User login
Concerns Regarding Long‐term Opioid Use
Overall rates of opioid use and chronic use for noncancer pain have increased markedly in the last 1 to 2 decades.[1, 2] Recognition of such rapidly increasing use has prompted a flurry of investigations examining the impact of what has been referred to as the opioid epidemic.[1, 3, 4] Patients receiving chronic opioid analgesics have previously been demonstrated to consume disproportionate shares of healthcare resources, including significantly more emergency room visits and days in the hospital.[5] In this issue of the Journal of Hospital Medicine, Liang and Turner[6] further demonstrate the impressive scope of healthcare resources consumed by this patient population, and extend these findings by examining the relationship between opioid dose and subsequent hospitalization in a large national cohort of middle‐aged health maintenance organization enrollees with noncancer pain. This is the first study to investigate the relationship in a general cohort of patients.
Perhaps the most striking finding of the study was an all‐cause hospitalization rate of 1120 per 10,000 person‐years among their cohort of opioid users. Considering that 5 to 8 million Americans use long‐term opioids,[7] this translates to about 500,000 to 900,000 admissions per year. The degree to which opioids themselves contribute to such hospitalizations (attributable risk) is uncertain, and it is likely that some of this risk can be explained by the idea that opioids are a marker for comorbidity, and that the conditions prompting opioid use independently increase risk of hospitalization. Studies examining more homogeneous patient populations could serve to shed light on this question. The issue of attributable risk notwithstanding, it is clear that this is a patient population that should have the attention of physicians, hospital administrators, and policy makers.
The main finding of their study is that the total opioid dose in any given 6‐month interval was more strongly associated with subsequent all‐cause hospitalization than the daily dose. This suggests that cumulative exposure is important, and possibly more important than the strength of any given prescription, at least when it comes to the outcome of hospitalization. That is not to say that the daily dose is unimportant, and the authors appropriately caution against such an interpretation. Daily dose matters, and to conclude otherwise would be incorrect for several reasons. First, among patients receiving high total doses of opioids, higher daily doses did seem to confer additional risk. Second, hospitalization is only 1 measure of risk, and multiple prior studies and a recent systematic review have concluded that higher opioid doses are strongly associated with adverse events, including overdose, abuse, addiction, motor vehicle accidents, and myocardial infarction.[8] Last, their finding that total dose more strongly predicts hospitalization than daily dose may reflect confounding by indication and underlying patient characteristics not captured in their analysis. Patients receiving a daily dose of 100 mg or more, but with a total dose of <1830 mg over 6 months, would necessarily have received opioids for a relatively brief period of time (<20 days). The indications forand patients receivingsuch short‐course, high‐dose therapy are likely to be vastly different from those for longer‐course, high‐dose therapy, in ways that could be meaningfully associated with hospitalization risk. Nonetheless, their results suggest that cumulative exposure is important as an additional metric by which to predict possible adverse consequences of opioid use.
That cumulative exposure and percent of time on opioids are associated with increased risk of subsequent hospitalization casts further doubt on the already questionable risk‐to‐benefit ratio of long‐term use of opioids for noncancer pain. A recent systematic review of the effectiveness and risks of long‐term opioid therapy for chronic pain found existing evidence insufficient to determine effectiveness for chronic pain and function, owing to lack of a single study evaluating long‐term outcomes in patients on opioid therapy versus no opioid therapy, and found evidence for a dose‐dependent risk for serious harms.[8] The authors conclude that the lack of scientific evidence on effectiveness of long‐term opioid therapy for chronic pain is in striking contrast to its widespread use in this setting. Studies examining the effect of long‐term opioid therapy on pain and function, and defining patient subgroups that may benefit from such therapy, are imperative and long overdue.
In the absence of data showing benefit, and in the face of a growing body of evidence demonstrating harm, we are obligated to reevaluate opioid prescribing for chronic noncancer pain. Until studies have evaluated the impact of opioid use on long‐term outcomes, physicians are missing a key piece of the risk‐benefit calculation, and prescribing must be done judiciously. Curbing the opioid epidemic will require initiatives of epidemic proportions, involving the entire spectrum of healthcare, from the primary care setting to the emergency department (where up to 25% of patients with chronic pain receive their opioids[7]), from researchers to policy makers, and ultimately from patient expectations to physician decision making.
Disclosures
Dr. Herzig was funded by grant number K23AG042459 from the National Institute on Aging. The funding organization had no involvement in any aspect of the study, including design, conduct, and reporting of the study. The author reports no conflicts of interest.
- Vital signs: overdoses of prescription opioid pain relievers—United States, 1999—2008. MMWR Morb Mortal Wkly Rep. 2011;60:1487–1492.
- Trends in long‐term opioid therapy for chronic non‐cancer pain. Pharmacoepidemiol Drug Saf. 2009;18:1166–1175. , , , et al.
- CDC grand rounds: prescription drug overdoses—a U.S. epidemic. MMWR Morb Mortal Wkly Rep. 2012;61:10–13.
- A flood of opioids, a rising tide of deaths. N Engl J Med. 2010;363:1981–1985. .
- Co‐morbidity and utilization of medical services by pain patients receiving opioid medications: data from an insurance claims database. Pain. 2009;144:20–27. , , , , , .
- National cohort study of opioid analgesic dose and risk of future hospitalization. J Hosp Med. 2015;10:000–000. , .
- National institutes of health pathways to prevention workshop: the role of opioids in the treatment of chronic pain. Ann Intern Med. 2015;162:295–300. , , , et al.
- The effectiveness and risks of long‐term opioid therapy for chronic pain: a systematic review for a national institutes of health pathways to prevention workshop. Ann Intern Med. 2015;162:276–286. , , , et al.
Overall rates of opioid use and chronic use for noncancer pain have increased markedly in the last 1 to 2 decades.[1, 2] Recognition of such rapidly increasing use has prompted a flurry of investigations examining the impact of what has been referred to as the opioid epidemic.[1, 3, 4] Patients receiving chronic opioid analgesics have previously been demonstrated to consume disproportionate shares of healthcare resources, including significantly more emergency room visits and days in the hospital.[5] In this issue of the Journal of Hospital Medicine, Liang and Turner[6] further demonstrate the impressive scope of healthcare resources consumed by this patient population, and extend these findings by examining the relationship between opioid dose and subsequent hospitalization in a large national cohort of middle‐aged health maintenance organization enrollees with noncancer pain. This is the first study to investigate the relationship in a general cohort of patients.
Perhaps the most striking finding of the study was an all‐cause hospitalization rate of 1120 per 10,000 person‐years among their cohort of opioid users. Considering that 5 to 8 million Americans use long‐term opioids,[7] this translates to about 500,000 to 900,000 admissions per year. The degree to which opioids themselves contribute to such hospitalizations (attributable risk) is uncertain, and it is likely that some of this risk can be explained by the idea that opioids are a marker for comorbidity, and that the conditions prompting opioid use independently increase risk of hospitalization. Studies examining more homogeneous patient populations could serve to shed light on this question. The issue of attributable risk notwithstanding, it is clear that this is a patient population that should have the attention of physicians, hospital administrators, and policy makers.
The main finding of their study is that the total opioid dose in any given 6‐month interval was more strongly associated with subsequent all‐cause hospitalization than the daily dose. This suggests that cumulative exposure is important, and possibly more important than the strength of any given prescription, at least when it comes to the outcome of hospitalization. That is not to say that the daily dose is unimportant, and the authors appropriately caution against such an interpretation. Daily dose matters, and to conclude otherwise would be incorrect for several reasons. First, among patients receiving high total doses of opioids, higher daily doses did seem to confer additional risk. Second, hospitalization is only 1 measure of risk, and multiple prior studies and a recent systematic review have concluded that higher opioid doses are strongly associated with adverse events, including overdose, abuse, addiction, motor vehicle accidents, and myocardial infarction.[8] Last, their finding that total dose more strongly predicts hospitalization than daily dose may reflect confounding by indication and underlying patient characteristics not captured in their analysis. Patients receiving a daily dose of 100 mg or more, but with a total dose of <1830 mg over 6 months, would necessarily have received opioids for a relatively brief period of time (<20 days). The indications forand patients receivingsuch short‐course, high‐dose therapy are likely to be vastly different from those for longer‐course, high‐dose therapy, in ways that could be meaningfully associated with hospitalization risk. Nonetheless, their results suggest that cumulative exposure is important as an additional metric by which to predict possible adverse consequences of opioid use.
That cumulative exposure and percent of time on opioids are associated with increased risk of subsequent hospitalization casts further doubt on the already questionable risk‐to‐benefit ratio of long‐term use of opioids for noncancer pain. A recent systematic review of the effectiveness and risks of long‐term opioid therapy for chronic pain found existing evidence insufficient to determine effectiveness for chronic pain and function, owing to lack of a single study evaluating long‐term outcomes in patients on opioid therapy versus no opioid therapy, and found evidence for a dose‐dependent risk for serious harms.[8] The authors conclude that the lack of scientific evidence on effectiveness of long‐term opioid therapy for chronic pain is in striking contrast to its widespread use in this setting. Studies examining the effect of long‐term opioid therapy on pain and function, and defining patient subgroups that may benefit from such therapy, are imperative and long overdue.
In the absence of data showing benefit, and in the face of a growing body of evidence demonstrating harm, we are obligated to reevaluate opioid prescribing for chronic noncancer pain. Until studies have evaluated the impact of opioid use on long‐term outcomes, physicians are missing a key piece of the risk‐benefit calculation, and prescribing must be done judiciously. Curbing the opioid epidemic will require initiatives of epidemic proportions, involving the entire spectrum of healthcare, from the primary care setting to the emergency department (where up to 25% of patients with chronic pain receive their opioids[7]), from researchers to policy makers, and ultimately from patient expectations to physician decision making.
Disclosures
Dr. Herzig was funded by grant number K23AG042459 from the National Institute on Aging. The funding organization had no involvement in any aspect of the study, including design, conduct, and reporting of the study. The author reports no conflicts of interest.
Overall rates of opioid use and chronic use for noncancer pain have increased markedly in the last 1 to 2 decades.[1, 2] Recognition of such rapidly increasing use has prompted a flurry of investigations examining the impact of what has been referred to as the opioid epidemic.[1, 3, 4] Patients receiving chronic opioid analgesics have previously been demonstrated to consume disproportionate shares of healthcare resources, including significantly more emergency room visits and days in the hospital.[5] In this issue of the Journal of Hospital Medicine, Liang and Turner[6] further demonstrate the impressive scope of healthcare resources consumed by this patient population, and extend these findings by examining the relationship between opioid dose and subsequent hospitalization in a large national cohort of middle‐aged health maintenance organization enrollees with noncancer pain. This is the first study to investigate the relationship in a general cohort of patients.
Perhaps the most striking finding of the study was an all‐cause hospitalization rate of 1120 per 10,000 person‐years among their cohort of opioid users. Considering that 5 to 8 million Americans use long‐term opioids,[7] this translates to about 500,000 to 900,000 admissions per year. The degree to which opioids themselves contribute to such hospitalizations (attributable risk) is uncertain, and it is likely that some of this risk can be explained by the idea that opioids are a marker for comorbidity, and that the conditions prompting opioid use independently increase risk of hospitalization. Studies examining more homogeneous patient populations could serve to shed light on this question. The issue of attributable risk notwithstanding, it is clear that this is a patient population that should have the attention of physicians, hospital administrators, and policy makers.
The main finding of their study is that the total opioid dose in any given 6‐month interval was more strongly associated with subsequent all‐cause hospitalization than the daily dose. This suggests that cumulative exposure is important, and possibly more important than the strength of any given prescription, at least when it comes to the outcome of hospitalization. That is not to say that the daily dose is unimportant, and the authors appropriately caution against such an interpretation. Daily dose matters, and to conclude otherwise would be incorrect for several reasons. First, among patients receiving high total doses of opioids, higher daily doses did seem to confer additional risk. Second, hospitalization is only 1 measure of risk, and multiple prior studies and a recent systematic review have concluded that higher opioid doses are strongly associated with adverse events, including overdose, abuse, addiction, motor vehicle accidents, and myocardial infarction.[8] Last, their finding that total dose more strongly predicts hospitalization than daily dose may reflect confounding by indication and underlying patient characteristics not captured in their analysis. Patients receiving a daily dose of 100 mg or more, but with a total dose of <1830 mg over 6 months, would necessarily have received opioids for a relatively brief period of time (<20 days). The indications forand patients receivingsuch short‐course, high‐dose therapy are likely to be vastly different from those for longer‐course, high‐dose therapy, in ways that could be meaningfully associated with hospitalization risk. Nonetheless, their results suggest that cumulative exposure is important as an additional metric by which to predict possible adverse consequences of opioid use.
That cumulative exposure and percent of time on opioids are associated with increased risk of subsequent hospitalization casts further doubt on the already questionable risk‐to‐benefit ratio of long‐term use of opioids for noncancer pain. A recent systematic review of the effectiveness and risks of long‐term opioid therapy for chronic pain found existing evidence insufficient to determine effectiveness for chronic pain and function, owing to lack of a single study evaluating long‐term outcomes in patients on opioid therapy versus no opioid therapy, and found evidence for a dose‐dependent risk for serious harms.[8] The authors conclude that the lack of scientific evidence on effectiveness of long‐term opioid therapy for chronic pain is in striking contrast to its widespread use in this setting. Studies examining the effect of long‐term opioid therapy on pain and function, and defining patient subgroups that may benefit from such therapy, are imperative and long overdue.
In the absence of data showing benefit, and in the face of a growing body of evidence demonstrating harm, we are obligated to reevaluate opioid prescribing for chronic noncancer pain. Until studies have evaluated the impact of opioid use on long‐term outcomes, physicians are missing a key piece of the risk‐benefit calculation, and prescribing must be done judiciously. Curbing the opioid epidemic will require initiatives of epidemic proportions, involving the entire spectrum of healthcare, from the primary care setting to the emergency department (where up to 25% of patients with chronic pain receive their opioids[7]), from researchers to policy makers, and ultimately from patient expectations to physician decision making.
Disclosures
Dr. Herzig was funded by grant number K23AG042459 from the National Institute on Aging. The funding organization had no involvement in any aspect of the study, including design, conduct, and reporting of the study. The author reports no conflicts of interest.
- Vital signs: overdoses of prescription opioid pain relievers—United States, 1999—2008. MMWR Morb Mortal Wkly Rep. 2011;60:1487–1492.
- Trends in long‐term opioid therapy for chronic non‐cancer pain. Pharmacoepidemiol Drug Saf. 2009;18:1166–1175. , , , et al.
- CDC grand rounds: prescription drug overdoses—a U.S. epidemic. MMWR Morb Mortal Wkly Rep. 2012;61:10–13.
- A flood of opioids, a rising tide of deaths. N Engl J Med. 2010;363:1981–1985. .
- Co‐morbidity and utilization of medical services by pain patients receiving opioid medications: data from an insurance claims database. Pain. 2009;144:20–27. , , , , , .
- National cohort study of opioid analgesic dose and risk of future hospitalization. J Hosp Med. 2015;10:000–000. , .
- National institutes of health pathways to prevention workshop: the role of opioids in the treatment of chronic pain. Ann Intern Med. 2015;162:295–300. , , , et al.
- The effectiveness and risks of long‐term opioid therapy for chronic pain: a systematic review for a national institutes of health pathways to prevention workshop. Ann Intern Med. 2015;162:276–286. , , , et al.
- Vital signs: overdoses of prescription opioid pain relievers—United States, 1999—2008. MMWR Morb Mortal Wkly Rep. 2011;60:1487–1492.
- Trends in long‐term opioid therapy for chronic non‐cancer pain. Pharmacoepidemiol Drug Saf. 2009;18:1166–1175. , , , et al.
- CDC grand rounds: prescription drug overdoses—a U.S. epidemic. MMWR Morb Mortal Wkly Rep. 2012;61:10–13.
- A flood of opioids, a rising tide of deaths. N Engl J Med. 2010;363:1981–1985. .
- Co‐morbidity and utilization of medical services by pain patients receiving opioid medications: data from an insurance claims database. Pain. 2009;144:20–27. , , , , , .
- National cohort study of opioid analgesic dose and risk of future hospitalization. J Hosp Med. 2015;10:000–000. , .
- National institutes of health pathways to prevention workshop: the role of opioids in the treatment of chronic pain. Ann Intern Med. 2015;162:295–300. , , , et al.
- The effectiveness and risks of long‐term opioid therapy for chronic pain: a systematic review for a national institutes of health pathways to prevention workshop. Ann Intern Med. 2015;162:276–286. , , , et al.
Reducing Inappropriate Acid Suppressives
Prior studies have found that up to 70% of acid‐suppressive medication (ASM) use in the hospital is not indicated, most commonly for stress ulcer prophylaxis in patients outside of the intensive care unit (ICU).[1, 2, 3, 4, 5, 6, 7] Accordingly, reducing inappropriate use of ASM for stress ulcer prophylaxis in hospitalized patients is 1 of the 5 opportunities for improved healthcare value identified by the Society of Hospital Medicine as part of the American Board of Internal Medicine's Choosing Wisely campaign.[8]
We designed and tested a computerized clinical decision support (CDS) intervention with the goal of reducing use of ASM for stress ulcer prophylaxis in hospitalized patients outside the ICU at an academic medical center.
METHODS
Study Design
We conducted a quasiexperimental study using an interrupted time series to analyze data collected prospectively during clinical care before and after implementation of our intervention. The study was deemed a quality improvement initiative by the Beth Israel Deaconess Medical Center Committee on Clinical Investigations/Institutional Review Board.
Patients and Setting
All admissions >18 years of age to a 649‐bed academic medical center in Boston, Massachusetts from September 12, 2011 through July 3, 2012 were included. The medical center consists of an East and West Campus, located across the street from each other. Care for both critically ill and noncritically ill medical and surgical patients occurs on both campuses. Differences include greater proportions of patients with gastrointestinal and oncologic conditions on the East Campus, and renal and cardiac conditions on the West Campus. Additionally, labor and delivery occurs exclusively on the East Campus, and the density of ICU beds is greater on the West Campus. Both campuses utilize a computer‐based provider order entry (POE) system.
Intervention
Our study was implemented in 2 phases (Figure 1).
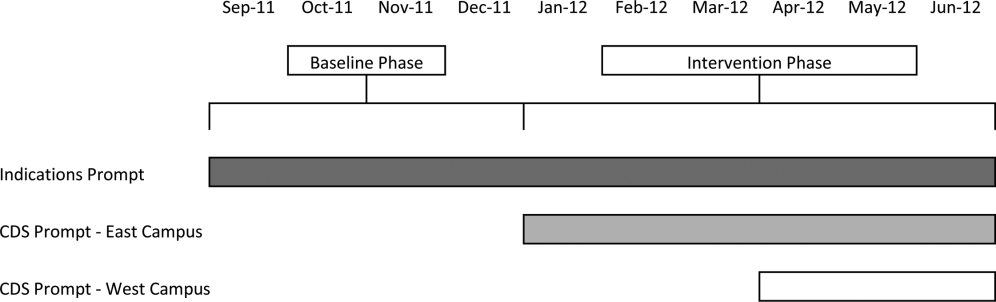
Baseline Phase
The purpose of the first phase was to obtain baseline data on ASM use prior to implementing our CDS tool designed to influence prescribing. During this baseline phase, a computerized prompt was activated through our POE system whenever a clinician initiated an order for ASM (histamine 2 receptor antagonists or proton pump inhibitors), asking the clinician to select the reason/reasons for the order based on the following predefined response options: (1) active/recent upper gastrointestinal bleed, (2) continuing preadmission medication, (3) Helicobacter pylori treatment, (4) prophylaxis in patient on medications that increase bleeding risk, (5) stress ulcer prophylaxis, (6) suspected/known peptic ulcer disease, gastritis, esophagitis, gastroesophageal reflux disease, and (7) other, with a free‐text box to input the indication. This indications prompt was rolled out to the entire medical center on September 12, 2011 and remained active for the duration of the study period.
Intervention Phase
In the second phase of the study, if a clinician selected stress ulcer prophylaxis as the only indication for ordering ASM, a CDS prompt alerted the clinician that Stress ulcer prophylaxis is not recommended for patients outside of the intensive care unit (ASHP Therapeutic Guidelines on Stress Ulcer Prophylaxis. Am J Health‐Syst Pharm. 1999, 56:347‐79). The clinician could then select either, For use in ICUOrder Medication, Choose Other Indication, or Cancel Order. This CDS prompt was rolled out in a staggered manner to the East Campus on January 3, 2012, followed by the West Campus on April 3, 2012.
Outcomes
The primary outcome was the rate of ASM use with stress ulcer prophylaxis selected as the only indication in a patient located outside of the ICU. We confirmed patient location in the 24 hours after the order was placed. Secondary outcomes were rates of overall ASM use, defined via pharmacy charges, and rates of use on discharge.
Statistical Analysis
To assure stable measurement of trends, we studied at least 3 months before and after the intervention on each campus. We used the Fisher exact test to compare the rates of our primary and secondary outcomes before and after the intervention, stratified by campus. For our primary outcomeat least 1 ASM order with stress ulcer prophylaxis selected as the only indication during hospitalizationwe developed a logistic regression model with a generalized estimating equation and exchangeable working correlation structure to control for admission characteristics (Table 1) and repeated admissions. Using a term for the interaction between time and the intervention, this model allowed us to assess changes in level and trend for the odds of a patient receiving at least 1 ASM order with stress ulcer prophylaxis as the only indication before, compared to after the intervention, stratified by campus. We used a 2‐sided type I error of <0.05 to indicate statistical significance.
Study Phase | Campus | |||
---|---|---|---|---|
East | West | |||
Baseline, n=3,747 | Intervention, n=6,191 | Baseline, n=11,177 | Intervention, n=5,285 | |
| ||||
Age, y, mean (SD) | 48.1 (18.5) | 47.7 (18.2) | 61.0 (18.0) | 60.3 (18.1) |
Gender, no. (%) | ||||
Female | 2744 (73.2%) | 4542 (73.4%) | 5551 (49.7%) | 2653 (50.2%) |
Male | 1003 (26.8%) | 1649 (26.6%) | 5626 (50.3%) | 2632 (49.8%) |
Race, no. (%) | ||||
Asian | 281 (7.5%) | 516 (8.3%) | 302 (2.7%) | 156 (3%) |
Black | 424 (11.3%) | 667 (10.8%) | 1426 (12.8%) | 685 (13%) |
Hispanic | 224 (6%) | 380 (6.1%) | 619 (5.5%) | 282 (5.3%) |
Other | 378 (10.1%) | 738 (11.9%) | 776 (6.9%) | 396 (7.5%) |
White | 2440 (65.1%) | 3890 (62.8%) | 8054 (72%) | 3766 (71.3%) |
Charlson score, mean (SD) | 0.8 (1.1) | 0.7 (1.1) | 1.5 (1.4) | 1.4 (1.4) |
Gastrointestinal bleeding, no. (%)* | 49 (1.3%) | 99 (1.6%) | 385 (3.4%) | 149 (2.8%) |
Other medication exposures, no. (%) | ||||
Therapeutic anticoagulant | 218 (5.8%) | 409 (6.6%) | 2242 (20.1%) | 1022 (19.3%) |
Prophylactic anticoagulant | 1081 (28.8%) | 1682 (27.2%) | 5999 (53.7%) | 2892 (54.7%) |
NSAID | 1899 (50.7%) | 3141 (50.7%) | 1248 (11.2%) | 575 (10.9%) |
Antiplatelet | 313 (8.4%) | 585 (9.4%) | 4543 (40.6%) | 2071 (39.2%) |
Admitting department, no. (%) | ||||
Surgery | 2507 (66.9%) | 4146 (67%) | 3255 (29.1%) | 1578 (29.9%) |
Nonsurgery | 1240 (33.1%) | 2045 (33%) | 7922 (70.9%) | 3707 (70.1%) |
Any ICU Stay, no. (%) | 217 (5.8%) | 383 (6.2%) | 2786 (24.9%) | 1252 (23.7%) |
RESULTS
There were 26,400 adult admissions during the study period, and 22,330 discrete orders for ASM. Overall, 12,056 (46%) admissions had at least 1 charge for ASM. Admission characteristics were similar before and after the intervention on each campus (Table 1).
Table 2 shows the indications chosen each time ASM was ordered, stratified by campus and study phase. Although selection of stress ulcer prophylaxis decreased on both campuses during the intervention phase, selection of continuing preadmission medication increased.
Study Phase | Campus | |||
---|---|---|---|---|
East | West | |||
Baseline, n=2,062 | Intervention, n=3,243 | Baseline, n=12,038 | Intervention, n=4,987 | |
| ||||
Indication* | ||||
Continuing preadmission medication | 910 (44.1%) | 1695 (52.3%) | 5597 (46.5%) | 2802 (56.2%) |
PUD, gastritis, esophagitis, GERD | 440 (21.3%) | 797 (24.6%) | 1303 (10.8%) | 582 (11.7%) |
Stress ulcer prophylaxis | 298 (14.4%) | 100 (3.1%) | 2659 (22.1%) | 681 (13.7%) |
Prophylaxis in patient on medications that increase bleeding risk | 226 (11.0%) | 259 (8.0%) | 965 (8.0%) | 411 (8.2%) |
Active/recent gastrointestinal bleed | 154 (7.5%) | 321 (9.9%) | 1450 (12.0%) | 515 (10.3) |
Helicobacter pylori treatment | 6 (0.2%) | 2 (0.1%) | 43 (0.4%) | 21 (0.4%) |
Other | 111 (5.4%) | 156 (4.8%) | 384 (3.2%) | 186 (3.7%) |
Table 3 shows the unadjusted comparison of outcomes between baseline and intervention phases on each campus. Use of ASM with stress ulcer prophylaxis as the only indication decreased during the intervention phase on both campuses. There was a nonsignificant reduction in overall rates of use on both campuses, and use on discharge was unchanged. Figure 2 demonstrates the unadjusted and modeled monthly rates of admissions with at least 1 ASM order with stress ulcer prophylaxis selected as the only indication, stratified by campus. After adjusting for the admission characteristics in Table 1, during the intervention phase on both campuses there was a significant immediate reduction in the odds of receiving an ASM with stress ulcer prophylaxis selected as the only indication (East Campus odds ratio [OR]: 0.36, 95% confidence interval [CI]: 0.180.71; West Campus OR: 0.41, 95% CI: 0.280.60), and a significant change in trend compared to the baseline phase (East Campus 1.5% daily decrease in odds of receiving ASM solely for stress ulcer prophylaxis, P=0.002; West Campus 0.9% daily decrease in odds of receiving ASM solely for stress ulcer prophylaxis, P=0.02).
