underlying logic (care has become too complex, patients are too sick, and dedicated personnel are needed) also played a major role in establishing our existence. One possibility is that like hospitalists, NPs and a growing cadre of “SNFists” will become major drivers of quality improvement, education, and leadership locally at these facilities, thereby leading to sustainable change.8 Similarly, current conditions may drive recognition that a specific set of skills is required to function effectively in the SNF environment,9 just as we believe hospitalists need unique skills to excel in today’s hospital environment.
Studies such as that of Rosen et al. are valuable for JHM because they prompt us to recognize that we as hospitalists have much to share and learn from nursing homes and the dedicated practitioners who work there. In fact, we argue that few places in the healthcare system are more in need of innovation than hospital–nursing home relationships, and hospitalists do not just have a vested clinical interest; in many ways, we see a mirror of our own development as a “specialty.” We encourage hospitals and hospitalists to take up this challenge on behalf of some of the most vulnerable patients in our system during critical times in their care trajectory. As the Commission for Long-Term Care (www.ltccommission.org) wrote in its final report to Congress: “The need is great. The time to act is now.”
Disclosures
Dr. Burke is supported by a VA Health Services Research and Development (HSR&D) Career Development Award. All opinions are those of the authors and do not necessarily represent those of the Department of Veterans Affairs. Dr. Greysen has nothing to disclose.
The landscape of postacute care in skilled nursing facilities (SNFs) in the United States is evolving. As the population ages, a growing number of elderly persons are being discharged to SNFs at an enormous cost and with clear evidence of disappointing outcomes. The reaction to these trends includes payment reforms that “bundle” hospital and postacute care, act as incentives to discourage SNFs, or penalize SNFs for undesired patient outcomes. Hospitalists are expected to increasingly feel the effect of these reforms.1
Thus, hospitals are demonstrating renewed interest in reducing readmissions from SNFs. In this issue of Journal of Hospital Medicine, Rosen and colleagues present the results of the Enhanced Care Program (ECP), a multicomponent intervention consisting of 9 nurse practitioners (NPs), a pharmacist, a pharmacy technician, a nurse educator, a program administrator, and a medical director.2 These providers are deployed to 8 SNFs around a large teaching hospital, providing direct clinical care as well as 24/7 call availability for enrolled patients, robust medication reconciliation, and monthly education for SNF nursing staff. A unique aspect of this model was that individual attending physicians in the associated SNFs could decide whether to enroll their patients in the model; patients not enrolled represented a contemporaneous control cohort. The authors found a nearly 30% reduction in the odds of 30-day readmission (OR 0.71 [0.60–0.85] after adjustment), which was robust to multiple sensitivity analyses, including a propensity-matched cohort comparison. The authors should be commended for working to mitigate these potential confounders, thereby strengthening their conclusions. Such a large reduction in readmissions reflects their high underlying prevalence (23% in the nonintervention cohort).
This report closely follows the evaluation of a similar program at the Cleveland Clinic called Connected Care Model (CCM), in which 4 physicians and 5 NPs or physician assistants provided care, including 24/7 call availability, in 7 associated SNFs.3 In a retrospective pre-post analysis comparing the 30-day readmission rates of these SNFs with those of others in the network, similar reductions in readmissions were observed. ECP and CCM represent important extensions of a much larger body of evidence, from the Evercare model4 to the Initiative to Reduce Avoidable Hospitalizations demonstration project, which suggests that adding NPs to nursing homes reduces hospitalizations.5
However, several factors have to be considered before disseminating ECP or CCM. First, other promising “proof of concept” quality improvement studies were not efficacious when rigorously tested in nursing homes.6 Second, these programs are representative of large academic medical centers, which may establish different relationships with different SNFs compared with smaller or less well-resourced hospitals. As the Initiative to Reduce Hospitalizations demonstrated, even a fundamentally similar intervention can have extremely different results depending on the nursing homes involved,5 and the science behind establishing effective hospital–SNF partnerships is still in its infancy.7 Third, both studies have significant methodological limitations, including most importantly that they are conducted within SNFs selected to be part of their hospitals’ network.
These significant early efforts also present an opportunity to reconsider the underlying assumption of these models: that adding more supervisory clinicians to SNFs is the right approach to reduce hospitalizations. Although adding resources is an attractive “plug and play” solution for many problems in healthcare delivery, placing only 1 NP in each of the 15,583 certified nursing facilities in the United States would employ fully 10% of the entire NP workforce. Amid rising concerns about costs related to our aging population, these interventions face substantial headwinds toward becoming the standard of care without demonstrating cost effectiveness. Furthermore, many SNF directors might suggest that hospitals and hospitalists working with them to address fundamental (but much more intransigent) problems in SNFs, such as high staff turnover, low concentration of highly skilled staff (RNs and MDs), regulatory burden, and hospitals using SNFs like stepdown units, could represent a generalizable and sustainable solution.
We realize that this argument is tricky for hospitalists because its underlying logic (care has become too complex, patients are too sick, and dedicated personnel are needed) also played a major role in establishing our existence. One possibility is that like hospitalists, NPs and a growing cadre of “SNFists” will become major drivers of quality improvement, education, and leadership locally at these facilities, thereby leading to sustainable change.8 Similarly, current conditions may drive recognition that a specific set of skills is required to function effectively in the SNF environment,9 just as we believe hospitalists need unique skills to excel in today’s hospital environment.
Studies such as that of Rosen et al. are valuable for JHM because they prompt us to recognize that we as hospitalists have much to share and learn from nursing homes and the dedicated practitioners who work there. In fact, we argue that few places in the healthcare system are more in need of innovation than hospital–nursing home relationships, and hospitalists do not just have a vested clinical interest; in many ways, we see a mirror of our own development as a “specialty.” We encourage hospitals and hospitalists to take up this challenge on behalf of some of the most vulnerable patients in our system during critical times in their care trajectory. As the Commission for Long-Term Care (www.ltccommission.org) wrote in its final report to Congress: “The need is great. The time to act is now.”
Disclosures
Dr. Burke is supported by a VA Health Services Research and Development (HSR&D) Career Development Award. All opinions are those of the authors and do not necessarily represent those of the Department of Veterans Affairs. Dr. Greysen has nothing to disclose.
1. Burke RE, Cumbler E, Coleman EA, Levy C. Post-acute care reform: Implications and opportunities for hospitalists. J Hosp Med. 2017;12(1):46-51. 10.3810/hp.2012.02.958. PubMed
2. Rosen BT, Halbert RJ, Hart K, Diniz MA, Isonaka S, Black JT. The enhanced care program: Impact of a care transitions program on 30-day hospital readmissions for patients discharged from an acute care facility to skilled nursing facilities. J Hosp Med. 2018;13(4):229-235.
3. Rothberg MB. Impact of a connected care model on 30-day readmission rates from skilled nursing facilities. J Hosp Med. 2017;12(4):238-244. 10.12788/jhm.2710. PubMed
4. Kane RL, Keckhafer G, Flood S, Bershadsky B, Siadaty MS. The effect of Evercare on hospital use. J Am Geriatr Soc. 2003;51(10):1427-1434. 10.1046/j.1532-5415.2003.51461.x. PubMed
5. Ingber MJ, Feng Z, Khatutsky G, et al. Initiative to reduce avoidable hospitalizations among nursing facility residents shows promising results. Health Aff Proj Hope. 2017;36(3):441-450. 10.1377/hlthaff.2016.1310. PubMed
6. Kane RL, Huckfeldt P, Tappen R, et al. Effects of an intervention to reduce hospitalizations from nursing homes: A randomized implementation trial of the INTERACT Program. JAMA Intern Med. 2017;177(9):1257-1264. 10.1001/jamainternmed.2017.2657. PubMed
7. Lage DE, Rusinak D, Carr D, Grabowski DC, Ackerly DC. Creating a network of high-quality skilled nursing facilities: preliminary data on the postacute care quality improvement experiences of an accountable care organization. J Am Geriatr Soc. 2015;63(4):804-808. 10.1111/jgs.13351. PubMed
8. Ryskina KL, Polsky D, Werner RM. Physicians and advanced practitioners specializing in nursing home care. JAMA. 2017;318(20):2040-2042. 10.1001/jama.2017.13378. PubMed
9. Gillespie SM, Levy CR, Katz PR. What exactly is an “SNF-ist?” JAMA Intern Med. 2018;178(1):153-154. 10.1001/jamainternmed.2017.7212. PubMed
1. Burke RE, Cumbler E, Coleman EA, Levy C. Post-acute care reform: Implications and opportunities for hospitalists. J Hosp Med. 2017;12(1):46-51. 10.3810/hp.2012.02.958. PubMed
2. Rosen BT, Halbert RJ, Hart K, Diniz MA, Isonaka S, Black JT. The enhanced care program: Impact of a care transitions program on 30-day hospital readmissions for patients discharged from an acute care facility to skilled nursing facilities. J Hosp Med. 2018;13(4):229-235.
3. Rothberg MB. Impact of a connected care model on 30-day readmission rates from skilled nursing facilities. J Hosp Med. 2017;12(4):238-244. 10.12788/jhm.2710. PubMed
4. Kane RL, Keckhafer G, Flood S, Bershadsky B, Siadaty MS. The effect of Evercare on hospital use. J Am Geriatr Soc. 2003;51(10):1427-1434. 10.1046/j.1532-5415.2003.51461.x. PubMed
5. Ingber MJ, Feng Z, Khatutsky G, et al. Initiative to reduce avoidable hospitalizations among nursing facility residents shows promising results. Health Aff Proj Hope. 2017;36(3):441-450. 10.1377/hlthaff.2016.1310. PubMed
6. Kane RL, Huckfeldt P, Tappen R, et al. Effects of an intervention to reduce hospitalizations from nursing homes: A randomized implementation trial of the INTERACT Program. JAMA Intern Med. 2017;177(9):1257-1264. 10.1001/jamainternmed.2017.2657. PubMed
7. Lage DE, Rusinak D, Carr D, Grabowski DC, Ackerly DC. Creating a network of high-quality skilled nursing facilities: preliminary data on the postacute care quality improvement experiences of an accountable care organization. J Am Geriatr Soc. 2015;63(4):804-808. 10.1111/jgs.13351. PubMed
8. Ryskina KL, Polsky D, Werner RM. Physicians and advanced practitioners specializing in nursing home care. JAMA. 2017;318(20):2040-2042. 10.1001/jama.2017.13378. PubMed
9. Gillespie SM, Levy CR, Katz PR. What exactly is an “SNF-ist?” JAMA Intern Med. 2018;178(1):153-154. 10.1001/jamainternmed.2017.7212. PubMed
© 2018 Society of Hospital Medicine
Use of Smartphones and Mobile Devices
Over 90% of Americans own mobile phones, and their use for internet access is rising rapidly (31% in 2009, 63% in 2013).[1] This has prompted growth in mobile health (mHealth) programs for outpatient settings,[2] and similar growth is anticipated for inpatient settings.[3] Hospitals and the healthcare systems they operate within are increasingly tied to patient experience scores (eg, Hospital Consumer Assessment of Healthcare Providers and Systems, Press Ganey) for both reputation and reimbursement.[4, 5] As a result, hospitals will need to invest future resources in a consumer‐facing digital experience. Despite these trends, basic information on mobile device ownership and usage by hospitalized patients is limited. This knowledge is needed to guide successful mHealth approaches to engage patients in acute care settings.
METHODS
We administered a 27‐question survey about mobile device use to all adult inpatients at a large urban California teaching hospital over 2 dates (October 27, 2013 and November 11, 2013) to create a cross‐sectional view of mobile device use at a hospital that offers free wireless Internet (WiFi) and personal health records (Internet‐accessible individualized medical records). Average census was 447, and we excluded patients for: age under 18 years (98), admission for neurological problems (75), altered mental status (35), nonEnglish speaking (30), or unavailable if patients were not in their room after 2 attempts spaced 30 to 60 minutes apart (36), leaving 173 eligible. We performed descriptive statistics and unadjusted associations ([2] test) to explore patterns of mobile device use.
RESULTS
We enrolled 152 patients (88% response rate): 77 (51%) male, average age 53 years (1992 years), 84 (56%) white, 115 (75%) with Medicare or commercial insurance. We found 85 (56%) patients brought a smartphone, and 82/85 (95%) used it during their hospital stay. Additionally, 41 (27%) patients brought a tablet, and 29 (19%) brought a laptop; usage was 37/41 (90%) for tablets and 24/29 (83%) for laptops. One hundred three (68%) patients brought at least 1 mobile computing device (smartphone, tablet, laptop) during their hospital stay. Overall device usage was highest among oncology patients (85%) and lowest among medicine patients (54%) (Table 1). Device usage also varied by age (<65 years old: 79% vs 65 years old: 27%), insurance status (private/Medicare: 70% vs Medicaid/other: 59%), and race/ethnicity (white: 73% vs non‐white: 62%), although only age was statistically significant (P<0.01; all others >0.05).
Total, N=152 | Medicine, n=39 | Surgery, n=47 | Oncology, n=34 | All Others, n=32* | |
---|---|---|---|---|---|
| |||||
Demographics | |||||
Average age, y (range) | 53.2 (1992) | 55.7 (2092) | 51.7 (1979) | 51.2 (2377) | 53.9 (2584) |
Medicare or commercial insurance | 75% (115) | 64% (25) | 87% (41) | 76% (26) | 72% (23) |
Medicaid, other, or no insurance | 25% (37) | 36% (14) | 13% (6) | 24% (8) | 28% (9) |
Non‐white race/ethnicity | 44% (68) | 56% (22) | 36% (17) | 38% (13) | 50%(16) |
Female gender | 49% (75) | 49% (19) | 45% (21) | 47% (16) | 59% (19) |
Device ownership/usage | |||||
Own smartphone | 62% (94) | 54% (21) | 66% (31) | 74% (25) | 53% (17) |
Brought smartphone | 55% (83) | 41% (16) | 60% (28) | 71% (24) | 48% (15) |
Brought laptop | 19% (29) | 18% (7) | 11% (5) | 41% (14) | 10% (3) |
Brought tablet | 27% (41) | 18% (7) | 26% (12) | 50% (17) | 16% (5) |
Brought 1 above devices | 68% (103) | 54% (21) | 68% (32) | 85% (29) | 68% (21) |
Ever used an app | 63% (95) | 51% (20) | 72% (34) | 79% (27) | 45% (14) |
Ever used an app for health purposes | 22% (34) | 18% (7) | 21% (10) | 24% (8) | 29% (9) |
Accessed PHR with mobile device | 31% (47) | 26% (10) | 26% (12) | 47% (16) | 29% (9) |
Of the patients with mobile devices (smartphone, tablet, laptop), 97/103 (94%) used them during their hospitalization and for a wide array of activities (Figure 1): 47/97 (48%) accessed their personal health record (PHR), and most of these patients (38/47, 81%) reported this improved their inpatient experience. Additionally, 43/97 (44%) patients used their mobile devices to search for information about doctors, conditions, or treatments; most of these patients (39/43, 91%) used Google to search for this information, and most 29/43 (67%) felt this information made them more confident in their care.
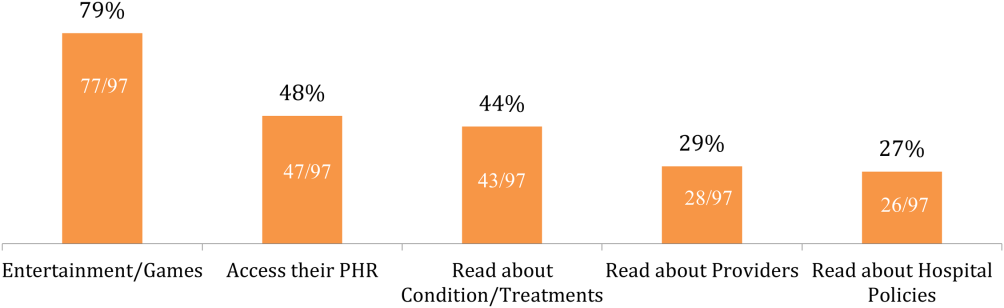
COMMENT
Over two‐thirds of patients in our study brought and used 1 or more mobile devices to the hospital. Despite this level of engagement with mobile devices, relatively few inpatients used their device to access their online PHR, which suggests information technology access is not the leading barrier to PHR access or mHealth engagement during hospitalization. In light of growing patient enthusiasm for PHRs,[6, 7] this represents an untapped opportunity to deliver personalized, patient‐centered care at the hospital bedside.
We also found that among the patients who did access their PHR on their mobile device, the vast majority (38/47, 81%) felt it improved their inpatient experience. Our PHR provides information such as test results and medications, but our survey suggests a number of patients look for health information, such as patient education tools, medication references, and provider information, outside of the PHR. For those patients, 29/43 (67%) felt these health‐related searches improved their experience. Although we did not ask patients why they used Web searches outside their PHR, we believe this suggests that patients desire more information than currently available via the PHR. Although this information might be difficult to incorporate into the PHR, at minimum, hospitals could develop mobile applications to provide patients with basic information about their providers and conditions. Beyond this, hospitals could develop or adopt mobile applications that align with strategic priorities such as improved physician‐provider communication, reduced hospital readmissions, and improved accuracy of medication reconciliation.
Our study has limitations. First, although we used a cross‐sectional, point‐in‐time approach to canvas the entire adult population in our hospital on 2 separate dates, our study was limited to 1 large urban hospital in California; device ownership and usage may vary in other settings. Second, although our hospital provides free WiFi, we did not assess whether patients experienced any connectivity issues that influenced their device usage patterns. Finally, we did not explore questions of access, ownership, and usage of mobile computing devices for family and friends who visited inpatients in our study. These questions are ripe for future research in this emerging area of mHeath.
In summary, our study suggests a role for hospitals to provide universal WiFi access to patients, and a role for both hospitals and healthcare providers to promote digital health programs. Our findings on mobile device use in the hospital are consistent with the growing popularity of mobile device usage nationwide. Patients are increasingly wired for new opportunities to both engage in their care and optimize their hospital experience through use of their mobile computing devices. Hospitals and providers should explore this potential for engagement, but may need to explore local trends in usage to target specific service lines and patient populations given differences in access and use.
Acknowledgements
The authors acknowledge contributions by Christina Quist, MD, and Emily Gottenborg, MD, who assisted in data collection.
Disclosures: Data from this project were presented at the 2014 Annual Scientific Meeting of the Society of Hospital Medicine, March 25, 2014 in Las Vegas, Nevada. The authors have no conflicts of interest to declare relative to this study. Dr. Ludwin, MD had full access to all data in the study and takes responsibility for the integrity of the data and the accuracy of the data analysis. This project by Drs. Ludwin and Greysen was supported by grants from the University of California, San Francisco (UCSF) Partners in Care (Ronald Rankin Award) and the UCSF Mount Zion Health Fund. Dr. Greysen is also funded by a Pilot Award for Junior Investigators in Digital Health from the UCSF Dean's Office, Research Evaluation and Allocation Committee (REAC). Additionally, Dr. Greysen receives career development support from the National Institutes of Health (NIH)National Institute of Aging (NIA) through the Claude D. Pepper Older Americans Independence Center at UCSF Division of Geriatric Medicine (#P30AG021342 NIH/NIA), a Career Development Award (1K23AG045338‐01), and the NIH‐NIA Loan Repayment Program.
- Device ownership over time. Pew Research Center. Available at: http://www.pewinternet.org/data‐trend/mobile/device‐ownership. Accessed April 3, 2014.
- The effectiveness of mobile‐health technologies to improve health care service delivery processes: a systematic review and meta‐analysis. PLoS Med. 2013;10(1):e1001363. , , , et al.
- Can mobile health technologies transform health care? JAMA. 2013;310(22):2395–2396. , , .
- Look ahead to succeed under VBP. Hosp Case Manag. 2014;22(7):92–93.
- The relationship between commercial website ratings and traditional hospital performance measures in the USA. BMJ Qual Saf. 2013;22(3):194–202. , , , .
- Consumers' perceptions of patient‐accessible electronic medical records. J Med Internet Res. 2013;15(8):e168. , , , , .
- Access, interest, and attitudes toward electronic communication for health care among patients in the medical safety net. J Gen Intern Med. 2013;28(7):914–920. , , , et al.
Over 90% of Americans own mobile phones, and their use for internet access is rising rapidly (31% in 2009, 63% in 2013).[1] This has prompted growth in mobile health (mHealth) programs for outpatient settings,[2] and similar growth is anticipated for inpatient settings.[3] Hospitals and the healthcare systems they operate within are increasingly tied to patient experience scores (eg, Hospital Consumer Assessment of Healthcare Providers and Systems, Press Ganey) for both reputation and reimbursement.[4, 5] As a result, hospitals will need to invest future resources in a consumer‐facing digital experience. Despite these trends, basic information on mobile device ownership and usage by hospitalized patients is limited. This knowledge is needed to guide successful mHealth approaches to engage patients in acute care settings.
METHODS
We administered a 27‐question survey about mobile device use to all adult inpatients at a large urban California teaching hospital over 2 dates (October 27, 2013 and November 11, 2013) to create a cross‐sectional view of mobile device use at a hospital that offers free wireless Internet (WiFi) and personal health records (Internet‐accessible individualized medical records). Average census was 447, and we excluded patients for: age under 18 years (98), admission for neurological problems (75), altered mental status (35), nonEnglish speaking (30), or unavailable if patients were not in their room after 2 attempts spaced 30 to 60 minutes apart (36), leaving 173 eligible. We performed descriptive statistics and unadjusted associations ([2] test) to explore patterns of mobile device use.
RESULTS
We enrolled 152 patients (88% response rate): 77 (51%) male, average age 53 years (1992 years), 84 (56%) white, 115 (75%) with Medicare or commercial insurance. We found 85 (56%) patients brought a smartphone, and 82/85 (95%) used it during their hospital stay. Additionally, 41 (27%) patients brought a tablet, and 29 (19%) brought a laptop; usage was 37/41 (90%) for tablets and 24/29 (83%) for laptops. One hundred three (68%) patients brought at least 1 mobile computing device (smartphone, tablet, laptop) during their hospital stay. Overall device usage was highest among oncology patients (85%) and lowest among medicine patients (54%) (Table 1). Device usage also varied by age (<65 years old: 79% vs 65 years old: 27%), insurance status (private/Medicare: 70% vs Medicaid/other: 59%), and race/ethnicity (white: 73% vs non‐white: 62%), although only age was statistically significant (P<0.01; all others >0.05).
Total, N=152 | Medicine, n=39 | Surgery, n=47 | Oncology, n=34 | All Others, n=32* | |
---|---|---|---|---|---|
| |||||
Demographics | |||||
Average age, y (range) | 53.2 (1992) | 55.7 (2092) | 51.7 (1979) | 51.2 (2377) | 53.9 (2584) |
Medicare or commercial insurance | 75% (115) | 64% (25) | 87% (41) | 76% (26) | 72% (23) |
Medicaid, other, or no insurance | 25% (37) | 36% (14) | 13% (6) | 24% (8) | 28% (9) |
Non‐white race/ethnicity | 44% (68) | 56% (22) | 36% (17) | 38% (13) | 50%(16) |
Female gender | 49% (75) | 49% (19) | 45% (21) | 47% (16) | 59% (19) |
Device ownership/usage | |||||
Own smartphone | 62% (94) | 54% (21) | 66% (31) | 74% (25) | 53% (17) |
Brought smartphone | 55% (83) | 41% (16) | 60% (28) | 71% (24) | 48% (15) |
Brought laptop | 19% (29) | 18% (7) | 11% (5) | 41% (14) | 10% (3) |
Brought tablet | 27% (41) | 18% (7) | 26% (12) | 50% (17) | 16% (5) |
Brought 1 above devices | 68% (103) | 54% (21) | 68% (32) | 85% (29) | 68% (21) |
Ever used an app | 63% (95) | 51% (20) | 72% (34) | 79% (27) | 45% (14) |
Ever used an app for health purposes | 22% (34) | 18% (7) | 21% (10) | 24% (8) | 29% (9) |
Accessed PHR with mobile device | 31% (47) | 26% (10) | 26% (12) | 47% (16) | 29% (9) |
Of the patients with mobile devices (smartphone, tablet, laptop), 97/103 (94%) used them during their hospitalization and for a wide array of activities (Figure 1): 47/97 (48%) accessed their personal health record (PHR), and most of these patients (38/47, 81%) reported this improved their inpatient experience. Additionally, 43/97 (44%) patients used their mobile devices to search for information about doctors, conditions, or treatments; most of these patients (39/43, 91%) used Google to search for this information, and most 29/43 (67%) felt this information made them more confident in their care.
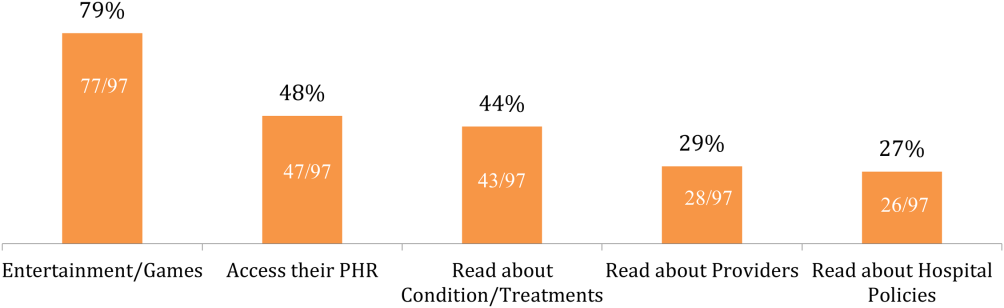
COMMENT
Over two‐thirds of patients in our study brought and used 1 or more mobile devices to the hospital. Despite this level of engagement with mobile devices, relatively few inpatients used their device to access their online PHR, which suggests information technology access is not the leading barrier to PHR access or mHealth engagement during hospitalization. In light of growing patient enthusiasm for PHRs,[6, 7] this represents an untapped opportunity to deliver personalized, patient‐centered care at the hospital bedside.
We also found that among the patients who did access their PHR on their mobile device, the vast majority (38/47, 81%) felt it improved their inpatient experience. Our PHR provides information such as test results and medications, but our survey suggests a number of patients look for health information, such as patient education tools, medication references, and provider information, outside of the PHR. For those patients, 29/43 (67%) felt these health‐related searches improved their experience. Although we did not ask patients why they used Web searches outside their PHR, we believe this suggests that patients desire more information than currently available via the PHR. Although this information might be difficult to incorporate into the PHR, at minimum, hospitals could develop mobile applications to provide patients with basic information about their providers and conditions. Beyond this, hospitals could develop or adopt mobile applications that align with strategic priorities such as improved physician‐provider communication, reduced hospital readmissions, and improved accuracy of medication reconciliation.
Our study has limitations. First, although we used a cross‐sectional, point‐in‐time approach to canvas the entire adult population in our hospital on 2 separate dates, our study was limited to 1 large urban hospital in California; device ownership and usage may vary in other settings. Second, although our hospital provides free WiFi, we did not assess whether patients experienced any connectivity issues that influenced their device usage patterns. Finally, we did not explore questions of access, ownership, and usage of mobile computing devices for family and friends who visited inpatients in our study. These questions are ripe for future research in this emerging area of mHeath.
In summary, our study suggests a role for hospitals to provide universal WiFi access to patients, and a role for both hospitals and healthcare providers to promote digital health programs. Our findings on mobile device use in the hospital are consistent with the growing popularity of mobile device usage nationwide. Patients are increasingly wired for new opportunities to both engage in their care and optimize their hospital experience through use of their mobile computing devices. Hospitals and providers should explore this potential for engagement, but may need to explore local trends in usage to target specific service lines and patient populations given differences in access and use.
Acknowledgements
The authors acknowledge contributions by Christina Quist, MD, and Emily Gottenborg, MD, who assisted in data collection.
Disclosures: Data from this project were presented at the 2014 Annual Scientific Meeting of the Society of Hospital Medicine, March 25, 2014 in Las Vegas, Nevada. The authors have no conflicts of interest to declare relative to this study. Dr. Ludwin, MD had full access to all data in the study and takes responsibility for the integrity of the data and the accuracy of the data analysis. This project by Drs. Ludwin and Greysen was supported by grants from the University of California, San Francisco (UCSF) Partners in Care (Ronald Rankin Award) and the UCSF Mount Zion Health Fund. Dr. Greysen is also funded by a Pilot Award for Junior Investigators in Digital Health from the UCSF Dean's Office, Research Evaluation and Allocation Committee (REAC). Additionally, Dr. Greysen receives career development support from the National Institutes of Health (NIH)National Institute of Aging (NIA) through the Claude D. Pepper Older Americans Independence Center at UCSF Division of Geriatric Medicine (#P30AG021342 NIH/NIA), a Career Development Award (1K23AG045338‐01), and the NIH‐NIA Loan Repayment Program.
Over 90% of Americans own mobile phones, and their use for internet access is rising rapidly (31% in 2009, 63% in 2013).[1] This has prompted growth in mobile health (mHealth) programs for outpatient settings,[2] and similar growth is anticipated for inpatient settings.[3] Hospitals and the healthcare systems they operate within are increasingly tied to patient experience scores (eg, Hospital Consumer Assessment of Healthcare Providers and Systems, Press Ganey) for both reputation and reimbursement.[4, 5] As a result, hospitals will need to invest future resources in a consumer‐facing digital experience. Despite these trends, basic information on mobile device ownership and usage by hospitalized patients is limited. This knowledge is needed to guide successful mHealth approaches to engage patients in acute care settings.
METHODS
We administered a 27‐question survey about mobile device use to all adult inpatients at a large urban California teaching hospital over 2 dates (October 27, 2013 and November 11, 2013) to create a cross‐sectional view of mobile device use at a hospital that offers free wireless Internet (WiFi) and personal health records (Internet‐accessible individualized medical records). Average census was 447, and we excluded patients for: age under 18 years (98), admission for neurological problems (75), altered mental status (35), nonEnglish speaking (30), or unavailable if patients were not in their room after 2 attempts spaced 30 to 60 minutes apart (36), leaving 173 eligible. We performed descriptive statistics and unadjusted associations ([2] test) to explore patterns of mobile device use.
RESULTS
We enrolled 152 patients (88% response rate): 77 (51%) male, average age 53 years (1992 years), 84 (56%) white, 115 (75%) with Medicare or commercial insurance. We found 85 (56%) patients brought a smartphone, and 82/85 (95%) used it during their hospital stay. Additionally, 41 (27%) patients brought a tablet, and 29 (19%) brought a laptop; usage was 37/41 (90%) for tablets and 24/29 (83%) for laptops. One hundred three (68%) patients brought at least 1 mobile computing device (smartphone, tablet, laptop) during their hospital stay. Overall device usage was highest among oncology patients (85%) and lowest among medicine patients (54%) (Table 1). Device usage also varied by age (<65 years old: 79% vs 65 years old: 27%), insurance status (private/Medicare: 70% vs Medicaid/other: 59%), and race/ethnicity (white: 73% vs non‐white: 62%), although only age was statistically significant (P<0.01; all others >0.05).
Total, N=152 | Medicine, n=39 | Surgery, n=47 | Oncology, n=34 | All Others, n=32* | |
---|---|---|---|---|---|
| |||||
Demographics | |||||
Average age, y (range) | 53.2 (1992) | 55.7 (2092) | 51.7 (1979) | 51.2 (2377) | 53.9 (2584) |
Medicare or commercial insurance | 75% (115) | 64% (25) | 87% (41) | 76% (26) | 72% (23) |
Medicaid, other, or no insurance | 25% (37) | 36% (14) | 13% (6) | 24% (8) | 28% (9) |
Non‐white race/ethnicity | 44% (68) | 56% (22) | 36% (17) | 38% (13) | 50%(16) |
Female gender | 49% (75) | 49% (19) | 45% (21) | 47% (16) | 59% (19) |
Device ownership/usage | |||||
Own smartphone | 62% (94) | 54% (21) | 66% (31) | 74% (25) | 53% (17) |
Brought smartphone | 55% (83) | 41% (16) | 60% (28) | 71% (24) | 48% (15) |
Brought laptop | 19% (29) | 18% (7) | 11% (5) | 41% (14) | 10% (3) |
Brought tablet | 27% (41) | 18% (7) | 26% (12) | 50% (17) | 16% (5) |
Brought 1 above devices | 68% (103) | 54% (21) | 68% (32) | 85% (29) | 68% (21) |
Ever used an app | 63% (95) | 51% (20) | 72% (34) | 79% (27) | 45% (14) |
Ever used an app for health purposes | 22% (34) | 18% (7) | 21% (10) | 24% (8) | 29% (9) |
Accessed PHR with mobile device | 31% (47) | 26% (10) | 26% (12) | 47% (16) | 29% (9) |
Of the patients with mobile devices (smartphone, tablet, laptop), 97/103 (94%) used them during their hospitalization and for a wide array of activities (Figure 1): 47/97 (48%) accessed their personal health record (PHR), and most of these patients (38/47, 81%) reported this improved their inpatient experience. Additionally, 43/97 (44%) patients used their mobile devices to search for information about doctors, conditions, or treatments; most of these patients (39/43, 91%) used Google to search for this information, and most 29/43 (67%) felt this information made them more confident in their care.
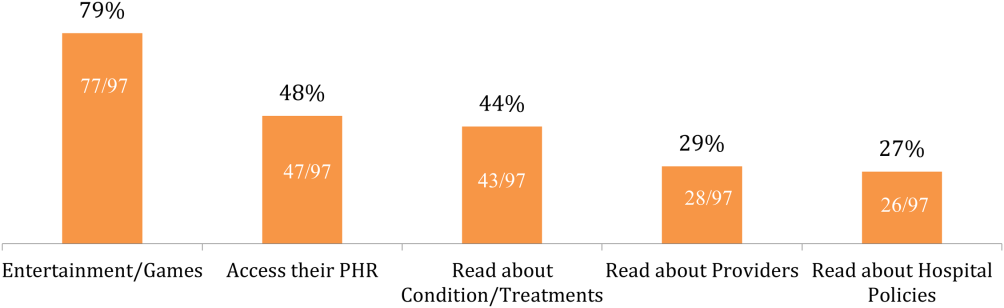
COMMENT
Over two‐thirds of patients in our study brought and used 1 or more mobile devices to the hospital. Despite this level of engagement with mobile devices, relatively few inpatients used their device to access their online PHR, which suggests information technology access is not the leading barrier to PHR access or mHealth engagement during hospitalization. In light of growing patient enthusiasm for PHRs,[6, 7] this represents an untapped opportunity to deliver personalized, patient‐centered care at the hospital bedside.
We also found that among the patients who did access their PHR on their mobile device, the vast majority (38/47, 81%) felt it improved their inpatient experience. Our PHR provides information such as test results and medications, but our survey suggests a number of patients look for health information, such as patient education tools, medication references, and provider information, outside of the PHR. For those patients, 29/43 (67%) felt these health‐related searches improved their experience. Although we did not ask patients why they used Web searches outside their PHR, we believe this suggests that patients desire more information than currently available via the PHR. Although this information might be difficult to incorporate into the PHR, at minimum, hospitals could develop mobile applications to provide patients with basic information about their providers and conditions. Beyond this, hospitals could develop or adopt mobile applications that align with strategic priorities such as improved physician‐provider communication, reduced hospital readmissions, and improved accuracy of medication reconciliation.
Our study has limitations. First, although we used a cross‐sectional, point‐in‐time approach to canvas the entire adult population in our hospital on 2 separate dates, our study was limited to 1 large urban hospital in California; device ownership and usage may vary in other settings. Second, although our hospital provides free WiFi, we did not assess whether patients experienced any connectivity issues that influenced their device usage patterns. Finally, we did not explore questions of access, ownership, and usage of mobile computing devices for family and friends who visited inpatients in our study. These questions are ripe for future research in this emerging area of mHeath.
In summary, our study suggests a role for hospitals to provide universal WiFi access to patients, and a role for both hospitals and healthcare providers to promote digital health programs. Our findings on mobile device use in the hospital are consistent with the growing popularity of mobile device usage nationwide. Patients are increasingly wired for new opportunities to both engage in their care and optimize their hospital experience through use of their mobile computing devices. Hospitals and providers should explore this potential for engagement, but may need to explore local trends in usage to target specific service lines and patient populations given differences in access and use.
Acknowledgements
The authors acknowledge contributions by Christina Quist, MD, and Emily Gottenborg, MD, who assisted in data collection.
Disclosures: Data from this project were presented at the 2014 Annual Scientific Meeting of the Society of Hospital Medicine, March 25, 2014 in Las Vegas, Nevada. The authors have no conflicts of interest to declare relative to this study. Dr. Ludwin, MD had full access to all data in the study and takes responsibility for the integrity of the data and the accuracy of the data analysis. This project by Drs. Ludwin and Greysen was supported by grants from the University of California, San Francisco (UCSF) Partners in Care (Ronald Rankin Award) and the UCSF Mount Zion Health Fund. Dr. Greysen is also funded by a Pilot Award for Junior Investigators in Digital Health from the UCSF Dean's Office, Research Evaluation and Allocation Committee (REAC). Additionally, Dr. Greysen receives career development support from the National Institutes of Health (NIH)National Institute of Aging (NIA) through the Claude D. Pepper Older Americans Independence Center at UCSF Division of Geriatric Medicine (#P30AG021342 NIH/NIA), a Career Development Award (1K23AG045338‐01), and the NIH‐NIA Loan Repayment Program.
- Device ownership over time. Pew Research Center. Available at: http://www.pewinternet.org/data‐trend/mobile/device‐ownership. Accessed April 3, 2014.
- The effectiveness of mobile‐health technologies to improve health care service delivery processes: a systematic review and meta‐analysis. PLoS Med. 2013;10(1):e1001363. , , , et al.
- Can mobile health technologies transform health care? JAMA. 2013;310(22):2395–2396. , , .
- Look ahead to succeed under VBP. Hosp Case Manag. 2014;22(7):92–93.
- The relationship between commercial website ratings and traditional hospital performance measures in the USA. BMJ Qual Saf. 2013;22(3):194–202. , , , .
- Consumers' perceptions of patient‐accessible electronic medical records. J Med Internet Res. 2013;15(8):e168. , , , , .
- Access, interest, and attitudes toward electronic communication for health care among patients in the medical safety net. J Gen Intern Med. 2013;28(7):914–920. , , , et al.
- Device ownership over time. Pew Research Center. Available at: http://www.pewinternet.org/data‐trend/mobile/device‐ownership. Accessed April 3, 2014.
- The effectiveness of mobile‐health technologies to improve health care service delivery processes: a systematic review and meta‐analysis. PLoS Med. 2013;10(1):e1001363. , , , et al.
- Can mobile health technologies transform health care? JAMA. 2013;310(22):2395–2396. , , .
- Look ahead to succeed under VBP. Hosp Case Manag. 2014;22(7):92–93.
- The relationship between commercial website ratings and traditional hospital performance measures in the USA. BMJ Qual Saf. 2013;22(3):194–202. , , , .
- Consumers' perceptions of patient‐accessible electronic medical records. J Med Internet Res. 2013;15(8):e168. , , , , .
- Access, interest, and attitudes toward electronic communication for health care among patients in the medical safety net. J Gen Intern Med. 2013;28(7):914–920. , , , et al.
Mobility Sensors for Hospital Patients
Functional impairment, such as difficulty with activities of daily living or limited mobility,[1] is common among hospitalized patients and correlated with important outcomes: approximately 50% of hospitalized Medicare seniors have some level of impairment that correlates with higher rates of readmission,[2] long‐term care placement,[3] and even death.[4]
Lack of consistent, accurate, and reliable data on functional mobility during hospitalization poses an important barrier for programs seeking to improve functional outcomes in hospitalized patients.[5, 6] More accurate mobility data could improve current hospital practices to diagnose mobility problems, target mobility interventions, and measure interventions' effectiveness. Although wearable mobility sensors (small, wireless accelerometers placed on patients' wrists, ankles, or waists) hold promise in overcoming these barriers and improving current practice, existing data are from small samples of focused populations and have not integrated sensor data into patient care.[7, 8]
In this issue of the Journal of Hospital Medicine, Sallis and colleagues used mobility sensors to study 777 hospitalized patients.[9] This article has several strengths that make it unique among the handful of articles in this area: it is the largest to date, the first to consider patients on both medical and surgical units, and the first to correlate sensor data with clinical assessments of mobility by providers (nurses). The authors found that, regardless of length of stay, patients averaged 1100 steps during the final 24 hours of their hospitalization. Older patients had slightly fewer steps on average (982 per 24 hours), but, taken collectively, these findings led the authors to postulate that 1000 steps per day might be a good normative value for discharge readiness in terms of patient mobility.
This idea of a normative value for steps taken by inpatients prior to discharge raises several interesting questions. First, could numbers of steps become a value that hospital providers routinely use to optimize care of hospitalized patients similar to other values such as blood pressure or blood sugar? Such a threshold could be used to define strategies that target tight mobility control for patients at high risk for decline, and others might be managed with a more traditional ad lib approach. Alternatively, perhaps physicians should focus more on improvement in mobility regardless of a population‐defined threshold. In this case, the measure would be progress toward a patient‐centered or patient‐defined goal. Second, it is important to note that Sallis and colleagues found that patients whose nurses documented their estimated mobility more frequently in the medical record also had substantially higher sensor step counts. This raises the question of whether more data from sensors can assist front‐line inpatient providers to more effectively engage patients in mobilizing to avoid functional deconditioning during hospitalization. Often we tell our patients to try to get out of bed todaygo for a walk around the unit, but we are rarely specific about how far they should walk, and patients do not get feedback on their daily progress toward a specific mobility goal. Perhaps data on the number of steps from mobility sensors could be shown to both patients and providers so as to encourage patients to reach their goal, whether that is the normative 1000 steps per day or slightly more or less.
This article also has limitations, which raise important questions for future research. First, patients in this study were ambulatory and relatively healthy (85% had Charlson scores 0 or 1) at the time of admission, making it difficult to determine whether the approach used or threshold defined are valid in higher‐risk populations, such as those with preexisting functional limitations. Second, lack of clinical outcomes data is another important limitation in this study, which is shared by many, but not all, inpatient sensor studies. For example, a recent study correlated discharge location (skilled nursing facility vs home) to levels of step mobility; however, the authors were unable to determine the degree to which their step measures were simply mirroring clinical decision making.[10] Another recent study demonstrated that decreased inpatient step counts are associated with early mortality; however, more proximal outcomes such as postdischarge function were not measured.[11] Moreover, future studies will need to assess whether mobility sensors can reliably predict postdischarge function, and even be used to improve mobility or reduce functional impairment in hospital populations that include sicker patients.
Ultimately, the results by Sallis et al. are a useful step in the right direction, but much more work is needed to determine the clinical utility of mobility sensors as part of larger efforts to harness the potential of mobile health (mHealth) efforts to improve care for hospitalized patients.[12] The future of mobility sensors in healthcare is likely about how well patients and providers can use them to successfully guide and support behavior change. This will require a strong health‐adopter focus in coaching patients to use mobility sensors and their mobile, patient‐facing applications.[13] Ultimately, the goal must be to embed these mHealth approaches into larger behavior management and health system redesign so that clinical goals such as improved function after hospital discharge are met.[14]
Disclosures
Nothing to report.
- Hospitalization‐associated disability: "She was probably able to ambulate, but I'm not sure." JAMA. 2011;306(16):1782–1793. , , .
- Functional impairment and readmissions in Medicare seniors [published online ahead of print February 2, 2015]. JAMA Intern Med. doi: 10.1001/jamainternmed.2014.7756. , , , .
- Loss of independence in activities of daily living in older adults hospitalized with medical illnesses: increased vulnerability with age. J Am Geriatr Soc. 2003;51(4):451–458. , , , et al.
- Prediction of recovery, dependence or death in elders who become disabled during hospitalization. J Gen Intern Med. 2013;28(2):261–268. , , , et al.
- Functional status—an important but overlooked variable in the readmissions equation. J Hosp Med. 2014;9(5):330–331. , .
- Association of impaired functional status at hospital discharge and subsequent rehospitalization. J Hosp Med. 2014;9(5):277–282. , , , , , .
- The under‐recognized epidemic of low mobility during hospitalization of older adults. J Am Geriatr Soc. 2009;57(9):1660–1665. , , , .
- Twenty‐four‐hour mobility during acute hospitalization in older medical patients. J Gerontol A Biol Sci Med Sci. 2013;68(3):331–337. , , , et al.
- Stepping towards discharge: level of ambulation in hospitalized patients. J Hosp Med. 2015;10(6):358–363. , , , et al.
- Functional recovery in the elderly after major surgery: assessment of mobility recovery using wireless technology. Ann Thorac Surg. 2013;96(3):1057–1061. , , , , .
- Mobility activity and its value as a prognostic indicator of survival in hospitalized older adults. J Am Geriatr Soc. 2013;61(4):551–557. , , , et al.
- Wired. Available at: http://www.wired.com/2014/11/where‐fitness‐trackers‐fail. Published November 6, 2014. Accessed January 21, 2015. . Wearables are totally failing the people who need them most.
- Wearable devices as facilitators, not drivers, of health behavior change. JAMA. 2015;313(5):459–460. , , .
- Digital medical tools and sensors. JAMA. 2015;313(4):353–354. , , .
Functional impairment, such as difficulty with activities of daily living or limited mobility,[1] is common among hospitalized patients and correlated with important outcomes: approximately 50% of hospitalized Medicare seniors have some level of impairment that correlates with higher rates of readmission,[2] long‐term care placement,[3] and even death.[4]
Lack of consistent, accurate, and reliable data on functional mobility during hospitalization poses an important barrier for programs seeking to improve functional outcomes in hospitalized patients.[5, 6] More accurate mobility data could improve current hospital practices to diagnose mobility problems, target mobility interventions, and measure interventions' effectiveness. Although wearable mobility sensors (small, wireless accelerometers placed on patients' wrists, ankles, or waists) hold promise in overcoming these barriers and improving current practice, existing data are from small samples of focused populations and have not integrated sensor data into patient care.[7, 8]
In this issue of the Journal of Hospital Medicine, Sallis and colleagues used mobility sensors to study 777 hospitalized patients.[9] This article has several strengths that make it unique among the handful of articles in this area: it is the largest to date, the first to consider patients on both medical and surgical units, and the first to correlate sensor data with clinical assessments of mobility by providers (nurses). The authors found that, regardless of length of stay, patients averaged 1100 steps during the final 24 hours of their hospitalization. Older patients had slightly fewer steps on average (982 per 24 hours), but, taken collectively, these findings led the authors to postulate that 1000 steps per day might be a good normative value for discharge readiness in terms of patient mobility.
This idea of a normative value for steps taken by inpatients prior to discharge raises several interesting questions. First, could numbers of steps become a value that hospital providers routinely use to optimize care of hospitalized patients similar to other values such as blood pressure or blood sugar? Such a threshold could be used to define strategies that target tight mobility control for patients at high risk for decline, and others might be managed with a more traditional ad lib approach. Alternatively, perhaps physicians should focus more on improvement in mobility regardless of a population‐defined threshold. In this case, the measure would be progress toward a patient‐centered or patient‐defined goal. Second, it is important to note that Sallis and colleagues found that patients whose nurses documented their estimated mobility more frequently in the medical record also had substantially higher sensor step counts. This raises the question of whether more data from sensors can assist front‐line inpatient providers to more effectively engage patients in mobilizing to avoid functional deconditioning during hospitalization. Often we tell our patients to try to get out of bed todaygo for a walk around the unit, but we are rarely specific about how far they should walk, and patients do not get feedback on their daily progress toward a specific mobility goal. Perhaps data on the number of steps from mobility sensors could be shown to both patients and providers so as to encourage patients to reach their goal, whether that is the normative 1000 steps per day or slightly more or less.
This article also has limitations, which raise important questions for future research. First, patients in this study were ambulatory and relatively healthy (85% had Charlson scores 0 or 1) at the time of admission, making it difficult to determine whether the approach used or threshold defined are valid in higher‐risk populations, such as those with preexisting functional limitations. Second, lack of clinical outcomes data is another important limitation in this study, which is shared by many, but not all, inpatient sensor studies. For example, a recent study correlated discharge location (skilled nursing facility vs home) to levels of step mobility; however, the authors were unable to determine the degree to which their step measures were simply mirroring clinical decision making.[10] Another recent study demonstrated that decreased inpatient step counts are associated with early mortality; however, more proximal outcomes such as postdischarge function were not measured.[11] Moreover, future studies will need to assess whether mobility sensors can reliably predict postdischarge function, and even be used to improve mobility or reduce functional impairment in hospital populations that include sicker patients.
Ultimately, the results by Sallis et al. are a useful step in the right direction, but much more work is needed to determine the clinical utility of mobility sensors as part of larger efforts to harness the potential of mobile health (mHealth) efforts to improve care for hospitalized patients.[12] The future of mobility sensors in healthcare is likely about how well patients and providers can use them to successfully guide and support behavior change. This will require a strong health‐adopter focus in coaching patients to use mobility sensors and their mobile, patient‐facing applications.[13] Ultimately, the goal must be to embed these mHealth approaches into larger behavior management and health system redesign so that clinical goals such as improved function after hospital discharge are met.[14]
Disclosures
Nothing to report.
Functional impairment, such as difficulty with activities of daily living or limited mobility,[1] is common among hospitalized patients and correlated with important outcomes: approximately 50% of hospitalized Medicare seniors have some level of impairment that correlates with higher rates of readmission,[2] long‐term care placement,[3] and even death.[4]
Lack of consistent, accurate, and reliable data on functional mobility during hospitalization poses an important barrier for programs seeking to improve functional outcomes in hospitalized patients.[5, 6] More accurate mobility data could improve current hospital practices to diagnose mobility problems, target mobility interventions, and measure interventions' effectiveness. Although wearable mobility sensors (small, wireless accelerometers placed on patients' wrists, ankles, or waists) hold promise in overcoming these barriers and improving current practice, existing data are from small samples of focused populations and have not integrated sensor data into patient care.[7, 8]
In this issue of the Journal of Hospital Medicine, Sallis and colleagues used mobility sensors to study 777 hospitalized patients.[9] This article has several strengths that make it unique among the handful of articles in this area: it is the largest to date, the first to consider patients on both medical and surgical units, and the first to correlate sensor data with clinical assessments of mobility by providers (nurses). The authors found that, regardless of length of stay, patients averaged 1100 steps during the final 24 hours of their hospitalization. Older patients had slightly fewer steps on average (982 per 24 hours), but, taken collectively, these findings led the authors to postulate that 1000 steps per day might be a good normative value for discharge readiness in terms of patient mobility.
This idea of a normative value for steps taken by inpatients prior to discharge raises several interesting questions. First, could numbers of steps become a value that hospital providers routinely use to optimize care of hospitalized patients similar to other values such as blood pressure or blood sugar? Such a threshold could be used to define strategies that target tight mobility control for patients at high risk for decline, and others might be managed with a more traditional ad lib approach. Alternatively, perhaps physicians should focus more on improvement in mobility regardless of a population‐defined threshold. In this case, the measure would be progress toward a patient‐centered or patient‐defined goal. Second, it is important to note that Sallis and colleagues found that patients whose nurses documented their estimated mobility more frequently in the medical record also had substantially higher sensor step counts. This raises the question of whether more data from sensors can assist front‐line inpatient providers to more effectively engage patients in mobilizing to avoid functional deconditioning during hospitalization. Often we tell our patients to try to get out of bed todaygo for a walk around the unit, but we are rarely specific about how far they should walk, and patients do not get feedback on their daily progress toward a specific mobility goal. Perhaps data on the number of steps from mobility sensors could be shown to both patients and providers so as to encourage patients to reach their goal, whether that is the normative 1000 steps per day or slightly more or less.
This article also has limitations, which raise important questions for future research. First, patients in this study were ambulatory and relatively healthy (85% had Charlson scores 0 or 1) at the time of admission, making it difficult to determine whether the approach used or threshold defined are valid in higher‐risk populations, such as those with preexisting functional limitations. Second, lack of clinical outcomes data is another important limitation in this study, which is shared by many, but not all, inpatient sensor studies. For example, a recent study correlated discharge location (skilled nursing facility vs home) to levels of step mobility; however, the authors were unable to determine the degree to which their step measures were simply mirroring clinical decision making.[10] Another recent study demonstrated that decreased inpatient step counts are associated with early mortality; however, more proximal outcomes such as postdischarge function were not measured.[11] Moreover, future studies will need to assess whether mobility sensors can reliably predict postdischarge function, and even be used to improve mobility or reduce functional impairment in hospital populations that include sicker patients.
Ultimately, the results by Sallis et al. are a useful step in the right direction, but much more work is needed to determine the clinical utility of mobility sensors as part of larger efforts to harness the potential of mobile health (mHealth) efforts to improve care for hospitalized patients.[12] The future of mobility sensors in healthcare is likely about how well patients and providers can use them to successfully guide and support behavior change. This will require a strong health‐adopter focus in coaching patients to use mobility sensors and their mobile, patient‐facing applications.[13] Ultimately, the goal must be to embed these mHealth approaches into larger behavior management and health system redesign so that clinical goals such as improved function after hospital discharge are met.[14]
Disclosures
Nothing to report.
- Hospitalization‐associated disability: "She was probably able to ambulate, but I'm not sure." JAMA. 2011;306(16):1782–1793. , , .
- Functional impairment and readmissions in Medicare seniors [published online ahead of print February 2, 2015]. JAMA Intern Med. doi: 10.1001/jamainternmed.2014.7756. , , , .
- Loss of independence in activities of daily living in older adults hospitalized with medical illnesses: increased vulnerability with age. J Am Geriatr Soc. 2003;51(4):451–458. , , , et al.
- Prediction of recovery, dependence or death in elders who become disabled during hospitalization. J Gen Intern Med. 2013;28(2):261–268. , , , et al.
- Functional status—an important but overlooked variable in the readmissions equation. J Hosp Med. 2014;9(5):330–331. , .
- Association of impaired functional status at hospital discharge and subsequent rehospitalization. J Hosp Med. 2014;9(5):277–282. , , , , , .