Study Phase | Campus | |||||
---|---|---|---|---|---|---|
East | West | |||||
Baseline, n=3,747 | Intervention, n=6,191 | P Value* | Baseline, n=11,177 | Intervention, n=5,285 | P Value* | |
| ||||||
Outcome | ||||||
Any inappropriate acid‐suppressive exposure | 4.0% | 0.6% | <0.001 | 7.7% | 2.2% | <0.001 |
Any acid‐suppressive exposure | 33.1% | 31.8% | 0.16 | 54.5% | 52.9% | 0.05 |
Discharged on acid‐suppressive medication | 18.9% | 19.6% | 0.40 | 34.7% | 34.7% | 0.95 |
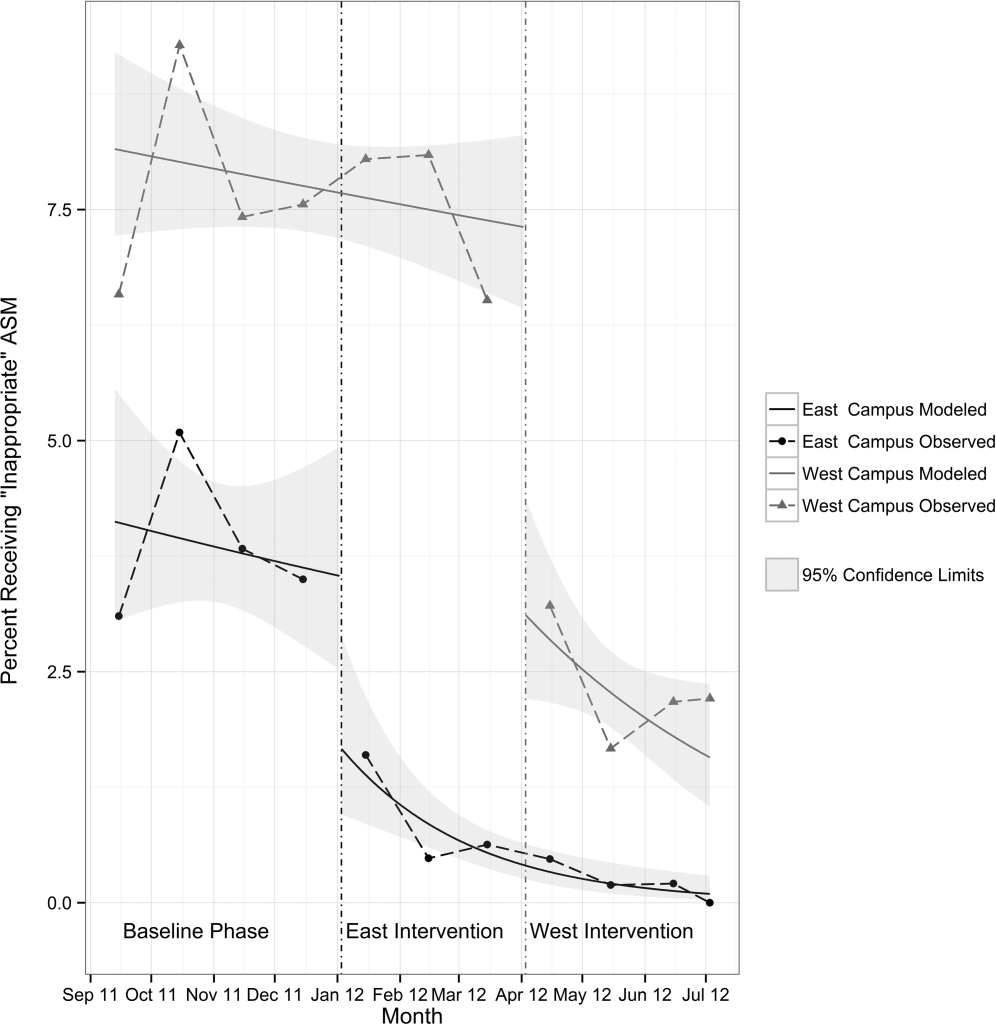
DISCUSSION
In this single‐center study, we found that a computerized CDS intervention resulted in a significant reduction in use of ASM for the sole purpose of stress ulcer prophylaxis in patients outside the ICU, a nonsignificant reduction in overall use, and no change in use on discharge. We found low rates of use for the isolated purpose of stress ulcer prophylaxis even before the intervention, and continuing preadmission medication was the most commonly selected indication throughout the study.
Although overall rates of ASM use declined after the intervention, the change was not statistically significant, and was not of the same magnitude as the decline in rates of use for the purpose of stress ulcer prophylaxis. This suggests that our intervention, in part, led to substitution of 1 indication for another. The indication that increased the most after rollout on both campuses was continuing preadmission medication. There are at least 2 possibilities for this finding: (1) the intervention prompted physicians to more accurately record the indication, or (2) physicians falsified the indication in order to execute the order. To explore these possibilities, we reviewed the charts of a random sample of 100 admissions during each of the baseline and intervention phases where continuing preadmission medication was selected as an indication for an ASM order. We found that 6/100 orders in the baseline phase and 7/100 orders in the intervention phase incorrectly indicated that the patient was on ASM prior to admission (P=0.77). This suggests that scenario 1 above is the more likely explanation for the increased use of this indication, and that the intervention, in part, simply unmasked the true rate of use at our medical center for the isolated purpose of stress ulcer prophylaxis.
These findings have implications for others attempting to use computerized CDS to better understand physician prescribing. They suggest that information collected through computer‐based interaction with clinicians at the point of care may not always be accurate or complete. As institutions increasingly use similar interventions to drive behavior, information obtained from such interaction should be validated, and when possible, patient outcomes should be measured.
Our findings suggest that rates of ASM use for the purpose of stress ulcer prophylaxis in the hospital may have declined over the last decade. Studies demonstrating that up to 70% of inpatient use of ASM was inappropriate were conducted 5 to 10 years ago.[1, 2, 3, 4, 5] Since then, studies have demonstrated risk of nosocomial infections in patients on ASM.[9, 10, 11] It is possible that the low rate of use for stress ulcer prophylaxis in our study is attributable to awareness of the risks of these medications, and limited our ability to detect differences in overall use. It is also possible, however, that a portion of the admissions with continuation of preadmission medication as the indication were started on these medications during a prior hospitalization. Thus, some portion of preadmission use is likely to represent failed medication reconciliation during a prior discharge. In this context, hospitalization may serve as an opportunity to evaluate the indication for ASM use even when these medications show up as preadmission medications.
There are additional limitations. First, the single‐center nature limits generalizability. Second, the first phase of our study, designed to obtain baseline data on ASM use, may have led to changes in prescribing prior to implementation of our CDS tool. Additionally, we did not validate the accuracy of each of the chosen indications, or the site of initial prescription in the case of preadmission exposure. Last, our study was not powered to investigate changes in rates of nosocomial gastrointestinal bleeding or nosocomial pneumonia owing to the infrequent nature of these complications.
In conclusion, we designed a simple computerized CDS intervention that was associated with a reduction in ASM use for stress ulcer prophylaxis in patients outside the ICU, a nonsignificant reduction in overall use, and no change in use on discharge. The majority of inpatient use represented continuation of preadmission medication, suggesting that interventions to improve the appropriateness of ASM prescribing should span the continuum of care. Future studies should investigate whether it is worthwhile and appropriate to reevaluate continued use of preadmission ASM during an inpatient stay.
Acknowledgements
The authors acknowledge Joshua Guthermann, MBA, and Jane Hui Chen Lim, MBA, for their assistance in the early phases of data analysis, and Long H. Ngo, PhD, for his statistical consultation.
Disclosures: Dr. Herzig was funded by a Young Clinician Research Award from the Center for Integration of Medicine and Innovative Technology, a nonprofit consortium of Boston teaching hospitals and universities, and grant number K23AG042459 from the National Institute on Aging. Dr. Marcantonio was funded by grant number K24AG035075 from the National Institute on Aging. The funding organizations had no involvement in any aspect of the study, including design, conduct, and reporting of the study. Dr. Herzig had full access to all of the data in the study and takes responsibility for the integrity of the data and the accuracy of the data analysis. Drs. Herzig and Marcantonio were responsible for the study concept and design. Drs. Herzig, Feinbloom, Howell, and Ms. Adra and Mr. Afonso were responsible for the acquisition of data. Drs. Herzig, Howell, Marcantonio, and Mr. Guess were responsible for the analysis and interpretation of the data. Dr. Herzig drafted the manuscript. All of the authors participated in the critical revision of the manuscript for important intellectual content. Drs. Herzig and Marcantonio were responsible for study supervision. The authors report no conflicts of interest.
- Stress ulcer prophylaxis in hospitalized patients not in intensive care units. Am J Health Syst Pharm. 2007;64(13):1396–1400. , .
- Magnitude and economic impact of inappropriate use of stress ulcer prophylaxis in non‐ICU hospitalized patients. Am J Gastroenterol. 2006;101(10):2200–2205. , .
- Stress‐ulcer prophylaxis for general medical patients: a review of the evidence. J Hosp Med. 2007;2(2):86–92. , .
- Hospital use of acid‐suppressive medications and its fall‐out on prescribing in general practice: a 1‐month survey. Aliment Pharmacol Ther. 2003;17(12):1503–1506. , , , et al.
- Inadequate use of acid‐suppressive therapy in hospitalized patients and its implications for general practice. Dig Dis Sci. 2005;50(12):2307–2311. , , , , , .
- Brief report: reducing inappropriate usage of stress ulcer prophylaxis among internal medicine residents. A practice‐based educational intervention. J Gen Intern Med. 2006;21(5):498–500. , .
- Inappropriate continuation of stress ulcer prophylactic therapy after discharge. Ann Pharmacother. 2007;41(10):1611–1616. , , .
- Choosing wisely in adult hospital medicine: five opportunities for improved healthcare value. J Hosp Med. 2013;8(9):486–492. , , , et al.
- Risk of Clostridium difficile diarrhea among hospital inpatients prescribed proton pump inhibitors: cohort and case‐control studies. CMAJ. 2004;171(1):33–38. , , , , .
- Iatrogenic gastric acid suppression and the risk of nosocomial Clostridium difficile infection. Arch Intern Med. 2010;170(9):784–790. , , , et al.
- Acid‐suppressive medication use and the risk for hospital‐acquired pneumonia. JAMA. 2009;301(20):2120–2128. , , , .
- Healthcare Cost and Utilization Project. Clinical classifications software (CCS) for ICD‐9‐CM. December 2009. Agency for Healthcare Research and Quality, Rockville, MD. Available at: www.hcup‐us.ahrq.gov/toolssoftware/ccs/ccs.jsp. Accessed June 18, 2014.
Prior studies have found that up to 70% of acid‐suppressive medication (ASM) use in the hospital is not indicated, most commonly for stress ulcer prophylaxis in patients outside of the intensive care unit (ICU).[1, 2, 3, 4, 5, 6, 7] Accordingly, reducing inappropriate use of ASM for stress ulcer prophylaxis in hospitalized patients is 1 of the 5 opportunities for improved healthcare value identified by the Society of Hospital Medicine as part of the American Board of Internal Medicine's Choosing Wisely campaign.[8]
We designed and tested a computerized clinical decision support (CDS) intervention with the goal of reducing use of ASM for stress ulcer prophylaxis in hospitalized patients outside the ICU at an academic medical center.
METHODS
Study Design
We conducted a quasiexperimental study using an interrupted time series to analyze data collected prospectively during clinical care before and after implementation of our intervention. The study was deemed a quality improvement initiative by the Beth Israel Deaconess Medical Center Committee on Clinical Investigations/Institutional Review Board.
Patients and Setting
All admissions >18 years of age to a 649‐bed academic medical center in Boston, Massachusetts from September 12, 2011 through July 3, 2012 were included. The medical center consists of an East and West Campus, located across the street from each other. Care for both critically ill and noncritically ill medical and surgical patients occurs on both campuses. Differences include greater proportions of patients with gastrointestinal and oncologic conditions on the East Campus, and renal and cardiac conditions on the West Campus. Additionally, labor and delivery occurs exclusively on the East Campus, and the density of ICU beds is greater on the West Campus. Both campuses utilize a computer‐based provider order entry (POE) system.
Intervention
Our study was implemented in 2 phases (Figure 1).
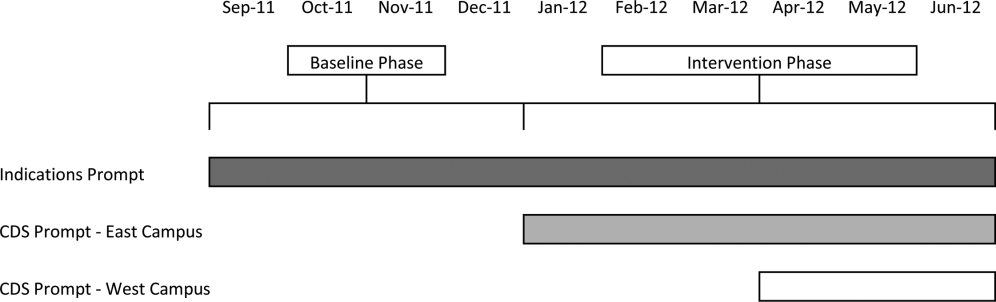
Baseline Phase
The purpose of the first phase was to obtain baseline data on ASM use prior to implementing our CDS tool designed to influence prescribing. During this baseline phase, a computerized prompt was activated through our POE system whenever a clinician initiated an order for ASM (histamine 2 receptor antagonists or proton pump inhibitors), asking the clinician to select the reason/reasons for the order based on the following predefined response options: (1) active/recent upper gastrointestinal bleed, (2) continuing preadmission medication, (3) Helicobacter pylori treatment, (4) prophylaxis in patient on medications that increase bleeding risk, (5) stress ulcer prophylaxis, (6) suspected/known peptic ulcer disease, gastritis, esophagitis, gastroesophageal reflux disease, and (7) other, with a free‐text box to input the indication. This indications prompt was rolled out to the entire medical center on September 12, 2011 and remained active for the duration of the study period.
Intervention Phase
In the second phase of the study, if a clinician selected stress ulcer prophylaxis as the only indication for ordering ASM, a CDS prompt alerted the clinician that Stress ulcer prophylaxis is not recommended for patients outside of the intensive care unit (ASHP Therapeutic Guidelines on Stress Ulcer Prophylaxis. Am J Health‐Syst Pharm. 1999, 56:347‐79). The clinician could then select either, For use in ICUOrder Medication, Choose Other Indication, or Cancel Order. This CDS prompt was rolled out in a staggered manner to the East Campus on January 3, 2012, followed by the West Campus on April 3, 2012.
Outcomes
The primary outcome was the rate of ASM use with stress ulcer prophylaxis selected as the only indication in a patient located outside of the ICU. We confirmed patient location in the 24 hours after the order was placed. Secondary outcomes were rates of overall ASM use, defined via pharmacy charges, and rates of use on discharge.
Statistical Analysis
To assure stable measurement of trends, we studied at least 3 months before and after the intervention on each campus. We used the Fisher exact test to compare the rates of our primary and secondary outcomes before and after the intervention, stratified by campus. For our primary outcomeat least 1 ASM order with stress ulcer prophylaxis selected as the only indication during hospitalizationwe developed a logistic regression model with a generalized estimating equation and exchangeable working correlation structure to control for admission characteristics (Table 1) and repeated admissions. Using a term for the interaction between time and the intervention, this model allowed us to assess changes in level and trend for the odds of a patient receiving at least 1 ASM order with stress ulcer prophylaxis as the only indication before, compared to after the intervention, stratified by campus. We used a 2‐sided type I error of <0.05 to indicate statistical significance.
Study Phase | Campus | |||
---|---|---|---|---|
East | West | |||
Baseline, n=3,747 | Intervention, n=6,191 | Baseline, n=11,177 | Intervention, n=5,285 | |
| ||||
Age, y, mean (SD) | 48.1 (18.5) | 47.7 (18.2) | 61.0 (18.0) | 60.3 (18.1) |
Gender, no. (%) | ||||
Female | 2744 (73.2%) | 4542 (73.4%) | 5551 (49.7%) | 2653 (50.2%) |
Male | 1003 (26.8%) | 1649 (26.6%) | 5626 (50.3%) | 2632 (49.8%) |
Race, no. (%) | ||||
Asian | 281 (7.5%) | 516 (8.3%) | 302 (2.7%) | 156 (3%) |
Black | 424 (11.3%) | 667 (10.8%) | 1426 (12.8%) | 685 (13%) |
Hispanic | 224 (6%) | 380 (6.1%) | 619 (5.5%) | 282 (5.3%) |
Other | 378 (10.1%) | 738 (11.9%) | 776 (6.9%) | 396 (7.5%) |
White | 2440 (65.1%) | 3890 (62.8%) | 8054 (72%) | 3766 (71.3%) |
Charlson score, mean (SD) | 0.8 (1.1) | 0.7 (1.1) | 1.5 (1.4) | 1.4 (1.4) |
Gastrointestinal bleeding, no. (%)* | 49 (1.3%) | 99 (1.6%) | 385 (3.4%) | 149 (2.8%) |
Other medication exposures, no. (%) | ||||
Therapeutic anticoagulant | 218 (5.8%) | 409 (6.6%) | 2242 (20.1%) | 1022 (19.3%) |
Prophylactic anticoagulant | 1081 (28.8%) | 1682 (27.2%) | 5999 (53.7%) | 2892 (54.7%) |
NSAID | 1899 (50.7%) | 3141 (50.7%) | 1248 (11.2%) | 575 (10.9%) |
Antiplatelet | 313 (8.4%) | 585 (9.4%) | 4543 (40.6%) | 2071 (39.2%) |
Admitting department, no. (%) | ||||
Surgery | 2507 (66.9%) | 4146 (67%) | 3255 (29.1%) | 1578 (29.9%) |
Nonsurgery | 1240 (33.1%) | 2045 (33%) | 7922 (70.9%) | 3707 (70.1%) |
Any ICU Stay, no. (%) | 217 (5.8%) | 383 (6.2%) | 2786 (24.9%) | 1252 (23.7%) |
RESULTS
There were 26,400 adult admissions during the study period, and 22,330 discrete orders for ASM. Overall, 12,056 (46%) admissions had at least 1 charge for ASM. Admission characteristics were similar before and after the intervention on each campus (Table 1).
Table 2 shows the indications chosen each time ASM was ordered, stratified by campus and study phase. Although selection of stress ulcer prophylaxis decreased on both campuses during the intervention phase, selection of continuing preadmission medication increased.
Study Phase | Campus | |||
---|---|---|---|---|
East | West | |||
Baseline, n=2,062 | Intervention, n=3,243 | Baseline, n=12,038 | Intervention, n=4,987 | |
| ||||
Indication* | ||||
Continuing preadmission medication | 910 (44.1%) | 1695 (52.3%) | 5597 (46.5%) | 2802 (56.2%) |
PUD, gastritis, esophagitis, GERD | 440 (21.3%) | 797 (24.6%) | 1303 (10.8%) | 582 (11.7%) |
Stress ulcer prophylaxis | 298 (14.4%) | 100 (3.1%) | 2659 (22.1%) | 681 (13.7%) |
Prophylaxis in patient on medications that increase bleeding risk | 226 (11.0%) | 259 (8.0%) | 965 (8.0%) | 411 (8.2%) |
Active/recent gastrointestinal bleed | 154 (7.5%) | 321 (9.9%) | 1450 (12.0%) | 515 (10.3) |
Helicobacter pylori treatment | 6 (0.2%) | 2 (0.1%) | 43 (0.4%) | 21 (0.4%) |
Other | 111 (5.4%) | 156 (4.8%) | 384 (3.2%) | 186 (3.7%) |
Table 3 shows the unadjusted comparison of outcomes between baseline and intervention phases on each campus. Use of ASM with stress ulcer prophylaxis as the only indication decreased during the intervention phase on both campuses. There was a nonsignificant reduction in overall rates of use on both campuses, and use on discharge was unchanged. Figure 2 demonstrates the unadjusted and modeled monthly rates of admissions with at least 1 ASM order with stress ulcer prophylaxis selected as the only indication, stratified by campus. After adjusting for the admission characteristics in Table 1, during the intervention phase on both campuses there was a significant immediate reduction in the odds of receiving an ASM with stress ulcer prophylaxis selected as the only indication (East Campus odds ratio [OR]: 0.36, 95% confidence interval [CI]: 0.180.71; West Campus OR: 0.41, 95% CI: 0.280.60), and a significant change in trend compared to the baseline phase (East Campus 1.5% daily decrease in odds of receiving ASM solely for stress ulcer prophylaxis, P=0.002; West Campus 0.9% daily decrease in odds of receiving ASM solely for stress ulcer prophylaxis, P=0.02).
Study Phase | Campus | |||||
---|---|---|---|---|---|---|
East | West | |||||
Baseline, n=3,747 | Intervention, n=6,191 | P Value* | Baseline, n=11,177 | Intervention, n=5,285 | P Value* | |
| ||||||
Outcome | ||||||
Any inappropriate acid‐suppressive exposure | 4.0% | 0.6% | <0.001 | 7.7% | 2.2% | <0.001 |
Any acid‐suppressive exposure | 33.1% | 31.8% | 0.16 | 54.5% | 52.9% | 0.05 |
Discharged on acid‐suppressive medication | 18.9% | 19.6% | 0.40 | 34.7% | 34.7% | 0.95 |
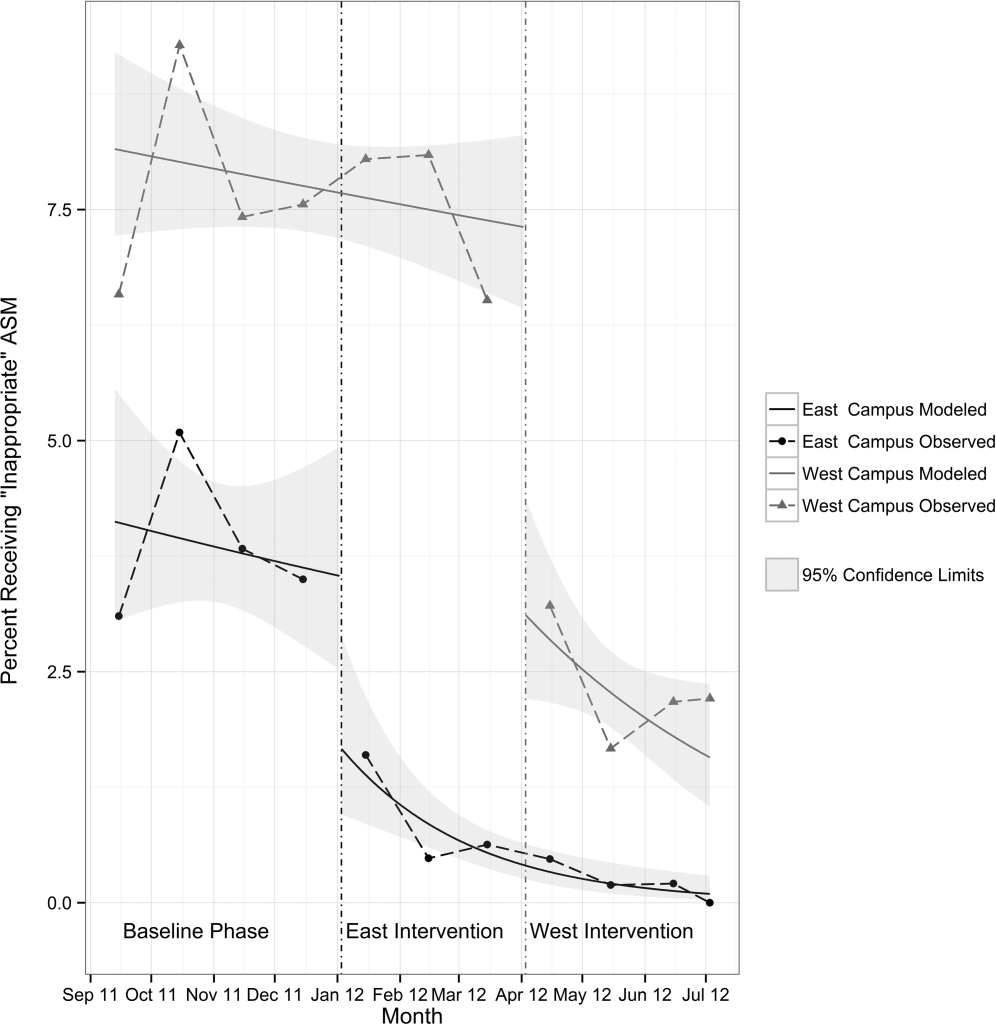
DISCUSSION
In this single‐center study, we found that a computerized CDS intervention resulted in a significant reduction in use of ASM for the sole purpose of stress ulcer prophylaxis in patients outside the ICU, a nonsignificant reduction in overall use, and no change in use on discharge. We found low rates of use for the isolated purpose of stress ulcer prophylaxis even before the intervention, and continuing preadmission medication was the most commonly selected indication throughout the study.
Although overall rates of ASM use declined after the intervention, the change was not statistically significant, and was not of the same magnitude as the decline in rates of use for the purpose of stress ulcer prophylaxis. This suggests that our intervention, in part, led to substitution of 1 indication for another. The indication that increased the most after rollout on both campuses was continuing preadmission medication. There are at least 2 possibilities for this finding: (1) the intervention prompted physicians to more accurately record the indication, or (2) physicians falsified the indication in order to execute the order. To explore these possibilities, we reviewed the charts of a random sample of 100 admissions during each of the baseline and intervention phases where continuing preadmission medication was selected as an indication for an ASM order. We found that 6/100 orders in the baseline phase and 7/100 orders in the intervention phase incorrectly indicated that the patient was on ASM prior to admission (P=0.77). This suggests that scenario 1 above is the more likely explanation for the increased use of this indication, and that the intervention, in part, simply unmasked the true rate of use at our medical center for the isolated purpose of stress ulcer prophylaxis.
These findings have implications for others attempting to use computerized CDS to better understand physician prescribing. They suggest that information collected through computer‐based interaction with clinicians at the point of care may not always be accurate or complete. As institutions increasingly use similar interventions to drive behavior, information obtained from such interaction should be validated, and when possible, patient outcomes should be measured.
Our findings suggest that rates of ASM use for the purpose of stress ulcer prophylaxis in the hospital may have declined over the last decade. Studies demonstrating that up to 70% of inpatient use of ASM was inappropriate were conducted 5 to 10 years ago.[1, 2, 3, 4, 5] Since then, studies have demonstrated risk of nosocomial infections in patients on ASM.[9, 10, 11] It is possible that the low rate of use for stress ulcer prophylaxis in our study is attributable to awareness of the risks of these medications, and limited our ability to detect differences in overall use. It is also possible, however, that a portion of the admissions with continuation of preadmission medication as the indication were started on these medications during a prior hospitalization. Thus, some portion of preadmission use is likely to represent failed medication reconciliation during a prior discharge. In this context, hospitalization may serve as an opportunity to evaluate the indication for ASM use even when these medications show up as preadmission medications.
There are additional limitations. First, the single‐center nature limits generalizability. Second, the first phase of our study, designed to obtain baseline data on ASM use, may have led to changes in prescribing prior to implementation of our CDS tool. Additionally, we did not validate the accuracy of each of the chosen indications, or the site of initial prescription in the case of preadmission exposure. Last, our study was not powered to investigate changes in rates of nosocomial gastrointestinal bleeding or nosocomial pneumonia owing to the infrequent nature of these complications.
In conclusion, we designed a simple computerized CDS intervention that was associated with a reduction in ASM use for stress ulcer prophylaxis in patients outside the ICU, a nonsignificant reduction in overall use, and no change in use on discharge. The majority of inpatient use represented continuation of preadmission medication, suggesting that interventions to improve the appropriateness of ASM prescribing should span the continuum of care. Future studies should investigate whether it is worthwhile and appropriate to reevaluate continued use of preadmission ASM during an inpatient stay.
Acknowledgements
The authors acknowledge Joshua Guthermann, MBA, and Jane Hui Chen Lim, MBA, for their assistance in the early phases of data analysis, and Long H. Ngo, PhD, for his statistical consultation.
Disclosures: Dr. Herzig was funded by a Young Clinician Research Award from the Center for Integration of Medicine and Innovative Technology, a nonprofit consortium of Boston teaching hospitals and universities, and grant number K23AG042459 from the National Institute on Aging. Dr. Marcantonio was funded by grant number K24AG035075 from the National Institute on Aging. The funding organizations had no involvement in any aspect of the study, including design, conduct, and reporting of the study. Dr. Herzig had full access to all of the data in the study and takes responsibility for the integrity of the data and the accuracy of the data analysis. Drs. Herzig and Marcantonio were responsible for the study concept and design. Drs. Herzig, Feinbloom, Howell, and Ms. Adra and Mr. Afonso were responsible for the acquisition of data. Drs. Herzig, Howell, Marcantonio, and Mr. Guess were responsible for the analysis and interpretation of the data. Dr. Herzig drafted the manuscript. All of the authors participated in the critical revision of the manuscript for important intellectual content. Drs. Herzig and Marcantonio were responsible for study supervision. The authors report no conflicts of interest.
Prior studies have found that up to 70% of acid‐suppressive medication (ASM) use in the hospital is not indicated, most commonly for stress ulcer prophylaxis in patients outside of the intensive care unit (ICU).[1, 2, 3, 4, 5, 6, 7] Accordingly, reducing inappropriate use of ASM for stress ulcer prophylaxis in hospitalized patients is 1 of the 5 opportunities for improved healthcare value identified by the Society of Hospital Medicine as part of the American Board of Internal Medicine's Choosing Wisely campaign.[8]
We designed and tested a computerized clinical decision support (CDS) intervention with the goal of reducing use of ASM for stress ulcer prophylaxis in hospitalized patients outside the ICU at an academic medical center.