- The under‐recognized epidemic of low mobility during hospitalization of older adults. J Am Geriatr Soc. 2009;57(9):1660–1665. , , , .
- Twenty‐four‐hour mobility during acute hospitalization in older medical patients. J Gerontol A Biol Sci Med Sci. 2013;68(3):331–337. , , , et al.
- Stepping towards discharge: level of ambulation in hospitalized patients. J Hosp Med. 2015;10(6):358–363. , , , et al.
- Functional recovery in the elderly after major surgery: assessment of mobility recovery using wireless technology. Ann Thorac Surg. 2013;96(3):1057–1061. , , , , .
- Mobility activity and its value as a prognostic indicator of survival in hospitalized older adults. J Am Geriatr Soc. 2013;61(4):551–557. , , , et al.
- Wired. Available at: http://www.wired.com/2014/11/where‐fitness‐trackers‐fail. Published November 6, 2014. Accessed January 21, 2015. . Wearables are totally failing the people who need them most.
- Wearable devices as facilitators, not drivers, of health behavior change. JAMA. 2015;313(5):459–460. , , .
- Digital medical tools and sensors. JAMA. 2015;313(4):353–354. , , .
- Hospitalization‐associated disability: "She was probably able to ambulate, but I'm not sure." JAMA. 2011;306(16):1782–1793. , , .
- Functional impairment and readmissions in Medicare seniors [published online ahead of print February 2, 2015]. JAMA Intern Med. doi: 10.1001/jamainternmed.2014.7756. , , , .
- Loss of independence in activities of daily living in older adults hospitalized with medical illnesses: increased vulnerability with age. J Am Geriatr Soc. 2003;51(4):451–458. , , , et al.
- Prediction of recovery, dependence or death in elders who become disabled during hospitalization. J Gen Intern Med. 2013;28(2):261–268. , , , et al.
- Functional status—an important but overlooked variable in the readmissions equation. J Hosp Med. 2014;9(5):330–331. , .
- Association of impaired functional status at hospital discharge and subsequent rehospitalization. J Hosp Med. 2014;9(5):277–282. , , , , , .
- The under‐recognized epidemic of low mobility during hospitalization of older adults. J Am Geriatr Soc. 2009;57(9):1660–1665. , , , .
- Twenty‐four‐hour mobility during acute hospitalization in older medical patients. J Gerontol A Biol Sci Med Sci. 2013;68(3):331–337. , , , et al.
- Stepping towards discharge: level of ambulation in hospitalized patients. J Hosp Med. 2015;10(6):358–363. , , , et al.
- Functional recovery in the elderly after major surgery: assessment of mobility recovery using wireless technology. Ann Thorac Surg. 2013;96(3):1057–1061. , , , , .
- Mobility activity and its value as a prognostic indicator of survival in hospitalized older adults. J Am Geriatr Soc. 2013;61(4):551–557. , , , et al.
- Wired. Available at: http://www.wired.com/2014/11/where‐fitness‐trackers‐fail. Published November 6, 2014. Accessed January 21, 2015. . Wearables are totally failing the people who need them most.
- Wearable devices as facilitators, not drivers, of health behavior change. JAMA. 2015;313(5):459–460. , , .
- Digital medical tools and sensors. JAMA. 2015;313(4):353–354. , , .
Hospital and Primary Care Collaboration
Poorly coordinated care between hospital and outpatient settings contributes to medical errors, poor outcomes, and high costs.[1, 2, 3] Recent policy has sought to motivate better care coordination after hospital discharge. Financial penalties for excessive hospital readmissionsa perceived marker of poorly coordinated carehave motivated hospitals to adopt transitional care programs to improve postdischarge care coordination.[4] However, the success of hospital‐initiated transitional care strategies in reducing hospital readmissions has been limited.[5] This may be due to the fact that many factors driving hospital readmissions, such as chronic medical illness, patient education, and availability of outpatient care, are outside of a hospital's control.[5, 6] Even among the most comprehensive hospital‐based transitional care intervention strategies, there is little evidence of active engagement of primary care providers or collaboration between hospitals and primary care practices in the transitional care planning process.[5] Better engagement of primary care into transitional care strategies may improve postdischarge care coordination.[7, 8]
The potential benefits of collaboration are particularly salient in healthcare safety nets.[9] The US health safety net is a patchwork of providers, funding, and programs unified by a shared missiondelivering care to patients regardless of ability to payrather than a coordinated system with shared governance.[9] Safety‐net hospitals are at risk for higher‐than‐average readmissions penalties.[10, 11] Medicaid expansion under the Affordable Care Act will likely increase demand for services in these settings, which could worsen fragmentation of care as a result of strained capacity.[12] Collaboration between hospitals and primary care clinics in the safety net could help overcome fragmentation, improve efficiencies in care, and reduce costs and readmissions.[12, 13, 14, 15]
Despite the potential benefits, we found no studies on how to enable collaboration between hospitals and primary care. We sought to understand systems‐level factors limiting and facilitating collaboration between hospitals and primary care practices around coordinating inpatient‐to‐outpatient care transitions by conducting a qualitative study, focusing on the perspective of primary care leaders in the safety net.
STUDY DATA AND METHODS
We conducted semistructured telephone interviews with primary care leaders in health safety nets across California from August 2012 through October 2012, prior to the implementation of the federal hospital readmissions penalties program. Primary care leaders were defined as clinicians or nonclinicians holding leadership positions, including chief executive officers, clinic medical directors, and local experts in care coordination or quality improvement. We defined safety‐net clinics as federally qualified health centers (FQHCs) and/or FQHC Look‐Alikes (clinics that meet eligibility requirements and receive the same benefits as FQHCs, except for Public Health Service Section 330 grants), community health centers, and public hospital‐affiliated clinics operating under a traditional fee‐for‐service model and serving a high proportion of Medicaid and uninsured patients.[9, 16] We defined public hospitals as government‐owned hospitals that provide care for individuals with limited access elsewhere.[17]
Sampling and Recruitment
We purposefully sampled participants to maximize diversity in geographic region, metropolitan status,[18] and type of county health delivery system to enable identification of common themes across different settings and contexts. Delivery systems were defined as per the Insure the Uninsured Project, a 501(c)(3) nonprofit organization that conducts research on the uninsured in California.[19] Provider systems are counties with a public hospital; payer systems are counties that contract with private hospitals to deliver uncompensated care in place of a public hospital; and County Medical Services Program is a state program that administers county health care in participating small counties, in lieu of a provider or payer system. We used the county delivery system type as a composite proxy of available county resources and market context given variations in funding, access, and eligibility by system type.
Participants were identified through online public directories, community clinic consortiums, and departments of public health websites. Additional participants were sought using snowball sampling. Potential participants were e‐mailed a recruitment letter describing the study, its purpose, topics to be covered, and confidentiality assurance. Participants who did not respond were called or e‐mailed within 1 week. When initial recruitment was unsuccessful, we attempted to recruit another participant within the same organization when possible. We recruited participants until reaching thematic saturation (i.e., no further new themes emerged from our interviews).[20] No participants were recruited through snowballing.
Data Collection and Interview Guides
We conducted in‐depth, semistructured interviews using interview guides informed by existing literature on collaboration and integration across healthcare systems[21, 22, 23] (see Supporting Information, Appendix 1, in the online version of this article). Interviews were digitally recorded and professionally transcribed verbatim.
We obtained contextual information for settings represented by each respondent, such as number of clinics and annual visits, through the California Primary Care Annual Utilization Data Report and clinic websites.[24]
Analysis
We employed thematic analysis[25] using an inductive framework to identify emergent and recurring themes. We developed and refined a coding template iteratively. Our multidisciplinary team included 2 general internists (O.K.N., L.E.G), 1 hospitalist (S.R.G.), a clinical nurse specialist with a doctorate in nursing (A.L.), and research staff with a public health background (J.K.). Two team members (O.K.N., J.K.) systematically coded all transcripts. Disagreements in coding were resolved through negotiated consensus. All investigators reviewed and discussed identified themes. We emailed summary findings to participants for confirmation to enhance the reliability of our findings.
The institutional review board at the University of California, San Francisco approved the study protocol.
RESULTS
Of 52 individuals contacted from 39 different organizations, 23 did not respond, 4 declined to participate, and 25 were scheduled for an interview. We interviewed 22 primary care leaders across 11 California counties (Table 1) and identified themes around factors influencing collaboration with hospitals (Table 2). Most respondents had prior positive experiences collaborating with hospitals on small, focused projects. However, they asserted the need for better hospitalclinic collaboration, and thought collaboration was critical to achieving high‐quality care transitions. We did not observe any differences in perspectives expressed by clinician versus nonclinician leaders. Nonparticipants were more likely than participants to be from northern rural or central counties, FQHCs, and smaller clinic settings.
| |
Leadership position | No. (%) |
Chief executive officer or equivalent* | 9 (41) |
Chief medical officer or medical director | 7 (32) |
Other | 6 (27) |
Clinical experience | |
Physician (MD or DO) | 15 (68) |
Registered nurse | 1 (5) |
Nonclinician | 6 (27) |
Clinic setting | |
Clinic type | |
FQHC and FQHC Look‐Alikes | 15 (68) |
Hospital based | 2 (9) |
Other | 5 (23) |
No. of clinics in system | |
14 | 9 (41) |
59 | 6 (27) |
10 | 7 (32) |
Annual no. of visits | |
<100,000 | 9 (41) |
100,000499,999 | 11 (50) |
500,000 | 2 (9) |
County characteristics | |
Health delivery system type | |
Provider | 13 (59) |
Payer | 2 (9) |
County Medical Services Program∥ | 7 (32) |
Rural county | 7 (32) |
Theme | Subtheme | Quote |
---|---|---|
| ||
Lack of institutional financial incentives for collaboration. | Collaboration may lead to increased responsibility without reimbursement for clinic. | Where the [payment] model breaks down is that the savings is only to the hospital; and there's an expectation on our part to go ahead and take on those additional patients. If that $400,000 savings doesn't at least have a portion to the team that's going to help keep the people out of the hospital, then it won't work. (Participant 17) |
Collaboration may lead to competition from the hospital for primary care patients. | Our biggest issues with working with the hospital[are] that we have a finite number of [Medicaid] patients [in our catchment area for whom] you get larger reimbursement. For a federally qualified health center, it is [crucial] to ensure we have a revenue stream that helps us take care of the uninsured. So you can see the natural kind of conflict when your pool of patients is very small. (Participant 10) | |
Collaboration may lead to increased financial risk for the hospital. | 70% to 80% of our adult patients have no insurance and the fact is that none of these hospitals want those patients. They do get disproportionate hospital savings and other thingsbut they don't have a strong business model when they have uninsured patients coming in their doors. That's just the reality. (Participant 21) | |
Collaboration may lead to decreased financial risk for the hospital. | Most of these patients either have very low reimbursement or no reimbursement, and so [the hospital doesn't] really want these people to end up in very expensive care because it's a burden on their systemphilosophically, everyone agrees that if we keep people well in the outpatient setting, that would be better for everyone. No, there is no financial incentive whatsoever for [the hospital] to not work with us. [emphasis added] (Participant 18) | |
Competing priorities limit primary care's ability to focus on care transitions. | I wouldn't say [improving care transitions is a high priority]. It's not because we don't want to do the job. We have other priorities. [T]he big issue is access. There's a massive demand for primary care in our communityand we're just trying to make sure we have enough capacity. [There are] requirements HRSA has been asking of health centers and other priorities. We're starting up a residency program. We're recruiting more doctors. We're upping our quality improvement processes internally. We're making a reinvestment in our [electronic medical record]. It never stops. (Participant 22) | |
The multitude of [care transitions and other quality] improvement imperatives makes it difficult to focus. It's not that any one of these things necessarily represents a flawed approach. It's just that when you have a variety of folks from the national, state, and local levels who all have different ideas about what constitutes appropriate improvement, it's very hard to respond to it all at once. (Participant 6) | ||
Mismatched expectations about the role and capacity of primary care in care transitions limit collaboration. | Perception of primary care being undervalued by hospitals as a key stakeholder in care transitions. | They just make sure the paperwork is set up.and they have it written down, See doctor in 7 days. And I think they [the hospitals] think that's where their responsibility stops. They don't actually look at our records or talk to us. (Participant 2) |
Perceived unrealistic expectations of primary care capacity to deliver postdischarge care. | [The hospital will] send anyone that's poor to us whether they are our patient or not. [T]hey say go to [our clinic] and they'll give you your outpatient medications. [But] we're at capacity. [W]e have a 79 month wait for a [new] primary care appointment. So then, we're stuck with the ethical dilemma of [do we send the patient back to the ER/hospital] for their medication or do we just [try to] take them in? (Participant 13) | |
The hospitals feel every undoctored patient must be ours. [But] it's not like we're sitting on our hands. We have more than enough patients. (Participant 22) | ||
Informal affiliations and partnerships, formed through personal relationships and interpersonal networking, facilitate collaboration. | Informal affiliations arise from existing personal relationships and/or interpersonal networking. | Our CEO [has been here] for the past 40 years, and has had very deep and ongoing relationships with the [hospital]. Those doors are very wide open. (Participant 18) |
Informal partnerships are particularly important for FQHCs. | As an FQHC we can't have any ties financially or politically, but there's a traditional connection. (Participant 2) | |
Increasing demands on clinical productivity lead to a loss of networking opportunities. | We're one of the few clinics that has their own inpatient service. I would say that the transitions between the hospital and [our] clinic start from a much higher level than anybody else. [However] we're about to close our hospital service. It's just too much work for our [clinic] doctors. (Participant 8) | |
There used to be a meeting once a month where quality improvement programs and issues were discussed. Our administration eliminated these in favor of productivity, to increase our numbers of patients seen. (Participant 12) | ||
Loss of relationships with hospital personnel amplifies challenges to collaboration. | Because the primary care docs are not visible in the hospital[quality improvement] projects [become] hospital‐based. Usually they forget that we exist. (Participant 11) | |
External funding and support can enable opportunities for networking and relationship building. | The [national stakeholder organization] has done a lot of work with us to bring us together and figure out what we're doing [across] different counties, settings, providers. (Participant 20) | |
Electronic health records enable collaboration by improving communication between hospitals and primary care. | Lack of timely communication between inpatient and outpatient settings is a major obstacle to postdischarge care coordination. | It's a lot of effort to get medical records back. It is often not timely. Patients are going to cycle in and out of more costly acute care because we don't know that it's happening. Communication between [outpatient and inpatient] facilities is one of the most challenging issues. (Participant 13) |
Optimism about potential of EHRs. | A lot of people are depending on [the EHR] to make a lot of communication changes [where there was] a disconnect in the past. (Participant 7) | |
Lack of EHR interoperability. | We have an EHR that's pieced together. The [emergency department] has their own [system]. The clinics have their own. The inpatient has their own. They're all electronic but they don't all talk to each other that well. (Participant 20) | |
Our system has reached our maximum capacity and we've had to rely on our community partners to see the overflow. [T]he difficult communication [is] magnified. (Participant 11) | ||
Privacy and legal concerns (nonuniform application of HIPAA standards). | There is a very different view from hospital to hospital about what it is they feel that they can share legally under HIPAA or not. It's a very strange thing and it almost depends more on the chief information officer at [each] hospital and less on what the [regulations] actually say. (Participant 21) | |
Yes, [the EHR] does communicate with the hospitals and the hospitals [communicate] back [with us]. [T]here are some technical issues, butthe biggest impediments to making the technology work are new issues around confidentiality and access. (Participant 17) | ||
Interpersonal contact is still needed even with robust EHRs. | I think [communication between systems is] getting better [due to the EHR], but there's still quite a few holes and a sense of the loop not being completely closed. It's like when you pick up the phoneyou don't want the automated system, you want to actually talk to somebody. (Participant 18) |
Lack of Institutional Financial Incentives for Collaboration
Primary care leaders felt that current reimbursement strategies rewarded hospitals for reducing readmissions rather than promoting shared savings with primary care. Seeking collaboration with hospitals would potentially increase clinic responsibility for postdischarge patient care without reimbursement for additional work.
In counties without public hospitals, leaders worried that collaboration with hospitals could lead to active loss of Medicaid patients from their practices. Developing closer relationships with local hospitals would enable those hospitals to redirect Medicaid patients to hospital‐owned primary care clinics, leading to a loss of important revenue and financial stability for their clinics.
A subset of these leaders also perceived that nonpublic hospitals were reluctant to collaborate with their clinics. They hypothesized that hospital leaders worried that collaborating with their primary care practices would lead to more uninsured patients at their hospitals, leading to an increase in uncompensated hospital care and reduced reimbursement. However, a second subset of leaders thought that nonpublic hospitals had increased financial incentives to collaborate with safety‐net clinics, because improved coordination with outpatient care could prevent uncompensated hospital care.
Competing Clinic Priorities Limit Primary Care Ability to Focus on Care Transitions
Clinic leaders struggled to balance competing priorities, including strained clinic capacity, regulatory/accreditation requirements, and financial strain. New patient‐centered medical home initiatives, which improve primary care financial incentives for postdischarge care coordination, were perceived as well intentioned but added to an overwhelming burden of ongoing quality improvement efforts.
Mismatched Expectations About the Role and Capacity of Primary Care in Care Transitions Limits Collaboration
Many leaders felt that hospitals undervalued the role of primary care as stakeholders in improving care transitions. They perceived that hospitals made little effort to directly contact primary care physicians about their patients' hospitalizations and discharges. Leaders were frustrated that hospitals had unrealistic expectations of primary care to deliver timely postdischarge care, given their strained capacity. Consequently, some were reluctant to seek opportunities to collaborate with hospitals to improve care transitions.
Informal Affiliations and Partnerships, Formed Through Personal Relationships and Interpersonal Networking, Facilitate Collaboration
Informal affiliations between hospitals and primary care clinics helped improve awareness of organizational roles and capacity and create a sense of shared mission, thus enabling collaboration in spite of other barriers. Such affiliations arose from existing, longstanding personal relationships and/or interpersonal networking between individual providers across settings. These informal affiliations were important for safety‐net clinics that were FQHCs or FQHC Look‐Alikes, because formal hospital affiliations are discouraged by federal regulations.[26]
Opportunities for building relationships and networking with hospital personnel arose when clinic physicians had hospital admitting privileges. This on‐site presence facilitated personal relationships and communication between clinic and hospital physicians, thus enabling better collaboration. However, increasing demands on outpatient clinical productivity often made a hospital presence infeasible. One health system promoted interpersonal networking through regular meetings between the clinic and the local hospital to foster collaboration on quality improvement and care delivery; however, clinical productivity demands ultimately took priority over these meetings. Although delegating inpatient care to hospitalists enabled clinics to maximize their productivity, it also decreased opportunities for networking, and consequently, clinic physicians felt their voices and opinions were not represented in improvement initiatives.
Outside funding and support, such as incentive programs and conferences sponsored by local health plans, clinic consortiums, or national stakeholder organizations, enabled the most successful networking. These successes were independent of whether the clinic staff rounded in the hospital.
Electronic Health Records Enable Collaboration By Improving Communication Between Hospitals And Primary Care
Challenges in communication and information flow were also challenges to collaboration with hospitals. No respondents reported receiving routine notification of patient hospitalizations at the time of admission. Many clinics were dedicating significant attention to implementing electronic health record (EHR) systems to receive financial incentives associated with meaningful use.[27] Implementation of EHRs helped mitigate issues with communication with hospitals, though to a lesser degree than expected. Clinics early in the process of EHR adoption were optimistic about the potential of EHRs to improve communication with hospitals. However, clinic leaders in settings with greater EHR experience were more guarded in their enthusiasm. They observed that lack of interoperability between clinic and hospital EHRs was a persistent and major issue in spite of meaningful use standards, limiting timely flow of information across settings. Even when hospitals and their associated clinics had integrated or interoperable EHRs (n=3), or were working toward EHR integration (n=5), the need to expand networks to include other community healthcare settings using different systems presented ongoing challenges to achieving seamless communication due to a lack of interoperability.
When information sharing was technically feasible, leaders noted that inconsistent understanding and application of privacy rules dictated by the Health Insurance Portability and Accountability Act (HIPAA) limited information sharing. The quality and types of information shared varied widely across settings, depending on how HIPAA regulations were interpreted.
Even with robust EHRs, interpersonal contact was still perceived as crucial to enabling collaboration. EHRs were perceived to help with information flow, but did not facilitate relationship building across settings.
DISCUSSION
We found that safety‐net primary care leaders identified several barriers to collaboration with hospitals: (1) lack of financial incentives for collaboration, (2) competing priorities, (3) mismatched expectations about the role and capacity of primary care, and (4) poor communication infrastructure. Interpersonal networking and use of EHRs helped overcome these obstacles to a limited extent.
Prior studies demonstrate that early follow‐up, timely communication, and continuity with primary care after hospital discharge are associated with improved postdischarge outcomes.[8, 28, 29, 30] Despite evidence that collaboration between primary care and hospitals may help optimize postdischarge outcomes, our study is the first to describe primary care leaders' perspectives on potential targets for improving collaboration between hospitals and primary care to improve care transitions.
Our results highlight the need to modify payment models to align financial incentives across settings for collaboration. Otherwise, it may be difficult for hospitals to engage primary care in collaborative efforts to improve care transitions. Recent pilot payment models aim to motivate improved postdischarge care coordination. The Centers for Medicare and Medicaid Services implemented two new Current Procedural Terminology Transitional Care Management codes to enable reimbursement of outpatient physicians for management of patients transitioning from the hospital to the community. This model does not require communication between accepting (outpatient) and discharging (hospital) physicians or other hospital staff.[31] Another pilot program pays primary care clinics $6 per beneficiary per month if they become level 3 patient‐centered medical homes, which have stringent requirements for communication and coordination with hospitals for postdischarge care.[32] Capitated payment models, such as expansion of Medicaid managed care, and shared‐savings models, such accountable care organizations, aim to promote shared responsibility between hospitals and primary care by creating financial incentives to prevent hospitalizations through effective use of outpatient resources. The effectiveness of these strategies to improve care transitions is not yet established.
Many tout the adoption of EHRs as a means to improve communication and collaboration across settings.[33] However, policies narrowly focused on EHR adoption fail to address broader issues regarding lack of EHR interoperability and inconsistently applied privacy regulations under HIPAA, which were substantial barriers to information sharing. Stage 2 meaningful use criteria will address some interoperability issues by implementing standards for exchange of laboratory data and summary care records for care transitions.[34] Additional regulatory policies should promote uniform application of privacy regulations to enable more fluid sharing of electronic data across various healthcare settings. Locally and regionally negotiated data sharing agreements, as well as arrangements such as regional health information exchanges, could temporize these issues until broader policies are enacted.
EHRs did not obviate the need for meaningful interpersonal communication between providers. Hospital‐based quality improvement teams could create networking opportunities to foster relationship‐building and communication across settings. Leadership should consider scheduling protected time to facilitate attendance. Colocation of outpatient staff, such as nurse coordinators and office managers, in the hospital may also improve relationship building and care coordination.[35] Such measures would bridge the perceived divide between inpatient and outpatient care, and create avenues to find mutually beneficial solutions to improving postdischarge care transitions.[36]
Our results should be interpreted in light of several limitations. This study focused on primary care practices in the California safety net; given variations in safety nets across different contexts, the transferability of our findings may be limited. Second, rural perspectives were relatively under‐represented in our study sample; there may be additional unidentified issues specific to rural areas or specific to other nonparticipants that may have not been captured in this study. For this hypothesis‐generating study, we focused on the perspectives of primary care leaders. Triangulating perspectives of other stakeholders, including hospital leadership, mental health, social services, and payer organizations, will offer a more comprehensive analysis of barriers and enablers to hospitalprimary care collaboration. We were unable to collect data on the payer mix of each facility, which may influence the perceived financial barriers to collaboration among facilities. However, we anticipate that the broader theme of lack of financial incentives for collaboration will resonate across many settings, as collaboration between inpatient and outpatient providers in general has been largely unfunded by payers.[37, 38, 39] Further, most primary care providers (PCPs) in and outside of safety‐net settings operate on slim margins that cannot support additional time by PCPs or staff to coordinate care transitions.[39, 40] Because our study was completed prior to the implementation of several new payment models motivating postdischarge care coordination, we were unable to assess their effect on clinics' collaboration with hospitals.
In conclusion, efforts to improve collaboration between clinical settings around postdischarge care transitions will require targeted policy and quality improvement efforts in 3 specific areas. Policy makers and administrators with the power to negotiate payment schemes and regulatory policies should first align financial incentives across settings to support postdischarge transitions and care coordination, and second, improve EHR interoperability and uniform application of HIPAA regulations. Third, clinic and hospital leaders, and front‐line providers should enhance opportunities for interpersonal networking between providers in hospital and primary care settings. With the expansion of insurance coverage and increased demand for primary care in the safety net and other settings, policies to promote care coordination should consider the perspective of both hospital and clinic incentives and mechanisms for coordinating care across settings.
Disclosures
Preliminary results from this study were presented at the Society of General Internal Medicine 36th Annual Meeting in Denver, Colorado, April 2013. Dr. Nguyen's work on this project was funded by a federal training grant from the National Research Service Award (NRSA T32HP19025‐07‐00). Dr. Goldman is the recipient of grants from the Agency for Health Care Research and Quality (K08 HS018090‐01). Drs. Goldman, Greysen, and Lyndon are supported by the National Institutes of Health, National Center for Research Resources, Office of the Director (UCSF‐CTSI grant no. KL2 RR024130). The authors report no conflicts of interest.
- Crossing the Quality Chasm: A New Health System for the 21st Century. Washington, DC: The National Academies Press; 2001.
- Deficits in communication and information transfer between hospital‐based and primary care physicians: implications for patient safety and continuity of care. JAMA. 2007;297(8):831–841. , , , , , .
- Medical errors related to discontinuity of care from an inpatient to an outpatient setting. J Gen Intern Med. 2003;18(8):646–651. , , , .
- Medicare Payment Advisory Commission. Report to the Congress: Promoting Greater Efficiency in Medicare. Washington, DC: Medicare Payment Advisory Commission; 2007.
- Hospital‐initiated transitional care interventions as a patient safety strategy: a systematic review. Ann Intern Med. 2013;158(5 pt 2):433–440. , , , , , .
- Thirty‐day readmission rates for Medicare beneficiaries by race and site of care. JAMA. 2011;305(7):675–681. , , .
- Improving care transitions: hospitalists partnering with primary care. J Hosp Med. 2010;5(7):375–377. , .
- Primary care physician communication at hospital discharge reduces medication discrepancies. J Hosp Med. 2013;8(12):672–677. , , , , .
- Institute of Medicine. America's Health Care Safety Net: Intact but Endangered. Washington, DC: Institute of Medicine; 2000.
- Higher readmissions at safety‐net hospitals and potential policy solutions. Issue Brief (Commonw Fund). 2012;34:1–16. , .
- Characteristics of hospitals receiving penalties under the hospital readmissions reduction program. JAMA. 2013;309(4):342–343. , .
- Ensuring Equity: A Post‐Reform Framework to Achieve High Performance Health Care for Vulnerable Populations. New York, NY: The Commonwealth Fund; 2011. , , , et al.
- Enhancing the Capacity of Community Centers to Achieve High Performance: Findings from the 2009 Commonwealth Fund National Survey of Federally Qualified Health Centers. New York, NY: The Commonwealth Fund; 2010. , , , , .
- Integration mechanisms and hospital efficiency in integrated health care delivery systems. J Med Syst. 2002;26(2):127–143. , , .
- Effect of physician collaboration network on hospitalization cost and readmission rate. Eur J Public Health. 2012;22(5):629–633. , , .
- Health Resources and Services Administration. Health Center Look‐Alikes Program. Available at: http://bphc.hrsa.gov/about/lookalike/index.html?IsPopUp=true. Accessed on September 5, 2014.
- Public hospitals in the United States, 2008. Healthcare Cost and Utilization Project. Agency for Healthcare Research and Quality, Rockville, MD. Available at: http://www.hcup‐us.ahrq.gov/reports/statbriefs/sb95.jsp. Published September 2010. Accessed on September 5, 2014. , , , .
- U.S. Department of Health and Human Services. Health Resources and Services Administration. Available at: http://www.hrsa.gov/shortage/. Accessed on September 5, 2014.
- California's Safety Net and The Need to Improve Local Collaboration in Care for the Uninsured: Counties, Clinics, Hospitals, and Local Health Plans. Available at: http://www.itup.org/Reports/Statewide/Safetynet_Report_Final.pdf. Published October 2008. Accessed on September 5, 2014. , .
- Unsatisfactory saturation: a critical exploration of the notion of saturated sample sizes in qualitative research. Qual Res. 2013;13(2):190–197. , .
- http://www.chairacademy.com/conference/2007/papers/leading_successful_interinstitutional_collaborations.pdf. Accessed on September 5, 2014. . Leading successful interinstitutional collaborations using the collaboration success measurement model. Paper presented at: The Chair Academy's 16th Annual International Conference: Navigating the Future through Authentic Leadership; 2007; Jacksonville, FL. Available at
- The difference between integration and collaboration in patient care: results from key informant interviews working in multiprofessional health care teams. J Manipulative Physiol Ther. 2009;32(9):715–722. , , , , .
- Implementing organized delivery systems: an integration scorecard. Health Care Manag Rev. 1994;19(3):7–20. , , , , , .
- State of California Office of Statewide Health Planning 3(2):77–101.
- Health Resources and Services Administration Primary Care: The Health Center Program. Affiliation agreements of community 303(17):1716–1722.
- Reducing hospital readmissions through primary care practice transformation. Journal Fam Pract. 2014;63(2):67–73. , , , , .
- Post‐hospitalization transitions: Examining the effects of timing of primary care provider follow‐up. J Hosp Med. 2010;5(7):392–397. , , .
- U.S. Department of Health and Human Services. Centers for Medicare 2009.
- Hospitalists and care transitions: the divorce of inpatient and outpatient care. Health Aff (Millwood). 2008;27(5):1315–1327. , , , .
- Reducing hospital readmissions: lessons from top‐performing hospitals. Available at: http://www.commonwealthfund.org/publications/case‐studies/2011/apr/reducing‐hospital‐readmissions. Published April 2011. Accessed on September 5, 2014. , , .
- Recasting readmissions by placing the hospital role in community context. JAMA. 2013;309(4):351–352. , , .
- A primary care physician's ideal transitions of care—where's the evidence? J Hosp Med. 2013;8(8):472–477. .
- Primary care: current problems and proposed solutions. Health Aff (Millwood). 2010;29(5):799–805. , .
Poorly coordinated care between hospital and outpatient settings contributes to medical errors, poor outcomes, and high costs.[1, 2, 3] Recent policy has sought to motivate better care coordination after hospital discharge. Financial penalties for excessive hospital readmissionsa perceived marker of poorly coordinated carehave motivated hospitals to adopt transitional care programs to improve postdischarge care coordination.[4] However, the success of hospital‐initiated transitional care strategies in reducing hospital readmissions has been limited.[5] This may be due to the fact that many factors driving hospital readmissions, such as chronic medical illness, patient education, and availability of outpatient care, are outside of a hospital's control.[5, 6] Even among the most comprehensive hospital‐based transitional care intervention strategies, there is little evidence of active engagement of primary care providers or collaboration between hospitals and primary care practices in the transitional care planning process.[5] Better engagement of primary care into transitional care strategies may improve postdischarge care coordination.[7, 8]
The potential benefits of collaboration are particularly salient in healthcare safety nets.[9] The US health safety net is a patchwork of providers, funding, and programs unified by a shared missiondelivering care to patients regardless of ability to payrather than a coordinated system with shared governance.[9] Safety‐net hospitals are at risk for higher‐than‐average readmissions penalties.[10, 11] Medicaid expansion under the Affordable Care Act will likely increase demand for services in these settings, which could worsen fragmentation of care as a result of strained capacity.[12] Collaboration between hospitals and primary care clinics in the safety net could help overcome fragmentation, improve efficiencies in care, and reduce costs and readmissions.[12, 13, 14, 15]
Despite the potential benefits, we found no studies on how to enable collaboration between hospitals and primary care. We sought to understand systems‐level factors limiting and facilitating collaboration between hospitals and primary care practices around coordinating inpatient‐to‐outpatient care transitions by conducting a qualitative study, focusing on the perspective of primary care leaders in the safety net.
STUDY DATA AND METHODS
We conducted semistructured telephone interviews with primary care leaders in health safety nets across California from August 2012 through October 2012, prior to the implementation of the federal hospital readmissions penalties program. Primary care leaders were defined as clinicians or nonclinicians holding leadership positions, including chief executive officers, clinic medical directors, and local experts in care coordination or quality improvement. We defined safety‐net clinics as federally qualified health centers (FQHCs) and/or FQHC Look‐Alikes (clinics that meet eligibility requirements and receive the same benefits as FQHCs, except for Public Health Service Section 330 grants), community health centers, and public hospital‐affiliated clinics operating under a traditional fee‐for‐service model and serving a high proportion of Medicaid and uninsured patients.[9, 16] We defined public hospitals as government‐owned hospitals that provide care for individuals with limited access elsewhere.[17]
Sampling and Recruitment
We purposefully sampled participants to maximize diversity in geographic region, metropolitan status,[18] and type of county health delivery system to enable identification of common themes across different settings and contexts. Delivery systems were defined as per the Insure the Uninsured Project, a 501(c)(3) nonprofit organization that conducts research on the uninsured in California.[19] Provider systems are counties with a public hospital; payer systems are counties that contract with private hospitals to deliver uncompensated care in place of a public hospital; and County Medical Services Program is a state program that administers county health care in participating small counties, in lieu of a provider or payer system. We used the county delivery system type as a composite proxy of available county resources and market context given variations in funding, access, and eligibility by system type.
Participants were identified through online public directories, community clinic consortiums, and departments of public health websites. Additional participants were sought using snowball sampling. Potential participants were e‐mailed a recruitment letter describing the study, its purpose, topics to be covered, and confidentiality assurance. Participants who did not respond were called or e‐mailed within 1 week. When initial recruitment was unsuccessful, we attempted to recruit another participant within the same organization when possible. We recruited participants until reaching thematic saturation (i.e., no further new themes emerged from our interviews).[20] No participants were recruited through snowballing.
Data Collection and Interview Guides
We conducted in‐depth, semistructured interviews using interview guides informed by existing literature on collaboration and integration across healthcare systems[21, 22, 23] (see Supporting Information, Appendix 1, in the online version of this article). Interviews were digitally recorded and professionally transcribed verbatim.
We obtained contextual information for settings represented by each respondent, such as number of clinics and annual visits, through the California Primary Care Annual Utilization Data Report and clinic websites.[24]
Analysis
We employed thematic analysis[25] using an inductive framework to identify emergent and recurring themes. We developed and refined a coding template iteratively. Our multidisciplinary team included 2 general internists (O.K.N., L.E.G), 1 hospitalist (S.R.G.), a clinical nurse specialist with a doctorate in nursing (A.L.), and research staff with a public health background (J.K.). Two team members (O.K.N., J.K.) systematically coded all transcripts. Disagreements in coding were resolved through negotiated consensus. All investigators reviewed and discussed identified themes. We emailed summary findings to participants for confirmation to enhance the reliability of our findings.
The institutional review board at the University of California, San Francisco approved the study protocol.
RESULTS
Of 52 individuals contacted from 39 different organizations, 23 did not respond, 4 declined to participate, and 25 were scheduled for an interview. We interviewed 22 primary care leaders across 11 California counties (Table 1) and identified themes around factors influencing collaboration with hospitals (Table 2). Most respondents had prior positive experiences collaborating with hospitals on small, focused projects. However, they asserted the need for better hospitalclinic collaboration, and thought collaboration was critical to achieving high‐quality care transitions. We did not observe any differences in perspectives expressed by clinician versus nonclinician leaders. Nonparticipants were more likely than participants to be from northern rural or central counties, FQHCs, and smaller clinic settings.
| |
Leadership position | No. (%) |
Chief executive officer or equivalent* | 9 (41) |
Chief medical officer or medical director | 7 (32) |
Other | 6 (27) |
Clinical experience | |
Physician (MD or DO) | 15 (68) |
Registered nurse | 1 (5) |
Nonclinician | 6 (27) |
Clinic setting | |
Clinic type | |
FQHC and FQHC Look‐Alikes | 15 (68) |
Hospital based | 2 (9) |
Other | 5 (23) |
No. of clinics in system | |
14 | 9 (41) |
59 | 6 (27) |
10 | 7 (32) |
Annual no. of visits | |
<100,000 | 9 (41) |
100,000499,999 | 11 (50) |
500,000 | 2 (9) |
County characteristics | |
Health delivery system type | |
Provider | 13 (59) |
Payer | 2 (9) |
County Medical Services Program∥ | 7 (32) |
Rural county | 7 (32) |
Theme | Subtheme | Quote |
---|---|---|
| ||
Lack of institutional financial incentives for collaboration. | Collaboration may lead to increased responsibility without reimbursement for clinic. | Where the [payment] model breaks down is that the savings is only to the hospital; and there's an expectation on our part to go ahead and take on those additional patients. If that $400,000 savings doesn't at least have a portion to the team that's going to help keep the people out of the hospital, then it won't work. (Participant 17) |
Collaboration may lead to competition from the hospital for primary care patients. | Our biggest issues with working with the hospital[are] that we have a finite number of [Medicaid] patients [in our catchment area for whom] you get larger reimbursement. For a federally qualified health center, it is [crucial] to ensure we have a revenue stream that helps us take care of the uninsured. So you can see the natural kind of conflict when your pool of patients is very small. (Participant 10) | |
Collaboration may lead to increased financial risk for the hospital. | 70% to 80% of our adult patients have no insurance and the fact is that none of these hospitals want those patients. They do get disproportionate hospital savings and other thingsbut they don't have a strong business model when they have uninsured patients coming in their doors. That's just the reality. (Participant 21) | |
Collaboration may lead to decreased financial risk for the hospital. | Most of these patients either have very low reimbursement or no reimbursement, and so [the hospital doesn't] really want these people to end up in very expensive care because it's a burden on their systemphilosophically, everyone agrees that if we keep people well in the outpatient setting, that would be better for everyone. No, there is no financial incentive whatsoever for [the hospital] to not work with us. [emphasis added] (Participant 18) | |
Competing priorities limit primary care's ability to focus on care transitions. | I wouldn't say [improving care transitions is a high priority]. It's not because we don't want to do the job. We have other priorities. [T]he big issue is access. There's a massive demand for primary care in our communityand we're just trying to make sure we have enough capacity. [There are] requirements HRSA has been asking of health centers and other priorities. We're starting up a residency program. We're recruiting more doctors. We're upping our quality improvement processes internally. We're making a reinvestment in our [electronic medical record]. It never stops. (Participant 22) | |
The multitude of [care transitions and other quality] improvement imperatives makes it difficult to focus. It's not that any one of these things necessarily represents a flawed approach. It's just that when you have a variety of folks from the national, state, and local levels who all have different ideas about what constitutes appropriate improvement, it's very hard to respond to it all at once. (Participant 6) | ||
Mismatched expectations about the role and capacity of primary care in care transitions limit collaboration. | Perception of primary care being undervalued by hospitals as a key stakeholder in care transitions. | They just make sure the paperwork is set up.and they have it written down, See doctor in 7 days. And I think they [the hospitals] think that's where their responsibility stops. They don't actually look at our records or talk to us. (Participant 2) |
Perceived unrealistic expectations of primary care capacity to deliver postdischarge care. | [The hospital will] send anyone that's poor to us whether they are our patient or not. [T]hey say go to [our clinic] and they'll give you your outpatient medications. [But] we're at capacity. [W]e have a 79 month wait for a [new] primary care appointment. So then, we're stuck with the ethical dilemma of [do we send the patient back to the ER/hospital] for their medication or do we just [try to] take them in? (Participant 13) | |
The hospitals feel every undoctored patient must be ours. [But] it's not like we're sitting on our hands. We have more than enough patients. (Participant 22) | ||
Informal affiliations and partnerships, formed through personal relationships and interpersonal networking, facilitate collaboration. | Informal affiliations arise from existing personal relationships and/or interpersonal networking. | Our CEO [has been here] for the past 40 years, and has had very deep and ongoing relationships with the [hospital]. Those doors are very wide open. (Participant 18) |
Informal partnerships are particularly important for FQHCs. | As an FQHC we can't have any ties financially or politically, but there's a traditional connection. (Participant 2) | |
Increasing demands on clinical productivity lead to a loss of networking opportunities. | We're one of the few clinics that has their own inpatient service. I would say that the transitions between the hospital and [our] clinic start from a much higher level than anybody else. [However] we're about to close our hospital service. It's just too much work for our [clinic] doctors. (Participant 8) | |
There used to be a meeting once a month where quality improvement programs and issues were discussed. Our administration eliminated these in favor of productivity, to increase our numbers of patients seen. (Participant 12) | ||
Loss of relationships with hospital personnel amplifies challenges to collaboration. | Because the primary care docs are not visible in the hospital[quality improvement] projects [become] hospital‐based. Usually they forget that we exist. (Participant 11) | |
External funding and support can enable opportunities for networking and relationship building. | The [national stakeholder organization] has done a lot of work with us to bring us together and figure out what we're doing [across] different counties, settings, providers. (Participant 20) | |
Electronic health records enable collaboration by improving communication between hospitals and primary care. | Lack of timely communication between inpatient and outpatient settings is a major obstacle to postdischarge care coordination. | It's a lot of effort to get medical records back. It is often not timely. Patients are going to cycle in and out of more costly acute care because we don't know that it's happening. Communication between [outpatient and inpatient] facilities is one of the most challenging issues. (Participant 13) |
Optimism about potential of EHRs. | A lot of people are depending on [the EHR] to make a lot of communication changes [where there was] a disconnect in the past. (Participant 7) | |
Lack of EHR interoperability. | We have an EHR that's pieced together. The [emergency department] has their own [system]. The clinics have their own. The inpatient has their own. They're all electronic but they don't all talk to each other that well. (Participant 20) | |
Our system has reached our maximum capacity and we've had to rely on our community partners to see the overflow. [T]he difficult communication [is] magnified. (Participant 11) | ||
Privacy and legal concerns (nonuniform application of HIPAA standards). | There is a very different view from hospital to hospital about what it is they feel that they can share legally under HIPAA or not. It's a very strange thing and it almost depends more on the chief information officer at [each] hospital and less on what the [regulations] actually say. (Participant 21) | |
Yes, [the EHR] does communicate with the hospitals and the hospitals [communicate] back [with us]. [T]here are some technical issues, butthe biggest impediments to making the technology work are new issues around confidentiality and access. (Participant 17) | ||
Interpersonal contact is still needed even with robust EHRs. | I think [communication between systems is] getting better [due to the EHR], but there's still quite a few holes and a sense of the loop not being completely closed. It's like when you pick up the phoneyou don't want the automated system, you want to actually talk to somebody. (Participant 18) |
Lack of Institutional Financial Incentives for Collaboration
Primary care leaders felt that current reimbursement strategies rewarded hospitals for reducing readmissions rather than promoting shared savings with primary care. Seeking collaboration with hospitals would potentially increase clinic responsibility for postdischarge patient care without reimbursement for additional work.
In counties without public hospitals, leaders worried that collaboration with hospitals could lead to active loss of Medicaid patients from their practices. Developing closer relationships with local hospitals would enable those hospitals to redirect Medicaid patients to hospital‐owned primary care clinics, leading to a loss of important revenue and financial stability for their clinics.
A subset of these leaders also perceived that nonpublic hospitals were reluctant to collaborate with their clinics. They hypothesized that hospital leaders worried that collaborating with their primary care practices would lead to more uninsured patients at their hospitals, leading to an increase in uncompensated hospital care and reduced reimbursement. However, a second subset of leaders thought that nonpublic hospitals had increased financial incentives to collaborate with safety‐net clinics, because improved coordination with outpatient care could prevent uncompensated hospital care.
Competing Clinic Priorities Limit Primary Care Ability to Focus on Care Transitions
Clinic leaders struggled to balance competing priorities, including strained clinic capacity, regulatory/accreditation requirements, and financial strain. New patient‐centered medical home initiatives, which improve primary care financial incentives for postdischarge care coordination, were perceived as well intentioned but added to an overwhelming burden of ongoing quality improvement efforts.
Mismatched Expectations About the Role and Capacity of Primary Care in Care Transitions Limits Collaboration
Many leaders felt that hospitals undervalued the role of primary care as stakeholders in improving care transitions. They perceived that hospitals made little effort to directly contact primary care physicians about their patients' hospitalizations and discharges. Leaders were frustrated that hospitals had unrealistic expectations of primary care to deliver timely postdischarge care, given their strained capacity. Consequently, some were reluctant to seek opportunities to collaborate with hospitals to improve care transitions.
Informal Affiliations and Partnerships, Formed Through Personal Relationships and Interpersonal Networking, Facilitate Collaboration
Informal affiliations between hospitals and primary care clinics helped improve awareness of organizational roles and capacity and create a sense of shared mission, thus enabling collaboration in spite of other barriers. Such affiliations arose from existing, longstanding personal relationships and/or interpersonal networking between individual providers across settings. These informal affiliations were important for safety‐net clinics that were FQHCs or FQHC Look‐Alikes, because formal hospital affiliations are discouraged by federal regulations.[26]
Opportunities for building relationships and networking with hospital personnel arose when clinic physicians had hospital admitting privileges. This on‐site presence facilitated personal relationships and communication between clinic and hospital physicians, thus enabling better collaboration. However, increasing demands on outpatient clinical productivity often made a hospital presence infeasible. One health system promoted interpersonal networking through regular meetings between the clinic and the local hospital to foster collaboration on quality improvement and care delivery; however, clinical productivity demands ultimately took priority over these meetings. Although delegating inpatient care to hospitalists enabled clinics to maximize their productivity, it also decreased opportunities for networking, and consequently, clinic physicians felt their voices and opinions were not represented in improvement initiatives.
Outside funding and support, such as incentive programs and conferences sponsored by local health plans, clinic consortiums, or national stakeholder organizations, enabled the most successful networking. These successes were independent of whether the clinic staff rounded in the hospital.
Electronic Health Records Enable Collaboration By Improving Communication Between Hospitals And Primary Care
Challenges in communication and information flow were also challenges to collaboration with hospitals. No respondents reported receiving routine notification of patient hospitalizations at the time of admission. Many clinics were dedicating significant attention to implementing electronic health record (EHR) systems to receive financial incentives associated with meaningful use.[27] Implementation of EHRs helped mitigate issues with communication with hospitals, though to a lesser degree than expected. Clinics early in the process of EHR adoption were optimistic about the potential of EHRs to improve communication with hospitals. However, clinic leaders in settings with greater EHR experience were more guarded in their enthusiasm. They observed that lack of interoperability between clinic and hospital EHRs was a persistent and major issue in spite of meaningful use standards, limiting timely flow of information across settings. Even when hospitals and their associated clinics had integrated or interoperable EHRs (n=3), or were working toward EHR integration (n=5), the need to expand networks to include other community healthcare settings using different systems presented ongoing challenges to achieving seamless communication due to a lack of interoperability.
When information sharing was technically feasible, leaders noted that inconsistent understanding and application of privacy rules dictated by the Health Insurance Portability and Accountability Act (HIPAA) limited information sharing. The quality and types of information shared varied widely across settings, depending on how HIPAA regulations were interpreted.
Even with robust EHRs, interpersonal contact was still perceived as crucial to enabling collaboration. EHRs were perceived to help with information flow, but did not facilitate relationship building across settings.
DISCUSSION
We found that safety‐net primary care leaders identified several barriers to collaboration with hospitals: (1) lack of financial incentives for collaboration, (2) competing priorities, (3) mismatched expectations about the role and capacity of primary care, and (4) poor communication infrastructure. Interpersonal networking and use of EHRs helped overcome these obstacles to a limited extent.
Prior studies demonstrate that early follow‐up, timely communication, and continuity with primary care after hospital discharge are associated with improved postdischarge outcomes.[8, 28, 29, 30] Despite evidence that collaboration between primary care and hospitals may help optimize postdischarge outcomes, our study is the first to describe primary care leaders' perspectives on potential targets for improving collaboration between hospitals and primary care to improve care transitions.
Our results highlight the need to modify payment models to align financial incentives across settings for collaboration. Otherwise, it may be difficult for hospitals to engage primary care in collaborative efforts to improve care transitions. Recent pilot payment models aim to motivate improved postdischarge care coordination. The Centers for Medicare and Medicaid Services implemented two new Current Procedural Terminology Transitional Care Management codes to enable reimbursement of outpatient physicians for management of patients transitioning from the hospital to the community. This model does not require communication between accepting (outpatient) and discharging (hospital) physicians or other hospital staff.[31] Another pilot program pays primary care clinics $6 per beneficiary per month if they become level 3 patient‐centered medical homes, which have stringent requirements for communication and coordination with hospitals for postdischarge care.[32] Capitated payment models, such as expansion of Medicaid managed care, and shared‐savings models, such accountable care organizations, aim to promote shared responsibility between hospitals and primary care by creating financial incentives to prevent hospitalizations through effective use of outpatient resources. The effectiveness of these strategies to improve care transitions is not yet established.
Many tout the adoption of EHRs as a means to improve communication and collaboration across settings.[33] However, policies narrowly focused on EHR adoption fail to address broader issues regarding lack of EHR interoperability and inconsistently applied privacy regulations under HIPAA, which were substantial barriers to information sharing. Stage 2 meaningful use criteria will address some interoperability issues by implementing standards for exchange of laboratory data and summary care records for care transitions.[34] Additional regulatory policies should promote uniform application of privacy regulations to enable more fluid sharing of electronic data across various healthcare settings. Locally and regionally negotiated data sharing agreements, as well as arrangements such as regional health information exchanges, could temporize these issues until broader policies are enacted.
EHRs did not obviate the need for meaningful interpersonal communication between providers. Hospital‐based quality improvement teams could create networking opportunities to foster relationship‐building and communication across settings. Leadership should consider scheduling protected time to facilitate attendance. Colocation of outpatient staff, such as nurse coordinators and office managers, in the hospital may also improve relationship building and care coordination.[35] Such measures would bridge the perceived divide between inpatient and outpatient care, and create avenues to find mutually beneficial solutions to improving postdischarge care transitions.[36]
Our results should be interpreted in light of several limitations. This study focused on primary care practices in the California safety net; given variations in safety nets across different contexts, the transferability of our findings may be limited. Second, rural perspectives were relatively under‐represented in our study sample; there may be additional unidentified issues specific to rural areas or specific to other nonparticipants that may have not been captured in this study. For this hypothesis‐generating study, we focused on the perspectives of primary care leaders. Triangulating perspectives of other stakeholders, including hospital leadership, mental health, social services, and payer organizations, will offer a more comprehensive analysis of barriers and enablers to hospitalprimary care collaboration. We were unable to collect data on the payer mix of each facility, which may influence the perceived financial barriers to collaboration among facilities. However, we anticipate that the broader theme of lack of financial incentives for collaboration will resonate across many settings, as collaboration between inpatient and outpatient providers in general has been largely unfunded by payers.[37, 38, 39] Further, most primary care providers (PCPs) in and outside of safety‐net settings operate on slim margins that cannot support additional time by PCPs or staff to coordinate care transitions.[39, 40] Because our study was completed prior to the implementation of several new payment models motivating postdischarge care coordination, we were unable to assess their effect on clinics' collaboration with hospitals.
In conclusion, efforts to improve collaboration between clinical settings around postdischarge care transitions will require targeted policy and quality improvement efforts in 3 specific areas. Policy makers and administrators with the power to negotiate payment schemes and regulatory policies should first align financial incentives across settings to support postdischarge transitions and care coordination, and second, improve EHR interoperability and uniform application of HIPAA regulations. Third, clinic and hospital leaders, and front‐line providers should enhance opportunities for interpersonal networking between providers in hospital and primary care settings. With the expansion of insurance coverage and increased demand for primary care in the safety net and other settings, policies to promote care coordination should consider the perspective of both hospital and clinic incentives and mechanisms for coordinating care across settings.
Disclosures
Preliminary results from this study were presented at the Society of General Internal Medicine 36th Annual Meeting in Denver, Colorado, April 2013. Dr. Nguyen's work on this project was funded by a federal training grant from the National Research Service Award (NRSA T32HP19025‐07‐00). Dr. Goldman is the recipient of grants from the Agency for Health Care Research and Quality (K08 HS018090‐01). Drs. Goldman, Greysen, and Lyndon are supported by the National Institutes of Health, National Center for Research Resources, Office of the Director (UCSF‐CTSI grant no. KL2 RR024130). The authors report no conflicts of interest.
Poorly coordinated care between hospital and outpatient settings contributes to medical errors, poor outcomes, and high costs.[1, 2, 3] Recent policy has sought to motivate better care coordination after hospital discharge. Financial penalties for excessive hospital readmissionsa perceived marker of poorly coordinated carehave motivated hospitals to adopt transitional care programs to improve postdischarge care coordination.[4] However, the success of hospital‐initiated transitional care strategies in reducing hospital readmissions has been limited.[5] This may be due to the fact that many factors driving hospital readmissions, such as chronic medical illness, patient education, and availability of outpatient care, are outside of a hospital's control.[5, 6] Even among the most comprehensive hospital‐based transitional care intervention strategies, there is little evidence of active engagement of primary care providers or collaboration between hospitals and primary care practices in the transitional care planning process.[5] Better engagement of primary care into transitional care strategies may improve postdischarge care coordination.[7, 8]
The potential benefits of collaboration are particularly salient in healthcare safety nets.[9] The US health safety net is a patchwork of providers, funding, and programs unified by a shared missiondelivering care to patients regardless of ability to payrather than a coordinated system with shared governance.[9] Safety‐net hospitals are at risk for higher‐than‐average readmissions penalties.[10, 11] Medicaid expansion under the Affordable Care Act will likely increase demand for services in these settings, which could worsen fragmentation of care as a result of strained capacity.[12] Collaboration between hospitals and primary care clinics in the safety net could help overcome fragmentation, improve efficiencies in care, and reduce costs and readmissions.[12, 13, 14, 15]
Despite the potential benefits, we found no studies on how to enable collaboration between hospitals and primary care. We sought to understand systems‐level factors limiting and facilitating collaboration between hospitals and primary care practices around coordinating inpatient‐to‐outpatient care transitions by conducting a qualitative study, focusing on the perspective of primary care leaders in the safety net.