METHODS
Study Design
We conducted a quasiexperimental study using an interrupted time series to analyze data collected prospectively during clinical care before and after implementation of our intervention. The study was deemed a quality improvement initiative by the Beth Israel Deaconess Medical Center Committee on Clinical Investigations/Institutional Review Board.
Patients and Setting
All admissions >18 years of age to a 649‐bed academic medical center in Boston, Massachusetts from September 12, 2011 through July 3, 2012 were included. The medical center consists of an East and West Campus, located across the street from each other. Care for both critically ill and noncritically ill medical and surgical patients occurs on both campuses. Differences include greater proportions of patients with gastrointestinal and oncologic conditions on the East Campus, and renal and cardiac conditions on the West Campus. Additionally, labor and delivery occurs exclusively on the East Campus, and the density of ICU beds is greater on the West Campus. Both campuses utilize a computer‐based provider order entry (POE) system.
Intervention
Our study was implemented in 2 phases (Figure 1).
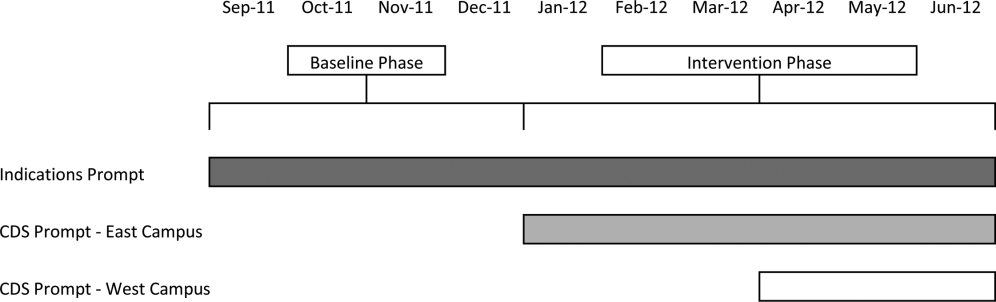
Baseline Phase
The purpose of the first phase was to obtain baseline data on ASM use prior to implementing our CDS tool designed to influence prescribing. During this baseline phase, a computerized prompt was activated through our POE system whenever a clinician initiated an order for ASM (histamine 2 receptor antagonists or proton pump inhibitors), asking the clinician to select the reason/reasons for the order based on the following predefined response options: (1) active/recent upper gastrointestinal bleed, (2) continuing preadmission medication, (3) Helicobacter pylori treatment, (4) prophylaxis in patient on medications that increase bleeding risk, (5) stress ulcer prophylaxis, (6) suspected/known peptic ulcer disease, gastritis, esophagitis, gastroesophageal reflux disease, and (7) other, with a free‐text box to input the indication. This indications prompt was rolled out to the entire medical center on September 12, 2011 and remained active for the duration of the study period.
Intervention Phase
In the second phase of the study, if a clinician selected stress ulcer prophylaxis as the only indication for ordering ASM, a CDS prompt alerted the clinician that Stress ulcer prophylaxis is not recommended for patients outside of the intensive care unit (ASHP Therapeutic Guidelines on Stress Ulcer Prophylaxis. Am J Health‐Syst Pharm. 1999, 56:347‐79). The clinician could then select either, For use in ICUOrder Medication, Choose Other Indication, or Cancel Order. This CDS prompt was rolled out in a staggered manner to the East Campus on January 3, 2012, followed by the West Campus on April 3, 2012.
Outcomes
The primary outcome was the rate of ASM use with stress ulcer prophylaxis selected as the only indication in a patient located outside of the ICU. We confirmed patient location in the 24 hours after the order was placed. Secondary outcomes were rates of overall ASM use, defined via pharmacy charges, and rates of use on discharge.
Statistical Analysis
To assure stable measurement of trends, we studied at least 3 months before and after the intervention on each campus. We used the Fisher exact test to compare the rates of our primary and secondary outcomes before and after the intervention, stratified by campus. For our primary outcomeat least 1 ASM order with stress ulcer prophylaxis selected as the only indication during hospitalizationwe developed a logistic regression model with a generalized estimating equation and exchangeable working correlation structure to control for admission characteristics (Table 1) and repeated admissions. Using a term for the interaction between time and the intervention, this model allowed us to assess changes in level and trend for the odds of a patient receiving at least 1 ASM order with stress ulcer prophylaxis as the only indication before, compared to after the intervention, stratified by campus. We used a 2‐sided type I error of <0.05 to indicate statistical significance.
Study Phase | Campus | |||
---|---|---|---|---|
East | West | |||
Baseline, n=3,747 | Intervention, n=6,191 | Baseline, n=11,177 | Intervention, n=5,285 | |
| ||||
Age, y, mean (SD) | 48.1 (18.5) | 47.7 (18.2) | 61.0 (18.0) | 60.3 (18.1) |
Gender, no. (%) | ||||
Female | 2744 (73.2%) | 4542 (73.4%) | 5551 (49.7%) | 2653 (50.2%) |
Male | 1003 (26.8%) | 1649 (26.6%) | 5626 (50.3%) | 2632 (49.8%) |
Race, no. (%) | ||||
Asian | 281 (7.5%) | 516 (8.3%) | 302 (2.7%) | 156 (3%) |
Black | 424 (11.3%) | 667 (10.8%) | 1426 (12.8%) | 685 (13%) |
Hispanic | 224 (6%) | 380 (6.1%) | 619 (5.5%) | 282 (5.3%) |
Other | 378 (10.1%) | 738 (11.9%) | 776 (6.9%) | 396 (7.5%) |
White | 2440 (65.1%) | 3890 (62.8%) | 8054 (72%) | 3766 (71.3%) |
Charlson score, mean (SD) | 0.8 (1.1) | 0.7 (1.1) | 1.5 (1.4) | 1.4 (1.4) |
Gastrointestinal bleeding, no. (%)* | 49 (1.3%) | 99 (1.6%) | 385 (3.4%) | 149 (2.8%) |
Other medication exposures, no. (%) | ||||
Therapeutic anticoagulant | 218 (5.8%) | 409 (6.6%) | 2242 (20.1%) | 1022 (19.3%) |
Prophylactic anticoagulant | 1081 (28.8%) | 1682 (27.2%) | 5999 (53.7%) | 2892 (54.7%) |
NSAID | 1899 (50.7%) | 3141 (50.7%) | 1248 (11.2%) | 575 (10.9%) |
Antiplatelet | 313 (8.4%) | 585 (9.4%) | 4543 (40.6%) | 2071 (39.2%) |
Admitting department, no. (%) | ||||
Surgery | 2507 (66.9%) | 4146 (67%) | 3255 (29.1%) | 1578 (29.9%) |
Nonsurgery | 1240 (33.1%) | 2045 (33%) | 7922 (70.9%) | 3707 (70.1%) |
Any ICU Stay, no. (%) | 217 (5.8%) | 383 (6.2%) | 2786 (24.9%) | 1252 (23.7%) |
RESULTS
There were 26,400 adult admissions during the study period, and 22,330 discrete orders for ASM. Overall, 12,056 (46%) admissions had at least 1 charge for ASM. Admission characteristics were similar before and after the intervention on each campus (Table 1).
Table 2 shows the indications chosen each time ASM was ordered, stratified by campus and study phase. Although selection of stress ulcer prophylaxis decreased on both campuses during the intervention phase, selection of continuing preadmission medication increased.
Study Phase | Campus | |||
---|---|---|---|---|
East | West | |||
Baseline, n=2,062 | Intervention, n=3,243 | Baseline, n=12,038 | Intervention, n=4,987 | |
| ||||
Indication* | ||||
Continuing preadmission medication | 910 (44.1%) | 1695 (52.3%) | 5597 (46.5%) | 2802 (56.2%) |
PUD, gastritis, esophagitis, GERD | 440 (21.3%) | 797 (24.6%) | 1303 (10.8%) | 582 (11.7%) |
Stress ulcer prophylaxis | 298 (14.4%) | 100 (3.1%) | 2659 (22.1%) | 681 (13.7%) |
Prophylaxis in patient on medications that increase bleeding risk | 226 (11.0%) | 259 (8.0%) | 965 (8.0%) | 411 (8.2%) |
Active/recent gastrointestinal bleed | 154 (7.5%) | 321 (9.9%) | 1450 (12.0%) | 515 (10.3) |
Helicobacter pylori treatment | 6 (0.2%) | 2 (0.1%) | 43 (0.4%) | 21 (0.4%) |
Other | 111 (5.4%) | 156 (4.8%) | 384 (3.2%) | 186 (3.7%) |
Table 3 shows the unadjusted comparison of outcomes between baseline and intervention phases on each campus. Use of ASM with stress ulcer prophylaxis as the only indication decreased during the intervention phase on both campuses. There was a nonsignificant reduction in overall rates of use on both campuses, and use on discharge was unchanged. Figure 2 demonstrates the unadjusted and modeled monthly rates of admissions with at least 1 ASM order with stress ulcer prophylaxis selected as the only indication, stratified by campus. After adjusting for the admission characteristics in Table 1, during the intervention phase on both campuses there was a significant immediate reduction in the odds of receiving an ASM with stress ulcer prophylaxis selected as the only indication (East Campus odds ratio [OR]: 0.36, 95% confidence interval [CI]: 0.180.71; West Campus OR: 0.41, 95% CI: 0.280.60), and a significant change in trend compared to the baseline phase (East Campus 1.5% daily decrease in odds of receiving ASM solely for stress ulcer prophylaxis, P=0.002; West Campus 0.9% daily decrease in odds of receiving ASM solely for stress ulcer prophylaxis, P=0.02).
Study Phase | Campus | |||||
---|---|---|---|---|---|---|
East | West | |||||
Baseline, n=3,747 | Intervention, n=6,191 | P Value* | Baseline, n=11,177 | Intervention, n=5,285 | P Value* | |
| ||||||
Outcome | ||||||
Any inappropriate acid‐suppressive exposure | 4.0% | 0.6% | <0.001 | 7.7% | 2.2% | <0.001 |
Any acid‐suppressive exposure | 33.1% | 31.8% | 0.16 | 54.5% | 52.9% | 0.05 |
Discharged on acid‐suppressive medication | 18.9% | 19.6% | 0.40 | 34.7% | 34.7% | 0.95 |
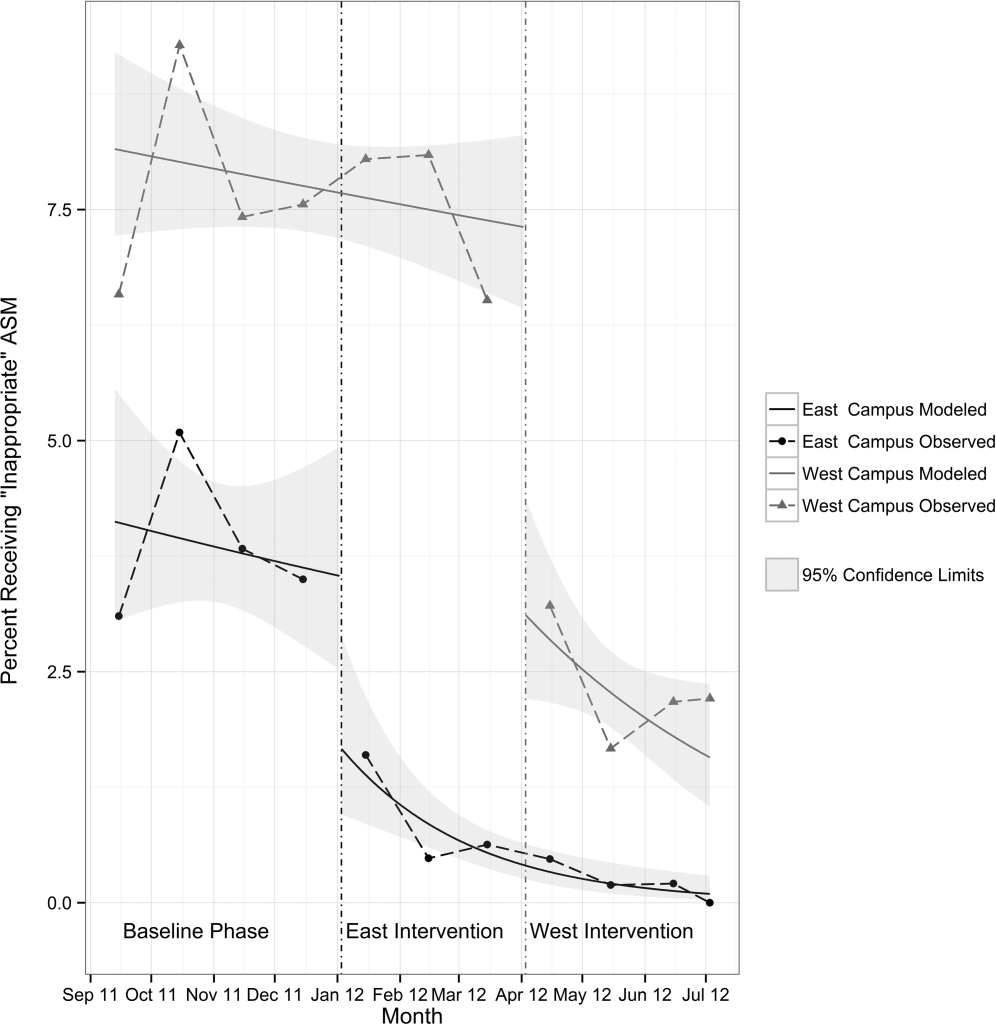
DISCUSSION
In this single‐center study, we found that a computerized CDS intervention resulted in a significant reduction in use of ASM for the sole purpose of stress ulcer prophylaxis in patients outside the ICU, a nonsignificant reduction in overall use, and no change in use on discharge. We found low rates of use for the isolated purpose of stress ulcer prophylaxis even before the intervention, and continuing preadmission medication was the most commonly selected indication throughout the study.
Although overall rates of ASM use declined after the intervention, the change was not statistically significant, and was not of the same magnitude as the decline in rates of use for the purpose of stress ulcer prophylaxis. This suggests that our intervention, in part, led to substitution of 1 indication for another. The indication that increased the most after rollout on both campuses was continuing preadmission medication. There are at least 2 possibilities for this finding: (1) the intervention prompted physicians to more accurately record the indication, or (2) physicians falsified the indication in order to execute the order. To explore these possibilities, we reviewed the charts of a random sample of 100 admissions during each of the baseline and intervention phases where continuing preadmission medication was selected as an indication for an ASM order. We found that 6/100 orders in the baseline phase and 7/100 orders in the intervention phase incorrectly indicated that the patient was on ASM prior to admission (P=0.77). This suggests that scenario 1 above is the more likely explanation for the increased use of this indication, and that the intervention, in part, simply unmasked the true rate of use at our medical center for the isolated purpose of stress ulcer prophylaxis.
These findings have implications for others attempting to use computerized CDS to better understand physician prescribing. They suggest that information collected through computer‐based interaction with clinicians at the point of care may not always be accurate or complete. As institutions increasingly use similar interventions to drive behavior, information obtained from such interaction should be validated, and when possible, patient outcomes should be measured.
Our findings suggest that rates of ASM use for the purpose of stress ulcer prophylaxis in the hospital may have declined over the last decade. Studies demonstrating that up to 70% of inpatient use of ASM was inappropriate were conducted 5 to 10 years ago.[1, 2, 3, 4, 5] Since then, studies have demonstrated risk of nosocomial infections in patients on ASM.[9, 10, 11] It is possible that the low rate of use for stress ulcer prophylaxis in our study is attributable to awareness of the risks of these medications, and limited our ability to detect differences in overall use. It is also possible, however, that a portion of the admissions with continuation of preadmission medication as the indication were started on these medications during a prior hospitalization. Thus, some portion of preadmission use is likely to represent failed medication reconciliation during a prior discharge. In this context, hospitalization may serve as an opportunity to evaluate the indication for ASM use even when these medications show up as preadmission medications.
There are additional limitations. First, the single‐center nature limits generalizability. Second, the first phase of our study, designed to obtain baseline data on ASM use, may have led to changes in prescribing prior to implementation of our CDS tool. Additionally, we did not validate the accuracy of each of the chosen indications, or the site of initial prescription in the case of preadmission exposure. Last, our study was not powered to investigate changes in rates of nosocomial gastrointestinal bleeding or nosocomial pneumonia owing to the infrequent nature of these complications.
In conclusion, we designed a simple computerized CDS intervention that was associated with a reduction in ASM use for stress ulcer prophylaxis in patients outside the ICU, a nonsignificant reduction in overall use, and no change in use on discharge. The majority of inpatient use represented continuation of preadmission medication, suggesting that interventions to improve the appropriateness of ASM prescribing should span the continuum of care. Future studies should investigate whether it is worthwhile and appropriate to reevaluate continued use of preadmission ASM during an inpatient stay.
Acknowledgements
The authors acknowledge Joshua Guthermann, MBA, and Jane Hui Chen Lim, MBA, for their assistance in the early phases of data analysis, and Long H. Ngo, PhD, for his statistical consultation.
Disclosures: Dr. Herzig was funded by a Young Clinician Research Award from the Center for Integration of Medicine and Innovative Technology, a nonprofit consortium of Boston teaching hospitals and universities, and grant number K23AG042459 from the National Institute on Aging. Dr. Marcantonio was funded by grant number K24AG035075 from the National Institute on Aging. The funding organizations had no involvement in any aspect of the study, including design, conduct, and reporting of the study. Dr. Herzig had full access to all of the data in the study and takes responsibility for the integrity of the data and the accuracy of the data analysis. Drs. Herzig and Marcantonio were responsible for the study concept and design. Drs. Herzig, Feinbloom, Howell, and Ms. Adra and Mr. Afonso were responsible for the acquisition of data. Drs. Herzig, Howell, Marcantonio, and Mr. Guess were responsible for the analysis and interpretation of the data. Dr. Herzig drafted the manuscript. All of the authors participated in the critical revision of the manuscript for important intellectual content. Drs. Herzig and Marcantonio were responsible for study supervision. The authors report no conflicts of interest.
- Stress ulcer prophylaxis in hospitalized patients not in intensive care units. Am J Health Syst Pharm. 2007;64(13):1396–1400. , .
- Magnitude and economic impact of inappropriate use of stress ulcer prophylaxis in non‐ICU hospitalized patients. Am J Gastroenterol. 2006;101(10):2200–2205. , .
- Stress‐ulcer prophylaxis for general medical patients: a review of the evidence. J Hosp Med. 2007;2(2):86–92. , .
- Hospital use of acid‐suppressive medications and its fall‐out on prescribing in general practice: a 1‐month survey. Aliment Pharmacol Ther. 2003;17(12):1503–1506. , , , et al.
- Inadequate use of acid‐suppressive therapy in hospitalized patients and its implications for general practice. Dig Dis Sci. 2005;50(12):2307–2311. , , , , , .
- Brief report: reducing inappropriate usage of stress ulcer prophylaxis among internal medicine residents. A practice‐based educational intervention. J Gen Intern Med. 2006;21(5):498–500. , .
- Inappropriate continuation of stress ulcer prophylactic therapy after discharge. Ann Pharmacother. 2007;41(10):1611–1616. , , .
- Choosing wisely in adult hospital medicine: five opportunities for improved healthcare value. J Hosp Med. 2013;8(9):486–492. , , , et al.
- Risk of Clostridium difficile diarrhea among hospital inpatients prescribed proton pump inhibitors: cohort and case‐control studies. CMAJ. 2004;171(1):33–38. , , , , .
- Iatrogenic gastric acid suppression and the risk of nosocomial Clostridium difficile infection. Arch Intern Med. 2010;170(9):784–790. , , , et al.
- Acid‐suppressive medication use and the risk for hospital‐acquired pneumonia. JAMA. 2009;301(20):2120–2128. , , , .
- Healthcare Cost and Utilization Project. Clinical classifications software (CCS) for ICD‐9‐CM. December 2009. Agency for Healthcare Research and Quality, Rockville, MD. Available at: www.hcup‐us.ahrq.gov/toolssoftware/ccs/ccs.jsp. Accessed June 18, 2014.
- Stress ulcer prophylaxis in hospitalized patients not in intensive care units. Am J Health Syst Pharm. 2007;64(13):1396–1400. , .
- Magnitude and economic impact of inappropriate use of stress ulcer prophylaxis in non‐ICU hospitalized patients. Am J Gastroenterol. 2006;101(10):2200–2205. , .
- Stress‐ulcer prophylaxis for general medical patients: a review of the evidence. J Hosp Med. 2007;2(2):86–92. , .
- Hospital use of acid‐suppressive medications and its fall‐out on prescribing in general practice: a 1‐month survey. Aliment Pharmacol Ther. 2003;17(12):1503–1506. , , , et al.
- Inadequate use of acid‐suppressive therapy in hospitalized patients and its implications for general practice. Dig Dis Sci. 2005;50(12):2307–2311. , , , , , .
- Brief report: reducing inappropriate usage of stress ulcer prophylaxis among internal medicine residents. A practice‐based educational intervention. J Gen Intern Med. 2006;21(5):498–500. , .
- Inappropriate continuation of stress ulcer prophylactic therapy after discharge. Ann Pharmacother. 2007;41(10):1611–1616. , , .
- Choosing wisely in adult hospital medicine: five opportunities for improved healthcare value. J Hosp Med. 2013;8(9):486–492. , , , et al.
- Risk of Clostridium difficile diarrhea among hospital inpatients prescribed proton pump inhibitors: cohort and case‐control studies. CMAJ. 2004;171(1):33–38. , , , , .
- Iatrogenic gastric acid suppression and the risk of nosocomial Clostridium difficile infection. Arch Intern Med. 2010;170(9):784–790. , , , et al.
- Acid‐suppressive medication use and the risk for hospital‐acquired pneumonia. JAMA. 2009;301(20):2120–2128. , , , .
- Healthcare Cost and Utilization Project. Clinical classifications software (CCS) for ICD‐9‐CM. December 2009. Agency for Healthcare Research and Quality, Rockville, MD. Available at: www.hcup‐us.ahrq.gov/toolssoftware/ccs/ccs.jsp. Accessed June 18, 2014.
Differentiating DNI From DNR
Since the introduction of defibrillation and closed chest cardiopulmonary resuscitation (CPR) in the 1950s, the ability to revive an arrested heart has been a realized possibility. Around the same time, endotracheal intubation with mechanical ventilation (MV) came into wide practice, allowing doctors to augment or even replace their patients' breathing. But just as the 1950s and 1960s saw the rise of these enhanced medical techniques, they also saw the increased importance of medical ethicsin particular, patient autonomy. A natural reaction to medicine's use of CPR and MV was the advent of advance directives and more specific do‐not‐resuscitate (DNR) and do‐not‐intubate (DNI) orders meant to protect a patient's ability to remain autonomous with their end of life decisions.[1]
Unfortunately, the code status discussions that lead to these orders often collapse cardiac arrest with prearrest respiratory failure and CPR with MV.[2, 3] This is a problem for a number of reasons. First, cardiac arrest and prearrest respiratory failure are unique end points, and though their respective treatments (CPR and MV) are often required simultaneously for an individual patient, they are distinct medical interventions with different goals, indications, and associated disease states. Although MV is typically a part of the cadre of interventions meant to ensure continued tissue oxygenation in the setting of a cardiac arrest, this accounts for <2% of indications for MV.[4] The vast majority of MV is used to treat prearrest causes of respiratory failure, such as pneumonia, congestive heart failure, acute exacerbations of chronic obstructive pulmonary disease, and following surgery.[4]
We do not believe these differences are adequately reflected in typical code status discussions.[2, 3] One study using audio‐recorded admission encounters included transcripts of hospitalist‐led code status discussions that all resembled the following: Physician: [I]f an emergency were to happenand your heart would (stop) or your breathing became so difficult that you needed to be attached to machines, would you want the nurses and doctors to attempt heroic measures to try to restart your heart and attach you to a breathing machine?[2] It would come as little surprise if a patient hearing this assumed that just 1 question were being asked and that decisions relating to any cause of respiratory failure (including prearrest causes) were being made. In practice, many physicians then extrapolate DNR orders to other treatment decision (including MV) and interpret them as precluding intubation, even for prearrest states.[5, 6, 7]
A second issue is that the mortality associated with cardiopulmonary arrest requiring CPR and prearrest respiratory failure requiring MV are not equal. Though the mortality after in‐hospital cardiac arrest has decreased over the last decade, it remains >75%.[8] The outcomes for MV for isolated respiratory failure, on the other hand, are not as grim; studies of the general population typically report mortality rates <40%. Despite this, descriptions of outcomes are often left out of goals of care discussions.[9, 10] For example, Sharma et al. recently reported that only one‐third of residents, including those who had undergone training on goals of care discussions, discussed outcomes.[9] And when outcomes are included, they are typically for CPR but not MV as an independent intervention for prearrest respiratory failure.[10] Given that many of the conditions that lead to respiratory failure are among the most common reasons for hospitalization,[11] distinguishing between decisions regarding CPR and prearrest MV with discussion of their associated outcomes is of particular importance to hospitalists. Failing to do so impedes patients from making informed autonomous decisions that incorporate an accurate understanding of the treatments being discussed.
Imagine you are caring for a 75‐year‐old man with a history of coronary artery disease and congestive heart failure now admitted with pneumonia. Given his age, admitting diagnosis, and comorbidities, you feel it would be appropriate to engage him in a discussion of goals of care. His chances of survival with near return to baseline after a cardiac arrest requiring CPR are not the same as his chances of surviving an episode of worsening pneumonia requiring MV. To discuss cardiac arrest and prearrest respiratory failure in the same breath, without acknowledging the differences, is misleading. Based on his goals and values, this patient may see a trial of MV as acceptable. One recent study supports this hypothesis, as 28% of hospitalized patients with a combined DNR/DNI order would accept a trial of MV for pneumonia.[12] If the genesis of these orders was our desire to ensure that patients' autonomous preferences are respected, we must actually know those preferences, and those preferences should be based on adequate information about the expected outcomes, highlighting the differences outlined above.
Some may consider separating CPR from MVtherefore allowing for more clearly separate DNR and DNI ordersproblematic, as it may result in a menu of choices for patients. However, although CPR and MV may be performed at the same time for the same patient, they do not overlap in 100% of their occurrences. This is conceptually different from discussing whether to use epinephrine versus vasopressin, for example, or offering options such as chest compressions alone. More clearly separating CPR from MV would not be dissimilar to what is done with renal dialysis; a patient may wish to be DNR while still electing to undergo dialysis for failing kidneys. Though the discussions surrounding renal dialysis are less urgent, this alone does not adequately explain why the topic is not routinely collapsed into the discussion of CPR. Instead, renal dialysis is an intervention with unique indications, goals, and outcomes; this is what prompts the separation. The same is true of MV.
No matter the situation, code status discussions should focus on determining an individual patient's values and goals of care and should guide physicians in provision (or omission) of certain interventions. For the patient with pneumonia described above, his goal may be to promote quality of life over extension of life. Although this may prompt a recommendation to forego CPR, (if it were felt that his quality of life, even after successful return of spontaneous circulation, would be low), it may not be inconsistent for him to accept a trial of MV were his pneumonia to get worse (if it were felt that he could quickly improve and return to a quality of life close to what he experienced before the episode of pneumonia). We recommend that when discussing options with patients, the indications for and outcomes of CPR and MV be more clearly separated. It may be as simple as saying, there are 2 different situations I would like to discuss with you, followed by a discussion of the associated scenarios and likely outcomes in the best judgment of the care team. For a hospitalist, framing the discussion of MV around anticipated causes of pre‐arrest respiratory failure (eg, pneumonia, acute pulmonary edema) is essential.
In conclusion, if DNR and DNI orders are going to meet their promise of ensuring patients make informed decisions congruent with their goals, then the discussions from which they follow will need to more clearly acknowledge the important differences in indications and outcomes. Although a patient's goals should still be the framework upon which decisions regarding interventions are made, an important distinction should be made between cardiopulmonary arrest and prearrest respiratory failure, with a more explicit accompanying discussion of how the corresponding interventions fit within the patient's overall goals of care.
Acknowledgements
The authors thank Rafael Campo, MD, and Sharon H. Chou, MD, for their suggestions and critical reading of this manuscript.