STUDY DATA AND METHODS
We conducted semistructured telephone interviews with primary care leaders in health safety nets across California from August 2012 through October 2012, prior to the implementation of the federal hospital readmissions penalties program. Primary care leaders were defined as clinicians or nonclinicians holding leadership positions, including chief executive officers, clinic medical directors, and local experts in care coordination or quality improvement. We defined safety‐net clinics as federally qualified health centers (FQHCs) and/or FQHC Look‐Alikes (clinics that meet eligibility requirements and receive the same benefits as FQHCs, except for Public Health Service Section 330 grants), community health centers, and public hospital‐affiliated clinics operating under a traditional fee‐for‐service model and serving a high proportion of Medicaid and uninsured patients.[9, 16] We defined public hospitals as government‐owned hospitals that provide care for individuals with limited access elsewhere.[17]
Sampling and Recruitment
We purposefully sampled participants to maximize diversity in geographic region, metropolitan status,[18] and type of county health delivery system to enable identification of common themes across different settings and contexts. Delivery systems were defined as per the Insure the Uninsured Project, a 501(c)(3) nonprofit organization that conducts research on the uninsured in California.[19] Provider systems are counties with a public hospital; payer systems are counties that contract with private hospitals to deliver uncompensated care in place of a public hospital; and County Medical Services Program is a state program that administers county health care in participating small counties, in lieu of a provider or payer system. We used the county delivery system type as a composite proxy of available county resources and market context given variations in funding, access, and eligibility by system type.
Participants were identified through online public directories, community clinic consortiums, and departments of public health websites. Additional participants were sought using snowball sampling. Potential participants were e‐mailed a recruitment letter describing the study, its purpose, topics to be covered, and confidentiality assurance. Participants who did not respond were called or e‐mailed within 1 week. When initial recruitment was unsuccessful, we attempted to recruit another participant within the same organization when possible. We recruited participants until reaching thematic saturation (i.e., no further new themes emerged from our interviews).[20] No participants were recruited through snowballing.
Data Collection and Interview Guides
We conducted in‐depth, semistructured interviews using interview guides informed by existing literature on collaboration and integration across healthcare systems[21, 22, 23] (see Supporting Information, Appendix 1, in the online version of this article). Interviews were digitally recorded and professionally transcribed verbatim.
We obtained contextual information for settings represented by each respondent, such as number of clinics and annual visits, through the California Primary Care Annual Utilization Data Report and clinic websites.[24]
Analysis
We employed thematic analysis[25] using an inductive framework to identify emergent and recurring themes. We developed and refined a coding template iteratively. Our multidisciplinary team included 2 general internists (O.K.N., L.E.G), 1 hospitalist (S.R.G.), a clinical nurse specialist with a doctorate in nursing (A.L.), and research staff with a public health background (J.K.). Two team members (O.K.N., J.K.) systematically coded all transcripts. Disagreements in coding were resolved through negotiated consensus. All investigators reviewed and discussed identified themes. We emailed summary findings to participants for confirmation to enhance the reliability of our findings.
The institutional review board at the University of California, San Francisco approved the study protocol.
RESULTS
Of 52 individuals contacted from 39 different organizations, 23 did not respond, 4 declined to participate, and 25 were scheduled for an interview. We interviewed 22 primary care leaders across 11 California counties (Table 1) and identified themes around factors influencing collaboration with hospitals (Table 2). Most respondents had prior positive experiences collaborating with hospitals on small, focused projects. However, they asserted the need for better hospitalclinic collaboration, and thought collaboration was critical to achieving high‐quality care transitions. We did not observe any differences in perspectives expressed by clinician versus nonclinician leaders. Nonparticipants were more likely than participants to be from northern rural or central counties, FQHCs, and smaller clinic settings.
| |
Leadership position | No. (%) |
Chief executive officer or equivalent* | 9 (41) |
Chief medical officer or medical director | 7 (32) |
Other | 6 (27) |
Clinical experience | |
Physician (MD or DO) | 15 (68) |
Registered nurse | 1 (5) |
Nonclinician | 6 (27) |
Clinic setting | |
Clinic type | |
FQHC and FQHC Look‐Alikes | 15 (68) |
Hospital based | 2 (9) |
Other | 5 (23) |
No. of clinics in system | |
14 | 9 (41) |
59 | 6 (27) |
10 | 7 (32) |
Annual no. of visits | |
<100,000 | 9 (41) |
100,000499,999 | 11 (50) |
500,000 | 2 (9) |
County characteristics | |
Health delivery system type | |
Provider | 13 (59) |
Payer | 2 (9) |
County Medical Services Program∥ | 7 (32) |
Rural county | 7 (32) |
Theme | Subtheme | Quote |
---|---|---|
| ||
Lack of institutional financial incentives for collaboration. | Collaboration may lead to increased responsibility without reimbursement for clinic. | Where the [payment] model breaks down is that the savings is only to the hospital; and there's an expectation on our part to go ahead and take on those additional patients. If that $400,000 savings doesn't at least have a portion to the team that's going to help keep the people out of the hospital, then it won't work. (Participant 17) |
Collaboration may lead to competition from the hospital for primary care patients. | Our biggest issues with working with the hospital[are] that we have a finite number of [Medicaid] patients [in our catchment area for whom] you get larger reimbursement. For a federally qualified health center, it is [crucial] to ensure we have a revenue stream that helps us take care of the uninsured. So you can see the natural kind of conflict when your pool of patients is very small. (Participant 10) | |
Collaboration may lead to increased financial risk for the hospital. | 70% to 80% of our adult patients have no insurance and the fact is that none of these hospitals want those patients. They do get disproportionate hospital savings and other thingsbut they don't have a strong business model when they have uninsured patients coming in their doors. That's just the reality. (Participant 21) | |
Collaboration may lead to decreased financial risk for the hospital. | Most of these patients either have very low reimbursement or no reimbursement, and so [the hospital doesn't] really want these people to end up in very expensive care because it's a burden on their systemphilosophically, everyone agrees that if we keep people well in the outpatient setting, that would be better for everyone. No, there is no financial incentive whatsoever for [the hospital] to not work with us. [emphasis added] (Participant 18) | |
Competing priorities limit primary care's ability to focus on care transitions. | I wouldn't say [improving care transitions is a high priority]. It's not because we don't want to do the job. We have other priorities. [T]he big issue is access. There's a massive demand for primary care in our communityand we're just trying to make sure we have enough capacity. [There are] requirements HRSA has been asking of health centers and other priorities. We're starting up a residency program. We're recruiting more doctors. We're upping our quality improvement processes internally. We're making a reinvestment in our [electronic medical record]. It never stops. (Participant 22) | |
The multitude of [care transitions and other quality] improvement imperatives makes it difficult to focus. It's not that any one of these things necessarily represents a flawed approach. It's just that when you have a variety of folks from the national, state, and local levels who all have different ideas about what constitutes appropriate improvement, it's very hard to respond to it all at once. (Participant 6) | ||
Mismatched expectations about the role and capacity of primary care in care transitions limit collaboration. | Perception of primary care being undervalued by hospitals as a key stakeholder in care transitions. | They just make sure the paperwork is set up.and they have it written down, See doctor in 7 days. And I think they [the hospitals] think that's where their responsibility stops. They don't actually look at our records or talk to us. (Participant 2) |
Perceived unrealistic expectations of primary care capacity to deliver postdischarge care. | [The hospital will] send anyone that's poor to us whether they are our patient or not. [T]hey say go to [our clinic] and they'll give you your outpatient medications. [But] we're at capacity. [W]e have a 79 month wait for a [new] primary care appointment. So then, we're stuck with the ethical dilemma of [do we send the patient back to the ER/hospital] for their medication or do we just [try to] take them in? (Participant 13) | |
The hospitals feel every undoctored patient must be ours. [But] it's not like we're sitting on our hands. We have more than enough patients. (Participant 22) | ||
Informal affiliations and partnerships, formed through personal relationships and interpersonal networking, facilitate collaboration. | Informal affiliations arise from existing personal relationships and/or interpersonal networking. | Our CEO [has been here] for the past 40 years, and has had very deep and ongoing relationships with the [hospital]. Those doors are very wide open. (Participant 18) |
Informal partnerships are particularly important for FQHCs. | As an FQHC we can't have any ties financially or politically, but there's a traditional connection. (Participant 2) | |
Increasing demands on clinical productivity lead to a loss of networking opportunities. | We're one of the few clinics that has their own inpatient service. I would say that the transitions between the hospital and [our] clinic start from a much higher level than anybody else. [However] we're about to close our hospital service. It's just too much work for our [clinic] doctors. (Participant 8) | |
There used to be a meeting once a month where quality improvement programs and issues were discussed. Our administration eliminated these in favor of productivity, to increase our numbers of patients seen. (Participant 12) | ||
Loss of relationships with hospital personnel amplifies challenges to collaboration. | Because the primary care docs are not visible in the hospital[quality improvement] projects [become] hospital‐based. Usually they forget that we exist. (Participant 11) | |
External funding and support can enable opportunities for networking and relationship building. | The [national stakeholder organization] has done a lot of work with us to bring us together and figure out what we're doing [across] different counties, settings, providers. (Participant 20) | |
Electronic health records enable collaboration by improving communication between hospitals and primary care. | Lack of timely communication between inpatient and outpatient settings is a major obstacle to postdischarge care coordination. | It's a lot of effort to get medical records back. It is often not timely. Patients are going to cycle in and out of more costly acute care because we don't know that it's happening. Communication between [outpatient and inpatient] facilities is one of the most challenging issues. (Participant 13) |
Optimism about potential of EHRs. | A lot of people are depending on [the EHR] to make a lot of communication changes [where there was] a disconnect in the past. (Participant 7) | |
Lack of EHR interoperability. | We have an EHR that's pieced together. The [emergency department] has their own [system]. The clinics have their own. The inpatient has their own. They're all electronic but they don't all talk to each other that well. (Participant 20) | |
Our system has reached our maximum capacity and we've had to rely on our community partners to see the overflow. [T]he difficult communication [is] magnified. (Participant 11) | ||
Privacy and legal concerns (nonuniform application of HIPAA standards). | There is a very different view from hospital to hospital about what it is they feel that they can share legally under HIPAA or not. It's a very strange thing and it almost depends more on the chief information officer at [each] hospital and less on what the [regulations] actually say. (Participant 21) | |
Yes, [the EHR] does communicate with the hospitals and the hospitals [communicate] back [with us]. [T]here are some technical issues, butthe biggest impediments to making the technology work are new issues around confidentiality and access. (Participant 17) | ||
Interpersonal contact is still needed even with robust EHRs. | I think [communication between systems is] getting better [due to the EHR], but there's still quite a few holes and a sense of the loop not being completely closed. It's like when you pick up the phoneyou don't want the automated system, you want to actually talk to somebody. (Participant 18) |
Lack of Institutional Financial Incentives for Collaboration
Primary care leaders felt that current reimbursement strategies rewarded hospitals for reducing readmissions rather than promoting shared savings with primary care. Seeking collaboration with hospitals would potentially increase clinic responsibility for postdischarge patient care without reimbursement for additional work.
In counties without public hospitals, leaders worried that collaboration with hospitals could lead to active loss of Medicaid patients from their practices. Developing closer relationships with local hospitals would enable those hospitals to redirect Medicaid patients to hospital‐owned primary care clinics, leading to a loss of important revenue and financial stability for their clinics.
A subset of these leaders also perceived that nonpublic hospitals were reluctant to collaborate with their clinics. They hypothesized that hospital leaders worried that collaborating with their primary care practices would lead to more uninsured patients at their hospitals, leading to an increase in uncompensated hospital care and reduced reimbursement. However, a second subset of leaders thought that nonpublic hospitals had increased financial incentives to collaborate with safety‐net clinics, because improved coordination with outpatient care could prevent uncompensated hospital care.
Competing Clinic Priorities Limit Primary Care Ability to Focus on Care Transitions
Clinic leaders struggled to balance competing priorities, including strained clinic capacity, regulatory/accreditation requirements, and financial strain. New patient‐centered medical home initiatives, which improve primary care financial incentives for postdischarge care coordination, were perceived as well intentioned but added to an overwhelming burden of ongoing quality improvement efforts.
Mismatched Expectations About the Role and Capacity of Primary Care in Care Transitions Limits Collaboration
Many leaders felt that hospitals undervalued the role of primary care as stakeholders in improving care transitions. They perceived that hospitals made little effort to directly contact primary care physicians about their patients' hospitalizations and discharges. Leaders were frustrated that hospitals had unrealistic expectations of primary care to deliver timely postdischarge care, given their strained capacity. Consequently, some were reluctant to seek opportunities to collaborate with hospitals to improve care transitions.
Informal Affiliations and Partnerships, Formed Through Personal Relationships and Interpersonal Networking, Facilitate Collaboration
Informal affiliations between hospitals and primary care clinics helped improve awareness of organizational roles and capacity and create a sense of shared mission, thus enabling collaboration in spite of other barriers. Such affiliations arose from existing, longstanding personal relationships and/or interpersonal networking between individual providers across settings. These informal affiliations were important for safety‐net clinics that were FQHCs or FQHC Look‐Alikes, because formal hospital affiliations are discouraged by federal regulations.[26]
Opportunities for building relationships and networking with hospital personnel arose when clinic physicians had hospital admitting privileges. This on‐site presence facilitated personal relationships and communication between clinic and hospital physicians, thus enabling better collaboration. However, increasing demands on outpatient clinical productivity often made a hospital presence infeasible. One health system promoted interpersonal networking through regular meetings between the clinic and the local hospital to foster collaboration on quality improvement and care delivery; however, clinical productivity demands ultimately took priority over these meetings. Although delegating inpatient care to hospitalists enabled clinics to maximize their productivity, it also decreased opportunities for networking, and consequently, clinic physicians felt their voices and opinions were not represented in improvement initiatives.
Outside funding and support, such as incentive programs and conferences sponsored by local health plans, clinic consortiums, or national stakeholder organizations, enabled the most successful networking. These successes were independent of whether the clinic staff rounded in the hospital.
Electronic Health Records Enable Collaboration By Improving Communication Between Hospitals And Primary Care
Challenges in communication and information flow were also challenges to collaboration with hospitals. No respondents reported receiving routine notification of patient hospitalizations at the time of admission. Many clinics were dedicating significant attention to implementing electronic health record (EHR) systems to receive financial incentives associated with meaningful use.[27] Implementation of EHRs helped mitigate issues with communication with hospitals, though to a lesser degree than expected. Clinics early in the process of EHR adoption were optimistic about the potential of EHRs to improve communication with hospitals. However, clinic leaders in settings with greater EHR experience were more guarded in their enthusiasm. They observed that lack of interoperability between clinic and hospital EHRs was a persistent and major issue in spite of meaningful use standards, limiting timely flow of information across settings. Even when hospitals and their associated clinics had integrated or interoperable EHRs (n=3), or were working toward EHR integration (n=5), the need to expand networks to include other community healthcare settings using different systems presented ongoing challenges to achieving seamless communication due to a lack of interoperability.
When information sharing was technically feasible, leaders noted that inconsistent understanding and application of privacy rules dictated by the Health Insurance Portability and Accountability Act (HIPAA) limited information sharing. The quality and types of information shared varied widely across settings, depending on how HIPAA regulations were interpreted.
Even with robust EHRs, interpersonal contact was still perceived as crucial to enabling collaboration. EHRs were perceived to help with information flow, but did not facilitate relationship building across settings.
DISCUSSION
We found that safety‐net primary care leaders identified several barriers to collaboration with hospitals: (1) lack of financial incentives for collaboration, (2) competing priorities, (3) mismatched expectations about the role and capacity of primary care, and (4) poor communication infrastructure. Interpersonal networking and use of EHRs helped overcome these obstacles to a limited extent.
Prior studies demonstrate that early follow‐up, timely communication, and continuity with primary care after hospital discharge are associated with improved postdischarge outcomes.[8, 28, 29, 30] Despite evidence that collaboration between primary care and hospitals may help optimize postdischarge outcomes, our study is the first to describe primary care leaders' perspectives on potential targets for improving collaboration between hospitals and primary care to improve care transitions.
Our results highlight the need to modify payment models to align financial incentives across settings for collaboration. Otherwise, it may be difficult for hospitals to engage primary care in collaborative efforts to improve care transitions. Recent pilot payment models aim to motivate improved postdischarge care coordination. The Centers for Medicare and Medicaid Services implemented two new Current Procedural Terminology Transitional Care Management codes to enable reimbursement of outpatient physicians for management of patients transitioning from the hospital to the community. This model does not require communication between accepting (outpatient) and discharging (hospital) physicians or other hospital staff.[31] Another pilot program pays primary care clinics $6 per beneficiary per month if they become level 3 patient‐centered medical homes, which have stringent requirements for communication and coordination with hospitals for postdischarge care.[32] Capitated payment models, such as expansion of Medicaid managed care, and shared‐savings models, such accountable care organizations, aim to promote shared responsibility between hospitals and primary care by creating financial incentives to prevent hospitalizations through effective use of outpatient resources. The effectiveness of these strategies to improve care transitions is not yet established.
Many tout the adoption of EHRs as a means to improve communication and collaboration across settings.[33] However, policies narrowly focused on EHR adoption fail to address broader issues regarding lack of EHR interoperability and inconsistently applied privacy regulations under HIPAA, which were substantial barriers to information sharing. Stage 2 meaningful use criteria will address some interoperability issues by implementing standards for exchange of laboratory data and summary care records for care transitions.[34] Additional regulatory policies should promote uniform application of privacy regulations to enable more fluid sharing of electronic data across various healthcare settings. Locally and regionally negotiated data sharing agreements, as well as arrangements such as regional health information exchanges, could temporize these issues until broader policies are enacted.
EHRs did not obviate the need for meaningful interpersonal communication between providers. Hospital‐based quality improvement teams could create networking opportunities to foster relationship‐building and communication across settings. Leadership should consider scheduling protected time to facilitate attendance. Colocation of outpatient staff, such as nurse coordinators and office managers, in the hospital may also improve relationship building and care coordination.[35] Such measures would bridge the perceived divide between inpatient and outpatient care, and create avenues to find mutually beneficial solutions to improving postdischarge care transitions.[36]
Our results should be interpreted in light of several limitations. This study focused on primary care practices in the California safety net; given variations in safety nets across different contexts, the transferability of our findings may be limited. Second, rural perspectives were relatively under‐represented in our study sample; there may be additional unidentified issues specific to rural areas or specific to other nonparticipants that may have not been captured in this study. For this hypothesis‐generating study, we focused on the perspectives of primary care leaders. Triangulating perspectives of other stakeholders, including hospital leadership, mental health, social services, and payer organizations, will offer a more comprehensive analysis of barriers and enablers to hospitalprimary care collaboration. We were unable to collect data on the payer mix of each facility, which may influence the perceived financial barriers to collaboration among facilities. However, we anticipate that the broader theme of lack of financial incentives for collaboration will resonate across many settings, as collaboration between inpatient and outpatient providers in general has been largely unfunded by payers.[37, 38, 39] Further, most primary care providers (PCPs) in and outside of safety‐net settings operate on slim margins that cannot support additional time by PCPs or staff to coordinate care transitions.[39, 40] Because our study was completed prior to the implementation of several new payment models motivating postdischarge care coordination, we were unable to assess their effect on clinics' collaboration with hospitals.
In conclusion, efforts to improve collaboration between clinical settings around postdischarge care transitions will require targeted policy and quality improvement efforts in 3 specific areas. Policy makers and administrators with the power to negotiate payment schemes and regulatory policies should first align financial incentives across settings to support postdischarge transitions and care coordination, and second, improve EHR interoperability and uniform application of HIPAA regulations. Third, clinic and hospital leaders, and front‐line providers should enhance opportunities for interpersonal networking between providers in hospital and primary care settings. With the expansion of insurance coverage and increased demand for primary care in the safety net and other settings, policies to promote care coordination should consider the perspective of both hospital and clinic incentives and mechanisms for coordinating care across settings.
Disclosures
Preliminary results from this study were presented at the Society of General Internal Medicine 36th Annual Meeting in Denver, Colorado, April 2013. Dr. Nguyen's work on this project was funded by a federal training grant from the National Research Service Award (NRSA T32HP19025‐07‐00). Dr. Goldman is the recipient of grants from the Agency for Health Care Research and Quality (K08 HS018090‐01). Drs. Goldman, Greysen, and Lyndon are supported by the National Institutes of Health, National Center for Research Resources, Office of the Director (UCSF‐CTSI grant no. KL2 RR024130). The authors report no conflicts of interest.
- Crossing the Quality Chasm: A New Health System for the 21st Century. Washington, DC: The National Academies Press; 2001.
- Deficits in communication and information transfer between hospital‐based and primary care physicians: implications for patient safety and continuity of care. JAMA. 2007;297(8):831–841. , , , , , .
- Medical errors related to discontinuity of care from an inpatient to an outpatient setting. J Gen Intern Med. 2003;18(8):646–651. , , , .
- Medicare Payment Advisory Commission. Report to the Congress: Promoting Greater Efficiency in Medicare. Washington, DC: Medicare Payment Advisory Commission; 2007.
- Hospital‐initiated transitional care interventions as a patient safety strategy: a systematic review. Ann Intern Med. 2013;158(5 pt 2):433–440. , , , , , .
- Thirty‐day readmission rates for Medicare beneficiaries by race and site of care. JAMA. 2011;305(7):675–681. , , .
- Improving care transitions: hospitalists partnering with primary care. J Hosp Med. 2010;5(7):375–377. , .
- Primary care physician communication at hospital discharge reduces medication discrepancies. J Hosp Med. 2013;8(12):672–677. , , , , .
- Institute of Medicine. America's Health Care Safety Net: Intact but Endangered. Washington, DC: Institute of Medicine; 2000.
- Higher readmissions at safety‐net hospitals and potential policy solutions. Issue Brief (Commonw Fund). 2012;34:1–16. , .
- Characteristics of hospitals receiving penalties under the hospital readmissions reduction program. JAMA. 2013;309(4):342–343. , .
- Ensuring Equity: A Post‐Reform Framework to Achieve High Performance Health Care for Vulnerable Populations. New York, NY: The Commonwealth Fund; 2011. , , , et al.
- Enhancing the Capacity of Community Centers to Achieve High Performance: Findings from the 2009 Commonwealth Fund National Survey of Federally Qualified Health Centers. New York, NY: The Commonwealth Fund; 2010. , , , , .
- Integration mechanisms and hospital efficiency in integrated health care delivery systems. J Med Syst. 2002;26(2):127–143. , , .
- Effect of physician collaboration network on hospitalization cost and readmission rate. Eur J Public Health. 2012;22(5):629–633. , , .
- Health Resources and Services Administration. Health Center Look‐Alikes Program. Available at: http://bphc.hrsa.gov/about/lookalike/index.html?IsPopUp=true. Accessed on September 5, 2014.
- Public hospitals in the United States, 2008. Healthcare Cost and Utilization Project. Agency for Healthcare Research and Quality, Rockville, MD. Available at: http://www.hcup‐us.ahrq.gov/reports/statbriefs/sb95.jsp. Published September 2010. Accessed on September 5, 2014. , , , .
- U.S. Department of Health and Human Services. Health Resources and Services Administration. Available at: http://www.hrsa.gov/shortage/. Accessed on September 5, 2014.
- California's Safety Net and The Need to Improve Local Collaboration in Care for the Uninsured: Counties, Clinics, Hospitals, and Local Health Plans. Available at: http://www.itup.org/Reports/Statewide/Safetynet_Report_Final.pdf. Published October 2008. Accessed on September 5, 2014. , .
- Unsatisfactory saturation: a critical exploration of the notion of saturated sample sizes in qualitative research. Qual Res. 2013;13(2):190–197. , .
- http://www.chairacademy.com/conference/2007/papers/leading_successful_interinstitutional_collaborations.pdf. Accessed on September 5, 2014. . Leading successful interinstitutional collaborations using the collaboration success measurement model. Paper presented at: The Chair Academy's 16th Annual International Conference: Navigating the Future through Authentic Leadership; 2007; Jacksonville, FL. Available at
- The difference between integration and collaboration in patient care: results from key informant interviews working in multiprofessional health care teams. J Manipulative Physiol Ther. 2009;32(9):715–722. , , , , .
- Implementing organized delivery systems: an integration scorecard. Health Care Manag Rev. 1994;19(3):7–20. , , , , , .
- State of California Office of Statewide Health Planning 3(2):77–101.
- Health Resources and Services Administration Primary Care: The Health Center Program. Affiliation agreements of community 303(17):1716–1722.
- Reducing hospital readmissions through primary care practice transformation. Journal Fam Pract. 2014;63(2):67–73. , , , , .
- Post‐hospitalization transitions: Examining the effects of timing of primary care provider follow‐up. J Hosp Med. 2010;5(7):392–397. , , .
- U.S. Department of Health and Human Services. Centers for Medicare 2009.
- Hospitalists and care transitions: the divorce of inpatient and outpatient care. Health Aff (Millwood). 2008;27(5):1315–1327. , , , .
- Reducing hospital readmissions: lessons from top‐performing hospitals. Available at: http://www.commonwealthfund.org/publications/case‐studies/2011/apr/reducing‐hospital‐readmissions. Published April 2011. Accessed on September 5, 2014. , , .
- Recasting readmissions by placing the hospital role in community context. JAMA. 2013;309(4):351–352. , , .
- A primary care physician's ideal transitions of care—where's the evidence? J Hosp Med. 2013;8(8):472–477. .
- Primary care: current problems and proposed solutions. Health Aff (Millwood). 2010;29(5):799–805. , .
- Crossing the Quality Chasm: A New Health System for the 21st Century. Washington, DC: The National Academies Press; 2001.
- Deficits in communication and information transfer between hospital‐based and primary care physicians: implications for patient safety and continuity of care. JAMA. 2007;297(8):831–841. , , , , , .
- Medical errors related to discontinuity of care from an inpatient to an outpatient setting. J Gen Intern Med. 2003;18(8):646–651. , , , .
- Medicare Payment Advisory Commission. Report to the Congress: Promoting Greater Efficiency in Medicare. Washington, DC: Medicare Payment Advisory Commission; 2007.
- Hospital‐initiated transitional care interventions as a patient safety strategy: a systematic review. Ann Intern Med. 2013;158(5 pt 2):433–440. , , , , , .
- Thirty‐day readmission rates for Medicare beneficiaries by race and site of care. JAMA. 2011;305(7):675–681. , , .
- Improving care transitions: hospitalists partnering with primary care. J Hosp Med. 2010;5(7):375–377. , .
- Primary care physician communication at hospital discharge reduces medication discrepancies. J Hosp Med. 2013;8(12):672–677. , , , , .
- Institute of Medicine. America's Health Care Safety Net: Intact but Endangered. Washington, DC: Institute of Medicine; 2000.
- Higher readmissions at safety‐net hospitals and potential policy solutions. Issue Brief (Commonw Fund). 2012;34:1–16. , .
- Characteristics of hospitals receiving penalties under the hospital readmissions reduction program. JAMA. 2013;309(4):342–343. , .
- Ensuring Equity: A Post‐Reform Framework to Achieve High Performance Health Care for Vulnerable Populations. New York, NY: The Commonwealth Fund; 2011. , , , et al.
- Enhancing the Capacity of Community Centers to Achieve High Performance: Findings from the 2009 Commonwealth Fund National Survey of Federally Qualified Health Centers. New York, NY: The Commonwealth Fund; 2010. , , , , .
- Integration mechanisms and hospital efficiency in integrated health care delivery systems. J Med Syst. 2002;26(2):127–143. , , .
- Effect of physician collaboration network on hospitalization cost and readmission rate. Eur J Public Health. 2012;22(5):629–633. , , .
- Health Resources and Services Administration. Health Center Look‐Alikes Program. Available at: http://bphc.hrsa.gov/about/lookalike/index.html?IsPopUp=true. Accessed on September 5, 2014.
- Public hospitals in the United States, 2008. Healthcare Cost and Utilization Project. Agency for Healthcare Research and Quality, Rockville, MD. Available at: http://www.hcup‐us.ahrq.gov/reports/statbriefs/sb95.jsp. Published September 2010. Accessed on September 5, 2014. , , , .
- U.S. Department of Health and Human Services. Health Resources and Services Administration. Available at: http://www.hrsa.gov/shortage/. Accessed on September 5, 2014.
- California's Safety Net and The Need to Improve Local Collaboration in Care for the Uninsured: Counties, Clinics, Hospitals, and Local Health Plans. Available at: http://www.itup.org/Reports/Statewide/Safetynet_Report_Final.pdf. Published October 2008. Accessed on September 5, 2014. , .
- Unsatisfactory saturation: a critical exploration of the notion of saturated sample sizes in qualitative research. Qual Res. 2013;13(2):190–197. , .
- http://www.chairacademy.com/conference/2007/papers/leading_successful_interinstitutional_collaborations.pdf. Accessed on September 5, 2014. . Leading successful interinstitutional collaborations using the collaboration success measurement model. Paper presented at: The Chair Academy's 16th Annual International Conference: Navigating the Future through Authentic Leadership; 2007; Jacksonville, FL. Available at
- The difference between integration and collaboration in patient care: results from key informant interviews working in multiprofessional health care teams. J Manipulative Physiol Ther. 2009;32(9):715–722. , , , , .
- Implementing organized delivery systems: an integration scorecard. Health Care Manag Rev. 1994;19(3):7–20. , , , , , .
- State of California Office of Statewide Health Planning 3(2):77–101.
- Health Resources and Services Administration Primary Care: The Health Center Program. Affiliation agreements of community 303(17):1716–1722.
- Reducing hospital readmissions through primary care practice transformation. Journal Fam Pract. 2014;63(2):67–73. , , , , .
- Post‐hospitalization transitions: Examining the effects of timing of primary care provider follow‐up. J Hosp Med. 2010;5(7):392–397. , , .
- U.S. Department of Health and Human Services. Centers for Medicare 2009.
- Hospitalists and care transitions: the divorce of inpatient and outpatient care. Health Aff (Millwood). 2008;27(5):1315–1327. , , , .
- Reducing hospital readmissions: lessons from top‐performing hospitals. Available at: http://www.commonwealthfund.org/publications/case‐studies/2011/apr/reducing‐hospital‐readmissions. Published April 2011. Accessed on September 5, 2014. , , .
- Recasting readmissions by placing the hospital role in community context. JAMA. 2013;309(4):351–352. , , .
- A primary care physician's ideal transitions of care—where's the evidence? J Hosp Med. 2013;8(8):472–477. .
- Primary care: current problems and proposed solutions. Health Aff (Millwood). 2010;29(5):799–805. , .
© 2014 Society of Hospital Medicine
Letter to the Editor
We agree with Drs. Arora and Mahmud that emerging mobile health (mHealth) approaches to improving patient engagement will need to demonstrate their value to advance health and healthcare. The potential for mHealth to do this has been often described[1, 2] but, so far, rarely measured or demonstrated.
The technology costs of our tablet‐based intervention[3] were low: 2 iPads at $400 each. The real expense was for personnel: research assistants needed to teach patients how to use the technology effectively. In the future, we hope to shift device and software orientation to patient‐care assistants, nurses, or even digital assistants, nonmedical personnel who have technical expertise with the health‐related devices and software needed to engage with the electronic health record and educational materials. Thus, at least part of the challenge of cost‐effectiveness aside from improved outcomeswill be demonstrating eventual time savings for providers who no longer need to hand deliver or explain paper pamphlets or printouts, or shepherd patients through their digitally assisted education.
One day we may muse, what did we do before mHealth? as we might do now when using mobile technologies for nonhealth‐related tasks like getting directions or making a call. Indeed, who can remember the last time they routinely used a paper map or phonebook for these daily tasks? Our prescription for tablets is a step in that direction, but we will need to also reimagine patient education and related daily tasks at the hospital and system level to realize the potential of lower costs and higher quality care we can achieve using mHealth.[4]
- Can mobile health technologies transform health care? JAMA. 2013;310(22):2395–2396. , , .
- The effectiveness of mobile‐health technologies to improve health care service delivery processes: a systematic review and meta‐analysis. PLoS Med. 2013;10(1):e1001363. , , , et al.
- Tablet computers for hospitalized patients: a pilot study to improve inpatient engagement [published online ahead of print February 13, 2013]. J Hosp Med. doi: 10.1002/jhm.2169. , , , , .
- Patient engagement in the inpatient setting: a systematic review [published online ahead of print November 22, 2013]. J Am Med Inform Assoc. doi: 10.1136/amiajnl‐2013‐002141. , , , et al.
We agree with Drs. Arora and Mahmud that emerging mobile health (mHealth) approaches to improving patient engagement will need to demonstrate their value to advance health and healthcare. The potential for mHealth to do this has been often described[1, 2] but, so far, rarely measured or demonstrated.
The technology costs of our tablet‐based intervention[3] were low: 2 iPads at $400 each. The real expense was for personnel: research assistants needed to teach patients how to use the technology effectively. In the future, we hope to shift device and software orientation to patient‐care assistants, nurses, or even digital assistants, nonmedical personnel who have technical expertise with the health‐related devices and software needed to engage with the electronic health record and educational materials. Thus, at least part of the challenge of cost‐effectiveness aside from improved outcomeswill be demonstrating eventual time savings for providers who no longer need to hand deliver or explain paper pamphlets or printouts, or shepherd patients through their digitally assisted education.
One day we may muse, what did we do before mHealth? as we might do now when using mobile technologies for nonhealth‐related tasks like getting directions or making a call. Indeed, who can remember the last time they routinely used a paper map or phonebook for these daily tasks? Our prescription for tablets is a step in that direction, but we will need to also reimagine patient education and related daily tasks at the hospital and system level to realize the potential of lower costs and higher quality care we can achieve using mHealth.[4]
We agree with Drs. Arora and Mahmud that emerging mobile health (mHealth) approaches to improving patient engagement will need to demonstrate their value to advance health and healthcare. The potential for mHealth to do this has been often described[1, 2] but, so far, rarely measured or demonstrated.
The technology costs of our tablet‐based intervention[3] were low: 2 iPads at $400 each. The real expense was for personnel: research assistants needed to teach patients how to use the technology effectively. In the future, we hope to shift device and software orientation to patient‐care assistants, nurses, or even digital assistants, nonmedical personnel who have technical expertise with the health‐related devices and software needed to engage with the electronic health record and educational materials. Thus, at least part of the challenge of cost‐effectiveness aside from improved outcomeswill be demonstrating eventual time savings for providers who no longer need to hand deliver or explain paper pamphlets or printouts, or shepherd patients through their digitally assisted education.
One day we may muse, what did we do before mHealth? as we might do now when using mobile technologies for nonhealth‐related tasks like getting directions or making a call. Indeed, who can remember the last time they routinely used a paper map or phonebook for these daily tasks? Our prescription for tablets is a step in that direction, but we will need to also reimagine patient education and related daily tasks at the hospital and system level to realize the potential of lower costs and higher quality care we can achieve using mHealth.[4]
- Can mobile health technologies transform health care? JAMA. 2013;310(22):2395–2396. , , .
- The effectiveness of mobile‐health technologies to improve health care service delivery processes: a systematic review and meta‐analysis. PLoS Med. 2013;10(1):e1001363. , , , et al.
- Tablet computers for hospitalized patients: a pilot study to improve inpatient engagement [published online ahead of print February 13, 2013]. J Hosp Med. doi: 10.1002/jhm.2169. , , , , .
- Patient engagement in the inpatient setting: a systematic review [published online ahead of print November 22, 2013]. J Am Med Inform Assoc. doi: 10.1136/amiajnl‐2013‐002141. , , , et al.
- Can mobile health technologies transform health care? JAMA. 2013;310(22):2395–2396. , , .
- The effectiveness of mobile‐health technologies to improve health care service delivery processes: a systematic review and meta‐analysis. PLoS Med. 2013;10(1):e1001363. , , , et al.
- Tablet computers for hospitalized patients: a pilot study to improve inpatient engagement [published online ahead of print February 13, 2013]. J Hosp Med. doi: 10.1002/jhm.2169. , , , , .
- Patient engagement in the inpatient setting: a systematic review [published online ahead of print November 22, 2013]. J Am Med Inform Assoc. doi: 10.1136/amiajnl‐2013‐002141. , , , et al.
Peer‐Reviewed Journals and Social Media
Only 20 years ago, science from peer‐reviewed journals was still distributed and consumed in the same fashion that evolved from the earliest days of medical science: in print at monthly or weekly intervals. The Internet radically accelerated this paradigm but left the essential processes intact; journals could publish the information and readers could read it more easily, but the basic forums for interaction and discussion over the content remained the same. Enter Web 2.0 and the era of social media. Authors, editors, and readers can now interact easily with each other over the content in real time and across great distances.
Social media may not have changed the way science is produced and reviewed, but it is certainly changing how people consume and use the science. Some have suggested that social media activity around particular articles or journals may be a more important measure of impact than traditional measures of citation,[1] and others have suggested that Twitter activity in particular has changed both the speed and quality of discussion about new studies within the scientific community.[2] In the face of these trends, the Journal of Hospital Medicine (JHM) has decided to develop a bold strategy for leadership in this emerging area, with an initial focus on increasing JHM's activity and visibility on Twitter.
As part of this initial focus, JHM has successfully developed and implemented a protocol for use by authors to compose 2 Tweets describing their publications: the first announces the article's publication (e.g., New evidence on white coats and risk for hospital‐acquired infections), and the second promotes a key point from the article (e.g., Does the doctor's white coat spread hospital infection?). These Tweets are encouraged (but not required) from the corresponding author for every article in every edition, and JHM's editorial staff works with individual authors to refine their message and maximize their impact. To help authors, we have developed several tips for effective tweeting (Table 1).
1. Make it short:The limit is 140 characters, but getting retweets requires additional room for others to add their 2 cents, so try to get it under 100 characters. |
2. Make it simple: If your tweet includes complex terminology or analytic methods, it is not likely to get picked up. Make it easy to read for the lay public. |
3. Make it clear: Your article may have several conclusions, but pick the most newsworthy for the general public. It is usually best to focus on the main finding. |
4. Pose a question: Raise interest by piquing the curiosity of potential readers. A good question can motivate readers to click on your article to find the answer. |
5. Add a hashtag: Hashtags index tweets on Twitter. It is best to pick 1 or 2 existing tags from the healthcare hashtag project that fit the focus of your article ( |
6. Build your following: Include your Twitter handle to alert current/prospective followers of your publication. |
Even after just 1 year of this Twitter‐focused strategy, we are already seeing noteworthy impact and have learned several lessons.
AUTHORS CAN AND WILL GENERATE TWEETS FOR THEIR ARTICLES
When we started asking authors to generate tweets for their articles, Twitter was relatively new, and we were unsure if authors would be willing and able to participate. Since we started, we have noticed a steady increase in the number of author‐generated tweets. Today, more than three‐quarters of tweets per issue are author generated. Anecdotal feedback has been very positive, and authors have expressed interest in the plan for tweeting as well as feedback on how well their tweets were written. If authors or institutions are on Twitter, we also encourage using the Twitter name or handle in the tweet, which serves as a way for others on Twitter to identify directly with the author or institution and often results in greater interest in a particular tweet. Of note, authors have no obligation to become regular users of Twitter or engage with followers of JHM's Twitter feed, but many find themselves following the journal's feed more closely (and responding to posts by other authors) once they have joined Twitter and tweeted about their own work via JHM.
#HASHTAGS MAKE IT HAPPEN
Because Twitter users are a very large crowd of people with diverse interests, it is important to target tweets to the groups that would be most interested in studies. The use of hashtags makes it easy to index tweets. One of the major edits of author‐generated tweets that we provide is to index the articles to the most popular hashtags. For example, medical education studies can be indexed under #meded, which is a popular hashtag for clinician educators. Other important hashtags for hospitalists include #ptsafety, #readmissions, #healthpolicy, #healthcosts, or #infectiousdisease. To select hashtags, we have found the healthcare hashtag directory maintained by Symplur (Upland, CA;
HIGH IMPACT STUDIES MAKE A BIGGER IMPACT ON TWITTER
We observed a high number of retweets and comments about articles that were the most viewed studies on JHM online, referring to Project BOOST (Better Outcomes for Older Adults Through Safe Transitions) and the Society of Hospital Medicine's Choosing Wisely campaign. This is not surprising given the national focus on readmissions as well as cost‐conscious care. Moreover, our experience is in line with observations that Twitter provides an additional source of page views and article downloads for medical journals[3] and research, which demonstrates that studies that are tweeted will eventually be cited more.[4, 5]
TECHNOLOGY STUDIES ARE ADORED BY TWITTER
Studies and research examining the use of smartphones, apps, or social media in healthcare draw a lot of attention on Twitter, particularly from other technophiles in healthcare who often use the #hscm healthcare social media hashtag. Such studies often resonate with Twitter users, who tend to be engaged in technology at a high level and are interested in how to advance the use of technology in the healthcare workplace.
JHM's social media strategy has already been very successful in its early implementation; the JHM twitter feed has >600 followers. Although most authors submit their own tweets (71/117 or 61% of articles over the last year), JHM has also created social media roles for editors to fill in tweets when missing and ensure timely and consistent output from the JHM feed. We have also started a Facebook page, with a rapidly growing number of followers, and we continue to see our social media influence scores rise. In the next year we hope to develop a JHM blog, with invited commentary as well as a process for unsolicited submissions from our readership.
Increasingly, a journal's impact (small i) is measured not only in the traditional metric of impact factor (a representation of the number of papers cited in a given journal publication year), but also by the journal's ability to disseminate knowledge and awareness of issues key to the field. Social media is a major element of the next phase of evidence dissemination, and JHM is pleased to be developing and growing its footprint in the digital world.
- Exploring the use of social media to measure journal article impact. AMIA Annu Symp Proc. 2011;2011:374–381. , .
- Peer review: trial by Twitter. Nature. 2011;469(7330):286–287. .
- Social media release increases dissemination of original articles in the clinical pain sciences. PLoS One. 2013;8(7):e68914. , , , .
- Can tweets predict citations? Metrics of social impact based on Twitter and correlation with traditional metrics of scientific impact. J Med Internet Res. 2011;13(4):e123. .
- Do altmetrics work? Twitter and ten other social web services. PLoS One. 2013;8(5):e64841. , , , .
Only 20 years ago, science from peer‐reviewed journals was still distributed and consumed in the same fashion that evolved from the earliest days of medical science: in print at monthly or weekly intervals. The Internet radically accelerated this paradigm but left the essential processes intact; journals could publish the information and readers could read it more easily, but the basic forums for interaction and discussion over the content remained the same. Enter Web 2.0 and the era of social media. Authors, editors, and readers can now interact easily with each other over the content in real time and across great distances.
Social media may not have changed the way science is produced and reviewed, but it is certainly changing how people consume and use the science. Some have suggested that social media activity around particular articles or journals may be a more important measure of impact than traditional measures of citation,[1] and others have suggested that Twitter activity in particular has changed both the speed and quality of discussion about new studies within the scientific community.[2] In the face of these trends, the Journal of Hospital Medicine (JHM) has decided to develop a bold strategy for leadership in this emerging area, with an initial focus on increasing JHM's activity and visibility on Twitter.
As part of this initial focus, JHM has successfully developed and implemented a protocol for use by authors to compose 2 Tweets describing their publications: the first announces the article's publication (e.g., New evidence on white coats and risk for hospital‐acquired infections), and the second promotes a key point from the article (e.g., Does the doctor's white coat spread hospital infection?). These Tweets are encouraged (but not required) from the corresponding author for every article in every edition, and JHM's editorial staff works with individual authors to refine their message and maximize their impact. To help authors, we have developed several tips for effective tweeting (Table 1).
1. Make it short:The limit is 140 characters, but getting retweets requires additional room for others to add their 2 cents, so try to get it under 100 characters. |
2. Make it simple: If your tweet includes complex terminology or analytic methods, it is not likely to get picked up. Make it easy to read for the lay public. |
3. Make it clear: Your article may have several conclusions, but pick the most newsworthy for the general public. It is usually best to focus on the main finding. |
4. Pose a question: Raise interest by piquing the curiosity of potential readers. A good question can motivate readers to click on your article to find the answer. |
5. Add a hashtag: Hashtags index tweets on Twitter. It is best to pick 1 or 2 existing tags from the healthcare hashtag project that fit the focus of your article ( |
6. Build your following: Include your Twitter handle to alert current/prospective followers of your publication. |
Even after just 1 year of this Twitter‐focused strategy, we are already seeing noteworthy impact and have learned several lessons.
AUTHORS CAN AND WILL GENERATE TWEETS FOR THEIR ARTICLES
When we started asking authors to generate tweets for their articles, Twitter was relatively new, and we were unsure if authors would be willing and able to participate. Since we started, we have noticed a steady increase in the number of author‐generated tweets. Today, more than three‐quarters of tweets per issue are author generated. Anecdotal feedback has been very positive, and authors have expressed interest in the plan for tweeting as well as feedback on how well their tweets were written. If authors or institutions are on Twitter, we also encourage using the Twitter name or handle in the tweet, which serves as a way for others on Twitter to identify directly with the author or institution and often results in greater interest in a particular tweet. Of note, authors have no obligation to become regular users of Twitter or engage with followers of JHM's Twitter feed, but many find themselves following the journal's feed more closely (and responding to posts by other authors) once they have joined Twitter and tweeted about their own work via JHM.
#HASHTAGS MAKE IT HAPPEN
Because Twitter users are a very large crowd of people with diverse interests, it is important to target tweets to the groups that would be most interested in studies. The use of hashtags makes it easy to index tweets. One of the major edits of author‐generated tweets that we provide is to index the articles to the most popular hashtags. For example, medical education studies can be indexed under #meded, which is a popular hashtag for clinician educators. Other important hashtags for hospitalists include #ptsafety, #readmissions, #healthpolicy, #healthcosts, or #infectiousdisease. To select hashtags, we have found the healthcare hashtag directory maintained by Symplur (Upland, CA;
HIGH IMPACT STUDIES MAKE A BIGGER IMPACT ON TWITTER
We observed a high number of retweets and comments about articles that were the most viewed studies on JHM online, referring to Project BOOST (Better Outcomes for Older Adults Through Safe Transitions) and the Society of Hospital Medicine's Choosing Wisely campaign. This is not surprising given the national focus on readmissions as well as cost‐conscious care. Moreover, our experience is in line with observations that Twitter provides an additional source of page views and article downloads for medical journals[3] and research, which demonstrates that studies that are tweeted will eventually be cited more.[4, 5]
TECHNOLOGY STUDIES ARE ADORED BY TWITTER
Studies and research examining the use of smartphones, apps, or social media in healthcare draw a lot of attention on Twitter, particularly from other technophiles in healthcare who often use the #hscm healthcare social media hashtag. Such studies often resonate with Twitter users, who tend to be engaged in technology at a high level and are interested in how to advance the use of technology in the healthcare workplace.
JHM's social media strategy has already been very successful in its early implementation; the JHM twitter feed has >600 followers. Although most authors submit their own tweets (71/117 or 61% of articles over the last year), JHM has also created social media roles for editors to fill in tweets when missing and ensure timely and consistent output from the JHM feed. We have also started a Facebook page, with a rapidly growing number of followers, and we continue to see our social media influence scores rise. In the next year we hope to develop a JHM blog, with invited commentary as well as a process for unsolicited submissions from our readership.
Increasingly, a journal's impact (small i) is measured not only in the traditional metric of impact factor (a representation of the number of papers cited in a given journal publication year), but also by the journal's ability to disseminate knowledge and awareness of issues key to the field. Social media is a major element of the next phase of evidence dissemination, and JHM is pleased to be developing and growing its footprint in the digital world.
Only 20 years ago, science from peer‐reviewed journals was still distributed and consumed in the same fashion that evolved from the earliest days of medical science: in print at monthly or weekly intervals. The Internet radically accelerated this paradigm but left the essential processes intact; journals could publish the information and readers could read it more easily, but the basic forums for interaction and discussion over the content remained the same. Enter Web 2.0 and the era of social media. Authors, editors, and readers can now interact easily with each other over the content in real time and across great distances.
Social media may not have changed the way science is produced and reviewed, but it is certainly changing how people consume and use the science. Some have suggested that social media activity around particular articles or journals may be a more important measure of impact than traditional measures of citation,[1] and others have suggested that Twitter activity in particular has changed both the speed and quality of discussion about new studies within the scientific community.[2] In the face of these trends, the Journal of Hospital Medicine (JHM) has decided to develop a bold strategy for leadership in this emerging area, with an initial focus on increasing JHM's activity and visibility on Twitter.
As part of this initial focus, JHM has successfully developed and implemented a protocol for use by authors to compose 2 Tweets describing their publications: the first announces the article's publication (e.g., New evidence on white coats and risk for hospital‐acquired infections), and the second promotes a key point from the article (e.g., Does the doctor's white coat spread hospital infection?). These Tweets are encouraged (but not required) from the corresponding author for every article in every edition, and JHM's editorial staff works with individual authors to refine their message and maximize their impact. To help authors, we have developed several tips for effective tweeting (Table 1).
1. Make it short:The limit is 140 characters, but getting retweets requires additional room for others to add their 2 cents, so try to get it under 100 characters. |
2. Make it simple: If your tweet includes complex terminology or analytic methods, it is not likely to get picked up. Make it easy to read for the lay public. |
3. Make it clear: Your article may have several conclusions, but pick the most newsworthy for the general public. It is usually best to focus on the main finding. |
4. Pose a question: Raise interest by piquing the curiosity of potential readers. A good question can motivate readers to click on your article to find the answer. |
5. Add a hashtag: Hashtags index tweets on Twitter. It is best to pick 1 or 2 existing tags from the healthcare hashtag project that fit the focus of your article ( |
6. Build your following: Include your Twitter handle to alert current/prospective followers of your publication. |
Even after just 1 year of this Twitter‐focused strategy, we are already seeing noteworthy impact and have learned several lessons.
AUTHORS CAN AND WILL GENERATE TWEETS FOR THEIR ARTICLES
When we started asking authors to generate tweets for their articles, Twitter was relatively new, and we were unsure if authors would be willing and able to participate. Since we started, we have noticed a steady increase in the number of author‐generated tweets. Today, more than three‐quarters of tweets per issue are author generated. Anecdotal feedback has been very positive, and authors have expressed interest in the plan for tweeting as well as feedback on how well their tweets were written. If authors or institutions are on Twitter, we also encourage using the Twitter name or handle in the tweet, which serves as a way for others on Twitter to identify directly with the author or institution and often results in greater interest in a particular tweet. Of note, authors have no obligation to become regular users of Twitter or engage with followers of JHM's Twitter feed, but many find themselves following the journal's feed more closely (and responding to posts by other authors) once they have joined Twitter and tweeted about their own work via JHM.
#HASHTAGS MAKE IT HAPPEN
Because Twitter users are a very large crowd of people with diverse interests, it is important to target tweets to the groups that would be most interested in studies. The use of hashtags makes it easy to index tweets. One of the major edits of author‐generated tweets that we provide is to index the articles to the most popular hashtags. For example, medical education studies can be indexed under #meded, which is a popular hashtag for clinician educators. Other important hashtags for hospitalists include #ptsafety, #readmissions, #healthpolicy, #healthcosts, or #infectiousdisease. To select hashtags, we have found the healthcare hashtag directory maintained by Symplur (Upland, CA;
HIGH IMPACT STUDIES MAKE A BIGGER IMPACT ON TWITTER
We observed a high number of retweets and comments about articles that were the most viewed studies on JHM online, referring to Project BOOST (Better Outcomes for Older Adults Through Safe Transitions) and the Society of Hospital Medicine's Choosing Wisely campaign. This is not surprising given the national focus on readmissions as well as cost‐conscious care. Moreover, our experience is in line with observations that Twitter provides an additional source of page views and article downloads for medical journals[3] and research, which demonstrates that studies that are tweeted will eventually be cited more.[4, 5]
TECHNOLOGY STUDIES ARE ADORED BY TWITTER
Studies and research examining the use of smartphones, apps, or social media in healthcare draw a lot of attention on Twitter, particularly from other technophiles in healthcare who often use the #hscm healthcare social media hashtag. Such studies often resonate with Twitter users, who tend to be engaged in technology at a high level and are interested in how to advance the use of technology in the healthcare workplace.
JHM's social media strategy has already been very successful in its early implementation; the JHM twitter feed has >600 followers. Although most authors submit their own tweets (71/117 or 61% of articles over the last year), JHM has also created social media roles for editors to fill in tweets when missing and ensure timely and consistent output from the JHM feed. We have also started a Facebook page, with a rapidly growing number of followers, and we continue to see our social media influence scores rise. In the next year we hope to develop a JHM blog, with invited commentary as well as a process for unsolicited submissions from our readership.
Increasingly, a journal's impact (small i) is measured not only in the traditional metric of impact factor (a representation of the number of papers cited in a given journal publication year), but also by the journal's ability to disseminate knowledge and awareness of issues key to the field. Social media is a major element of the next phase of evidence dissemination, and JHM is pleased to be developing and growing its footprint in the digital world.
- Exploring the use of social media to measure journal article impact. AMIA Annu Symp Proc. 2011;2011:374–381. , .
- Peer review: trial by Twitter. Nature. 2011;469(7330):286–287. .
- Social media release increases dissemination of original articles in the clinical pain sciences. PLoS One. 2013;8(7):e68914. , , , .
- Can tweets predict citations? Metrics of social impact based on Twitter and correlation with traditional metrics of scientific impact. J Med Internet Res. 2011;13(4):e123. .
- Do altmetrics work? Twitter and ten other social web services. PLoS One. 2013;8(5):e64841. , , , .