Disclosures:
Dr. Herzig was funded by grant number K23AG042459 from the National Institute on Aging.
- Orders not to resuscitate. N Engl J Med. 1976;295(7):364–366. , , .
- Code status discussions between attending hospitalist physicians and medical patients at hospital admission. J Gen Intern Med. 2010;26(4):359–366. , , , , .
- How do medical residents discuss resuscitation with patients? J Gen Intern Med. 1995;10(8):436–442. , , .
- Characteristics and outcomes in adult patients receiving mechanical ventilation: a 28‐day international study. JAMA. 2002;287(3):345–355. , , , et al.
- The effect of do‐not‐resuscitate orders on physician decision‐making. J Am Geriatr Soc. 2002;50(12):2057–2061. , .
- Hospital do‐not‐resuscitate orders: why they have failed and how to fix them. J Gen Intern Med. 2011;26(7):791–797. , , .
- Clinician perspectives regarding the do‐not‐resuscitate order. JAMA Pediatr. 2013;167(10):954–958. , , .
- Trends in survival after in‐hospital cardiac arrest. N Engl J Med. 2012;367(20):1912–1920. , , , , , .
- Unpacking resident‐led code status discussions: results from a mixed methods study. J Gen Intern Med. 2014;29(5):750–7. , , , , , .
- If asked, hospitalized patients will choose whether to receive life‐sustaining therapies. J Hosp Med. 2006;1(3):161–167. , , , et al.
- Healthcare Cost and Utilization Project (HCUP). HCUP Facts and Figures: Statistics on Hospital‐Based Care in the United States, 2009. Rockville, MD: Agency for Healthcare Research and Quality; 2011.
- Preferences for resuscitation and intubation among patients with do‐not‐resuscitate/do‐not‐intubate orders. Mayo Clin Proceed. 2013;88(7):658–665. , , , et al.
Since the introduction of defibrillation and closed chest cardiopulmonary resuscitation (CPR) in the 1950s, the ability to revive an arrested heart has been a realized possibility. Around the same time, endotracheal intubation with mechanical ventilation (MV) came into wide practice, allowing doctors to augment or even replace their patients' breathing. But just as the 1950s and 1960s saw the rise of these enhanced medical techniques, they also saw the increased importance of medical ethicsin particular, patient autonomy. A natural reaction to medicine's use of CPR and MV was the advent of advance directives and more specific do‐not‐resuscitate (DNR) and do‐not‐intubate (DNI) orders meant to protect a patient's ability to remain autonomous with their end of life decisions.[1]
Unfortunately, the code status discussions that lead to these orders often collapse cardiac arrest with prearrest respiratory failure and CPR with MV.[2, 3] This is a problem for a number of reasons. First, cardiac arrest and prearrest respiratory failure are unique end points, and though their respective treatments (CPR and MV) are often required simultaneously for an individual patient, they are distinct medical interventions with different goals, indications, and associated disease states. Although MV is typically a part of the cadre of interventions meant to ensure continued tissue oxygenation in the setting of a cardiac arrest, this accounts for <2% of indications for MV.[4] The vast majority of MV is used to treat prearrest causes of respiratory failure, such as pneumonia, congestive heart failure, acute exacerbations of chronic obstructive pulmonary disease, and following surgery.[4]
We do not believe these differences are adequately reflected in typical code status discussions.[2, 3] One study using audio‐recorded admission encounters included transcripts of hospitalist‐led code status discussions that all resembled the following: Physician: [I]f an emergency were to happenand your heart would (stop) or your breathing became so difficult that you needed to be attached to machines, would you want the nurses and doctors to attempt heroic measures to try to restart your heart and attach you to a breathing machine?[2] It would come as little surprise if a patient hearing this assumed that just 1 question were being asked and that decisions relating to any cause of respiratory failure (including prearrest causes) were being made. In practice, many physicians then extrapolate DNR orders to other treatment decision (including MV) and interpret them as precluding intubation, even for prearrest states.[5, 6, 7]
A second issue is that the mortality associated with cardiopulmonary arrest requiring CPR and prearrest respiratory failure requiring MV are not equal. Though the mortality after in‐hospital cardiac arrest has decreased over the last decade, it remains >75%.[8] The outcomes for MV for isolated respiratory failure, on the other hand, are not as grim; studies of the general population typically report mortality rates <40%. Despite this, descriptions of outcomes are often left out of goals of care discussions.[9, 10] For example, Sharma et al. recently reported that only one‐third of residents, including those who had undergone training on goals of care discussions, discussed outcomes.[9] And when outcomes are included, they are typically for CPR but not MV as an independent intervention for prearrest respiratory failure.[10] Given that many of the conditions that lead to respiratory failure are among the most common reasons for hospitalization,[11] distinguishing between decisions regarding CPR and prearrest MV with discussion of their associated outcomes is of particular importance to hospitalists. Failing to do so impedes patients from making informed autonomous decisions that incorporate an accurate understanding of the treatments being discussed.
Imagine you are caring for a 75‐year‐old man with a history of coronary artery disease and congestive heart failure now admitted with pneumonia. Given his age, admitting diagnosis, and comorbidities, you feel it would be appropriate to engage him in a discussion of goals of care. His chances of survival with near return to baseline after a cardiac arrest requiring CPR are not the same as his chances of surviving an episode of worsening pneumonia requiring MV. To discuss cardiac arrest and prearrest respiratory failure in the same breath, without acknowledging the differences, is misleading. Based on his goals and values, this patient may see a trial of MV as acceptable. One recent study supports this hypothesis, as 28% of hospitalized patients with a combined DNR/DNI order would accept a trial of MV for pneumonia.[12] If the genesis of these orders was our desire to ensure that patients' autonomous preferences are respected, we must actually know those preferences, and those preferences should be based on adequate information about the expected outcomes, highlighting the differences outlined above.
Some may consider separating CPR from MVtherefore allowing for more clearly separate DNR and DNI ordersproblematic, as it may result in a menu of choices for patients. However, although CPR and MV may be performed at the same time for the same patient, they do not overlap in 100% of their occurrences. This is conceptually different from discussing whether to use epinephrine versus vasopressin, for example, or offering options such as chest compressions alone. More clearly separating CPR from MV would not be dissimilar to what is done with renal dialysis; a patient may wish to be DNR while still electing to undergo dialysis for failing kidneys. Though the discussions surrounding renal dialysis are less urgent, this alone does not adequately explain why the topic is not routinely collapsed into the discussion of CPR. Instead, renal dialysis is an intervention with unique indications, goals, and outcomes; this is what prompts the separation. The same is true of MV.
No matter the situation, code status discussions should focus on determining an individual patient's values and goals of care and should guide physicians in provision (or omission) of certain interventions. For the patient with pneumonia described above, his goal may be to promote quality of life over extension of life. Although this may prompt a recommendation to forego CPR, (if it were felt that his quality of life, even after successful return of spontaneous circulation, would be low), it may not be inconsistent for him to accept a trial of MV were his pneumonia to get worse (if it were felt that he could quickly improve and return to a quality of life close to what he experienced before the episode of pneumonia). We recommend that when discussing options with patients, the indications for and outcomes of CPR and MV be more clearly separated. It may be as simple as saying, there are 2 different situations I would like to discuss with you, followed by a discussion of the associated scenarios and likely outcomes in the best judgment of the care team. For a hospitalist, framing the discussion of MV around anticipated causes of pre‐arrest respiratory failure (eg, pneumonia, acute pulmonary edema) is essential.
In conclusion, if DNR and DNI orders are going to meet their promise of ensuring patients make informed decisions congruent with their goals, then the discussions from which they follow will need to more clearly acknowledge the important differences in indications and outcomes. Although a patient's goals should still be the framework upon which decisions regarding interventions are made, an important distinction should be made between cardiopulmonary arrest and prearrest respiratory failure, with a more explicit accompanying discussion of how the corresponding interventions fit within the patient's overall goals of care.
Acknowledgements
The authors thank Rafael Campo, MD, and Sharon H. Chou, MD, for their suggestions and critical reading of this manuscript.
Disclosures:
Dr. Herzig was funded by grant number K23AG042459 from the National Institute on Aging.
Since the introduction of defibrillation and closed chest cardiopulmonary resuscitation (CPR) in the 1950s, the ability to revive an arrested heart has been a realized possibility. Around the same time, endotracheal intubation with mechanical ventilation (MV) came into wide practice, allowing doctors to augment or even replace their patients' breathing. But just as the 1950s and 1960s saw the rise of these enhanced medical techniques, they also saw the increased importance of medical ethicsin particular, patient autonomy. A natural reaction to medicine's use of CPR and MV was the advent of advance directives and more specific do‐not‐resuscitate (DNR) and do‐not‐intubate (DNI) orders meant to protect a patient's ability to remain autonomous with their end of life decisions.[1]
Unfortunately, the code status discussions that lead to these orders often collapse cardiac arrest with prearrest respiratory failure and CPR with MV.[2, 3] This is a problem for a number of reasons. First, cardiac arrest and prearrest respiratory failure are unique end points, and though their respective treatments (CPR and MV) are often required simultaneously for an individual patient, they are distinct medical interventions with different goals, indications, and associated disease states. Although MV is typically a part of the cadre of interventions meant to ensure continued tissue oxygenation in the setting of a cardiac arrest, this accounts for <2% of indications for MV.[4] The vast majority of MV is used to treat prearrest causes of respiratory failure, such as pneumonia, congestive heart failure, acute exacerbations of chronic obstructive pulmonary disease, and following surgery.[4]
We do not believe these differences are adequately reflected in typical code status discussions.[2, 3] One study using audio‐recorded admission encounters included transcripts of hospitalist‐led code status discussions that all resembled the following: Physician: [I]f an emergency were to happenand your heart would (stop) or your breathing became so difficult that you needed to be attached to machines, would you want the nurses and doctors to attempt heroic measures to try to restart your heart and attach you to a breathing machine?[2] It would come as little surprise if a patient hearing this assumed that just 1 question were being asked and that decisions relating to any cause of respiratory failure (including prearrest causes) were being made. In practice, many physicians then extrapolate DNR orders to other treatment decision (including MV) and interpret them as precluding intubation, even for prearrest states.[5, 6, 7]
A second issue is that the mortality associated with cardiopulmonary arrest requiring CPR and prearrest respiratory failure requiring MV are not equal. Though the mortality after in‐hospital cardiac arrest has decreased over the last decade, it remains >75%.[8] The outcomes for MV for isolated respiratory failure, on the other hand, are not as grim; studies of the general population typically report mortality rates <40%. Despite this, descriptions of outcomes are often left out of goals of care discussions.[9, 10] For example, Sharma et al. recently reported that only one‐third of residents, including those who had undergone training on goals of care discussions, discussed outcomes.[9] And when outcomes are included, they are typically for CPR but not MV as an independent intervention for prearrest respiratory failure.[10] Given that many of the conditions that lead to respiratory failure are among the most common reasons for hospitalization,[11] distinguishing between decisions regarding CPR and prearrest MV with discussion of their associated outcomes is of particular importance to hospitalists. Failing to do so impedes patients from making informed autonomous decisions that incorporate an accurate understanding of the treatments being discussed.
Imagine you are caring for a 75‐year‐old man with a history of coronary artery disease and congestive heart failure now admitted with pneumonia. Given his age, admitting diagnosis, and comorbidities, you feel it would be appropriate to engage him in a discussion of goals of care. His chances of survival with near return to baseline after a cardiac arrest requiring CPR are not the same as his chances of surviving an episode of worsening pneumonia requiring MV. To discuss cardiac arrest and prearrest respiratory failure in the same breath, without acknowledging the differences, is misleading. Based on his goals and values, this patient may see a trial of MV as acceptable. One recent study supports this hypothesis, as 28% of hospitalized patients with a combined DNR/DNI order would accept a trial of MV for pneumonia.[12] If the genesis of these orders was our desire to ensure that patients' autonomous preferences are respected, we must actually know those preferences, and those preferences should be based on adequate information about the expected outcomes, highlighting the differences outlined above.
Some may consider separating CPR from MVtherefore allowing for more clearly separate DNR and DNI ordersproblematic, as it may result in a menu of choices for patients. However, although CPR and MV may be performed at the same time for the same patient, they do not overlap in 100% of their occurrences. This is conceptually different from discussing whether to use epinephrine versus vasopressin, for example, or offering options such as chest compressions alone. More clearly separating CPR from MV would not be dissimilar to what is done with renal dialysis; a patient may wish to be DNR while still electing to undergo dialysis for failing kidneys. Though the discussions surrounding renal dialysis are less urgent, this alone does not adequately explain why the topic is not routinely collapsed into the discussion of CPR. Instead, renal dialysis is an intervention with unique indications, goals, and outcomes; this is what prompts the separation. The same is true of MV.
No matter the situation, code status discussions should focus on determining an individual patient's values and goals of care and should guide physicians in provision (or omission) of certain interventions. For the patient with pneumonia described above, his goal may be to promote quality of life over extension of life. Although this may prompt a recommendation to forego CPR, (if it were felt that his quality of life, even after successful return of spontaneous circulation, would be low), it may not be inconsistent for him to accept a trial of MV were his pneumonia to get worse (if it were felt that he could quickly improve and return to a quality of life close to what he experienced before the episode of pneumonia). We recommend that when discussing options with patients, the indications for and outcomes of CPR and MV be more clearly separated. It may be as simple as saying, there are 2 different situations I would like to discuss with you, followed by a discussion of the associated scenarios and likely outcomes in the best judgment of the care team. For a hospitalist, framing the discussion of MV around anticipated causes of pre‐arrest respiratory failure (eg, pneumonia, acute pulmonary edema) is essential.
In conclusion, if DNR and DNI orders are going to meet their promise of ensuring patients make informed decisions congruent with their goals, then the discussions from which they follow will need to more clearly acknowledge the important differences in indications and outcomes. Although a patient's goals should still be the framework upon which decisions regarding interventions are made, an important distinction should be made between cardiopulmonary arrest and prearrest respiratory failure, with a more explicit accompanying discussion of how the corresponding interventions fit within the patient's overall goals of care.
Acknowledgements
The authors thank Rafael Campo, MD, and Sharon H. Chou, MD, for their suggestions and critical reading of this manuscript.
Disclosures:
Dr. Herzig was funded by grant number K23AG042459 from the National Institute on Aging.
- Orders not to resuscitate. N Engl J Med. 1976;295(7):364–366. , , .
- Code status discussions between attending hospitalist physicians and medical patients at hospital admission. J Gen Intern Med. 2010;26(4):359–366. , , , , .
- How do medical residents discuss resuscitation with patients? J Gen Intern Med. 1995;10(8):436–442. , , .
- Characteristics and outcomes in adult patients receiving mechanical ventilation: a 28‐day international study. JAMA. 2002;287(3):345–355. , , , et al.
- The effect of do‐not‐resuscitate orders on physician decision‐making. J Am Geriatr Soc. 2002;50(12):2057–2061. , .
- Hospital do‐not‐resuscitate orders: why they have failed and how to fix them. J Gen Intern Med. 2011;26(7):791–797. , , .
- Clinician perspectives regarding the do‐not‐resuscitate order. JAMA Pediatr. 2013;167(10):954–958. , , .
- Trends in survival after in‐hospital cardiac arrest. N Engl J Med. 2012;367(20):1912–1920. , , , , , .
- Unpacking resident‐led code status discussions: results from a mixed methods study. J Gen Intern Med. 2014;29(5):750–7. , , , , , .
- If asked, hospitalized patients will choose whether to receive life‐sustaining therapies. J Hosp Med. 2006;1(3):161–167. , , , et al.
- Healthcare Cost and Utilization Project (HCUP). HCUP Facts and Figures: Statistics on Hospital‐Based Care in the United States, 2009. Rockville, MD: Agency for Healthcare Research and Quality; 2011.
- Preferences for resuscitation and intubation among patients with do‐not‐resuscitate/do‐not‐intubate orders. Mayo Clin Proceed. 2013;88(7):658–665. , , , et al.
- Orders not to resuscitate. N Engl J Med. 1976;295(7):364–366. , , .
- Code status discussions between attending hospitalist physicians and medical patients at hospital admission. J Gen Intern Med. 2010;26(4):359–366. , , , , .
- How do medical residents discuss resuscitation with patients? J Gen Intern Med. 1995;10(8):436–442. , , .
- Characteristics and outcomes in adult patients receiving mechanical ventilation: a 28‐day international study. JAMA. 2002;287(3):345–355. , , , et al.
- The effect of do‐not‐resuscitate orders on physician decision‐making. J Am Geriatr Soc. 2002;50(12):2057–2061. , .
- Hospital do‐not‐resuscitate orders: why they have failed and how to fix them. J Gen Intern Med. 2011;26(7):791–797. , , .
- Clinician perspectives regarding the do‐not‐resuscitate order. JAMA Pediatr. 2013;167(10):954–958. , , .
- Trends in survival after in‐hospital cardiac arrest. N Engl J Med. 2012;367(20):1912–1920. , , , , , .
- Unpacking resident‐led code status discussions: results from a mixed methods study. J Gen Intern Med. 2014;29(5):750–7. , , , , , .
- If asked, hospitalized patients will choose whether to receive life‐sustaining therapies. J Hosp Med. 2006;1(3):161–167. , , , et al.
- Healthcare Cost and Utilization Project (HCUP). HCUP Facts and Figures: Statistics on Hospital‐Based Care in the United States, 2009. Rockville, MD: Agency for Healthcare Research and Quality; 2011.
- Preferences for resuscitation and intubation among patients with do‐not‐resuscitate/do‐not‐intubate orders. Mayo Clin Proceed. 2013;88(7):658–665. , , , et al.
Management of Chronic Conditions
Over the past 2 decades, the care of the hospitalized patient has changed dramatically. Hospitalists now account for the care of more than one‐third of general medicine inpatients, and this number is likely to grow.[1] The emergence of hospital medicine has resulted in a partnership between primary care physicians (PCPs) and hospitalists wherein hospitalists focus on acute medical issues requiring hospitalization, whereas more chronic issues unrelated to the reason for hospitalization remain largely the domain of the PCP.[2, 3]
However, several evolving financial and quality incentives have already begun to blur the distinction between inpatient and outpatient care. First, as private and public payers increasingly scrutinize readmission rates, it has become clear that the responsibility for patient outcomes extends beyond the day of discharge.[4] The birth of Accountable Care Organizations and patient‐centered medical homes may further blur distinctions between what has traditionally constituted inpatient and outpatient care.[5] Bundled payments may force providers to ensure that each visit, whether hospital‐ or clinic‐based, is taken as an opportunity to enact meaningful change.[6] The Centers for Medicare and Medicaid Services (CMS) are already tracking hospital performance on institution of medical therapy for certain conditions regardless of their relatedness to the reason for hospitalization.[7]
No published literature has yet examined the attitudes of inpatient and outpatient providers regarding this issue. Through a case‐based survey conducted at 3 large urban academic medical centers, we aimed to assess opinions among hospitalists and PCPs regarding the role of hospitalists in the management of conditions unrelated to the reason for admission. Our study had 2 main objectives: (1) to determine whether surveyed physicians were more likely to rate an inpatient intervention as appropriate when it related to the reason for admission as compared to interventions unrelated to the reason for admission; and (2) to determine whether these attitudes differed between PCPs and hospitalists.
METHODS
Setting and Subjects
We surveyed hospitalists and hospital‐based PCPs at Beth Israel Deaconess Medical Center (BIDMC), Brigham and Women's Hospital, and Massachusetts General Hospital, 3 large academic medical centers in Boston, Massachusetts. Each hospitalist group includes both teaching and nonteaching services and admits patients from both the surveyed hospital‐based PCP groups and other nonhospital‐based PCP groups. All 3 study sites use electronic medical records with patient information for each hospital‐based PCP available to treating hospitalists.
Survey Design
Using a commercially available online product (SurveyMonkey, Palo Alto, CA), we created a 3‐part case‐based survey instrument. The first section included demographic questions regarding age, sex, primary clinical role (hospitalist or PCP), prior experience as a PCP (for hospitalists only) or a hospitalist (for PCPs only; defined as a position with >30% of clinical time as the attending of record in the inpatient setting), years of clinical experience, and hospital affiliation.
The second section aimed to indirectly assess physician opinions on the appropriateness of inpatient management of conditions unrelated to the reason for admission. It consisted of 6 paired case scenarios, each with an inpatient management decision for a hypothetical hospitalist (Table 1). For each pair, 1 case dealt with management of the condition prompting admission (eg, starting aspirin in a patient admitted with acute nonST‐elevation myocardial infarction). The partner case involved the same intervention (eg, starting aspirin) but for a patient with a chronic condition (eg, history of prior myocardial infarction) and an alternate admitting diagnosis (eg, cellulitis). In an attempt to mitigate concerns regarding the flow of information and communication between providers, the survey asked respondents to assume that the hospitalist has access to the patient's outpatient electronic medical record, and that the hospitalist communicates the details of any hospitalizations at the time of discharge. For each case, the physician was asked to rate the appropriateness of enacting the intervention without discussing it with the PCP on a 5‐point scale from very inappropriate to very appropriate. When a physician answered that an intervention was inappropriate or very inappropriate, an additional question soliciting reasons for inappropriateness was included, with multiple predefined answer choices, as well as the option of a free‐text reply under the other designation.
| |
Starting aspirin (related to the reason for admission) | A 60‐year‐old patient is admitted with a nonST‐elevation MI, medically managed without cardiac catheterization or percutaneous coronary intervention. Knowing that aspirin reduces mortality as part of secondary prevention in cardiovascular disease, how appropriate is it for the hospitalist to start the patient on this medication without discussing it with the primary care physician? |
Starting aspirin (unrelated to the reason for admission) | A 60‐year‐old patient with a past medical history of a prior nonST‐elevation MI that was medically managed is admitted to the hospital for treatment of cellulitis. The hospitalist notes the patient is not on aspirin at home. Knowing that aspirin reduces mortality as part of secondary prevention in cardiovascular disease, how appropriate is it for the hospitalist to start the patient on this medication without discussing it with the primary care physician? |
Starting spironolactone (related to the reason for admission) | A 70‐year‐old patient with a past medical history significant for NYHA class II congestive heart failure (LVEF of 20%) is admitted for acute on chronic, left‐sided systolic congestive heart failure. The patient has been maintained on furosemide, metoprolol, and lisinopril. Admission serum potassium and creatinine are both normal. Knowing that spironolactone decreases mortality in heart failure, how appropriate is it for the hospitalist to start this medication without discussing it with the primary care physician? |
Starting spironolactone (unrelated to the reason for admission) | A 70‐year‐old patient with a past history of NYHA class II congestive heart failure (LVEF of 20%) on furosemide, metoprolol, and lisinopril is admitted with pneumonia. Serum potassium and creatinine are both normal. Knowing that spironolactone decreases mortality in heart failure, how appropriate is it for the hospitalist to start this medication without discussing it with the primary care physician? |
Starting warfarin (related to the reason for admission) | A 75‐year‐old patient with a past medical history of hypertension and diabetes is admitted with new atrial fibrillation. Given the patient's CHADS2 score of 3, the hospitalist calculates that the patient has a significant risk of thromboembolic stroke. Knowing that warfarin will decrease the risk of thromboembolic stroke, how appropriate is it for the hospitalist to start the patient on this medication without discussing it with the primary care physician (assume that an outpatient anticoagulation clinic is able to see the patient within 3 days of discharge)? |
Starting warfarin (unrelated to the reason for admission) | A 75‐year‐old patient with a past medical history of hypertension, diabetes, and atrial fibrillation is admitted with pneumonia. The patient is not anticoagulation therapy. Given the patient's CHADS2 score of 3, the hospitalist calculates that the patient has a significant risk of thromboembolic stroke. Knowing that warfarin will decrease the risk of thromboembolic stroke, how appropriate is it for the hospitalist to start the patient on this medication without discussing it with the primary care physician (assume that an outpatient anticoagulation clinic is able to see the patient within 3 days of discharge)? |
Stopping proton pump inhibitor (related to the reason for admission) | A 65‐year‐old patient with a past medical history of GERD maintained on a proton pump inhibitor is admitted for treatment of Clostridium difficile colitis. The patient denies having any GERD‐like symptoms for several years. Knowing that proton pump inhibitors can increase the risk of C difficile colitis and recurrence (as well as pneumonia and osteoporosis), how appropriate is it for the hospitalist to initiate a taper of this medication without discussing it with the primary care physician? |
Stopping proton pump inhibitor (unrelated to the reason for admission) | A 65‐year‐old patient with a past medical history of GERD maintained on a proton pump inhibitor is admitted for treatment of a urinary tract infection. The patient denies having any GERD‐like symptoms for several years. Knowing that proton pump inhibitors can increase the risk of C difficile colitis and recurrence (as well as pneumonia and osteoporosis), how appropriate is it for the hospitalist to initiate a taper of this medication without discussing it with the primary care physician? |
Stopping statin or fibrate (related to the reason for admission) | A 60‐year‐old patient with a history of hyperlipidemia is admitted with an elevated creatine kinase to 5000. The hospitalist notes that the patient is on both simvastatin and gemfibrozil. The patient's most recent serum LDL was at goal. Knowing that coadministration of simvastatin and gemfibrozil can increase the risk of rhabdomyolysis, how appropriate is it for the hospitalist to stop one of these medications without discussing it with the primary care physician? |
Stopping statin or fibrate (unrelated to the reason for admission) | A 60‐year‐old patient is admitted with an acute diarrheal illness. The hospitalist notes that the patient is on both simvastatin and gemfibrozil. The patient's most recent LDL was at goal. Knowing that coadministration of simvastatin and gemfibrozil can increase the risk of rhabdomyolysis, how appropriate is it for the hospitalist to stop one of these medications without discussing it with the primary care physician? |
Changing statin (related to the reason for admission) | A 65‐year‐old patient with a past medical history of hyperlipidemia on maximum‐dose simvastatin is admitted with a nonST‐elevation MI. The patient's cholesterol is noted to be above goal. Knowing that improving lipid management reduces mortality in cardiovascular disease, how appropriate is it for the hospitalist to replace simvastatin with atorvastatin without discussing it with the primary care physician? |
Changing statin (unrelated to the reason for admission) | A 65‐year‐old patient with a past medical history of a prior nonST‐elevation MI that was medically managed and hyperlipidemia on maximum‐dose simvastatin is admitted with pneumonia. Incidentally, the hospitalist notes that the patient's cholesterol has been above goal for the last 2 years. Knowing that improving lipid management reduces mortality in cardiovascular disease, how appropriate is it for the hospitalist to replace simvastatin with atorvastatin without discussing it with the primary care physician? |
The third section aimed to directly assess physicians' opinions. It consisted of questions regarding the appropriateness of inpatient management of conditions related to and unrelated to a patient's reason for admission.
Prior to administration, we conducted focus groups of hospitalists and PCPs to help hypothesize current physician perceptions on inpatient management, assess physician understanding of survey cases and questions, and to evaluate survey length.
Survey Administration
Between October 23, 2012 and November 10, 2012, 3 emails containing a link to the online survey were sent to all hospitalist and hospital‐based PCPs at the 3 study institutions. The BIDMC Committee on Clinical Investigations, to whom authority was ceded by the remaining 2 study institutions, certified this research protocol as exempt.
Statistical Analysis
We hypothesized that respondents as a whole would be more likely to rate an intervention as appropriate or very appropriate if it was related to the reason for admission, compared to unrelated, and that there would be no difference between PCPs and hospitalists.
We used 2 and Fisher exact tests (where applicable) to compare categorical variables, and a nonparametric median test for continuous variables. We used the Fisher exact test to compare the percent of respondents rating each intervention as appropriate or very appropriate by relatedness or unrelatedness to the reason for admission, and by PCP vs hospitalist. To derive the relative risk (RR) of rating each intervention as appropriate or very appropriate by PCPs compared to hospitalists, adjusting for potential confounders including years out of residency and sex, we used multivariable generalized estimating equation models, each with a Poisson distribution error term, a log link, and an exchangeable working correlation structure to account for dependency of observations arising from clustering at either the hospital or participant level, depending on the comparison: for comparisons within a given case, we controlled for clustering at the hospital level; for comparisons of cases in aggregate, owing to multiple responses from each participant, we controlled for clustering at the individual level.
Assuming a 50% response rate from both PCPs and hospitalists, and that 50% of PCPs would rate a given intervention as appropriate, we calculated that we would have 90% power to detect a 50% increase in the proportion of hospitalists rating an intervention as appropriate as compared to PCPs, using an of .05.
RESULTS
Demographics
One hundred sixty‐two out of 295 providers (55%) responded to the survey (Table 2). The response rate did not differ between hospitalists (70 out of 128; 55%) and PCPs (92 out of 167; 55%). Female respondents made up 58.7% of the PCP and 50.0% of the hospitalist groups (P=0.34). On average, PCPs were older (P<0.001) with a greater median number of years since graduation from residency (P<0.001). A greater percentage of hospitalists spent more than three‐quarters of their time clinically (42.9% vs 19.6%, P=0.009).
Total, n=162 (100.0%) | PCP, n=92 (6.8%) | Hospitalist, n=70 (43.2%) | P Valuea | |
---|---|---|---|---|
| ||||
Hospital, n (%) | ||||
BIDMC | 79 (48.8) | 48 (60.8) | 31 (39.2) | 0.115 |
BWH | 36 (22.2) | 15 (41.7) | 21 (58.3) | |
MGH | 47 (29.0) | 29 (61.7) | 18 (38.3) | |
Sex, n (%) | ||||
Male | 73 (45.1) | 38 (41.3) | 35 (50.0) | 0.339 |
Female | 89 (54.9) | 54 (58.7) | 35 (50.0) | |
Age interval, y, n (%) | ||||
2534 | 36 (22.2) | 9 (9.8) | 27 (38.6) | <0.001 |
3544 | 67 (41.4) | 34 (37.0) | 33 (47.1) | |
4554 | 35 (21.6) | 29 (31.5) | 6 (8.6) | |
5564 | 19 (11.7) | 16 (17.4) | 3 (4.3) | |
6574 | 5 (3.1) | 4 (4.4) | 1 (1.4) | |
Years out of residency, median (IQR) | 10 (417) | 15 (74) | 5 (211) | <0.001 |
Clinical FTE, n (%) | ||||
0.25 | 30 (18.6) | 22 (23.9) | 8 (11.4) | 0.009 |
0.260.50 | 41 (25.3) | 25 (27.2) | 16 (22.9) | |
0.510.75 | 43 (26.5) | 27 (29.4) | 16 (22.9) | |
>0.75 | 48 (29.6) | 18 (19.6) | 30 (42.9) | |
Worked as PCP?b | ||||
Yes | 6 (8.6) | |||
No | 64 (91.4) | |||
Worked as hospitalist? | ||||
Yes | 11 (12.0) | |||
No | 81 (88.0) | |||
AOR for admitted patients | ||||
Always | 16 (17.4) | |||
Mostly | 8 (8.7) | |||
Rarely | 7 (7.6) | |||
Never | 60 (65.2) |
Appropriateness of Inpatient Management Based on Admitting Diagnosis
For each of the 6 case pairings individually and in aggregate, respondents were significantly more likely to deem the intervention appropriate or very appropriate if it was related to the reason for admission, compared to those interventions unrelated to the reason for admission (in aggregate, 78.9% vs 38.8% respectively, P<0.001). For example, whereas 96.9% felt that the addition of aspirin in a patient admitted with acute myocardial infarction (MI) was appropriate, only 54.3% felt it appropriate to start aspirin in a patient with a prior history of MI admitted with cellulitis (P<0.001). Significant differences (all P values <0.001) were seen for all case pairs: starting spironolactone (68.1% when related to the reason for reason for admission vs 43.1% when unrelated to reason for admission); starting warfarin (62.3% vs 23.3%), stopping proton pump inhibitor (72.3% vs 42.8%), stopping statin or fibrate (90.6% vs 28.3%), and changing statin (83.0% vs 40.5%).
Appropriateness of Inpatient Management based on Primary Role
Table 3 compares the percent of PCPs and hospitalists rating each intervention as appropriate or very appropriate, by relatedness of the intervention to the reason for admission. In both unadjusted and adjusted comparisons for all cases in aggregate, PCPs were significantly more likely than hospitalists to rate the inpatient interventions as appropriate or very appropriate when the intervention was related to the reason for admission (83.4% of PCP responses vs 73.0% of hospitalist responses, P<0.001; RR: 1.2, 95% confidence interval [CI]: 1.11.3), unrelated to the reason for admission (44.7% vs 31.1%, P<0.001; RR: 1.5, 95% CI: 1.11.9), and overall (64.1% vs 52.1%, P<0.001; RR: 1.3, 95% CI: 1.11.4).
Relationship to Admission Diagnosis | PCP, n (%) | Hospitalist, n (%) | P Value | Adjusted RR | 95% CI |
---|---|---|---|---|---|
| |||||
Related | 453 (83.4) | 303 (73.0) | <0.001 | 1.2a | 1.11.3 |
Unrelated | 242 (44.7) | 129 (31.1) | <0.001 | 1.5a | 1.11.9 |
Overall | 695 (64.1) | 432 (52.1) | <0.001 | 1.3b | 1.11.4 |
Reasons for Inappropriate Designation
Among those respondents rating an intervention as inappropriate or very inappropriate, the 3 most common reasons selected as explanation for perceived inappropriateness from our predefined answer choices were: This medication will necessitate follow‐up testing/monitoring, for which the PCP will be responsible (chosen by physicians in 49.4% of instances); I am not confident that the hospitalist will have access to all of the medical history necessary to make this decision (35.7%); and Even if the hospitalist has all of the medical history and reviews it, the PCP should be involved in all decisions surrounding new medications (34.6%). The least common explanation chosen was I do not believe this is an appropriate pharmacologic intervention for this particular medical problem (6.5%). See Table 4 for a complete list of explanations, overall and stratified by PCP/hospitalist.
Predefined Reason for Inappropriateness | Total, n=583 (%) | PCP, n=318 (%) | Hospitalist, n=265 (%) | P Value |
---|---|---|---|---|
| ||||
This medication will necessitate follow‐up testing/monitoring, for which the PCP will be responsible. | 288 (49.4) | 151 (47.5) | 137 (51.7) | 0.32 |
I am not confident that the hospitalist will have access to all of the medical history necessary to make this decision. | 208 (35.7) | 98 (30.8) | 110 (41.5) | 0.009 |
Even if the hospitalist has all of the medical history and reviews it, the PCP should be involved in all decisions surrounding new medications. | 201 (34.5) | 125 (39.3) | 76 (28.7) | 0.009 |
I am not confident that the hospitalist will adequately review the medical history necessary to make this decision. | 184 (31.6) | 130 (40.9) | 54 (20.4) | <0.001 |
Even if the hospitalist has all of the medical history, I do not believe hospitalization is the right time to start this new medication | 106 (21.4) | 69 (21.7) | 56 (21.1) | 0.92 |
I am not confident that the hospitalist will appropriately discuss the risks and benefits of this new medication with the patient. | 106 (18.2) | 85 (26.7) | 21 (7.9) | <0.001 |
The benefit of this medication will be too remote to justify starting it in the acute setting. | 66 (11.3) | 40 (12.6) | 26 (9.8) | 0.36 |
I do not believe this is an appropriate pharmacologic intervention for this particular medical problem. | 38 (6.5) | 27 (8.5) | 11 (4.2) | 0.04 |
There were significant differences in the proportion of PCPs and hospitalists choosing several of the prespecified reasons for inappropriateness. Although hospitalists were more likely than PCPs to select I am not confident that the hospitalist will have access to all of the medical history necessary to make this decision (chosen by 41.5% of hospitalists vs 30.8% of PCPs, P=0.009), PCPs were more likely than hospitalists to select, I am not confident that the hospitalist will adequately review the medical history necessary to make this decision (chosen by 40.9% of PCPs vs 20.4% of hospitalists, P<0.001) and I am not confident that the hospitalist will appropriately discuss the risks and benefits of this new medication with the patient (26.7% of PCPs vs 9.8% of hospitalists, P<0.001).
Opinions on Current Management of Conditions Related and Unrelated to Admission
A minority of PCPs and hospitalists agreed or strongly agreed that hospitalists should play a larger role in the management of medical conditions unrelated to the reason for admission (28.1% of PCPs vs 34.8% of hospitalists; P=0.39).
DISCUSSION
In this survey‐based study of PCPs and hospitalists across 3 Boston‐area academic medical centers, we found that: (1) physicians were more likely to see inpatient interventions as appropriate when those interventions dealt with the reason for admission as compared to interventions unrelated to the reason for admission; and (2) PCPs were more likely than hospitalists to feel that inpatient interventions were appropriate, even when they targeted chronic conditions unrelated to the reason for admission. To our knowledge, this study represents the first investigation into the attitudes of PCPs and hospitalists regarding the inpatient management of conditions unrelated to the reason for admission.
That surveyed physicians, regardless of role, were less likely to report an intervention unrelated to the reason for hospitalization as appropriateeven those with likely mortality benefitsuggests that opportunities to affect meaningful change may be missed in a healthcare system that adheres to strict inpatient and outpatient roles. For several of the cases, a change in therapy could lead to benefit soon after implementation. For example, aldosterone antagonists reduce mortality as early as 1 month after initiation in select patients.[8] If a major goal of inpatient care is to reduce 30‐day mortality, it could be argued that hospitalists should more actively adjust congestive heart failure therapy in appropriate inpatients, even when this is not their admitting diagnosis.
For some conditions, CMS is already tracking hospital performance. Since 2003, hospitals have been required to document whether a patient with congestive heart failure (either acute or chronic and regardless of the relationship to admission) was prescribed an angiotensin‐converting enzyme (ACE) inhibitor or angiotensin receptor blocker (ARB) at the time of discharge.[7] CMS has determined that the proven benefits of ACE inhibitors and ARBs confer hospital accountability for their inclusion in appropriate patients, independent of the acuity of heart failure. There are many potential therapeutic maneuvers on which health systems (and their physicians) may be graded, and accepting the view that a hospitalization provides a window of opportunity for medical optimization may allow for more fruitful interventions and more patient‐centered care.
Despite the potential benefits of addressing chronic medical issues during hospitalization, there are important limitations on what can and/or should be done in the hospital setting. Hospitalizations are a time of fluctuating clinical status, which continues beyond discharge and is often accompanied by several medication changes.[9] In our study, more than 20% of those who believed that a medication intervention was inappropriate selected I do not believe hospitalization is the right time to start this new medication as one of their explanations. Although some medication interventions have been shown in randomized controlled trials to reduce short‐term mortality, the ability to generalize these findings to the average hospitalized patient with multiple comorbidities, concurrent medication changes, and rapidly fluctuating clinical status is limited. Furthermore, there are interventions most would agree should not be dealt with in the hospital (eg, screening colonoscopy) and encounters that may be too short to allow for change (eg, 24‐hour observation). These issues notwithstanding, the average 4‐day hospitalization likely provides an opportunity for monitored change that may currently be underutilized.
Our study suggests several additional explanations for physicians' current practice and opinions. Only 6.5% of respondents who answered that an intervention was inappropriate indicated as a justification that I do not believe this is an appropriate pharmacologic intervention for this particular medical problem. This suggests that the hesitancy has little to do with a lack of benefit but instead relates to systems issues (eg, access to all pertinent records and concerns regarding follow‐up testing) and perceived limitations to what a hospitalist should and should not do without actively involving the PCP. There are likely additional concerns that the medical record and/or patient histories do not fully outline the rationale for exclusion or inclusion of particular medications. Advances in information technology that enhance information exchange and enable streamlined communication may help to address these perceived barriers. However, an additional barrier may be trust, as PCPs appear more concerned that hospitalists will not review all the pertinent records or discuss risks and benefits before enacting important medication changes. Increased attempts at communication between hospitalists and outpatient providers may help to build trust and alleviate concerns regarding the loss of information that often occurs both on admission and at discharge.
We also noted that PCPs were more likely than hospitalists to feel that inpatient interventions were appropriate, even when targeting chronic conditions unrelated to the reason for admission. It may be that PCPs, with an increasing number of problems to address per outpatient visit,[10, 11] are more open to hospitalists managing any medical problems during their patients' admissions. At the same time, with increased acuity[12, 13, 14] and shortened length of stays,[15, 16] hospitalists have only a finite amount of time to ensure acute issues are managed, leaving potentially modifiable chronic conditions to the outpatient setting. These differences aside, a minority of both PCPs and hospitalists in our study were ready to embrace the idea of hospitalists playing a larger role in the management of conditions unrelated to the reason for hospitalization.
Even though our study benefits from its multisite design, there are a number of limitations. First, although we crafted our survey with input from general medicine focus groups, our survey instrument has not been validated. In addition, the cases are necessarily contrived and do not take into account the complexities of inpatient medicine. Furthermore, though our goal was to create paired cases that isolate a management decision as being simply based on whether it was related or unrelated to the reason for admission, it is possible that other factors, not captured by our survey, influenced the responses. For example, the benefits of aspirin as part of secondary prevention are not equal to the benefits in an acute MI.[17]
In an attempt to isolate the hospitalists' role in these management decisions, respondents were instructed to assume that the decisions were being made without discussing it with the primary care physician, but that the hospitalist would communicate the details of any hospitalization at the time of discharge. They were also instructed to assume that the hospitalist has access to the patient's outpatient electronic medical record. These assumptions were made to address concerns regarding the flow of information and communication, and to simulate the ideal system from a communication and information accessibility standpoint. Had these assumptions not been placed, the responses may have differed. It is likely that PCPs and hospitalists practicing in systems without shared, accessible inpatient/outpatient medical records would be even more reluctant to enact medication changes unrelated to the reason for admission.
Along the same lines, our physician cohort consisted of several metropolitan academic physician groups, in which hospitalists have had a presence for almost 20 years. As a result, our findings may not be generalizable to other academic hospitals, community‐based hospitalist programs, or nonhospital‐based PCP practices. Finally, we do not know whether survey nonresponders differed from responders in ways that could have meaningfully affected our results.
In conclusion, our findings suggest that both PCPs and hospitalists see the management of conditions unrelated to the reason for admission as less appropriate than the management of conditions related to the reason for admission. Our findings also suggest that PCPs may be more open to this practice when compared to hospitalists. Failure to capitalize on opportunities for meaningful medical interventions, independent of patient location, suggests a possible lack of patient centeredness in the current partnership between PCPs and hospitalists. Further studies should examine existing barriers and investigate interventions designed to address those barriers, in an effort to improve both quality of care and the degree of patient‐centeredness in our current healthcare system.
Disclosures: Dr. Herzig is supported by a grant from the National Institute on Aging (K23 AG042459). Dr. Herzig had full access to all of the data in the study and takes responsibility for the integrity of the data and the accuracy of the data analysis. Author contributions: study concept and design, Breu, Allen‐Dicker, Mueller, Herzig; acquisition of data, Breu, Allen‐Dicker, Mueller, Palamara, Herzig; analysis and interpretation of data, Breu, Allen‐Dicker, Hinami, Herzig; drafting of the manuscript, Breu; critical revision of the manuscript for important intellectual content, Breu, Allen‐Dicker, Mueller, Palamara, Hinami, Herzig; statistical analysis, Allen‐Dicker, Hinami, Herzig; study supervision, Breu, Herzig. This study was presented as a poster at the Society of Hospital Medicine National Meeting, Washington, DC, May 17, 2013.
- Growth in the care of older patients by hospitalists in the United States. N Engl J Med. 2009;360(11):1102–1112. , , , .
- The emerging role of “hospitalists” in the American health care system. N Engl J Med. 1996;335(7):514–517. , .
- An introduction to the hospitalist model. Ann Intern Med. 1999;130(4 pt 2):338–342. .
- Hospital readmission as an accountability measure. JAMA. 2011;305(5):504–505. , .
- A national strategy to put accountable care into practice. Health Aff (Millwood). 2010;29(5):982–990. , , , , .
- Keeping score under a global payment system. N Engl J Med. 2012;366(5):393–395. .
- Reporting Hospital Quality Data for Annual Payment Update. Available at: http://www.cms.gov/Medicare/Quality‐Initiatives‐Patient‐Assessment‐Instruments/HospitalQualityInits/Downloads/HospitalRHQDAPU200808. Accessed December 18, 2013.
- Eplerenone in patients with systolic heart failure and mild symptoms. N Engl J Med. 2011;364(1):11–21. , , , et al.
- How are drug regimen changes during hospitalisation handled after discharge: a cohort study. BMJ Open. 2012;2(6):e001461. , , , , , .
- Primary care visit duration and quality: does good care take longer? Arch Intern Med. 2009;169(20):1866–1872. , , .
- The increasing number of clinical items addressed during the time of adult primary care visits. J Gen Intern Med. 2008;23(12):2058–2065. , , ,
- Multiple chronic conditions among adults aged 45 and over: trends over the past 10 years. NCHS Data Brief. 2012;(100):1–8. , , .
- Prevalence of multiple chronic conditions in the United States' Medicare population. Health Qual Life Outcomes. 2009;7(1):82. , , .
- Multiple chronic conditions: prevalence, health consequences, and implications for quality, care management, and costs. J Gen Intern Med. 2007;22(suppl 3):391–395. , , , et al.
- Associations between reduced hospital length of stay and 30‐day readmission rate and mortality: 14‐year experience in 129 Veterans Affairs hospitals. Ann Intern Med. 2012;157(12):837–845. , , , et al.
- Trends in length of stay and short‐term outcomes among Medicare patients hospitalized for heart failure, 1993–2006. JAMA. 2010;303(21):2141–2147. , , , et al.
- Antithrombotic Trialists' Collaboration. Collaborative meta‐analysis of randomised trials of antiplatelet therapy for prevention of death, myocardial infarction, and stroke in high risk patients. BMJ. 2002;324(7329):71–86.
Over the past 2 decades, the care of the hospitalized patient has changed dramatically. Hospitalists now account for the care of more than one‐third of general medicine inpatients, and this number is likely to grow.[1] The emergence of hospital medicine has resulted in a partnership between primary care physicians (PCPs) and hospitalists wherein hospitalists focus on acute medical issues requiring hospitalization, whereas more chronic issues unrelated to the reason for hospitalization remain largely the domain of the PCP.[2, 3]
However, several evolving financial and quality incentives have already begun to blur the distinction between inpatient and outpatient care. First, as private and public payers increasingly scrutinize readmission rates, it has become clear that the responsibility for patient outcomes extends beyond the day of discharge.[4] The birth of Accountable Care Organizations and patient‐centered medical homes may further blur distinctions between what has traditionally constituted inpatient and outpatient care.[5] Bundled payments may force providers to ensure that each visit, whether hospital‐ or clinic‐based, is taken as an opportunity to enact meaningful change.[6] The Centers for Medicare and Medicaid Services (CMS) are already tracking hospital performance on institution of medical therapy for certain conditions regardless of their relatedness to the reason for hospitalization.[7]
No published literature has yet examined the attitudes of inpatient and outpatient providers regarding this issue. Through a case‐based survey conducted at 3 large urban academic medical centers, we aimed to assess opinions among hospitalists and PCPs regarding the role of hospitalists in the management of conditions unrelated to the reason for admission. Our study had 2 main objectives: (1) to determine whether surveyed physicians were more likely to rate an inpatient intervention as appropriate when it related to the reason for admission as compared to interventions unrelated to the reason for admission; and (2) to determine whether these attitudes differed between PCPs and hospitalists.
METHODS
Setting and Subjects
We surveyed hospitalists and hospital‐based PCPs at Beth Israel Deaconess Medical Center (BIDMC), Brigham and Women's Hospital, and Massachusetts General Hospital, 3 large academic medical centers in Boston, Massachusetts. Each hospitalist group includes both teaching and nonteaching services and admits patients from both the surveyed hospital‐based PCP groups and other nonhospital‐based PCP groups. All 3 study sites use electronic medical records with patient information for each hospital‐based PCP available to treating hospitalists.
Survey Design
Using a commercially available online product (SurveyMonkey, Palo Alto, CA), we created a 3‐part case‐based survey instrument. The first section included demographic questions regarding age, sex, primary clinical role (hospitalist or PCP), prior experience as a PCP (for hospitalists only) or a hospitalist (for PCPs only; defined as a position with >30% of clinical time as the attending of record in the inpatient setting), years of clinical experience, and hospital affiliation.
The second section aimed to indirectly assess physician opinions on the appropriateness of inpatient management of conditions unrelated to the reason for admission. It consisted of 6 paired case scenarios, each with an inpatient management decision for a hypothetical hospitalist (Table 1). For each pair, 1 case dealt with management of the condition prompting admission (eg, starting aspirin in a patient admitted with acute nonST‐elevation myocardial infarction). The partner case involved the same intervention (eg, starting aspirin) but for a patient with a chronic condition (eg, history of prior myocardial infarction) and an alternate admitting diagnosis (eg, cellulitis). In an attempt to mitigate concerns regarding the flow of information and communication between providers, the survey asked respondents to assume that the hospitalist has access to the patient's outpatient electronic medical record, and that the hospitalist communicates the details of any hospitalizations at the time of discharge. For each case, the physician was asked to rate the appropriateness of enacting the intervention without discussing it with the PCP on a 5‐point scale from very inappropriate to very appropriate. When a physician answered that an intervention was inappropriate or very inappropriate, an additional question soliciting reasons for inappropriateness was included, with multiple predefined answer choices, as well as the option of a free‐text reply under the other designation.
| |
Starting aspirin (related to the reason for admission) | A 60‐year‐old patient is admitted with a nonST‐elevation MI, medically managed without cardiac catheterization or percutaneous coronary intervention. Knowing that aspirin reduces mortality as part of secondary prevention in cardiovascular disease, how appropriate is it for the hospitalist to start the patient on this medication without discussing it with the primary care physician? |
Starting aspirin (unrelated to the reason for admission) | A 60‐year‐old patient with a past medical history of a prior nonST‐elevation MI that was medically managed is admitted to the hospital for treatment of cellulitis. The hospitalist notes the patient is not on aspirin at home. Knowing that aspirin reduces mortality as part of secondary prevention in cardiovascular disease, how appropriate is it for the hospitalist to start the patient on this medication without discussing it with the primary care physician? |
Starting spironolactone (related to the reason for admission) | A 70‐year‐old patient with a past medical history significant for NYHA class II congestive heart failure (LVEF of 20%) is admitted for acute on chronic, left‐sided systolic congestive heart failure. The patient has been maintained on furosemide, metoprolol, and lisinopril. Admission serum potassium and creatinine are both normal. Knowing that spironolactone decreases mortality in heart failure, how appropriate is it for the hospitalist to start this medication without discussing it with the primary care physician? |
Starting spironolactone (unrelated to the reason for admission) | A 70‐year‐old patient with a past history of NYHA class II congestive heart failure (LVEF of 20%) on furosemide, metoprolol, and lisinopril is admitted with pneumonia. Serum potassium and creatinine are both normal. Knowing that spironolactone decreases mortality in heart failure, how appropriate is it for the hospitalist to start this medication without discussing it with the primary care physician? |
Starting warfarin (related to the reason for admission) | A 75‐year‐old patient with a past medical history of hypertension and diabetes is admitted with new atrial fibrillation. Given the patient's CHADS2 score of 3, the hospitalist calculates that the patient has a significant risk of thromboembolic stroke. Knowing that warfarin will decrease the risk of thromboembolic stroke, how appropriate is it for the hospitalist to start the patient on this medication without discussing it with the primary care physician (assume that an outpatient anticoagulation clinic is able to see the patient within 3 days of discharge)? |
Starting warfarin (unrelated to the reason for admission) | A 75‐year‐old patient with a past medical history of hypertension, diabetes, and atrial fibrillation is admitted with pneumonia. The patient is not anticoagulation therapy. Given the patient's CHADS2 score of 3, the hospitalist calculates that the patient has a significant risk of thromboembolic stroke. Knowing that warfarin will decrease the risk of thromboembolic stroke, how appropriate is it for the hospitalist to start the patient on this medication without discussing it with the primary care physician (assume that an outpatient anticoagulation clinic is able to see the patient within 3 days of discharge)? |
Stopping proton pump inhibitor (related to the reason for admission) | A 65‐year‐old patient with a past medical history of GERD maintained on a proton pump inhibitor is admitted for treatment of Clostridium difficile colitis. The patient denies having any GERD‐like symptoms for several years. Knowing that proton pump inhibitors can increase the risk of C difficile colitis and recurrence (as well as pneumonia and osteoporosis), how appropriate is it for the hospitalist to initiate a taper of this medication without discussing it with the primary care physician? |
Stopping proton pump inhibitor (unrelated to the reason for admission) | A 65‐year‐old patient with a past medical history of GERD maintained on a proton pump inhibitor is admitted for treatment of a urinary tract infection. The patient denies having any GERD‐like symptoms for several years. Knowing that proton pump inhibitors can increase the risk of C difficile colitis and recurrence (as well as pneumonia and osteoporosis), how appropriate is it for the hospitalist to initiate a taper of this medication without discussing it with the primary care physician? |
Stopping statin or fibrate (related to the reason for admission) | A 60‐year‐old patient with a history of hyperlipidemia is admitted with an elevated creatine kinase to 5000. The hospitalist notes that the patient is on both simvastatin and gemfibrozil. The patient's most recent serum LDL was at goal. Knowing that coadministration of simvastatin and gemfibrozil can increase the risk of rhabdomyolysis, how appropriate is it for the hospitalist to stop one of these medications without discussing it with the primary care physician? |
Stopping statin or fibrate (unrelated to the reason for admission) | A 60‐year‐old patient is admitted with an acute diarrheal illness. The hospitalist notes that the patient is on both simvastatin and gemfibrozil. The patient's most recent LDL was at goal. Knowing that coadministration of simvastatin and gemfibrozil can increase the risk of rhabdomyolysis, how appropriate is it for the hospitalist to stop one of these medications without discussing it with the primary care physician? |
Changing statin (related to the reason for admission) | A 65‐year‐old patient with a past medical history of hyperlipidemia on maximum‐dose simvastatin is admitted with a nonST‐elevation MI. The patient's cholesterol is noted to be above goal. Knowing that improving lipid management reduces mortality in cardiovascular disease, how appropriate is it for the hospitalist to replace simvastatin with atorvastatin without discussing it with the primary care physician? |
Changing statin (unrelated to the reason for admission) | A 65‐year‐old patient with a past medical history of a prior nonST‐elevation MI that was medically managed and hyperlipidemia on maximum‐dose simvastatin is admitted with pneumonia. Incidentally, the hospitalist notes that the patient's cholesterol has been above goal for the last 2 years. Knowing that improving lipid management reduces mortality in cardiovascular disease, how appropriate is it for the hospitalist to replace simvastatin with atorvastatin without discussing it with the primary care physician? |
The third section aimed to directly assess physicians' opinions. It consisted of questions regarding the appropriateness of inpatient management of conditions related to and unrelated to a patient's reason for admission.
Prior to administration, we conducted focus groups of hospitalists and PCPs to help hypothesize current physician perceptions on inpatient management, assess physician understanding of survey cases and questions, and to evaluate survey length.
Survey Administration
Between October 23, 2012 and November 10, 2012, 3 emails containing a link to the online survey were sent to all hospitalist and hospital‐based PCPs at the 3 study institutions. The BIDMC Committee on Clinical Investigations, to whom authority was ceded by the remaining 2 study institutions, certified this research protocol as exempt.
Statistical Analysis
We hypothesized that respondents as a whole would be more likely to rate an intervention as appropriate or very appropriate if it was related to the reason for admission, compared to unrelated, and that there would be no difference between PCPs and hospitalists.
We used 2 and Fisher exact tests (where applicable) to compare categorical variables, and a nonparametric median test for continuous variables. We used the Fisher exact test to compare the percent of respondents rating each intervention as appropriate or very appropriate by relatedness or unrelatedness to the reason for admission, and by PCP vs hospitalist. To derive the relative risk (RR) of rating each intervention as appropriate or very appropriate by PCPs compared to hospitalists, adjusting for potential confounders including years out of residency and sex, we used multivariable generalized estimating equation models, each with a Poisson distribution error term, a log link, and an exchangeable working correlation structure to account for dependency of observations arising from clustering at either the hospital or participant level, depending on the comparison: for comparisons within a given case, we controlled for clustering at the hospital level; for comparisons of cases in aggregate, owing to multiple responses from each participant, we controlled for clustering at the individual level.