- Exploring the use of social media to measure journal article impact. AMIA Annu Symp Proc. 2011;2011:374–381. , .
- Peer review: trial by Twitter. Nature. 2011;469(7330):286–287. .
- Social media release increases dissemination of original articles in the clinical pain sciences. PLoS One. 2013;8(7):e68914. , , , .
- Can tweets predict citations? Metrics of social impact based on Twitter and correlation with traditional metrics of scientific impact. J Med Internet Res. 2011;13(4):e123. .
- Do altmetrics work? Twitter and ten other social web services. PLoS One. 2013;8(5):e64841. , , , .
Functional Status
Hospital readmission is not a new problem, but ever since the Centers for Medicaid and Medicare Services (CMS) announced that hospital reimbursement would be linked to readmission rates, the quest to understand drivers of this outcome has taken on new and remarkable vigor. Despite the avalanche of new studies on readmission factors[1] and transition interventions,[2, 3] surprisingly few have focused on conditions more prevalent in the aging Medicare population such as functional limitations. This trend in the literature reflects what is perhaps the greatest irony of the CMS readmission policy itself: while focused on improving care for a predominantly over 65‐year‐old population, it is agnostic to core geriatric vulnerabilities like function and cognition.
In this issue of the Journal of Hospital Medicine, Hoyer and colleagues take an important first step toward exploring such vulnerabilities.[4] Although it may not surprise many hospitalists that these play a role in complex outcomes such as readmission, the effects reported here are striking. The odds for readmission were 300% higher for patients with the lowest functional scores compared to those with highest scores after adjusting for other known factors such as comorbidities, age, and severity of illness. In terms of readmission rates, 29% of functionally impaired medical patients were readmitted compared to 11% of those with high function. Similar but less profound trends were seen in patients discharged from neurology and orthopedic services.
Although this was a single‐site study, and functional assessments were made on admission to an acute rehabilitation facility after hospital discharge, these findings are compelling and suggest many important areas for future research. First, these results suggest a need for replication in nationally representative data to better understand their scope and generalizability. Certainly, the number of participants (9405 patients) gives this study plenty of power; however, the sample is limited in that presumably all patients had some level of functional decline, but enough potential for functional recovery to warrant discharge to acute rehabilitation. We do not know what effects functional limitations might have on patients discharged to other settings (eg, community with home rehabilitation or skilled nursing facility with rehabilitation). Thus, future research should examine whether the impact of functional limitations described in this sample applies to the larger universe of hospital discharges.
We also do not know anything about the functional status of these patients at admission or their functional trajectory prior to hospitalization, which limits conclusions about whether the disabilities observed were hospital acquired. Functional ability, like vital signs, can be quite variable during the course of acute illness and should be interpreted in the context of each patient's baseline. The functional trajectory for a patient who was impaired at the time of hospital discharge, but independent before hospitalization, is likely very different than one who was chronically impaired at baseline. Thus, postdischarge is only half the story at best, and future research should explore the functional status and trajectory of patients before admission too.
Finally, to assess functional status, the authors of this study used the Functional Independence Measure (FIM) score, a well‐validated instrument used in rehabilitation facilities. One advantage of using this measure to predict readmission is that in addition to 12 items that assess physical domains overlapping with the Activities of Daily Living (ADL) measures commonly used in hospitals, it also includes 5 items about cognition and thus gives an overall view of both physical and mental status in context of functional ability. On the down side, the FIM score is less well known in the acute care setting and does not include instrumental ADLs, such as shopping, housekeeping, food preparation/cleanup, telephone, transportation, and technology like computers, that are often important for patients returning home. Given the interesting findings by Hoyer et al., future research should explore possible associations with these activities in patients discharged to community as well.
The results by Hoyer et al. also have important implications for policy and practice. At the level of national policy and ongoing healthcare reform, Medicare should consider ways to incentivize hospitals to collect data on functional status of patients more consistently. Currently, there is no International Classification of Diseases, 9th Revision code to capture functional limitation during hospitalization as a diagnosis or comorbidity (whether hospital acquired or not), which precludes any discussion about including functional status as an adjustor in the current CMS model for expected readmission rates for hospitals. Regardless of CMS policy and performance incentives or penalties, a lot more could be done at the level of hospital policy and practice to improve screening for functional vulnerabilities on admission and prior to discharge. Although this may require greater investment in standardizing physical therapy evaluation for most patients (especially those over 65 years old), the increased readmission rates found by Hoyer et al. in functionally impaired patients suggest it would be penny wise but pound foolish not to do so. In other words, if hospitals want to reduce their readmission rates by identifying and intervening on high‐risk patients, identifying functionally impaired patients seems to be the low‐hanging fruit.
In summary, Hoyer and colleagues have made an important contribution to the ever‐expanding literature on readmission risk factors, but they have likely just identified the tip of the iceberg. As Medicare enrollment continues to climb with the growth of baby boomers over 65 years old, the demand for acute care in older adults will continue to grow.[5] Moreover, as pressure mounts to improve the quality and reduce the costs of hospital care, greater understanding of geriatric vulnerabilities in this population will be increasingly important.
- Risk prediction models for hospital readmission: a systematic review. JAMA. 2011;306(15):1688–1698. , , , et al.
- Interventions to reduce 30‐day rehospitalization: a systematic review. Ann Intern Med. 2011;155(8):520–528. , , , , .
- Hospital‐initiated transitional care interventions as a patient safety strategy: a systematic review. Ann Intern Med. 2013;158:433–440. , , , , , .
- J Hosp Med. 2014;9(5):277–282. et al.
- US population aging and demand for inpatient services. J Hosp Med. 2014;9(3):193–196. et al.
Hospital readmission is not a new problem, but ever since the Centers for Medicaid and Medicare Services (CMS) announced that hospital reimbursement would be linked to readmission rates, the quest to understand drivers of this outcome has taken on new and remarkable vigor. Despite the avalanche of new studies on readmission factors[1] and transition interventions,[2, 3] surprisingly few have focused on conditions more prevalent in the aging Medicare population such as functional limitations. This trend in the literature reflects what is perhaps the greatest irony of the CMS readmission policy itself: while focused on improving care for a predominantly over 65‐year‐old population, it is agnostic to core geriatric vulnerabilities like function and cognition.
In this issue of the Journal of Hospital Medicine, Hoyer and colleagues take an important first step toward exploring such vulnerabilities.[4] Although it may not surprise many hospitalists that these play a role in complex outcomes such as readmission, the effects reported here are striking. The odds for readmission were 300% higher for patients with the lowest functional scores compared to those with highest scores after adjusting for other known factors such as comorbidities, age, and severity of illness. In terms of readmission rates, 29% of functionally impaired medical patients were readmitted compared to 11% of those with high function. Similar but less profound trends were seen in patients discharged from neurology and orthopedic services.
Although this was a single‐site study, and functional assessments were made on admission to an acute rehabilitation facility after hospital discharge, these findings are compelling and suggest many important areas for future research. First, these results suggest a need for replication in nationally representative data to better understand their scope and generalizability. Certainly, the number of participants (9405 patients) gives this study plenty of power; however, the sample is limited in that presumably all patients had some level of functional decline, but enough potential for functional recovery to warrant discharge to acute rehabilitation. We do not know what effects functional limitations might have on patients discharged to other settings (eg, community with home rehabilitation or skilled nursing facility with rehabilitation). Thus, future research should examine whether the impact of functional limitations described in this sample applies to the larger universe of hospital discharges.
We also do not know anything about the functional status of these patients at admission or their functional trajectory prior to hospitalization, which limits conclusions about whether the disabilities observed were hospital acquired. Functional ability, like vital signs, can be quite variable during the course of acute illness and should be interpreted in the context of each patient's baseline. The functional trajectory for a patient who was impaired at the time of hospital discharge, but independent before hospitalization, is likely very different than one who was chronically impaired at baseline. Thus, postdischarge is only half the story at best, and future research should explore the functional status and trajectory of patients before admission too.
Finally, to assess functional status, the authors of this study used the Functional Independence Measure (FIM) score, a well‐validated instrument used in rehabilitation facilities. One advantage of using this measure to predict readmission is that in addition to 12 items that assess physical domains overlapping with the Activities of Daily Living (ADL) measures commonly used in hospitals, it also includes 5 items about cognition and thus gives an overall view of both physical and mental status in context of functional ability. On the down side, the FIM score is less well known in the acute care setting and does not include instrumental ADLs, such as shopping, housekeeping, food preparation/cleanup, telephone, transportation, and technology like computers, that are often important for patients returning home. Given the interesting findings by Hoyer et al., future research should explore possible associations with these activities in patients discharged to community as well.
The results by Hoyer et al. also have important implications for policy and practice. At the level of national policy and ongoing healthcare reform, Medicare should consider ways to incentivize hospitals to collect data on functional status of patients more consistently. Currently, there is no International Classification of Diseases, 9th Revision code to capture functional limitation during hospitalization as a diagnosis or comorbidity (whether hospital acquired or not), which precludes any discussion about including functional status as an adjustor in the current CMS model for expected readmission rates for hospitals. Regardless of CMS policy and performance incentives or penalties, a lot more could be done at the level of hospital policy and practice to improve screening for functional vulnerabilities on admission and prior to discharge. Although this may require greater investment in standardizing physical therapy evaluation for most patients (especially those over 65 years old), the increased readmission rates found by Hoyer et al. in functionally impaired patients suggest it would be penny wise but pound foolish not to do so. In other words, if hospitals want to reduce their readmission rates by identifying and intervening on high‐risk patients, identifying functionally impaired patients seems to be the low‐hanging fruit.
In summary, Hoyer and colleagues have made an important contribution to the ever‐expanding literature on readmission risk factors, but they have likely just identified the tip of the iceberg. As Medicare enrollment continues to climb with the growth of baby boomers over 65 years old, the demand for acute care in older adults will continue to grow.[5] Moreover, as pressure mounts to improve the quality and reduce the costs of hospital care, greater understanding of geriatric vulnerabilities in this population will be increasingly important.
Hospital readmission is not a new problem, but ever since the Centers for Medicaid and Medicare Services (CMS) announced that hospital reimbursement would be linked to readmission rates, the quest to understand drivers of this outcome has taken on new and remarkable vigor. Despite the avalanche of new studies on readmission factors[1] and transition interventions,[2, 3] surprisingly few have focused on conditions more prevalent in the aging Medicare population such as functional limitations. This trend in the literature reflects what is perhaps the greatest irony of the CMS readmission policy itself: while focused on improving care for a predominantly over 65‐year‐old population, it is agnostic to core geriatric vulnerabilities like function and cognition.
In this issue of the Journal of Hospital Medicine, Hoyer and colleagues take an important first step toward exploring such vulnerabilities.[4] Although it may not surprise many hospitalists that these play a role in complex outcomes such as readmission, the effects reported here are striking. The odds for readmission were 300% higher for patients with the lowest functional scores compared to those with highest scores after adjusting for other known factors such as comorbidities, age, and severity of illness. In terms of readmission rates, 29% of functionally impaired medical patients were readmitted compared to 11% of those with high function. Similar but less profound trends were seen in patients discharged from neurology and orthopedic services.
Although this was a single‐site study, and functional assessments were made on admission to an acute rehabilitation facility after hospital discharge, these findings are compelling and suggest many important areas for future research. First, these results suggest a need for replication in nationally representative data to better understand their scope and generalizability. Certainly, the number of participants (9405 patients) gives this study plenty of power; however, the sample is limited in that presumably all patients had some level of functional decline, but enough potential for functional recovery to warrant discharge to acute rehabilitation. We do not know what effects functional limitations might have on patients discharged to other settings (eg, community with home rehabilitation or skilled nursing facility with rehabilitation). Thus, future research should examine whether the impact of functional limitations described in this sample applies to the larger universe of hospital discharges.
We also do not know anything about the functional status of these patients at admission or their functional trajectory prior to hospitalization, which limits conclusions about whether the disabilities observed were hospital acquired. Functional ability, like vital signs, can be quite variable during the course of acute illness and should be interpreted in the context of each patient's baseline. The functional trajectory for a patient who was impaired at the time of hospital discharge, but independent before hospitalization, is likely very different than one who was chronically impaired at baseline. Thus, postdischarge is only half the story at best, and future research should explore the functional status and trajectory of patients before admission too.
Finally, to assess functional status, the authors of this study used the Functional Independence Measure (FIM) score, a well‐validated instrument used in rehabilitation facilities. One advantage of using this measure to predict readmission is that in addition to 12 items that assess physical domains overlapping with the Activities of Daily Living (ADL) measures commonly used in hospitals, it also includes 5 items about cognition and thus gives an overall view of both physical and mental status in context of functional ability. On the down side, the FIM score is less well known in the acute care setting and does not include instrumental ADLs, such as shopping, housekeeping, food preparation/cleanup, telephone, transportation, and technology like computers, that are often important for patients returning home. Given the interesting findings by Hoyer et al., future research should explore possible associations with these activities in patients discharged to community as well.
The results by Hoyer et al. also have important implications for policy and practice. At the level of national policy and ongoing healthcare reform, Medicare should consider ways to incentivize hospitals to collect data on functional status of patients more consistently. Currently, there is no International Classification of Diseases, 9th Revision code to capture functional limitation during hospitalization as a diagnosis or comorbidity (whether hospital acquired or not), which precludes any discussion about including functional status as an adjustor in the current CMS model for expected readmission rates for hospitals. Regardless of CMS policy and performance incentives or penalties, a lot more could be done at the level of hospital policy and practice to improve screening for functional vulnerabilities on admission and prior to discharge. Although this may require greater investment in standardizing physical therapy evaluation for most patients (especially those over 65 years old), the increased readmission rates found by Hoyer et al. in functionally impaired patients suggest it would be penny wise but pound foolish not to do so. In other words, if hospitals want to reduce their readmission rates by identifying and intervening on high‐risk patients, identifying functionally impaired patients seems to be the low‐hanging fruit.
In summary, Hoyer and colleagues have made an important contribution to the ever‐expanding literature on readmission risk factors, but they have likely just identified the tip of the iceberg. As Medicare enrollment continues to climb with the growth of baby boomers over 65 years old, the demand for acute care in older adults will continue to grow.[5] Moreover, as pressure mounts to improve the quality and reduce the costs of hospital care, greater understanding of geriatric vulnerabilities in this population will be increasingly important.
- Risk prediction models for hospital readmission: a systematic review. JAMA. 2011;306(15):1688–1698. , , , et al.
- Interventions to reduce 30‐day rehospitalization: a systematic review. Ann Intern Med. 2011;155(8):520–528. , , , , .
- Hospital‐initiated transitional care interventions as a patient safety strategy: a systematic review. Ann Intern Med. 2013;158:433–440. , , , , , .
- J Hosp Med. 2014;9(5):277–282. et al.
- US population aging and demand for inpatient services. J Hosp Med. 2014;9(3):193–196. et al.
- Risk prediction models for hospital readmission: a systematic review. JAMA. 2011;306(15):1688–1698. , , , et al.
- Interventions to reduce 30‐day rehospitalization: a systematic review. Ann Intern Med. 2011;155(8):520–528. , , , , .
- Hospital‐initiated transitional care interventions as a patient safety strategy: a systematic review. Ann Intern Med. 2013;158:433–440. , , , , , .
- J Hosp Med. 2014;9(5):277–282. et al.
- US population aging and demand for inpatient services. J Hosp Med. 2014;9(3):193–196. et al.
Tablet Computers to Engage Patients
BACKGROUND
Many hospitals have initiated intense efforts to improve transitions of care[1] such as discharge coordinators or transition coaches,[2, 3] but use of mobile devices as approaches to add or extend the value of human interventions have been understudied.[4] Additionally, many hospitalized patients experience substantial inactive time between provider visits, tests, and treatments. This time could be used to engage patients in their care through interactive health education modules and by learning to use their PHR to manage medications and postdischarge appointments.
Greater understanding of the advantages and limitations of mobile devices may be important for improving transitions of care and may help leverage existing hospital personnel resources. However, prior studies have focused on healthcare provider uses of tablet computers for medical education,[5] to collect clinical registration data,[6] or to do clinical work (eg, check labs, write notes)[7, 8, 9] primarily in outpatient settings; few studies have focused on patient uses for this technology in hospital settings.[10, 11] To address these knowledge gaps, we conducted a pilot project to explore inpatient satisfaction with bedside tablets and barriers to usability. Additionally, we evaluated use of these devices to deliver 2 specific Web‐based programs: (1) an interactive video to improve inpatient education about hospital safety, and (2) PHR access to promote inpatient engagement in discharge planning.
METHODS
Study Design, Patient Selection, and Devices/Programs
We conducted a prospective study of tablet computers to engage patients in their care and discharge planning through Web‐based interactive health education modules and use of PHRs. We used 2 tablets, distributed daily by research assistants (RAs) to eligible patients after morning rounds. Inclusion criteria for patients were ability to speak English and admission to the medical (hospitalist) service at University of California San Francisco (UCSF) Medical Center. Exclusion criteria were intensive care unit admission, contact isolation, or inability to complete the consent process due to altered mental status or cognitive impairment.
RAs screened patients for inclusion/exclusion via the electronic medical record and then approached them after rounds for enrollment (11:00 am1:00 pm). RAs then performed a tiered orientation tailored to individual patient experience and needs. First, they delivered a brief tutorial focused on the tablet itself and its basic functions (touchscreen, keypad, and Internet browser use). Second, RAs showed patients how to access the online educational health module and how to navigate content within the module. RAs next explained what the PHR is and demonstrated how to login, how to navigate tabs within the PHR, and how to perform basic tasks (view/refill medications, view/modify appointments, and view/send messages to providers). The RAs left devices with patients for 3 to 5 hours and returned to collect them and perform debriefing interviews. After each device was returned, RAs cleaned devices with disinfectant wipes available in patient rooms and checked for physical damage or software malfunctions (eg, unable to turn on or unlock). Finally, RAs used the reset function to erase any personal data or setting modifications made by patients and docked the devices overnight to resynchronize the original settings and recharge the batteries.
We used the 16 gigabyte Apple iPad2 (Apple Inc., Cupertino, CA) without any enclosures, cases, or security devices. Our educational health module was Patient Safety in the Hospital, which was professionally developed by Emmi Solutions (
Survey Instruments and Data Collection
We developed pre‐ and postintervention surveys to characterize patients' demographics, device ownership, and health‐related Internet activities in the last year based on questions used in the Centers for Disease Control and Prevention National Health Interview Study (
Analyses
We used frequency analysis to describe patient demographics, ability to complete online health educational modules, and utilization of their PHR. We performed bivariate analyses (Fisher exact test) to assess correlations between demographics (age, device ownership, Internet use) and key pilot program performance measures (orientation time 15 minutes, online health module completion, and completion of 1 essential function in the PHR). All analyses were performed in SAS 9.2 (SAS Institute Inc., Cary, NC). The institutional review board of record for UCSF approved this study.
RESULTS
As shown in Table 1, we enrolled 30 patients. Most participants (60%) were aged 40 years or older, and most (87%) owned a mobile device; 70% owned a laptop and 60% owned a smartphone, but few (22%) owned a computer tablet. Most participants accessed the Internet daily, but fewer reported Internet use for health tasks; about half (52%) communicated with a provider, but few refilled a prescription (27%) or scheduled an appointment (21%) online over the last year.
Characteristic | No. (%) |
---|---|
Age, y | |
1839 | 11 (38%) |
4049 | 5 (18%) |
5059 | 4 (14%) |
6069 | 5 (18%) |
7079 | 3 (10%) |
Gender, female | 17 (60%) |
Device ownership | |
Desktop computer | 12 (44%) |
Laptop computer | 19 (70%) |
Smart phone | 17 (60%) |
Tablet computer | 6 (22%) |
Any mobile device (laptop, smartphone, or tablet) | 26 (87%) |
Internet use | |
Daily | 21 (72%) |
Several times a week | 3 (10%) |
Once a week or less | 5 (18%) |
Prestudy online health tasks | |
Looked up health information | 21 (72%) |
Communicated with provider | 15 (52%) |
Refilled prescription | 8 (27%) |
Scheduled medical appointment | 6 (21%) |
Nearly all participants (90%) were satisfied or very satisfied with their experience using the tablet in the hospital (Figure 1). Most (87%) required 30 minutes or less for basic orientation, and 70% required only 15 minutes or less. Most participants (83%) were able to independently complete an interactive health education module on hospital safety and were highly satisfied with the module. Despite the fact that 73% of participants were first‐time users of our PHR, the majority were able to login and independently access their medication list, verify scheduled appointments, or send a secure message to their primary care provider.
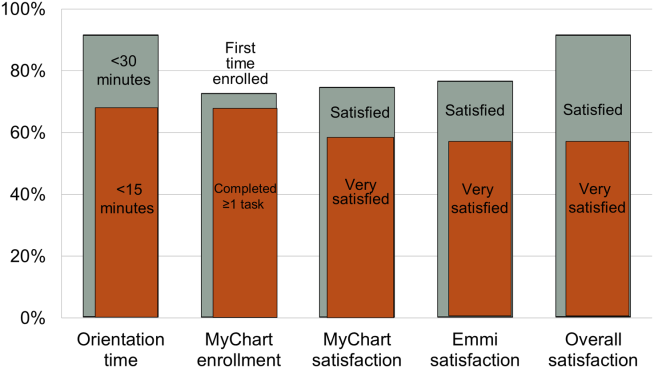
Participants aged 50 years or older were less likely to complete orientation in 15 minutes or less compared to those under 50 years old (25% vs 79%, P=0.025); however, age was not a significant factor in ability to complete the online health educational module or perform at least 1 essential PHR function. Other demographic features, such as device ownership and daily Internet use, did not correlate with shorter orientation time, educational module completion, or PHR use (data available on request).
Participants also made suggestions for improvement during the debrief interviews. Several suggested applications for entertainment (gaming, magazines, or music) and 2 suggested that all patients should be introduced to their PHR during hospitalization (data available on request). No device software malfunction (eg, device freezes, Internet connection failures), hardware issues (eg, damage from falls, wetness, or repeated disinfectant exposure), or theft or misappropriation were reported by patients or observed by the RAs to date.
DISCUSSION
Our pilot study suggests that tablet‐based access to educational modules and PHRs can increase inpatient engagement in care with high satisfaction and minimal time for orientation. Surprisingly, even older patients and those who might be considered less tech savvy in terms of Internet use and device ownership were equally able to utilize our tablet interventions. Furthermore, we did not experience any hardware issues in the harsh physical environment of inpatient wards. These preliminary findings suggest the potential utility of tablets for clinically meaningful tasks by inpatients with a low rate of technical issues.
From a technical standpoint, our experience suggests several next steps. First, although orientation time was minimal, it might be even less if patients used their own mobile devices because most patients already owned one. This bring your own device (BYOD) approach could also promote postdischarge patient engagement. Second, the flexibility of a BYOD approach raises the question of whether to also allow patients a choice of application‐based versus browser‐based platforms for specific programs such as the PHR and educational video we used. Indeed, although we used a browser‐based approach, several other teams have developed patient‐facing engagement applications (or apps) for mobile devices,[4, 12] and hospitalists could prescribe apps at discharge as a more providers are now doing in outpatient settings.[13] Of course, maximizing flexibility for BYOD and Web‐based versus app‐based approaches would likely increase patient engagement but would come at the cost of more time and effort for hospital providers to vet apps/websites and educate patients about their use. Third, regardless of the devices and programs used, broader engagement of patients, nurses, hospitalists, and other providers will be needed in the future to identify key areas for development to avoid overburdening patients and providers.
From a quality‐improvement perspective, recent literature has considered broad clinical uses for tablets by hospital providers,[14, 15] but our experience suggests more specific opportunities to improve transitions of care though direct patient engagement. Tablets and other mobile devices may help improve discharge education for patients taking high‐risk medications such as warfarin or insulin using interactive educational modules similar to the hospital safety modules we used. Additionally, clinical staff, such as nurses and pharmacists, can be trained to deliver mobile device interventions such as education on high‐risk medications.[16] Ultimately, scale up for our intervention will require that mobile devices and content eventually improve and replace current practices by hospital staff (especially nurses) in a way that streamlines, rather than compounds, current workflow. This could increase efficiency in these discharge tasks and extend contributions of these providers to high‐quality transitions.
Our study has several limitations. First, although this is a pilot study with only 30 patients, it adds needed scale to much smaller (N=58) published feasibility studies of tablet computer use by inpatients.[11, 12] Beyond more robust feasibility testing, our study adds new data about mobile device use for specific clinical tasks in the hospital such as patient education and PHR use. Second, we did not track postdischarge outcomes to test the effects of our intervention on transition care quality; this will be a focus of our future research. Third, we used existing platforms for interactive educational modules and PHR access at our site; participant satisfaction in our study may not generalize to other platforms. Furthermore, most PHR platforms (including ours) are not optimally configured to engage patients during transitions of care, but we plan to integrate existing functions (such as ability to refill medications or change appointments) into discharge education and planning. Finally, we have not engaged caregivers as surrogates for cognitively impaired patients or adapted our platform for non‐English speakers; these are areas for development in our ongoing work. Overall, our pilot results help set the stage to deploy mobile devices for better patient monitoring, engagement, and quality of care in the inpatient setting.[17]
In conclusion, our pilot project demonstrates that tablet computers can be used to improve inpatient education and patient engagement in discharge planning. Inpatients are highly satisfied with the use of tablets to complete health education modules and access their PHR, with minimal time required for patient training and device management by hospital staff. Tablets and other mobile devices have significant potential to improve patients' education and engagement in their hospital care.
Acknowledgements
The authors thank the UCSF mHealth group and Center for Digital Health Innovation for advice and for providing tablet computers for this pilot project.
Disclosures: This article was presented as a finalist in the Research, Innovations, and Clinical Vignettes competition (Innovations category) at the 2013 Annual Meeting of the Society for Hospital Medicine. Dr. Auerbach was supported by grant K24HL098372 (NHLBI). Dr. Greysen was supported by a career development award (KL‐2) from the UCSF Clinical Translational Sciences Institute. The authors have declared they have no financial, personal, or other conflicts of interest relevant to this study.
- Hospital readmissions and the Affordable Care Act: paying for coordinated quality care. JAMA. 2011;306(16):1794–1795. , .
- A reengineered hospital discharge program to decrease re‐hospitalization. Ann Intern Med. 2009;150:178–187. , , , et al.
- The care transitions intervention: results of a randomized controlled trial. Arch Intern Med. 2006;166:1822–1828. , , , .
- Project RED. Meet Louise…and virtual patient advocates. Available at: http://www.bu.edu/fammed/projectred/publications/VirtualPatientAdvocateWebsiteInfo2.pdf. Accessed July 12, 2013.
- Use of handheld computers in medical education. A systematic review. J Gen Intern Med. 2006;21(5):531–537. , , , .
- Ongoing evaluation of ease‐of‐use and usefulness of wireless tablet computers within an ambulatory care unit. Stud Health Tech Inform. 2009;143:459–464. , , , .
- Use of a tablet personal computer to enhance patient care on multidisciplinary rounds. Am J Health Syst Pharm. 2009;66(21):1909–1911. .
- Experiences incorporating Tablet PCs into clinical pharmacists' workflow. J Healthc Inf Manag. 2005;19(4):32–37. , .