Assuming a 50% response rate from both PCPs and hospitalists, and that 50% of PCPs would rate a given intervention as appropriate, we calculated that we would have 90% power to detect a 50% increase in the proportion of hospitalists rating an intervention as appropriate as compared to PCPs, using an of .05.
RESULTS
Demographics
One hundred sixty‐two out of 295 providers (55%) responded to the survey (Table 2). The response rate did not differ between hospitalists (70 out of 128; 55%) and PCPs (92 out of 167; 55%). Female respondents made up 58.7% of the PCP and 50.0% of the hospitalist groups (P=0.34). On average, PCPs were older (P<0.001) with a greater median number of years since graduation from residency (P<0.001). A greater percentage of hospitalists spent more than three‐quarters of their time clinically (42.9% vs 19.6%, P=0.009).
Total, n=162 (100.0%) | PCP, n=92 (6.8%) | Hospitalist, n=70 (43.2%) | P Valuea | |
---|---|---|---|---|
| ||||
Hospital, n (%) | ||||
BIDMC | 79 (48.8) | 48 (60.8) | 31 (39.2) | 0.115 |
BWH | 36 (22.2) | 15 (41.7) | 21 (58.3) | |
MGH | 47 (29.0) | 29 (61.7) | 18 (38.3) | |
Sex, n (%) | ||||
Male | 73 (45.1) | 38 (41.3) | 35 (50.0) | 0.339 |
Female | 89 (54.9) | 54 (58.7) | 35 (50.0) | |
Age interval, y, n (%) | ||||
2534 | 36 (22.2) | 9 (9.8) | 27 (38.6) | <0.001 |
3544 | 67 (41.4) | 34 (37.0) | 33 (47.1) | |
4554 | 35 (21.6) | 29 (31.5) | 6 (8.6) | |
5564 | 19 (11.7) | 16 (17.4) | 3 (4.3) | |
6574 | 5 (3.1) | 4 (4.4) | 1 (1.4) | |
Years out of residency, median (IQR) | 10 (417) | 15 (74) | 5 (211) | <0.001 |
Clinical FTE, n (%) | ||||
0.25 | 30 (18.6) | 22 (23.9) | 8 (11.4) | 0.009 |
0.260.50 | 41 (25.3) | 25 (27.2) | 16 (22.9) | |
0.510.75 | 43 (26.5) | 27 (29.4) | 16 (22.9) | |
>0.75 | 48 (29.6) | 18 (19.6) | 30 (42.9) | |
Worked as PCP?b | ||||
Yes | 6 (8.6) | |||
No | 64 (91.4) | |||
Worked as hospitalist? | ||||
Yes | 11 (12.0) | |||
No | 81 (88.0) | |||
AOR for admitted patients | ||||
Always | 16 (17.4) | |||
Mostly | 8 (8.7) | |||
Rarely | 7 (7.6) | |||
Never | 60 (65.2) |
Appropriateness of Inpatient Management Based on Admitting Diagnosis
For each of the 6 case pairings individually and in aggregate, respondents were significantly more likely to deem the intervention appropriate or very appropriate if it was related to the reason for admission, compared to those interventions unrelated to the reason for admission (in aggregate, 78.9% vs 38.8% respectively, P<0.001). For example, whereas 96.9% felt that the addition of aspirin in a patient admitted with acute myocardial infarction (MI) was appropriate, only 54.3% felt it appropriate to start aspirin in a patient with a prior history of MI admitted with cellulitis (P<0.001). Significant differences (all P values <0.001) were seen for all case pairs: starting spironolactone (68.1% when related to the reason for reason for admission vs 43.1% when unrelated to reason for admission); starting warfarin (62.3% vs 23.3%), stopping proton pump inhibitor (72.3% vs 42.8%), stopping statin or fibrate (90.6% vs 28.3%), and changing statin (83.0% vs 40.5%).
Appropriateness of Inpatient Management based on Primary Role
Table 3 compares the percent of PCPs and hospitalists rating each intervention as appropriate or very appropriate, by relatedness of the intervention to the reason for admission. In both unadjusted and adjusted comparisons for all cases in aggregate, PCPs were significantly more likely than hospitalists to rate the inpatient interventions as appropriate or very appropriate when the intervention was related to the reason for admission (83.4% of PCP responses vs 73.0% of hospitalist responses, P<0.001; RR: 1.2, 95% confidence interval [CI]: 1.11.3), unrelated to the reason for admission (44.7% vs 31.1%, P<0.001; RR: 1.5, 95% CI: 1.11.9), and overall (64.1% vs 52.1%, P<0.001; RR: 1.3, 95% CI: 1.11.4).
Relationship to Admission Diagnosis | PCP, n (%) | Hospitalist, n (%) | P Value | Adjusted RR | 95% CI |
---|---|---|---|---|---|
| |||||
Related | 453 (83.4) | 303 (73.0) | <0.001 | 1.2a | 1.11.3 |
Unrelated | 242 (44.7) | 129 (31.1) | <0.001 | 1.5a | 1.11.9 |
Overall | 695 (64.1) | 432 (52.1) | <0.001 | 1.3b | 1.11.4 |
Reasons for Inappropriate Designation
Among those respondents rating an intervention as inappropriate or very inappropriate, the 3 most common reasons selected as explanation for perceived inappropriateness from our predefined answer choices were: This medication will necessitate follow‐up testing/monitoring, for which the PCP will be responsible (chosen by physicians in 49.4% of instances); I am not confident that the hospitalist will have access to all of the medical history necessary to make this decision (35.7%); and Even if the hospitalist has all of the medical history and reviews it, the PCP should be involved in all decisions surrounding new medications (34.6%). The least common explanation chosen was I do not believe this is an appropriate pharmacologic intervention for this particular medical problem (6.5%). See Table 4 for a complete list of explanations, overall and stratified by PCP/hospitalist.
Predefined Reason for Inappropriateness | Total, n=583 (%) | PCP, n=318 (%) | Hospitalist, n=265 (%) | P Value |
---|---|---|---|---|
| ||||
This medication will necessitate follow‐up testing/monitoring, for which the PCP will be responsible. | 288 (49.4) | 151 (47.5) | 137 (51.7) | 0.32 |
I am not confident that the hospitalist will have access to all of the medical history necessary to make this decision. | 208 (35.7) | 98 (30.8) | 110 (41.5) | 0.009 |
Even if the hospitalist has all of the medical history and reviews it, the PCP should be involved in all decisions surrounding new medications. | 201 (34.5) | 125 (39.3) | 76 (28.7) | 0.009 |
I am not confident that the hospitalist will adequately review the medical history necessary to make this decision. | 184 (31.6) | 130 (40.9) | 54 (20.4) | <0.001 |
Even if the hospitalist has all of the medical history, I do not believe hospitalization is the right time to start this new medication | 106 (21.4) | 69 (21.7) | 56 (21.1) | 0.92 |
I am not confident that the hospitalist will appropriately discuss the risks and benefits of this new medication with the patient. | 106 (18.2) | 85 (26.7) | 21 (7.9) | <0.001 |
The benefit of this medication will be too remote to justify starting it in the acute setting. | 66 (11.3) | 40 (12.6) | 26 (9.8) | 0.36 |
I do not believe this is an appropriate pharmacologic intervention for this particular medical problem. | 38 (6.5) | 27 (8.5) | 11 (4.2) | 0.04 |
There were significant differences in the proportion of PCPs and hospitalists choosing several of the prespecified reasons for inappropriateness. Although hospitalists were more likely than PCPs to select I am not confident that the hospitalist will have access to all of the medical history necessary to make this decision (chosen by 41.5% of hospitalists vs 30.8% of PCPs, P=0.009), PCPs were more likely than hospitalists to select, I am not confident that the hospitalist will adequately review the medical history necessary to make this decision (chosen by 40.9% of PCPs vs 20.4% of hospitalists, P<0.001) and I am not confident that the hospitalist will appropriately discuss the risks and benefits of this new medication with the patient (26.7% of PCPs vs 9.8% of hospitalists, P<0.001).
Opinions on Current Management of Conditions Related and Unrelated to Admission
A minority of PCPs and hospitalists agreed or strongly agreed that hospitalists should play a larger role in the management of medical conditions unrelated to the reason for admission (28.1% of PCPs vs 34.8% of hospitalists; P=0.39).
DISCUSSION
In this survey‐based study of PCPs and hospitalists across 3 Boston‐area academic medical centers, we found that: (1) physicians were more likely to see inpatient interventions as appropriate when those interventions dealt with the reason for admission as compared to interventions unrelated to the reason for admission; and (2) PCPs were more likely than hospitalists to feel that inpatient interventions were appropriate, even when they targeted chronic conditions unrelated to the reason for admission. To our knowledge, this study represents the first investigation into the attitudes of PCPs and hospitalists regarding the inpatient management of conditions unrelated to the reason for admission.
That surveyed physicians, regardless of role, were less likely to report an intervention unrelated to the reason for hospitalization as appropriateeven those with likely mortality benefitsuggests that opportunities to affect meaningful change may be missed in a healthcare system that adheres to strict inpatient and outpatient roles. For several of the cases, a change in therapy could lead to benefit soon after implementation. For example, aldosterone antagonists reduce mortality as early as 1 month after initiation in select patients.[8] If a major goal of inpatient care is to reduce 30‐day mortality, it could be argued that hospitalists should more actively adjust congestive heart failure therapy in appropriate inpatients, even when this is not their admitting diagnosis.
For some conditions, CMS is already tracking hospital performance. Since 2003, hospitals have been required to document whether a patient with congestive heart failure (either acute or chronic and regardless of the relationship to admission) was prescribed an angiotensin‐converting enzyme (ACE) inhibitor or angiotensin receptor blocker (ARB) at the time of discharge.[7] CMS has determined that the proven benefits of ACE inhibitors and ARBs confer hospital accountability for their inclusion in appropriate patients, independent of the acuity of heart failure. There are many potential therapeutic maneuvers on which health systems (and their physicians) may be graded, and accepting the view that a hospitalization provides a window of opportunity for medical optimization may allow for more fruitful interventions and more patient‐centered care.
Despite the potential benefits of addressing chronic medical issues during hospitalization, there are important limitations on what can and/or should be done in the hospital setting. Hospitalizations are a time of fluctuating clinical status, which continues beyond discharge and is often accompanied by several medication changes.[9] In our study, more than 20% of those who believed that a medication intervention was inappropriate selected I do not believe hospitalization is the right time to start this new medication as one of their explanations. Although some medication interventions have been shown in randomized controlled trials to reduce short‐term mortality, the ability to generalize these findings to the average hospitalized patient with multiple comorbidities, concurrent medication changes, and rapidly fluctuating clinical status is limited. Furthermore, there are interventions most would agree should not be dealt with in the hospital (eg, screening colonoscopy) and encounters that may be too short to allow for change (eg, 24‐hour observation). These issues notwithstanding, the average 4‐day hospitalization likely provides an opportunity for monitored change that may currently be underutilized.
Our study suggests several additional explanations for physicians' current practice and opinions. Only 6.5% of respondents who answered that an intervention was inappropriate indicated as a justification that I do not believe this is an appropriate pharmacologic intervention for this particular medical problem. This suggests that the hesitancy has little to do with a lack of benefit but instead relates to systems issues (eg, access to all pertinent records and concerns regarding follow‐up testing) and perceived limitations to what a hospitalist should and should not do without actively involving the PCP. There are likely additional concerns that the medical record and/or patient histories do not fully outline the rationale for exclusion or inclusion of particular medications. Advances in information technology that enhance information exchange and enable streamlined communication may help to address these perceived barriers. However, an additional barrier may be trust, as PCPs appear more concerned that hospitalists will not review all the pertinent records or discuss risks and benefits before enacting important medication changes. Increased attempts at communication between hospitalists and outpatient providers may help to build trust and alleviate concerns regarding the loss of information that often occurs both on admission and at discharge.
We also noted that PCPs were more likely than hospitalists to feel that inpatient interventions were appropriate, even when targeting chronic conditions unrelated to the reason for admission. It may be that PCPs, with an increasing number of problems to address per outpatient visit,[10, 11] are more open to hospitalists managing any medical problems during their patients' admissions. At the same time, with increased acuity[12, 13, 14] and shortened length of stays,[15, 16] hospitalists have only a finite amount of time to ensure acute issues are managed, leaving potentially modifiable chronic conditions to the outpatient setting. These differences aside, a minority of both PCPs and hospitalists in our study were ready to embrace the idea of hospitalists playing a larger role in the management of conditions unrelated to the reason for hospitalization.
Even though our study benefits from its multisite design, there are a number of limitations. First, although we crafted our survey with input from general medicine focus groups, our survey instrument has not been validated. In addition, the cases are necessarily contrived and do not take into account the complexities of inpatient medicine. Furthermore, though our goal was to create paired cases that isolate a management decision as being simply based on whether it was related or unrelated to the reason for admission, it is possible that other factors, not captured by our survey, influenced the responses. For example, the benefits of aspirin as part of secondary prevention are not equal to the benefits in an acute MI.[17]
In an attempt to isolate the hospitalists' role in these management decisions, respondents were instructed to assume that the decisions were being made without discussing it with the primary care physician, but that the hospitalist would communicate the details of any hospitalization at the time of discharge. They were also instructed to assume that the hospitalist has access to the patient's outpatient electronic medical record. These assumptions were made to address concerns regarding the flow of information and communication, and to simulate the ideal system from a communication and information accessibility standpoint. Had these assumptions not been placed, the responses may have differed. It is likely that PCPs and hospitalists practicing in systems without shared, accessible inpatient/outpatient medical records would be even more reluctant to enact medication changes unrelated to the reason for admission.
Along the same lines, our physician cohort consisted of several metropolitan academic physician groups, in which hospitalists have had a presence for almost 20 years. As a result, our findings may not be generalizable to other academic hospitals, community‐based hospitalist programs, or nonhospital‐based PCP practices. Finally, we do not know whether survey nonresponders differed from responders in ways that could have meaningfully affected our results.
In conclusion, our findings suggest that both PCPs and hospitalists see the management of conditions unrelated to the reason for admission as less appropriate than the management of conditions related to the reason for admission. Our findings also suggest that PCPs may be more open to this practice when compared to hospitalists. Failure to capitalize on opportunities for meaningful medical interventions, independent of patient location, suggests a possible lack of patient centeredness in the current partnership between PCPs and hospitalists. Further studies should examine existing barriers and investigate interventions designed to address those barriers, in an effort to improve both quality of care and the degree of patient‐centeredness in our current healthcare system.
Disclosures: Dr. Herzig is supported by a grant from the National Institute on Aging (K23 AG042459). Dr. Herzig had full access to all of the data in the study and takes responsibility for the integrity of the data and the accuracy of the data analysis. Author contributions: study concept and design, Breu, Allen‐Dicker, Mueller, Herzig; acquisition of data, Breu, Allen‐Dicker, Mueller, Palamara, Herzig; analysis and interpretation of data, Breu, Allen‐Dicker, Hinami, Herzig; drafting of the manuscript, Breu; critical revision of the manuscript for important intellectual content, Breu, Allen‐Dicker, Mueller, Palamara, Hinami, Herzig; statistical analysis, Allen‐Dicker, Hinami, Herzig; study supervision, Breu, Herzig. This study was presented as a poster at the Society of Hospital Medicine National Meeting, Washington, DC, May 17, 2013.
Over the past 2 decades, the care of the hospitalized patient has changed dramatically. Hospitalists now account for the care of more than one‐third of general medicine inpatients, and this number is likely to grow.[1] The emergence of hospital medicine has resulted in a partnership between primary care physicians (PCPs) and hospitalists wherein hospitalists focus on acute medical issues requiring hospitalization, whereas more chronic issues unrelated to the reason for hospitalization remain largely the domain of the PCP.[2, 3]
However, several evolving financial and quality incentives have already begun to blur the distinction between inpatient and outpatient care. First, as private and public payers increasingly scrutinize readmission rates, it has become clear that the responsibility for patient outcomes extends beyond the day of discharge.[4] The birth of Accountable Care Organizations and patient‐centered medical homes may further blur distinctions between what has traditionally constituted inpatient and outpatient care.[5] Bundled payments may force providers to ensure that each visit, whether hospital‐ or clinic‐based, is taken as an opportunity to enact meaningful change.[6] The Centers for Medicare and Medicaid Services (CMS) are already tracking hospital performance on institution of medical therapy for certain conditions regardless of their relatedness to the reason for hospitalization.[7]
No published literature has yet examined the attitudes of inpatient and outpatient providers regarding this issue. Through a case‐based survey conducted at 3 large urban academic medical centers, we aimed to assess opinions among hospitalists and PCPs regarding the role of hospitalists in the management of conditions unrelated to the reason for admission. Our study had 2 main objectives: (1) to determine whether surveyed physicians were more likely to rate an inpatient intervention as appropriate when it related to the reason for admission as compared to interventions unrelated to the reason for admission; and (2) to determine whether these attitudes differed between PCPs and hospitalists.
METHODS
Setting and Subjects
We surveyed hospitalists and hospital‐based PCPs at Beth Israel Deaconess Medical Center (BIDMC), Brigham and Women's Hospital, and Massachusetts General Hospital, 3 large academic medical centers in Boston, Massachusetts. Each hospitalist group includes both teaching and nonteaching services and admits patients from both the surveyed hospital‐based PCP groups and other nonhospital‐based PCP groups. All 3 study sites use electronic medical records with patient information for each hospital‐based PCP available to treating hospitalists.
Survey Design
Using a commercially available online product (SurveyMonkey, Palo Alto, CA), we created a 3‐part case‐based survey instrument. The first section included demographic questions regarding age, sex, primary clinical role (hospitalist or PCP), prior experience as a PCP (for hospitalists only) or a hospitalist (for PCPs only; defined as a position with >30% of clinical time as the attending of record in the inpatient setting), years of clinical experience, and hospital affiliation.
The second section aimed to indirectly assess physician opinions on the appropriateness of inpatient management of conditions unrelated to the reason for admission. It consisted of 6 paired case scenarios, each with an inpatient management decision for a hypothetical hospitalist (Table 1). For each pair, 1 case dealt with management of the condition prompting admission (eg, starting aspirin in a patient admitted with acute nonST‐elevation myocardial infarction). The partner case involved the same intervention (eg, starting aspirin) but for a patient with a chronic condition (eg, history of prior myocardial infarction) and an alternate admitting diagnosis (eg, cellulitis). In an attempt to mitigate concerns regarding the flow of information and communication between providers, the survey asked respondents to assume that the hospitalist has access to the patient's outpatient electronic medical record, and that the hospitalist communicates the details of any hospitalizations at the time of discharge. For each case, the physician was asked to rate the appropriateness of enacting the intervention without discussing it with the PCP on a 5‐point scale from very inappropriate to very appropriate. When a physician answered that an intervention was inappropriate or very inappropriate, an additional question soliciting reasons for inappropriateness was included, with multiple predefined answer choices, as well as the option of a free‐text reply under the other designation.
| |
Starting aspirin (related to the reason for admission) | A 60‐year‐old patient is admitted with a nonST‐elevation MI, medically managed without cardiac catheterization or percutaneous coronary intervention. Knowing that aspirin reduces mortality as part of secondary prevention in cardiovascular disease, how appropriate is it for the hospitalist to start the patient on this medication without discussing it with the primary care physician? |
Starting aspirin (unrelated to the reason for admission) | A 60‐year‐old patient with a past medical history of a prior nonST‐elevation MI that was medically managed is admitted to the hospital for treatment of cellulitis. The hospitalist notes the patient is not on aspirin at home. Knowing that aspirin reduces mortality as part of secondary prevention in cardiovascular disease, how appropriate is it for the hospitalist to start the patient on this medication without discussing it with the primary care physician? |
Starting spironolactone (related to the reason for admission) | A 70‐year‐old patient with a past medical history significant for NYHA class II congestive heart failure (LVEF of 20%) is admitted for acute on chronic, left‐sided systolic congestive heart failure. The patient has been maintained on furosemide, metoprolol, and lisinopril. Admission serum potassium and creatinine are both normal. Knowing that spironolactone decreases mortality in heart failure, how appropriate is it for the hospitalist to start this medication without discussing it with the primary care physician? |
Starting spironolactone (unrelated to the reason for admission) | A 70‐year‐old patient with a past history of NYHA class II congestive heart failure (LVEF of 20%) on furosemide, metoprolol, and lisinopril is admitted with pneumonia. Serum potassium and creatinine are both normal. Knowing that spironolactone decreases mortality in heart failure, how appropriate is it for the hospitalist to start this medication without discussing it with the primary care physician? |
Starting warfarin (related to the reason for admission) | A 75‐year‐old patient with a past medical history of hypertension and diabetes is admitted with new atrial fibrillation. Given the patient's CHADS2 score of 3, the hospitalist calculates that the patient has a significant risk of thromboembolic stroke. Knowing that warfarin will decrease the risk of thromboembolic stroke, how appropriate is it for the hospitalist to start the patient on this medication without discussing it with the primary care physician (assume that an outpatient anticoagulation clinic is able to see the patient within 3 days of discharge)? |
Starting warfarin (unrelated to the reason for admission) | A 75‐year‐old patient with a past medical history of hypertension, diabetes, and atrial fibrillation is admitted with pneumonia. The patient is not anticoagulation therapy. Given the patient's CHADS2 score of 3, the hospitalist calculates that the patient has a significant risk of thromboembolic stroke. Knowing that warfarin will decrease the risk of thromboembolic stroke, how appropriate is it for the hospitalist to start the patient on this medication without discussing it with the primary care physician (assume that an outpatient anticoagulation clinic is able to see the patient within 3 days of discharge)? |
Stopping proton pump inhibitor (related to the reason for admission) | A 65‐year‐old patient with a past medical history of GERD maintained on a proton pump inhibitor is admitted for treatment of Clostridium difficile colitis. The patient denies having any GERD‐like symptoms for several years. Knowing that proton pump inhibitors can increase the risk of C difficile colitis and recurrence (as well as pneumonia and osteoporosis), how appropriate is it for the hospitalist to initiate a taper of this medication without discussing it with the primary care physician? |
Stopping proton pump inhibitor (unrelated to the reason for admission) | A 65‐year‐old patient with a past medical history of GERD maintained on a proton pump inhibitor is admitted for treatment of a urinary tract infection. The patient denies having any GERD‐like symptoms for several years. Knowing that proton pump inhibitors can increase the risk of C difficile colitis and recurrence (as well as pneumonia and osteoporosis), how appropriate is it for the hospitalist to initiate a taper of this medication without discussing it with the primary care physician? |
Stopping statin or fibrate (related to the reason for admission) | A 60‐year‐old patient with a history of hyperlipidemia is admitted with an elevated creatine kinase to 5000. The hospitalist notes that the patient is on both simvastatin and gemfibrozil. The patient's most recent serum LDL was at goal. Knowing that coadministration of simvastatin and gemfibrozil can increase the risk of rhabdomyolysis, how appropriate is it for the hospitalist to stop one of these medications without discussing it with the primary care physician? |
Stopping statin or fibrate (unrelated to the reason for admission) | A 60‐year‐old patient is admitted with an acute diarrheal illness. The hospitalist notes that the patient is on both simvastatin and gemfibrozil. The patient's most recent LDL was at goal. Knowing that coadministration of simvastatin and gemfibrozil can increase the risk of rhabdomyolysis, how appropriate is it for the hospitalist to stop one of these medications without discussing it with the primary care physician? |
Changing statin (related to the reason for admission) | A 65‐year‐old patient with a past medical history of hyperlipidemia on maximum‐dose simvastatin is admitted with a nonST‐elevation MI. The patient's cholesterol is noted to be above goal. Knowing that improving lipid management reduces mortality in cardiovascular disease, how appropriate is it for the hospitalist to replace simvastatin with atorvastatin without discussing it with the primary care physician? |
Changing statin (unrelated to the reason for admission) | A 65‐year‐old patient with a past medical history of a prior nonST‐elevation MI that was medically managed and hyperlipidemia on maximum‐dose simvastatin is admitted with pneumonia. Incidentally, the hospitalist notes that the patient's cholesterol has been above goal for the last 2 years. Knowing that improving lipid management reduces mortality in cardiovascular disease, how appropriate is it for the hospitalist to replace simvastatin with atorvastatin without discussing it with the primary care physician? |
The third section aimed to directly assess physicians' opinions. It consisted of questions regarding the appropriateness of inpatient management of conditions related to and unrelated to a patient's reason for admission.
Prior to administration, we conducted focus groups of hospitalists and PCPs to help hypothesize current physician perceptions on inpatient management, assess physician understanding of survey cases and questions, and to evaluate survey length.
Survey Administration
Between October 23, 2012 and November 10, 2012, 3 emails containing a link to the online survey were sent to all hospitalist and hospital‐based PCPs at the 3 study institutions. The BIDMC Committee on Clinical Investigations, to whom authority was ceded by the remaining 2 study institutions, certified this research protocol as exempt.
Statistical Analysis
We hypothesized that respondents as a whole would be more likely to rate an intervention as appropriate or very appropriate if it was related to the reason for admission, compared to unrelated, and that there would be no difference between PCPs and hospitalists.
We used 2 and Fisher exact tests (where applicable) to compare categorical variables, and a nonparametric median test for continuous variables. We used the Fisher exact test to compare the percent of respondents rating each intervention as appropriate or very appropriate by relatedness or unrelatedness to the reason for admission, and by PCP vs hospitalist. To derive the relative risk (RR) of rating each intervention as appropriate or very appropriate by PCPs compared to hospitalists, adjusting for potential confounders including years out of residency and sex, we used multivariable generalized estimating equation models, each with a Poisson distribution error term, a log link, and an exchangeable working correlation structure to account for dependency of observations arising from clustering at either the hospital or participant level, depending on the comparison: for comparisons within a given case, we controlled for clustering at the hospital level; for comparisons of cases in aggregate, owing to multiple responses from each participant, we controlled for clustering at the individual level.
Assuming a 50% response rate from both PCPs and hospitalists, and that 50% of PCPs would rate a given intervention as appropriate, we calculated that we would have 90% power to detect a 50% increase in the proportion of hospitalists rating an intervention as appropriate as compared to PCPs, using an of .05.
RESULTS
Demographics
One hundred sixty‐two out of 295 providers (55%) responded to the survey (Table 2). The response rate did not differ between hospitalists (70 out of 128; 55%) and PCPs (92 out of 167; 55%). Female respondents made up 58.7% of the PCP and 50.0% of the hospitalist groups (P=0.34). On average, PCPs were older (P<0.001) with a greater median number of years since graduation from residency (P<0.001). A greater percentage of hospitalists spent more than three‐quarters of their time clinically (42.9% vs 19.6%, P=0.009).