- The impact of mobile handheld technology on hospital physicians' work practices and patient care: a systematic review. J Am Med Inform Assoc. 2009;16(6):792–801. , , .
- An investigation of the efficacy of electronic consenting interfaces of research permissions management system in a hospital setting. Int J Med Inform. 2013;82(9):854–863. , , , et al.
- A tablet computer application for patients to participate in their hospital care. AMIA Annu Symp Proc. 2011;2011:1428–1435. , , , et al.
- Building and testing a patient‐centric electronic bedside communication center. J Gerontol Nurs. 2013;39(1):15–19. , , , et al.
- How apps are changing family medicine. J Fam Pract. 2013Jul;62(7):362–367. .
- The iPad: gadget or medical godsend? Ann Emerg Med. 2010;56(1):A21–A22. .
- How might the iPad change healthcare? J R Soc Med. 2012;105(6):233–241. , , , et al.
- Keeping the patient focus: using tablet technology to enhance education and practice. J Contin Educ Nurs. 2012;43(6):249–250. .
- Advancing the science of mHealth. J Health Commun. 2012;17(suppl 1):5–10. , , , et al.
BACKGROUND
Many hospitals have initiated intense efforts to improve transitions of care[1] such as discharge coordinators or transition coaches,[2, 3] but use of mobile devices as approaches to add or extend the value of human interventions have been understudied.[4] Additionally, many hospitalized patients experience substantial inactive time between provider visits, tests, and treatments. This time could be used to engage patients in their care through interactive health education modules and by learning to use their PHR to manage medications and postdischarge appointments.
Greater understanding of the advantages and limitations of mobile devices may be important for improving transitions of care and may help leverage existing hospital personnel resources. However, prior studies have focused on healthcare provider uses of tablet computers for medical education,[5] to collect clinical registration data,[6] or to do clinical work (eg, check labs, write notes)[7, 8, 9] primarily in outpatient settings; few studies have focused on patient uses for this technology in hospital settings.[10, 11] To address these knowledge gaps, we conducted a pilot project to explore inpatient satisfaction with bedside tablets and barriers to usability. Additionally, we evaluated use of these devices to deliver 2 specific Web‐based programs: (1) an interactive video to improve inpatient education about hospital safety, and (2) PHR access to promote inpatient engagement in discharge planning.
METHODS
Study Design, Patient Selection, and Devices/Programs
We conducted a prospective study of tablet computers to engage patients in their care and discharge planning through Web‐based interactive health education modules and use of PHRs. We used 2 tablets, distributed daily by research assistants (RAs) to eligible patients after morning rounds. Inclusion criteria for patients were ability to speak English and admission to the medical (hospitalist) service at University of California San Francisco (UCSF) Medical Center. Exclusion criteria were intensive care unit admission, contact isolation, or inability to complete the consent process due to altered mental status or cognitive impairment.
RAs screened patients for inclusion/exclusion via the electronic medical record and then approached them after rounds for enrollment (11:00 am1:00 pm). RAs then performed a tiered orientation tailored to individual patient experience and needs. First, they delivered a brief tutorial focused on the tablet itself and its basic functions (touchscreen, keypad, and Internet browser use). Second, RAs showed patients how to access the online educational health module and how to navigate content within the module. RAs next explained what the PHR is and demonstrated how to login, how to navigate tabs within the PHR, and how to perform basic tasks (view/refill medications, view/modify appointments, and view/send messages to providers). The RAs left devices with patients for 3 to 5 hours and returned to collect them and perform debriefing interviews. After each device was returned, RAs cleaned devices with disinfectant wipes available in patient rooms and checked for physical damage or software malfunctions (eg, unable to turn on or unlock). Finally, RAs used the reset function to erase any personal data or setting modifications made by patients and docked the devices overnight to resynchronize the original settings and recharge the batteries.
We used the 16 gigabyte Apple iPad2 (Apple Inc., Cupertino, CA) without any enclosures, cases, or security devices. Our educational health module was Patient Safety in the Hospital, which was professionally developed by Emmi Solutions (
Survey Instruments and Data Collection
We developed pre‐ and postintervention surveys to characterize patients' demographics, device ownership, and health‐related Internet activities in the last year based on questions used in the Centers for Disease Control and Prevention National Health Interview Study (
Analyses
We used frequency analysis to describe patient demographics, ability to complete online health educational modules, and utilization of their PHR. We performed bivariate analyses (Fisher exact test) to assess correlations between demographics (age, device ownership, Internet use) and key pilot program performance measures (orientation time 15 minutes, online health module completion, and completion of 1 essential function in the PHR). All analyses were performed in SAS 9.2 (SAS Institute Inc., Cary, NC). The institutional review board of record for UCSF approved this study.
RESULTS
As shown in Table 1, we enrolled 30 patients. Most participants (60%) were aged 40 years or older, and most (87%) owned a mobile device; 70% owned a laptop and 60% owned a smartphone, but few (22%) owned a computer tablet. Most participants accessed the Internet daily, but fewer reported Internet use for health tasks; about half (52%) communicated with a provider, but few refilled a prescription (27%) or scheduled an appointment (21%) online over the last year.
Characteristic | No. (%) |
---|---|
Age, y | |
1839 | 11 (38%) |
4049 | 5 (18%) |
5059 | 4 (14%) |
6069 | 5 (18%) |
7079 | 3 (10%) |
Gender, female | 17 (60%) |
Device ownership | |
Desktop computer | 12 (44%) |
Laptop computer | 19 (70%) |
Smart phone | 17 (60%) |
Tablet computer | 6 (22%) |
Any mobile device (laptop, smartphone, or tablet) | 26 (87%) |
Internet use | |
Daily | 21 (72%) |
Several times a week | 3 (10%) |
Once a week or less | 5 (18%) |
Prestudy online health tasks | |
Looked up health information | 21 (72%) |
Communicated with provider | 15 (52%) |
Refilled prescription | 8 (27%) |
Scheduled medical appointment | 6 (21%) |
Nearly all participants (90%) were satisfied or very satisfied with their experience using the tablet in the hospital (Figure 1). Most (87%) required 30 minutes or less for basic orientation, and 70% required only 15 minutes or less. Most participants (83%) were able to independently complete an interactive health education module on hospital safety and were highly satisfied with the module. Despite the fact that 73% of participants were first‐time users of our PHR, the majority were able to login and independently access their medication list, verify scheduled appointments, or send a secure message to their primary care provider.
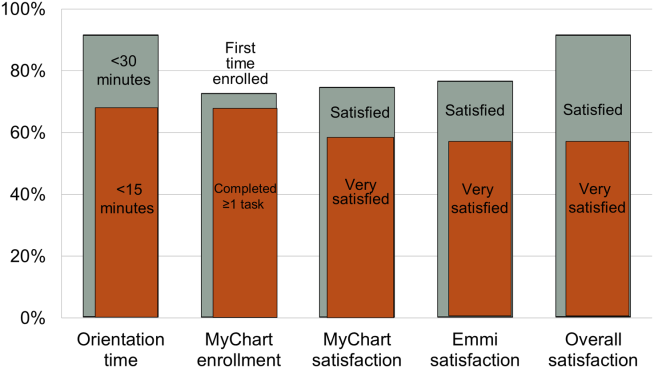
Participants aged 50 years or older were less likely to complete orientation in 15 minutes or less compared to those under 50 years old (25% vs 79%, P=0.025); however, age was not a significant factor in ability to complete the online health educational module or perform at least 1 essential PHR function. Other demographic features, such as device ownership and daily Internet use, did not correlate with shorter orientation time, educational module completion, or PHR use (data available on request).
Participants also made suggestions for improvement during the debrief interviews. Several suggested applications for entertainment (gaming, magazines, or music) and 2 suggested that all patients should be introduced to their PHR during hospitalization (data available on request). No device software malfunction (eg, device freezes, Internet connection failures), hardware issues (eg, damage from falls, wetness, or repeated disinfectant exposure), or theft or misappropriation were reported by patients or observed by the RAs to date.
DISCUSSION
Our pilot study suggests that tablet‐based access to educational modules and PHRs can increase inpatient engagement in care with high satisfaction and minimal time for orientation. Surprisingly, even older patients and those who might be considered less tech savvy in terms of Internet use and device ownership were equally able to utilize our tablet interventions. Furthermore, we did not experience any hardware issues in the harsh physical environment of inpatient wards. These preliminary findings suggest the potential utility of tablets for clinically meaningful tasks by inpatients with a low rate of technical issues.
From a technical standpoint, our experience suggests several next steps. First, although orientation time was minimal, it might be even less if patients used their own mobile devices because most patients already owned one. This bring your own device (BYOD) approach could also promote postdischarge patient engagement. Second, the flexibility of a BYOD approach raises the question of whether to also allow patients a choice of application‐based versus browser‐based platforms for specific programs such as the PHR and educational video we used. Indeed, although we used a browser‐based approach, several other teams have developed patient‐facing engagement applications (or apps) for mobile devices,[4, 12] and hospitalists could prescribe apps at discharge as a more providers are now doing in outpatient settings.[13] Of course, maximizing flexibility for BYOD and Web‐based versus app‐based approaches would likely increase patient engagement but would come at the cost of more time and effort for hospital providers to vet apps/websites and educate patients about their use. Third, regardless of the devices and programs used, broader engagement of patients, nurses, hospitalists, and other providers will be needed in the future to identify key areas for development to avoid overburdening patients and providers.
From a quality‐improvement perspective, recent literature has considered broad clinical uses for tablets by hospital providers,[14, 15] but our experience suggests more specific opportunities to improve transitions of care though direct patient engagement. Tablets and other mobile devices may help improve discharge education for patients taking high‐risk medications such as warfarin or insulin using interactive educational modules similar to the hospital safety modules we used. Additionally, clinical staff, such as nurses and pharmacists, can be trained to deliver mobile device interventions such as education on high‐risk medications.[16] Ultimately, scale up for our intervention will require that mobile devices and content eventually improve and replace current practices by hospital staff (especially nurses) in a way that streamlines, rather than compounds, current workflow. This could increase efficiency in these discharge tasks and extend contributions of these providers to high‐quality transitions.
Our study has several limitations. First, although this is a pilot study with only 30 patients, it adds needed scale to much smaller (N=58) published feasibility studies of tablet computer use by inpatients.[11, 12] Beyond more robust feasibility testing, our study adds new data about mobile device use for specific clinical tasks in the hospital such as patient education and PHR use. Second, we did not track postdischarge outcomes to test the effects of our intervention on transition care quality; this will be a focus of our future research. Third, we used existing platforms for interactive educational modules and PHR access at our site; participant satisfaction in our study may not generalize to other platforms. Furthermore, most PHR platforms (including ours) are not optimally configured to engage patients during transitions of care, but we plan to integrate existing functions (such as ability to refill medications or change appointments) into discharge education and planning. Finally, we have not engaged caregivers as surrogates for cognitively impaired patients or adapted our platform for non‐English speakers; these are areas for development in our ongoing work. Overall, our pilot results help set the stage to deploy mobile devices for better patient monitoring, engagement, and quality of care in the inpatient setting.[17]
In conclusion, our pilot project demonstrates that tablet computers can be used to improve inpatient education and patient engagement in discharge planning. Inpatients are highly satisfied with the use of tablets to complete health education modules and access their PHR, with minimal time required for patient training and device management by hospital staff. Tablets and other mobile devices have significant potential to improve patients' education and engagement in their hospital care.
Acknowledgements
The authors thank the UCSF mHealth group and Center for Digital Health Innovation for advice and for providing tablet computers for this pilot project.
Disclosures: This article was presented as a finalist in the Research, Innovations, and Clinical Vignettes competition (Innovations category) at the 2013 Annual Meeting of the Society for Hospital Medicine. Dr. Auerbach was supported by grant K24HL098372 (NHLBI). Dr. Greysen was supported by a career development award (KL‐2) from the UCSF Clinical Translational Sciences Institute. The authors have declared they have no financial, personal, or other conflicts of interest relevant to this study.
BACKGROUND
Many hospitals have initiated intense efforts to improve transitions of care[1] such as discharge coordinators or transition coaches,[2, 3] but use of mobile devices as approaches to add or extend the value of human interventions have been understudied.[4] Additionally, many hospitalized patients experience substantial inactive time between provider visits, tests, and treatments. This time could be used to engage patients in their care through interactive health education modules and by learning to use their PHR to manage medications and postdischarge appointments.
Greater understanding of the advantages and limitations of mobile devices may be important for improving transitions of care and may help leverage existing hospital personnel resources. However, prior studies have focused on healthcare provider uses of tablet computers for medical education,[5] to collect clinical registration data,[6] or to do clinical work (eg, check labs, write notes)[7, 8, 9] primarily in outpatient settings; few studies have focused on patient uses for this technology in hospital settings.[10, 11] To address these knowledge gaps, we conducted a pilot project to explore inpatient satisfaction with bedside tablets and barriers to usability. Additionally, we evaluated use of these devices to deliver 2 specific Web‐based programs: (1) an interactive video to improve inpatient education about hospital safety, and (2) PHR access to promote inpatient engagement in discharge planning.
METHODS
Study Design, Patient Selection, and Devices/Programs
We conducted a prospective study of tablet computers to engage patients in their care and discharge planning through Web‐based interactive health education modules and use of PHRs. We used 2 tablets, distributed daily by research assistants (RAs) to eligible patients after morning rounds. Inclusion criteria for patients were ability to speak English and admission to the medical (hospitalist) service at University of California San Francisco (UCSF) Medical Center. Exclusion criteria were intensive care unit admission, contact isolation, or inability to complete the consent process due to altered mental status or cognitive impairment.
RAs screened patients for inclusion/exclusion via the electronic medical record and then approached them after rounds for enrollment (11:00 am1:00 pm). RAs then performed a tiered orientation tailored to individual patient experience and needs. First, they delivered a brief tutorial focused on the tablet itself and its basic functions (touchscreen, keypad, and Internet browser use). Second, RAs showed patients how to access the online educational health module and how to navigate content within the module. RAs next explained what the PHR is and demonstrated how to login, how to navigate tabs within the PHR, and how to perform basic tasks (view/refill medications, view/modify appointments, and view/send messages to providers). The RAs left devices with patients for 3 to 5 hours and returned to collect them and perform debriefing interviews. After each device was returned, RAs cleaned devices with disinfectant wipes available in patient rooms and checked for physical damage or software malfunctions (eg, unable to turn on or unlock). Finally, RAs used the reset function to erase any personal data or setting modifications made by patients and docked the devices overnight to resynchronize the original settings and recharge the batteries.
We used the 16 gigabyte Apple iPad2 (Apple Inc., Cupertino, CA) without any enclosures, cases, or security devices. Our educational health module was Patient Safety in the Hospital, which was professionally developed by Emmi Solutions (
Survey Instruments and Data Collection
We developed pre‐ and postintervention surveys to characterize patients' demographics, device ownership, and health‐related Internet activities in the last year based on questions used in the Centers for Disease Control and Prevention National Health Interview Study (
Analyses
We used frequency analysis to describe patient demographics, ability to complete online health educational modules, and utilization of their PHR. We performed bivariate analyses (Fisher exact test) to assess correlations between demographics (age, device ownership, Internet use) and key pilot program performance measures (orientation time 15 minutes, online health module completion, and completion of 1 essential function in the PHR). All analyses were performed in SAS 9.2 (SAS Institute Inc., Cary, NC). The institutional review board of record for UCSF approved this study.
RESULTS
As shown in Table 1, we enrolled 30 patients. Most participants (60%) were aged 40 years or older, and most (87%) owned a mobile device; 70% owned a laptop and 60% owned a smartphone, but few (22%) owned a computer tablet. Most participants accessed the Internet daily, but fewer reported Internet use for health tasks; about half (52%) communicated with a provider, but few refilled a prescription (27%) or scheduled an appointment (21%) online over the last year.
Characteristic | No. (%) |
---|---|
Age, y | |
1839 | 11 (38%) |
4049 | 5 (18%) |
5059 | 4 (14%) |
6069 | 5 (18%) |
7079 | 3 (10%) |
Gender, female | 17 (60%) |
Device ownership | |
Desktop computer | 12 (44%) |
Laptop computer | 19 (70%) |
Smart phone | 17 (60%) |
Tablet computer | 6 (22%) |
Any mobile device (laptop, smartphone, or tablet) | 26 (87%) |
Internet use | |
Daily | 21 (72%) |
Several times a week | 3 (10%) |
Once a week or less | 5 (18%) |
Prestudy online health tasks | |
Looked up health information | 21 (72%) |
Communicated with provider | 15 (52%) |
Refilled prescription | 8 (27%) |
Scheduled medical appointment | 6 (21%) |
Nearly all participants (90%) were satisfied or very satisfied with their experience using the tablet in the hospital (Figure 1). Most (87%) required 30 minutes or less for basic orientation, and 70% required only 15 minutes or less. Most participants (83%) were able to independently complete an interactive health education module on hospital safety and were highly satisfied with the module. Despite the fact that 73% of participants were first‐time users of our PHR, the majority were able to login and independently access their medication list, verify scheduled appointments, or send a secure message to their primary care provider.
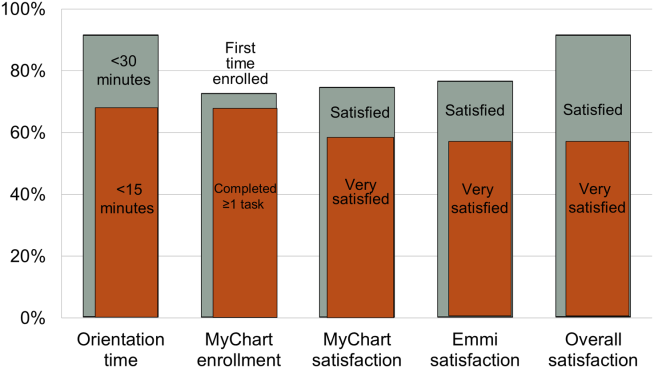
Participants aged 50 years or older were less likely to complete orientation in 15 minutes or less compared to those under 50 years old (25% vs 79%, P=0.025); however, age was not a significant factor in ability to complete the online health educational module or perform at least 1 essential PHR function. Other demographic features, such as device ownership and daily Internet use, did not correlate with shorter orientation time, educational module completion, or PHR use (data available on request).
Participants also made suggestions for improvement during the debrief interviews. Several suggested applications for entertainment (gaming, magazines, or music) and 2 suggested that all patients should be introduced to their PHR during hospitalization (data available on request). No device software malfunction (eg, device freezes, Internet connection failures), hardware issues (eg, damage from falls, wetness, or repeated disinfectant exposure), or theft or misappropriation were reported by patients or observed by the RAs to date.
DISCUSSION
Our pilot study suggests that tablet‐based access to educational modules and PHRs can increase inpatient engagement in care with high satisfaction and minimal time for orientation. Surprisingly, even older patients and those who might be considered less tech savvy in terms of Internet use and device ownership were equally able to utilize our tablet interventions. Furthermore, we did not experience any hardware issues in the harsh physical environment of inpatient wards. These preliminary findings suggest the potential utility of tablets for clinically meaningful tasks by inpatients with a low rate of technical issues.
From a technical standpoint, our experience suggests several next steps. First, although orientation time was minimal, it might be even less if patients used their own mobile devices because most patients already owned one. This bring your own device (BYOD) approach could also promote postdischarge patient engagement. Second, the flexibility of a BYOD approach raises the question of whether to also allow patients a choice of application‐based versus browser‐based platforms for specific programs such as the PHR and educational video we used. Indeed, although we used a browser‐based approach, several other teams have developed patient‐facing engagement applications (or apps) for mobile devices,[4, 12] and hospitalists could prescribe apps at discharge as a more providers are now doing in outpatient settings.[13] Of course, maximizing flexibility for BYOD and Web‐based versus app‐based approaches would likely increase patient engagement but would come at the cost of more time and effort for hospital providers to vet apps/websites and educate patients about their use. Third, regardless of the devices and programs used, broader engagement of patients, nurses, hospitalists, and other providers will be needed in the future to identify key areas for development to avoid overburdening patients and providers.
From a quality‐improvement perspective, recent literature has considered broad clinical uses for tablets by hospital providers,[14, 15] but our experience suggests more specific opportunities to improve transitions of care though direct patient engagement. Tablets and other mobile devices may help improve discharge education for patients taking high‐risk medications such as warfarin or insulin using interactive educational modules similar to the hospital safety modules we used. Additionally, clinical staff, such as nurses and pharmacists, can be trained to deliver mobile device interventions such as education on high‐risk medications.[16] Ultimately, scale up for our intervention will require that mobile devices and content eventually improve and replace current practices by hospital staff (especially nurses) in a way that streamlines, rather than compounds, current workflow. This could increase efficiency in these discharge tasks and extend contributions of these providers to high‐quality transitions.
Our study has several limitations. First, although this is a pilot study with only 30 patients, it adds needed scale to much smaller (N=58) published feasibility studies of tablet computer use by inpatients.[11, 12] Beyond more robust feasibility testing, our study adds new data about mobile device use for specific clinical tasks in the hospital such as patient education and PHR use. Second, we did not track postdischarge outcomes to test the effects of our intervention on transition care quality; this will be a focus of our future research. Third, we used existing platforms for interactive educational modules and PHR access at our site; participant satisfaction in our study may not generalize to other platforms. Furthermore, most PHR platforms (including ours) are not optimally configured to engage patients during transitions of care, but we plan to integrate existing functions (such as ability to refill medications or change appointments) into discharge education and planning. Finally, we have not engaged caregivers as surrogates for cognitively impaired patients or adapted our platform for non‐English speakers; these are areas for development in our ongoing work. Overall, our pilot results help set the stage to deploy mobile devices for better patient monitoring, engagement, and quality of care in the inpatient setting.[17]
In conclusion, our pilot project demonstrates that tablet computers can be used to improve inpatient education and patient engagement in discharge planning. Inpatients are highly satisfied with the use of tablets to complete health education modules and access their PHR, with minimal time required for patient training and device management by hospital staff. Tablets and other mobile devices have significant potential to improve patients' education and engagement in their hospital care.
Acknowledgements
The authors thank the UCSF mHealth group and Center for Digital Health Innovation for advice and for providing tablet computers for this pilot project.
Disclosures: This article was presented as a finalist in the Research, Innovations, and Clinical Vignettes competition (Innovations category) at the 2013 Annual Meeting of the Society for Hospital Medicine. Dr. Auerbach was supported by grant K24HL098372 (NHLBI). Dr. Greysen was supported by a career development award (KL‐2) from the UCSF Clinical Translational Sciences Institute. The authors have declared they have no financial, personal, or other conflicts of interest relevant to this study.
- Hospital readmissions and the Affordable Care Act: paying for coordinated quality care. JAMA. 2011;306(16):1794–1795. , .
- A reengineered hospital discharge program to decrease re‐hospitalization. Ann Intern Med. 2009;150:178–187. , , , et al.
- The care transitions intervention: results of a randomized controlled trial. Arch Intern Med. 2006;166:1822–1828. , , , .
- Project RED. Meet Louise…and virtual patient advocates. Available at: http://www.bu.edu/fammed/projectred/publications/VirtualPatientAdvocateWebsiteInfo2.pdf. Accessed July 12, 2013.
- Use of handheld computers in medical education. A systematic review. J Gen Intern Med. 2006;21(5):531–537. , , , .
- Ongoing evaluation of ease‐of‐use and usefulness of wireless tablet computers within an ambulatory care unit. Stud Health Tech Inform. 2009;143:459–464. , , , .
- Use of a tablet personal computer to enhance patient care on multidisciplinary rounds. Am J Health Syst Pharm. 2009;66(21):1909–1911. .
- Experiences incorporating Tablet PCs into clinical pharmacists' workflow. J Healthc Inf Manag. 2005;19(4):32–37. , .
- The impact of mobile handheld technology on hospital physicians' work practices and patient care: a systematic review. J Am Med Inform Assoc. 2009;16(6):792–801. , , .
- An investigation of the efficacy of electronic consenting interfaces of research permissions management system in a hospital setting. Int J Med Inform. 2013;82(9):854–863. , , , et al.
- A tablet computer application for patients to participate in their hospital care. AMIA Annu Symp Proc. 2011;2011:1428–1435. , , , et al.
- Building and testing a patient‐centric electronic bedside communication center. J Gerontol Nurs. 2013;39(1):15–19. , , , et al.
- How apps are changing family medicine. J Fam Pract. 2013Jul;62(7):362–367. .
- The iPad: gadget or medical godsend? Ann Emerg Med. 2010;56(1):A21–A22. .
- How might the iPad change healthcare? J R Soc Med. 2012;105(6):233–241. , , , et al.
- Keeping the patient focus: using tablet technology to enhance education and practice. J Contin Educ Nurs. 2012;43(6):249–250. .
- Advancing the science of mHealth. J Health Commun. 2012;17(suppl 1):5–10. , , , et al.
- Hospital readmissions and the Affordable Care Act: paying for coordinated quality care. JAMA. 2011;306(16):1794–1795. , .
- A reengineered hospital discharge program to decrease re‐hospitalization. Ann Intern Med. 2009;150:178–187. , , , et al.
- The care transitions intervention: results of a randomized controlled trial. Arch Intern Med. 2006;166:1822–1828. , , , .
- Project RED. Meet Louise…and virtual patient advocates. Available at: http://www.bu.edu/fammed/projectred/publications/VirtualPatientAdvocateWebsiteInfo2.pdf. Accessed July 12, 2013.
- Use of handheld computers in medical education. A systematic review. J Gen Intern Med. 2006;21(5):531–537. , , , .
- Ongoing evaluation of ease‐of‐use and usefulness of wireless tablet computers within an ambulatory care unit. Stud Health Tech Inform. 2009;143:459–464. , , , .
- Use of a tablet personal computer to enhance patient care on multidisciplinary rounds. Am J Health Syst Pharm. 2009;66(21):1909–1911. .
- Experiences incorporating Tablet PCs into clinical pharmacists' workflow. J Healthc Inf Manag. 2005;19(4):32–37. , .
- The impact of mobile handheld technology on hospital physicians' work practices and patient care: a systematic review. J Am Med Inform Assoc. 2009;16(6):792–801. , , .
- An investigation of the efficacy of electronic consenting interfaces of research permissions management system in a hospital setting. Int J Med Inform. 2013;82(9):854–863. , , , et al.
- A tablet computer application for patients to participate in their hospital care. AMIA Annu Symp Proc. 2011;2011:1428–1435. , , , et al.
- Building and testing a patient‐centric electronic bedside communication center. J Gerontol Nurs. 2013;39(1):15–19. , , , et al.
- How apps are changing family medicine. J Fam Pract. 2013Jul;62(7):362–367. .
- The iPad: gadget or medical godsend? Ann Emerg Med. 2010;56(1):A21–A22. .
- How might the iPad change healthcare? J R Soc Med. 2012;105(6):233–241. , , , et al.
- Keeping the patient focus: using tablet technology to enhance education and practice. J Contin Educ Nurs. 2012;43(6):249–250. .
- Advancing the science of mHealth. J Health Commun. 2012;17(suppl 1):5–10. , , , et al.