Total, n=162 (100.0%) | PCP, n=92 (6.8%) | Hospitalist, n=70 (43.2%) | P Valuea | |
---|---|---|---|---|
| ||||
Hospital, n (%) | ||||
BIDMC | 79 (48.8) | 48 (60.8) | 31 (39.2) | 0.115 |
BWH | 36 (22.2) | 15 (41.7) | 21 (58.3) | |
MGH | 47 (29.0) | 29 (61.7) | 18 (38.3) | |
Sex, n (%) | ||||
Male | 73 (45.1) | 38 (41.3) | 35 (50.0) | 0.339 |
Female | 89 (54.9) | 54 (58.7) | 35 (50.0) | |
Age interval, y, n (%) | ||||
2534 | 36 (22.2) | 9 (9.8) | 27 (38.6) | <0.001 |
3544 | 67 (41.4) | 34 (37.0) | 33 (47.1) | |
4554 | 35 (21.6) | 29 (31.5) | 6 (8.6) | |
5564 | 19 (11.7) | 16 (17.4) | 3 (4.3) | |
6574 | 5 (3.1) | 4 (4.4) | 1 (1.4) | |
Years out of residency, median (IQR) | 10 (417) | 15 (74) | 5 (211) | <0.001 |
Clinical FTE, n (%) | ||||
0.25 | 30 (18.6) | 22 (23.9) | 8 (11.4) | 0.009 |
0.260.50 | 41 (25.3) | 25 (27.2) | 16 (22.9) | |
0.510.75 | 43 (26.5) | 27 (29.4) | 16 (22.9) | |
>0.75 | 48 (29.6) | 18 (19.6) | 30 (42.9) | |
Worked as PCP?b | ||||
Yes | 6 (8.6) | |||
No | 64 (91.4) | |||
Worked as hospitalist? | ||||
Yes | 11 (12.0) | |||
No | 81 (88.0) | |||
AOR for admitted patients | ||||
Always | 16 (17.4) | |||
Mostly | 8 (8.7) | |||
Rarely | 7 (7.6) | |||
Never | 60 (65.2) |
Appropriateness of Inpatient Management Based on Admitting Diagnosis
For each of the 6 case pairings individually and in aggregate, respondents were significantly more likely to deem the intervention appropriate or very appropriate if it was related to the reason for admission, compared to those interventions unrelated to the reason for admission (in aggregate, 78.9% vs 38.8% respectively, P<0.001). For example, whereas 96.9% felt that the addition of aspirin in a patient admitted with acute myocardial infarction (MI) was appropriate, only 54.3% felt it appropriate to start aspirin in a patient with a prior history of MI admitted with cellulitis (P<0.001). Significant differences (all P values <0.001) were seen for all case pairs: starting spironolactone (68.1% when related to the reason for reason for admission vs 43.1% when unrelated to reason for admission); starting warfarin (62.3% vs 23.3%), stopping proton pump inhibitor (72.3% vs 42.8%), stopping statin or fibrate (90.6% vs 28.3%), and changing statin (83.0% vs 40.5%).
Appropriateness of Inpatient Management based on Primary Role
Table 3 compares the percent of PCPs and hospitalists rating each intervention as appropriate or very appropriate, by relatedness of the intervention to the reason for admission. In both unadjusted and adjusted comparisons for all cases in aggregate, PCPs were significantly more likely than hospitalists to rate the inpatient interventions as appropriate or very appropriate when the intervention was related to the reason for admission (83.4% of PCP responses vs 73.0% of hospitalist responses, P<0.001; RR: 1.2, 95% confidence interval [CI]: 1.11.3), unrelated to the reason for admission (44.7% vs 31.1%, P<0.001; RR: 1.5, 95% CI: 1.11.9), and overall (64.1% vs 52.1%, P<0.001; RR: 1.3, 95% CI: 1.11.4).
Relationship to Admission Diagnosis | PCP, n (%) | Hospitalist, n (%) | P Value | Adjusted RR | 95% CI |
---|---|---|---|---|---|
| |||||
Related | 453 (83.4) | 303 (73.0) | <0.001 | 1.2a | 1.11.3 |
Unrelated | 242 (44.7) | 129 (31.1) | <0.001 | 1.5a | 1.11.9 |
Overall | 695 (64.1) | 432 (52.1) | <0.001 | 1.3b | 1.11.4 |
Reasons for Inappropriate Designation
Among those respondents rating an intervention as inappropriate or very inappropriate, the 3 most common reasons selected as explanation for perceived inappropriateness from our predefined answer choices were: This medication will necessitate follow‐up testing/monitoring, for which the PCP will be responsible (chosen by physicians in 49.4% of instances); I am not confident that the hospitalist will have access to all of the medical history necessary to make this decision (35.7%); and Even if the hospitalist has all of the medical history and reviews it, the PCP should be involved in all decisions surrounding new medications (34.6%). The least common explanation chosen was I do not believe this is an appropriate pharmacologic intervention for this particular medical problem (6.5%). See Table 4 for a complete list of explanations, overall and stratified by PCP/hospitalist.
Predefined Reason for Inappropriateness | Total, n=583 (%) | PCP, n=318 (%) | Hospitalist, n=265 (%) | P Value |
---|---|---|---|---|
| ||||
This medication will necessitate follow‐up testing/monitoring, for which the PCP will be responsible. | 288 (49.4) | 151 (47.5) | 137 (51.7) | 0.32 |
I am not confident that the hospitalist will have access to all of the medical history necessary to make this decision. | 208 (35.7) | 98 (30.8) | 110 (41.5) | 0.009 |
Even if the hospitalist has all of the medical history and reviews it, the PCP should be involved in all decisions surrounding new medications. | 201 (34.5) | 125 (39.3) | 76 (28.7) | 0.009 |
I am not confident that the hospitalist will adequately review the medical history necessary to make this decision. | 184 (31.6) | 130 (40.9) | 54 (20.4) | <0.001 |
Even if the hospitalist has all of the medical history, I do not believe hospitalization is the right time to start this new medication | 106 (21.4) | 69 (21.7) | 56 (21.1) | 0.92 |
I am not confident that the hospitalist will appropriately discuss the risks and benefits of this new medication with the patient. | 106 (18.2) | 85 (26.7) | 21 (7.9) | <0.001 |
The benefit of this medication will be too remote to justify starting it in the acute setting. | 66 (11.3) | 40 (12.6) | 26 (9.8) | 0.36 |
I do not believe this is an appropriate pharmacologic intervention for this particular medical problem. | 38 (6.5) | 27 (8.5) | 11 (4.2) | 0.04 |
There were significant differences in the proportion of PCPs and hospitalists choosing several of the prespecified reasons for inappropriateness. Although hospitalists were more likely than PCPs to select I am not confident that the hospitalist will have access to all of the medical history necessary to make this decision (chosen by 41.5% of hospitalists vs 30.8% of PCPs, P=0.009), PCPs were more likely than hospitalists to select, I am not confident that the hospitalist will adequately review the medical history necessary to make this decision (chosen by 40.9% of PCPs vs 20.4% of hospitalists, P<0.001) and I am not confident that the hospitalist will appropriately discuss the risks and benefits of this new medication with the patient (26.7% of PCPs vs 9.8% of hospitalists, P<0.001).
Opinions on Current Management of Conditions Related and Unrelated to Admission
A minority of PCPs and hospitalists agreed or strongly agreed that hospitalists should play a larger role in the management of medical conditions unrelated to the reason for admission (28.1% of PCPs vs 34.8% of hospitalists; P=0.39).
DISCUSSION
In this survey‐based study of PCPs and hospitalists across 3 Boston‐area academic medical centers, we found that: (1) physicians were more likely to see inpatient interventions as appropriate when those interventions dealt with the reason for admission as compared to interventions unrelated to the reason for admission; and (2) PCPs were more likely than hospitalists to feel that inpatient interventions were appropriate, even when they targeted chronic conditions unrelated to the reason for admission. To our knowledge, this study represents the first investigation into the attitudes of PCPs and hospitalists regarding the inpatient management of conditions unrelated to the reason for admission.
That surveyed physicians, regardless of role, were less likely to report an intervention unrelated to the reason for hospitalization as appropriateeven those with likely mortality benefitsuggests that opportunities to affect meaningful change may be missed in a healthcare system that adheres to strict inpatient and outpatient roles. For several of the cases, a change in therapy could lead to benefit soon after implementation. For example, aldosterone antagonists reduce mortality as early as 1 month after initiation in select patients.[8] If a major goal of inpatient care is to reduce 30‐day mortality, it could be argued that hospitalists should more actively adjust congestive heart failure therapy in appropriate inpatients, even when this is not their admitting diagnosis.
For some conditions, CMS is already tracking hospital performance. Since 2003, hospitals have been required to document whether a patient with congestive heart failure (either acute or chronic and regardless of the relationship to admission) was prescribed an angiotensin‐converting enzyme (ACE) inhibitor or angiotensin receptor blocker (ARB) at the time of discharge.[7] CMS has determined that the proven benefits of ACE inhibitors and ARBs confer hospital accountability for their inclusion in appropriate patients, independent of the acuity of heart failure. There are many potential therapeutic maneuvers on which health systems (and their physicians) may be graded, and accepting the view that a hospitalization provides a window of opportunity for medical optimization may allow for more fruitful interventions and more patient‐centered care.
Despite the potential benefits of addressing chronic medical issues during hospitalization, there are important limitations on what can and/or should be done in the hospital setting. Hospitalizations are a time of fluctuating clinical status, which continues beyond discharge and is often accompanied by several medication changes.[9] In our study, more than 20% of those who believed that a medication intervention was inappropriate selected I do not believe hospitalization is the right time to start this new medication as one of their explanations. Although some medication interventions have been shown in randomized controlled trials to reduce short‐term mortality, the ability to generalize these findings to the average hospitalized patient with multiple comorbidities, concurrent medication changes, and rapidly fluctuating clinical status is limited. Furthermore, there are interventions most would agree should not be dealt with in the hospital (eg, screening colonoscopy) and encounters that may be too short to allow for change (eg, 24‐hour observation). These issues notwithstanding, the average 4‐day hospitalization likely provides an opportunity for monitored change that may currently be underutilized.
Our study suggests several additional explanations for physicians' current practice and opinions. Only 6.5% of respondents who answered that an intervention was inappropriate indicated as a justification that I do not believe this is an appropriate pharmacologic intervention for this particular medical problem. This suggests that the hesitancy has little to do with a lack of benefit but instead relates to systems issues (eg, access to all pertinent records and concerns regarding follow‐up testing) and perceived limitations to what a hospitalist should and should not do without actively involving the PCP. There are likely additional concerns that the medical record and/or patient histories do not fully outline the rationale for exclusion or inclusion of particular medications. Advances in information technology that enhance information exchange and enable streamlined communication may help to address these perceived barriers. However, an additional barrier may be trust, as PCPs appear more concerned that hospitalists will not review all the pertinent records or discuss risks and benefits before enacting important medication changes. Increased attempts at communication between hospitalists and outpatient providers may help to build trust and alleviate concerns regarding the loss of information that often occurs both on admission and at discharge.
We also noted that PCPs were more likely than hospitalists to feel that inpatient interventions were appropriate, even when targeting chronic conditions unrelated to the reason for admission. It may be that PCPs, with an increasing number of problems to address per outpatient visit,[10, 11] are more open to hospitalists managing any medical problems during their patients' admissions. At the same time, with increased acuity[12, 13, 14] and shortened length of stays,[15, 16] hospitalists have only a finite amount of time to ensure acute issues are managed, leaving potentially modifiable chronic conditions to the outpatient setting. These differences aside, a minority of both PCPs and hospitalists in our study were ready to embrace the idea of hospitalists playing a larger role in the management of conditions unrelated to the reason for hospitalization.
Even though our study benefits from its multisite design, there are a number of limitations. First, although we crafted our survey with input from general medicine focus groups, our survey instrument has not been validated. In addition, the cases are necessarily contrived and do not take into account the complexities of inpatient medicine. Furthermore, though our goal was to create paired cases that isolate a management decision as being simply based on whether it was related or unrelated to the reason for admission, it is possible that other factors, not captured by our survey, influenced the responses. For example, the benefits of aspirin as part of secondary prevention are not equal to the benefits in an acute MI.[17]
In an attempt to isolate the hospitalists' role in these management decisions, respondents were instructed to assume that the decisions were being made without discussing it with the primary care physician, but that the hospitalist would communicate the details of any hospitalization at the time of discharge. They were also instructed to assume that the hospitalist has access to the patient's outpatient electronic medical record. These assumptions were made to address concerns regarding the flow of information and communication, and to simulate the ideal system from a communication and information accessibility standpoint. Had these assumptions not been placed, the responses may have differed. It is likely that PCPs and hospitalists practicing in systems without shared, accessible inpatient/outpatient medical records would be even more reluctant to enact medication changes unrelated to the reason for admission.
Along the same lines, our physician cohort consisted of several metropolitan academic physician groups, in which hospitalists have had a presence for almost 20 years. As a result, our findings may not be generalizable to other academic hospitals, community‐based hospitalist programs, or nonhospital‐based PCP practices. Finally, we do not know whether survey nonresponders differed from responders in ways that could have meaningfully affected our results.
In conclusion, our findings suggest that both PCPs and hospitalists see the management of conditions unrelated to the reason for admission as less appropriate than the management of conditions related to the reason for admission. Our findings also suggest that PCPs may be more open to this practice when compared to hospitalists. Failure to capitalize on opportunities for meaningful medical interventions, independent of patient location, suggests a possible lack of patient centeredness in the current partnership between PCPs and hospitalists. Further studies should examine existing barriers and investigate interventions designed to address those barriers, in an effort to improve both quality of care and the degree of patient‐centeredness in our current healthcare system.
Disclosures: Dr. Herzig is supported by a grant from the National Institute on Aging (K23 AG042459). Dr. Herzig had full access to all of the data in the study and takes responsibility for the integrity of the data and the accuracy of the data analysis. Author contributions: study concept and design, Breu, Allen‐Dicker, Mueller, Herzig; acquisition of data, Breu, Allen‐Dicker, Mueller, Palamara, Herzig; analysis and interpretation of data, Breu, Allen‐Dicker, Hinami, Herzig; drafting of the manuscript, Breu; critical revision of the manuscript for important intellectual content, Breu, Allen‐Dicker, Mueller, Palamara, Hinami, Herzig; statistical analysis, Allen‐Dicker, Hinami, Herzig; study supervision, Breu, Herzig. This study was presented as a poster at the Society of Hospital Medicine National Meeting, Washington, DC, May 17, 2013.
- Growth in the care of older patients by hospitalists in the United States. N Engl J Med. 2009;360(11):1102–1112. , , , .
- The emerging role of “hospitalists” in the American health care system. N Engl J Med. 1996;335(7):514–517. , .
- An introduction to the hospitalist model. Ann Intern Med. 1999;130(4 pt 2):338–342. .
- Hospital readmission as an accountability measure. JAMA. 2011;305(5):504–505. , .
- A national strategy to put accountable care into practice. Health Aff (Millwood). 2010;29(5):982–990. , , , , .
- Keeping score under a global payment system. N Engl J Med. 2012;366(5):393–395. .
- Reporting Hospital Quality Data for Annual Payment Update. Available at: http://www.cms.gov/Medicare/Quality‐Initiatives‐Patient‐Assessment‐Instruments/HospitalQualityInits/Downloads/HospitalRHQDAPU200808. Accessed December 18, 2013.
- Eplerenone in patients with systolic heart failure and mild symptoms. N Engl J Med. 2011;364(1):11–21. , , , et al.
- How are drug regimen changes during hospitalisation handled after discharge: a cohort study. BMJ Open. 2012;2(6):e001461. , , , , , .
- Primary care visit duration and quality: does good care take longer? Arch Intern Med. 2009;169(20):1866–1872. , , .
- The increasing number of clinical items addressed during the time of adult primary care visits. J Gen Intern Med. 2008;23(12):2058–2065. , , ,
- Multiple chronic conditions among adults aged 45 and over: trends over the past 10 years. NCHS Data Brief. 2012;(100):1–8. , , .
- Prevalence of multiple chronic conditions in the United States' Medicare population. Health Qual Life Outcomes. 2009;7(1):82. , , .
- Multiple chronic conditions: prevalence, health consequences, and implications for quality, care management, and costs. J Gen Intern Med. 2007;22(suppl 3):391–395. , , , et al.
- Associations between reduced hospital length of stay and 30‐day readmission rate and mortality: 14‐year experience in 129 Veterans Affairs hospitals. Ann Intern Med. 2012;157(12):837–845. , , , et al.
- Trends in length of stay and short‐term outcomes among Medicare patients hospitalized for heart failure, 1993–2006. JAMA. 2010;303(21):2141–2147. , , , et al.
- Antithrombotic Trialists' Collaboration. Collaborative meta‐analysis of randomised trials of antiplatelet therapy for prevention of death, myocardial infarction, and stroke in high risk patients. BMJ. 2002;324(7329):71–86.
- Growth in the care of older patients by hospitalists in the United States. N Engl J Med. 2009;360(11):1102–1112. , , , .
- The emerging role of “hospitalists” in the American health care system. N Engl J Med. 1996;335(7):514–517. , .
- An introduction to the hospitalist model. Ann Intern Med. 1999;130(4 pt 2):338–342. .
- Hospital readmission as an accountability measure. JAMA. 2011;305(5):504–505. , .
- A national strategy to put accountable care into practice. Health Aff (Millwood). 2010;29(5):982–990. , , , , .
- Keeping score under a global payment system. N Engl J Med. 2012;366(5):393–395. .
- Reporting Hospital Quality Data for Annual Payment Update. Available at: http://www.cms.gov/Medicare/Quality‐Initiatives‐Patient‐Assessment‐Instruments/HospitalQualityInits/Downloads/HospitalRHQDAPU200808. Accessed December 18, 2013.
- Eplerenone in patients with systolic heart failure and mild symptoms. N Engl J Med. 2011;364(1):11–21. , , , et al.
- How are drug regimen changes during hospitalisation handled after discharge: a cohort study. BMJ Open. 2012;2(6):e001461. , , , , , .
- Primary care visit duration and quality: does good care take longer? Arch Intern Med. 2009;169(20):1866–1872. , , .
- The increasing number of clinical items addressed during the time of adult primary care visits. J Gen Intern Med. 2008;23(12):2058–2065. , , ,
- Multiple chronic conditions among adults aged 45 and over: trends over the past 10 years. NCHS Data Brief. 2012;(100):1–8. , , .
- Prevalence of multiple chronic conditions in the United States' Medicare population. Health Qual Life Outcomes. 2009;7(1):82. , , .
- Multiple chronic conditions: prevalence, health consequences, and implications for quality, care management, and costs. J Gen Intern Med. 2007;22(suppl 3):391–395. , , , et al.
- Associations between reduced hospital length of stay and 30‐day readmission rate and mortality: 14‐year experience in 129 Veterans Affairs hospitals. Ann Intern Med. 2012;157(12):837–845. , , , et al.
- Trends in length of stay and short‐term outcomes among Medicare patients hospitalized for heart failure, 1993–2006. JAMA. 2010;303(21):2141–2147. , , , et al.
- Antithrombotic Trialists' Collaboration. Collaborative meta‐analysis of randomised trials of antiplatelet therapy for prevention of death, myocardial infarction, and stroke in high risk patients. BMJ. 2002;324(7329):71–86.
© 2014 Society of Hospital Medicine
Opioids and Opioid‐Related Adverse Events
Recent reports have drawn attention to the high and increasing rates of opioid prescribing and overdose‐related deaths in the United States.[1, 2, 3, 4, 5, 6, 7, 8, 9] These studies have focused on community‐based and emergency department prescribing, leaving prescribing practices in the inpatient setting unexamined. Given that pain is a frequent complaint in hospitalized patients, and that the Joint Commission mandates assessing pain as a vital sign, hospitalization is potentially a time of heightened use of such medications and could significantly contribute to nosocomial complications and subsequent outpatient use.[10] Variation in prescribing practices, unrelated to patient characteristics, could be a marker of inappropriate prescribing practices and poor quality of care.
Using a large, nationally representative cohort of admissions from July 2009 to June 2010, we sought to determine patterns and predictors of opioid utilization in nonsurgical admissions to US medical centers, hospital variation in use, and the association between hospital‐level use and the risk of opioid‐related adverse events. We hypothesized that hospitals with higher rates of opioid use would have an increased risk of an opioid‐related adverse event per patient exposed.
METHODS
Setting and Patients
We conducted a retrospective cohort study using data from 286 US nonfederal acute‐care facilities contributing to the database maintained by Premier (Premier Healthcare Solutions, Inc., Charlotte, NC). This database, created to measure healthcare utilization and quality of care, is drawn from voluntarily participating hospitals and contains data on approximately 1 in every 4 discharges nationwide.[11] Participating hospitals are similar in geographic distribution and metropolitan (urban/rural) status to hospitals nationwide, although large, nonteaching hospitals are slightly overrepresented in Premier. The database contains patient demographics, International Classification of Diseases, Ninth Revision, Clinical Modification (ICD‐9‐CM) codes, hospital demographics, and a date‐stamped log of all charges during the course of each hospitalization, including diagnostic tests, therapeutic treatments, and medications with dose and route of administration. The study was approved by the institutional review board at Beth Israel Deaconess Medical Center and granted a waiver of informed consent.
We studied a cohort of all adult nonsurgical admissions to participating hospitals from July 1, 2009, through June 30, 2010. We chose to study nonsurgical admissions, as patients undergoing surgical procedures have a clear indication for, and almost always receive, opioid pain medications. We defined a nonsurgical admission as an admission in which there were no charges for operating‐room procedures (including labor and delivery) and the attending of record was not a surgeon. We excluded admissions with unknown gender, since this is a key demographic variable, and admissions with a length of stay greater than 365 days, as these admissions are not representative of the typical admission to an acute‐care hospital. At the hospital level, we excluded hospitals contributing <100 admissions owing to resultant lack of precision in corresponding hospital prescribing rates, and hospitals that did not prescribe the full range of opioid medications (these hospitals had charges for codeine only), as these facilities seemed likely to have unusual limitations on prescribing or incomplete data capture.
Opioid Exposure
We defined opioid exposure as presence of 1 charge for an opioid medication during the admission. Opioid medications included morphine, hydrocodone, hydromorphone, oxycodone, fentanyl, meperidine, methadone, codeine, tramadol, buprenorphine, levorphanol, oxymorphone, pentazocine, propoxyphene, tapentadol, butorphanol, dezocine, and nalbuphine. We grouped the last 9 into an other category owing to infrequent use and/or differing characteristics from the main opioid drug types, such as synthetic, semisynthetic, and partial agonist qualities.
Severe Opioid‐Related Adverse Events
We defined severe opioid‐related adverse events as either naloxone exposure or an opioid‐related adverse drug event diagnosis code. Naloxone use in an adult patient exposed to opioids is one of the Institute for Healthcare Improvement's Trigger Tools for identifying adverse drug events[12] and previously has been demonstrated to have high positive predictive value for a confirmed adverse drug event.[13] We defined naloxone exposure as presence of 1 charge for naloxone. We excluded charges on hospital day 1 to focus on nosocomial events. We defined opioid‐related adverse drug events using ICD‐9‐CM diagnosis codes for poisoning by opioids (overdose, wrong substance given, or taken in error; ICD‐9‐CM 965.02, 965.09, E850.1, E850.2) and drugs causing adverse effects in therapeutic use (ICD‐9‐CM E935.1, E935.2), as specified in prior analyses by the Agency for Healthcare Research and Quality (AHRQ).[14, 15] To avoid capturing adverse events associated with outpatient use, we required the ICD‐9‐CM code to be qualified as not present on admission using the present on admission indicator required by the Centers for Medicare and Medicaid Services for all discharge diagnosis codes since 2008.[16]
Covariates of Interest
We were interested in the relationship between both patient and hospital characteristics and opioid exposure. Patient characteristics of interest included (1) demographic variables, such as age, sex, race (self‐reported by patients at the time of admission), marital status, and payer; (2) whether or not the patient spent any time in the intensive care unit (ICU); (3) comorbidities, identified via ICD‐9‐CM secondary diagnosis codes and diagnosis‐related groups using Healthcare Cost and Utilization Project Comorbidity Software, version 3.7, based on the work of Elixhauser et al[17, 18]; (4) primary ICD‐9‐CM discharge diagnosis groupings, selected based on hypothesized associations with receipt of opioids, and based on the Clinical Classifications Software (CCS)a diagnosis and procedure categorization scheme maintained by the AHRQ, and defined in the Appendix[19]; (5) and nonoperating‐room‐based procedures potentially necessitating opioids during the admission, selected from the 50 most common ICD‐9‐CM procedure codes in our cohort and grouped as cardiovascular procedures (catheterization and insertion of vascular stents), gastrointestinal procedures (upper and lower endoscopy), and mechanical ventilation, further defined in the Appendix. Hospital characteristics of interest included number of beds, population served (urban vs rural), teaching status, and US census region (Northeast, Midwest, South, West).
Statistical Analysis
We calculated the percent of admissions with exposure to any opioid and the percent exposed to each opioid, along with the total number of different opioid medications used during each admission. We also calculated the percent of admissions with parenteral administration and the percent of admissions with oral administration, among those exposed to the individual categories, and in aggregate. Because medications after discharge were unavailable in Premier's dataset, we report the percent of patients with a charge for opioids on the day of discharge.
We determined the daily dose of an opioid by taking the sum of the doses for that opioid charged on a given day. The average daily dose of an opioid was determined by taking the sum of the daily doses and dividing by the number of days on which 1 dose was charged. To facilitate comparison, all opioids, with the exception of those for which standard equivalences are unavailable (tramadol, other opioid category, oral fentanyl, epidural route for all), were converted to oral morphine equivalents using a standard equivalence conversion table.[20, 21] We excluded from our dosage calculations those charges for which standard morphine equivalence was unavailable, or for which dosage was missing. We also excluded from our dosage calculations any dose that was >3 standard deviations (SD) above the mean dose for that opioid, as such extreme values seemed physiologically implausible and more likely to be a data entry error that could lead to significant overestimation of the mean for that opioid.
All multivariable models used a generalized estimating equation (GEE) via the genmod procedure in SAS, with a Poisson distribution error term and a log link, controlling for repeated patient admissions with an autoregressive correlation structure.
To identify independent predictors of opioid receipt, we used a GEE model of opioid receipt where all patient and hospital characteristics listed in Table 1 were included as independent variables.
Patient characteristics, N=1,139,419 | N | % |
---|---|---|
| ||
Age group, y | ||
1824 | 37,464 | 3 |
2534 | 66,541 | 6 |
3544 | 102,701 | 9 |
4554 | 174,830 | 15 |
5564 | 192,570 | 17 |
6574 | 196,407 | 17 |
75+ | 368,906 | 32 |
Sex | ||
Male | 527,062 | 46 |
Female | 612,357 | 54 |
Race | ||
White | 711,993 | 62 |
Black | 176,993 | 16 |
Hispanic | 54,406 | 5 |
Other | 196,027 | 17 |
Marital status | ||
Married | 427,648 | 38 |
Single | 586,343 | 51 |
Unknown/other | 125,428 | 11 |
Primary insurance | ||
Private/commercial | 269,725 | 24 |
Medicare traditional | 502,301 | 44 |
Medicare managed care | 126,344 | 11 |
Medicaid | 125,025 | 11 |
Self‐pay/other | 116,024 | 10 |
ICU care | ||
No | 1,023,027 | 90 |
Yes | 116,392 | 10 |
Comorbidities | ||
AIDS | 5724 | 1 |
Alcohol abuse | 79,633 | 7 |
Deficiency anemias | 213,437 | 19 |
RA/collagen vascular disease | 35,210 | 3 |
Chronic blood‐loss anemia | 10,860 | 1 |
CHF | 190,085 | 17 |
Chronic pulmonary disease | 285,954 | 25 |
Coagulopathy | 48,513 | 4 |
Depression | 145,553 | 13 |
DM without chronic complications | 270,087 | 24 |
DM with chronic complications | 70,732 | 6 |
Drug abuse | 66,886 | 6 |
Hypertension | 696,299 | 61 |
Hypothyroidism | 146,136 | 13 |
Liver disease | 38,130 | 3 |
Lymphoma | 14,032 | 1 |
Fluid and electrolyte disorders | 326,576 | 29 |
Metastatic cancer | 33,435 | 3 |
Other neurological disorders | 124,195 | 11 |
Obesity | 118,915 | 10 |
Paralysis | 38,584 | 3 |
PVD | 77,334 | 7 |
Psychoses | 101,856 | 9 |
Pulmonary circulation disease | 52,106 | 5 |
Renal failure | 175,398 | 15 |
Solid tumor without metastasis | 29,594 | 3 |
Peptic ulcer disease excluding bleeding | 536 | 0 |
Valvular disease | 86,616 | 8 |
Weight loss | 45,132 | 4 |
Primary discharge diagnoses | ||
Cancer | 19,168 | 2 |
Musculoskeletal injuries | 16,798 | 1 |
Pain‐related diagnosesb | 101,533 | 9 |
Alcohol‐related disorders | 16,777 | 1 |
Substance‐related disorders | 13,697 | 1 |
Psychiatric disorders | 41,153 | 4 |
Mood disorders | 28,761 | 3 |
Schizophrenia and other psychotic disorders | 12,392 | 1 |
Procedures | ||
Cardiovascular procedures | 59,901 | 5 |
GI procedures | 31,224 | 3 |
Mechanical ventilation | 7853 | 1 |
Hospital characteristics, N=286 | ||
Number of beds | ||
<200 | 103 | 36 |
201300 | 63 | 22 |
301500 | 81 | 28 |
>500 | 39 | 14 |
Population served | ||
Urban | 225 | 79 |
Rural | 61 | 21 |
Teaching status | ||
Nonteaching | 207 | 72 |
Teaching | 79 | 28 |
US Census region | ||
Northeast | 47 | 16 |
Midwest | 63 | 22 |
South | 115 | 40 |
West | 61 | 21 |
To assess hospital variation in opioid prescribing after adjusting for patient characteristics, we used a GEE model of opioid receipt, controlling for all patient characteristics listed in Table 1. We then took the mean of the predicted probabilities of opioid receipt for the patients within each hospital in our cohort to derive the hospital prescribing rate adjusted for patient characteristics. We report the mean, SD, and range of the prescribing rates for the hospitals in our cohort before and after adjustment for patient characteristics.
To assess whether patients admitted to hospitals with higher rates of opioid prescribing have higher relative risk of severe opioid‐related adverse events, we stratified hospitals into opioid‐prescribing rate quartiles and compared the rates of opioid‐related adverse eventsboth overall and among opioid exposedbetween quartiles. To adjust for patient characteristics, we used a GEE model in which severe opioid‐related adverse event (yes/no) was the dependent variable and hospital‐prescribing rate quartile and all patient characteristics in Table 1 were independent variables. We also performed a sensitivity analysis in which we assessed the association between hospital prescribing‐rate quartile and the individual components of our composite outcome. Our results were qualitatively unchanged using this approach, and only the results of our main analysis are presented.
All analyses were carried out using SAS software, version 9.2 (SAS Institute Inc., Cary, NC).
RESULTS
Patient Admission Characteristics
There were 3,190,934 adult admissions to 300 acute‐care hospitals during our study period. After excluding admissions with a length of stay >365 days (n=25), missing patient sex (n=17), and charges for operating‐room procedures or a surgical attending of record (n=2,018,553), 1,172,339 admissions were available for analysis. There were 12 hospitals with incomplete opioid‐prescribing data (n=32,794) and 2 hospitals that contributed <100 admissions each (n=126), leaving 1,139,419 admissions in 286 hospitals in our analytic cohort. The median age of the cohort was 64 years (interquartile range, 4979 years), and 527,062 (46%) were men. Table 1 shows the characteristics of the admissions in the cohort.
Rate, Route, and Dose of Opioid Exposures
Overall, there were 576,373 (51%) admissions with charges for opioid medications. Among those exposed, 244,760 (43%) had charges for multiple opioids during the admission; 172,090 (30%) had charges for 2 different opioids; and 72,670 (13%) had charges for 3 different opioids. Table 2 shows the percent exposed to each opioid, the percent of exposed with parenteral and oral routes of administration, and the mean daily dose received in oral morphine equivalents.
Exposed | Parenteral Administration | Oral Administration | Dose Received, in Oral Morphine Equivalents | |||||
---|---|---|---|---|---|---|---|---|
N | %a | N | %b | N | %b | Mean | SDc | |
| ||||||||
All opioids | 576,373 | 51 | 378,771 | 66 | 371,796 | 65 | 68 | 185 |
Morphine | 224,811 | 20 | 209,040 | 93 | 21,645 | 10 | 40 | 121 |
Hydrocodone | 162,558 | 14 | 0 | 0 | 160,941 | 99 | 14 | 12 |
Hydromorphone | 146,236 | 13 | 137,936 | 94 | 16,052 | 11 | 113 | 274 |
Oxycodone | 126,733 | 11 | 0 | 0 | 125,033 | 99 | 26 | 37 |
Fentanyl | 105,052 | 9 | 103,113 | 98 | 641 | 1 | 64 | 75 |
Tramadol | 35,570 | 3 | 0 | 0 | 35,570 | 100 | ||
Meperidine | 24,850 | 2 | 24,398 | 98 | 515 | 2 | 36 | 34 |
Methadone | 15,302 | 1 | 370 | 2 | 14,781 | 97 | 337 | 384 |
Codeine | 22,818 | 2 | 178 | 1 | 22,183 | 97 | 9 | 15 |
Other | 45,469 | 4 | 5821 | 13 | 39,618 | 87 |
Among the medications/routes for which conversion to morphine equivalents was possible, dosage was missing in 39,728 out of 2,294,673 opioid charges (2%). The average daily dose received in oral morphine equivalents was 68 mg. A total dose of 50 mg per day was received in 39% of exposed, and a total dose of 100 mg per day was received in 23% of exposed. Among those exposed, 52% (26% of overall admissions) had charges for opioids on the day of discharge.
Rates of Opioid Use by Patient and Hospital Characteristics
Table 3 reports the association between admission characteristics and opioid use. Use was highest in patients between the ages of 25 and 54 years. Although use declined with age, 44% of admissions age 65 years had charges for opioid medication. After adjustment for patient demographics, comorbidities, and hospital characteristics, opioid use was more common in females than males, those age 2554 years compared with those older and younger, those of Caucasian race compared with non‐Caucasian race, and those with Medicare or Medicaid primary insurance. Among the primary discharge diagnoses, patients with musculoskeletal injuries, various specific and nonspecific pain‐related diagnoses, and cancer were significantly more likely to receive opioids than patients without these diagnoses, whereas patients with alcohol‐related disorders and psychiatric disorders were significantly less likely to receive opioids than patients without these diagnoses. Patients admitted to hospitals in the Midwest, South, and West were significantly more likely to receive opioid medications than patients in the Northeast.
Exposed, N=576,373 | Unexposed, N=563,046 | % Exposed | Adjusted RRa | 95% CI | |
---|---|---|---|---|---|
| |||||
Patient characteristics | |||||
Age group, y | |||||
1824 | 17,360 | 20,104 | 46 | (ref) | |
2534 | 37,793 | 28,748 | 57 | 1.17 | 1.16‐1.19 |
3544 | 60,712 | 41,989 | 59 | 1.16 | 1.15‐1.17 |
4554 | 103,798 | 71,032 | 59 | 1.11 | 1.09‐1.12 |
5564 | 108,256 | 84,314 | 56 | 1.00 | 0.98‐1.01 |
6574 | 98,110 | 98,297 | 50 | 0.84 | 0.83‐0.85 |
75+ | 150,344 | 218,562 | 41 | 0.71 | 0.70‐0.72 |
Sex | |||||
Male | 255,315 | 271,747 | 48 | (ref) | |
Female | 321,058 | 291,299 | 52 | 1.11 | 1.10‐1.11 |
Race | |||||
White | 365,107 | 346,886 | 51 | (ref) | |
Black | 92,013 | 84,980 | 52 | 0.93 | 0.92‐0.93 |
Hispanic | 27,592 | 26,814 | 51 | 0.94 | 0.93‐0.94 |
Other | 91,661 | 104,366 | 47 | 0.93 | 0.92‐0.93 |
Marital status | |||||
Married | 222,912 | 204,736 | 52 | (ref) | |
Single | 297,742 | 288,601 | 51 | 1.00 | 0.99‐1.01 |
Unknown/other | 55,719 | 69,709 | 44 | 0.94 | 0.93‐0.95 |
Primary insurance | |||||
Private/commercial | 143,954 | 125,771 | 53 | (ref) | |
Medicare traditional | 236,114 | 266,187 | 47 | 1.10 | 1.09‐1.10 |
Medicare managed care | 59,104 | 67,240 | 47 | 1.11 | 1.11‐1.12 |
Medicaid | 73,583 | 51,442 | 59 | 1.13 | 1.12‐1.13 |
Self‐pay/other | 63,618 | 52,406 | 55 | 1.03 | 1.02‐1.04 |
ICU care | |||||
No | 510,654 | 512,373 | 50 | (ref) | |
Yes | 65,719 | 50,673 | 56 | 1.02 | 1.01‐1.03 |
Comorbiditiesb | |||||
AIDS | 3655 | 2069 | 64 | 1.09 | 1.07‐1.12 |
Alcohol abuse | 35,112 | 44,521 | 44 | 0.92 | 0.91‐0.93 |
Deficiency anemias | 115,842 | 97,595 | 54 | 1.08 | 1.08‐1.09 |
RA/collagen vascular disease | 22,519 | 12,691 | 64 | 1.22 | 1.21‐1.23 |
Chronic blood‐loss anemia | 6444 | 4416 | 59 | 1.04 | 1.02‐1.05 |
CHF | 88,895 | 101,190 | 47 | 0.99 | 0.98‐0.99 |
Chronic pulmonary disease | 153,667 | 132,287 | 54 | 1.08 | 1.08‐1.08 |
Coagulopathy | 25,802 | 22,711 | 53 | 1.03 | 1.02‐1.04 |
Depression | 83,051 | 62,502 | 57 | 1.08 | 1.08‐1.09 |
DM without chronic complications | 136,184 | 133,903 | 50 | 0.99 | 0.99‐0.99 |
DM with chronic complications | 38,696 | 32,036 | 55 | 1.04 | 1.03‐1.05 |
Drug abuse | 37,202 | 29,684 | 56 | 1.14 | 1.13‐1.15 |
Hypertension | 344,718 | 351,581 | 50 | 0.98 | 0.97‐0.98 |
Hypothyroidism | 70,786 | 75,350 | 48 | 0.99 | 0.99‐0.99 |
Liver disease | 24,067 | 14,063 | 63 | 1.15 | 1.14‐1.16 |
Lymphoma | 7727 | 6305 | 55 | 1.16 | 1.14‐1.17 |
Fluid and electrolyte disorders | 168,814 | 157,762 | 52 | 1.04 | 1.03‐1.04 |
Metastatic cancer | 23,920 | 9515 | 72 | 1.40 | 1.39‐1.42 |
Other neurological disorders | 51,091 | 73,104 | 41 | 0.87 | 0.86‐0.87 |
Obesity | 69,584 | 49,331 | 59 | 1.05 | 1.04‐1.05 |
Paralysis | 17,497 | 21,087 | 45 | 0.97 | 0.96‐0.98 |
PVD | 42,176 | 35,158 | 55 | 1.11 | 1.11‐1.12 |
Psychoses | 38,638 | 63,218 | 38 | 0.91 | 0.90‐0.92 |
Pulmonary circulation disease | 26,656 | 25,450 | 51 | 1.05 | 1.04‐1.06 |
Renal failure | 86,565 | 88,833 | 49 | 1.01 | 1.01‐1.02 |
Solid tumor without metastasis | 16,258 | 13,336 | 55 | 1.14 | 1.13‐1.15 |
Peptic ulcer disease excluding bleeding | 376 | 160 | 70 | 1.12 | 1.07‐1.18 |
Valvular disease | 38,396 | 48,220 | 44 | 0.93 | 0.92‐0.94 |
Weight loss | 25,724 | 19,408 | 57 | 1.09 | 1.08‐1.10 |
Primary discharge diagnosesb | |||||
Cancer | 13,986 | 5182 | 73 | 1.20 | 1.19‐1.21 |
Musculoskeletal injuries | 14,638 | 2160 | 87 | 2.02 | 2.002.04 |
Pain‐related diagnosesc | 64,656 | 36,877 | 64 | 1.20 | 1.20‐1.21 |
Alcohol‐related disorders | 3425 | 13,352 | 20 | 0.46 | 0.44‐0.47 |
Substance‐related disorders | 8680 | 5017 | 63 | 1.03 | 1.01‐1.04 |
Psychiatric disorders | 7253 | 33,900 | 18 | 0.37 | 0.36‐0.38 |
Mood disorders | 5943 | 22,818 | 21 | ||
Schizophrenia and other psychotic disorders | 1310 | 11,082 | 11 | ||
Proceduresb | |||||
Cardiovascular procedures | 50,997 | 8904 | 85 | 1.80 | 1.79‐1.81 |
GI procedures | 27,206 | 4018 | 87 | 1.70 | 1.69‐1.71 |
Mechanical ventilation | 5341 | 2512 | 68 | 1.37 | 1.34‐1.39 |
Hospital characteristics | |||||
Number of beds | |||||
<200 | 100,900 | 88,439 | 53 | (ref) | |
201300 | 104,213 | 99,995 | 51 | 0.95 | 0.95‐0.96 |
301500 | 215,340 | 209,104 | 51 | 0.94 | 0.94‐0.95 |
>500 | 155,920 | 165,508 | 49 | 0.96 | 0.95‐0.96 |
Population served | |||||
Urban | 511,727 | 506,803 | 50 | (ref) | |
Rural | 64,646 | 56,243 | 53 | 0.98 | 0.97‐0.99 |
Teaching status | |||||
Nonteaching | 366,623 | 343,581 | 52 | (ref) | |
Teaching | 209,750 | 219,465 | 49 | 1.00 | 0.99‐1.01 |
US Census region | |||||
Northeast | 99,377 | 149,446 | 40 | (ref) | |
Midwest | 123,194 | 120,322 | 51 | 1.26 | (1.25‐1.27) |
South | 251,624 | 213,029 | 54 | 1.33 | (1.33‐1.34) |
West | 102,178 | 80,249 | 56 | 1.37 | (1.36‐1.38) |
Variation in Opioid Prescribing
Figure 1 shows the histograms of hospital opioid prescribing rate for the 286 hospitals in our cohort (A) before and (B) after adjustment for patient characteristics. The observed rates ranged from 5% in the lowest‐prescribing hospital to 72% in the highest‐prescribing hospital, with a mean (SD) of 51% (10%). After adjusting for patient characteristics, the adjusted opioid‐prescribing rates ranged from 33% to 64%, with a mean (SD) of 50% (4%).
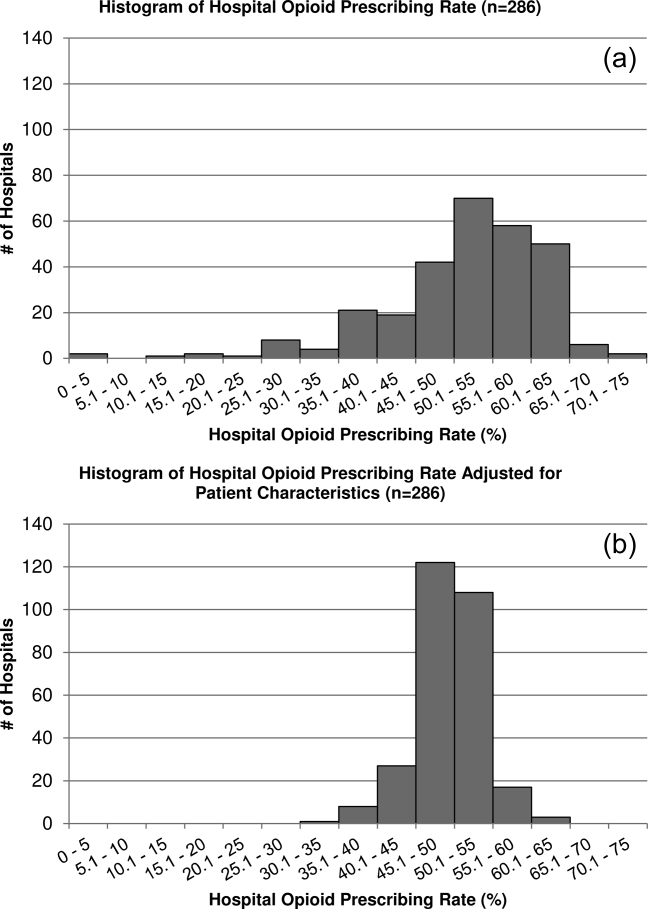
Severe Opioid‐Related Adverse Events
Among admissions with opioid exposure (n=576,373), naloxone use occurred in 2345 (0.41%) and opioid‐related adverse drug events in 1174 (0.20%), for a total of 3441 (0.60%) severe opioid‐related adverse events (some patients experienced both). Table 4 reports the opioid exposure and severe opioid‐related adverse‐event rates within hospital opioid‐prescribing rate quartiles, along with the adjusted association between the hospital opioid‐prescribing rate quartile and severe opioid‐related adverse events. After adjusting for patient characteristics, the relative risk of a severe opioid‐related adverse event was significantly greater in hospitals with higher opioid‐prescribing rates, both overall and among opioid exposed.
Quartile | No. of Patients | Opioid Exposed, n (%) | Opioid‐Related Adverse Events, n (%) | Adjusted RR in All Patients, RR (95% CI), N=1,139,419a | Adjusted RR in Opioid Exposed, RR (95% CI), N=576,373a |
---|---|---|---|---|---|
| |||||
1 | 349,747 | 132,824 (38) | 719 (0.21) | (ref) | (ref) |
2 | 266,652 | 134,590 (50) | 729 (0.27) | 1.31 (1.17‐1.45) | 1.07 (0.96‐1.18) |
3 | 251,042 | 139,770 (56) | 922 (0.37) | 1.72 (1.56‐1.90) | 1.31 (1.19‐1.44) |
4 | 271,978 | 169,189 (62) | 1071 (0.39) | 1.73 (1.57‐1.90) | 1.23 (1.12‐1.35) |
DISCUSSION
In this analysis of a large cohort of hospitalized nonsurgical patients, we found that more than half of all patients received opioids, with 43% of those exposed receiving multiple opioids during their admission and 52% receiving opioids on the day of discharge. Considerable hospital variation in opioid use was evident, and this was not fully explained by patient characteristics. Severe opioid‐related adverse events occurred more frequently at hospitals with higher opioid‐prescribing rates, and the relative risk of a severe adverse event per patient prescribed opioids was also higher in these hospitals. To our knowledge, this is the first study to describe the scope of opioid utilization and the relationship between utilization and severe opioid‐related adverse events in a sample of nonsurgical patients in US acute‐care facilities.
Our use of naloxone charges and opioid‐specific ICD‐9‐CM coding to define an opioid‐related adverse event was intended to capture only the most severe opioid‐related adverse events. We chose to focus on these events in our analysis to maximize the specificity of our outcome definition and thereby minimize confounding in our observed associations. The rate of less‐severe opioid‐related adverse events, such as nausea, constipation, and pruritis, is likely much higher and not captured in our outcome definition. Prior analyses have found variable rates of opioid‐related adverse events of approximately 1.8% to 13.6% of exposed patients.[22, 23, 24] However, these analyses focused on surgical patients and included less‐severe events. To our knowledge, ours is the first analysis of severe opioid‐related adverse events in nonsurgical patients.
Our finding that severe opioid‐related adverse events increase as opioid prescribing increases is consistent with that which has been demonstrated in the community setting, where rates of opioid‐related adverse events and mortality are higher in communities with higher levels of opioid prescribing.[2, 8, 25] This finding is expected, as greater use of a class of medications with known side effects would be expected to result in a higher overall rate of adverse events. More concerning, however, is the fact that this relationship persists when focusing exclusively on opioid‐exposed patients. Among similar patients receiving opioids at different hospitals, those hospitalized in facilities with higher opioid‐prescribing rates have higher rates of severe opioid‐related adverse events. This suggests that hospitals that use opioids more frequently do not do so more safely. Rather, the increased overall prescribing rates are associated with heightened risk for a serious adverse event per patient exposed and may reflect unsafe prescribing practices.
Furthermore, our results demonstrate both regional and hospital variation in use of opioids not fully explained by patient characteristics, similar to that which has been demonstrated for other drugs and heathcare services.[26, 27, 28, 29, 30] The implications of these findings are limited by our lack of information on pain severity or prior outpatient treatment, and our resultant inability to evaluate the appropriateness of opioid use in this analysis. Additionally, although we controlled for a large number of patient and hospital characteristics, there could be other significant predictors of use not accounted for in our analysis. However, it seems unlikely that differential pain severity or patient characteristics between patients in different regions of the country could fully explain a 37% relative difference in prescribing between the lowest‐ and highest‐prescribing regions, after accounting for the 44 patient‐level variables in our models. Whereas variation in use unrelated to patient factors could represent inappropriate prescribing practices, it could also be a marker of uncertainty regarding what constitutes appropriate prescribing and high‐quality care in this realm. Although guidelines advocate for standard pain assessments and a step‐up approach to treatment,[31, 32, 33] the lack of objective measures of pain severity and lack of evidence‐based recommendations on the use of opioids for noncancer pain[34] will almost certainly lead to persistent variation in opioid prescribing despite guideline‐driven care.
Nonetheless, our findings suggest that opportunities exist to make opioid prescribing safer in hospitalized patients. Studies aimed at elucidating the source of regional and hospital variation are necessary. Additionally, efforts should focus on identifying patient and prescribing characteristics associated with heightened risk of opioid‐related adverse events. Prior studies have demonstrated that the risks of opioid medications increase with increasing age of the patient.[35, 36] Although opioid use in our cohort declined with age, 44% of admissions age 65 years had charges for opioid medications. Studies in outpatients have also demonstrated that the risks of opioid overdose and overdose‐related death increase with dose.[5, 7] One study demonstrated a 3.7‐fold increased risk of overdose at doses of 5099 mg/day in oral morphine equivalents, and an 8.9‐fold increased risk at doses of 100 mg/day, compared with doses of 20 mg/day.[7] The prevalence of high dose exposure observed in our cohort, coupled with the older age of hospitalized patients, suggests potential targets for promoting safer use in hospitalized patients through interventions such as computerized decision support and enhanced monitoring in those at highest risk.
Because medications after discharge were unavailable in our dataset, the percentage of patients given a prescription for opioid medication on discharge is unknown. However, given that opioids are often tapered rather than abruptly discontinued, our finding that 26% of hospitalized nonsurgical patients received opioids on the day of discharge suggests that a substantial proportion of patients may be discharged with a prescription for opioid medication. Given the possibility of coexistent outpatient opioid prescriptions, these findings draw attention to the importance of assuring development and streamlined accessibility of data from state prescription drug monitoring programs and suggest that increased attention should be paid to the role that inpatient opioid prescribing plays in the increased rates of chronic opioid use and overdose‐related deaths in the United States.
There are additional limitations to our analysis. First, although the database used for this analysis captures a large proportion of admissions to US acute‐care facilities and is similar in composition, it is possible that participating medical centers differ from nonparticipating medical centers in ways that could be associated with opioid prescribing. Additionally, although Premier performs extensive validation and correction processes to assure the quality of their data, there is still likely to be a small amount of random error in the database, which could particularly impact dosage calculations. The lack of preadmission medications in our database precluded identification of the proportion of patients newly started on opioid medications. Lastly, it is possible that the hospital prescribing‐rate quartile is associated with patient characteristics unaccounted for in our analysis, and, therefore, the possibility of residual confounding still exists.
In conclusion, the majority of hospitalized nonsurgical patients are exposed to opioid medications during the course of their hospitalizations, often at high doses. More than half of those exposed are still receiving these medications on the day of discharge. We found hospital and regional variation in opioid use that was not fully explained by patient characteristics, and higher levels of hospital use were associated with higher risk of severe opioid‐related adverse events in opioid‐exposed patients. Further research is necessary to investigate the appropriateness of opioid use in this patient population, the sources of variation in use, and the predictors of opioid‐related adverse events in hospitalized patients to allow development of interventions to make hospital use safer.
Disclosures
Disclosures: Dr. Herzig had full access to all of the data in the study and takes responsibility for the integrity of the data and the accuracy of the data analysis. Dr. Herzig was funded by grant no. K23AG042459 from the National Institute on Aging. Dr. Marcantonio was funded by grant nos. P01AG031720, R01AG030618, R03AG028189, and K24AG035075 from the National Institute on Aging. The funding organization had no involvement in any aspect of the study, including design, conduct, and reporting of the study. None of the authors have any conflicts of interest to disclose.
- A flood of opioids, a rising tide of deaths. N Engl J Med. 2010;363(21):1981–1985. .
- Increasing deaths from opioid analgesics in the United States. Pharmacoepidemiol Drug Saf. 2006;15(9):618–627. , , .
- Trends in opioid prescribing by race/ethnicity for patients seeking care in US emergency departments. JAMA. 2008;299(1):70–78. , , , .
- Trends in medical use and abuse of opioid analgesics. JAMA. 2000;283(13):1710–1714. , , , .
- Association between opioid prescribing patterns and opioid overdose‐related deaths. JAMA. 2011;305(13):1315–1321. , , , et al.
- Prescription opioid mortality trends in New York City, 1990–2006: examining the emergence of an epidemic. Drug Alcohol Depend. 2013;132(1‐2):53–62. , , , et al.
- Opioid prescriptions for chronic pain and overdose: a cohort study. Ann Intern Med. 2010;152(2):85–92. , , , et al.
- Relationship of opioid prescription sales and overdoses, North Carolina. Drug Alcohol Depend. 2013;132(1‐2):81–86. , , , et al.
- Deaths from prescription opioids soar in New York. BMJ. 2013;346:f921.
- Mortality in elderly dementia patients treated with risperidone. J Clin Psychopharmacol. 2006;26(6):566–570. , , .
- Medication reconciliation: a practical tool to reduce the risk of medication errors. J Crit Care. 2003;18(4):201–205. , , , et al.
- Adverse drug event trigger tool: a practical methodology for measuring medication related harm. Qual Saf Health Care. 2003;12(3):194–200. , , .
- Improvement in the detection of adverse drug events by the use of electronic health and prescription records: an evaluation of two trigger tools. Eur J Clin Pharmacol. 2013;69(2):255–259. , , , , .
- Adverse Drug Events in U.S. Hospitals, 2004. Rockville, MD: Agency for Healthcare Research and Quality; April 2007. Statistical Brief 29. , .
- Medication‐Related Adverse Outcomes in U.S. Hospitals and Emergency Departments, 2008. Rockville, MD: Agency for Healthcare Research and Quality; April 2011. Statistical Brief 109. , , .
- US Centers for Medicare and Medicaid Services. Hospital‐Acquired Conditions (Present on Admission Indicator). Available at: http://www.cms.hhs.gov/HospitalAcqCond/. Accessed August 16, 2011. Updated September 20, 2012.
- Fracture risk associated with the use of morphine and opiates. J Intern Med. 2006;260(1):76–87. , , .
- Comorbidity measures for use with administrative data. Med Care. 1998;36(1):8–27. , , , .
- Clinical Classifications Software, Healthcare Cost and Utilization Project (HCUP). Rockville, MD; Agency for Healthcare Research and Quality; December 2009.
- Clinical application of opioid equianalgesic data. Clin J Pain. 2003;19(5)286–297. , , , , .
- Choosing the unit of measurement counts: the use of oral morphine equivalents in studies of opioid consumption is a useful addition to defined daily doses. Palliat Med. 2011;25(7):725–732. , , , , , .
- Cost and quality implications of opioid‐based postsurgical pain control using administrative claims data from a large health system: opioid‐related adverse events and their impact on clinical and economic outcomes. Pharmacotherapy. 2013;33(4):383–391. , , , .
- Opioid‐related adverse drug events in surgical hospitalizations: impact on costs and length of stay. Ann Pharmacother. 2007;41(3):400–406. , , , et al.
- Cost of opioid‐related adverse drug events in surgical patients. J Pain Symptom Manage. 2003;25(3):276–283. , , , et al.
- Opioid analgesics and rates of fatal drug poisoning in the United States. Am J Prev Med. 2006;31(6):506–511. , .
- Geographic variation in the treatment of acute myocardial infarction: the Cooperative Cardiovascular Project. JAMA. 1999;281(7):627–633. , , , et al.
- GUSTO‐1 Investigators. Regional variation across the United States in the management of acute myocardial infarction. Global Utilization of Streptokinase and Tissue Plasminogen Activator for Occluded Coronary Arteries. N Engl J Med. 1995;333(9):565–572. , , , et al;
- Predictors of broad‐spectrum antibiotic prescribing for acute respiratory tract infections in adult primary care. JAMA. 2003;289(6):719–725. , , .
- Geographic variation in Medicare drug spending. N Engl J Med. 2010;363(5):405–409. , , .
- Geographic variation in outpatient antibiotic prescribing among older adults. Arch Intern Med. 2012;172(19):1465–1471. , , .
- Clinical policy: critical issues in the prescribing of opioids for adult patients in the emergency department. Ann Emerg Med. 2012;60(4):499–525. , , , et al.
- The Joint Commission. Facts About Pain Management. Available at: http://www.jointcommission.org/pain_management/. Accessed July 23, 2012.
- The Joint Commission. Sentinel Event Alert: Safe Use of Opioids in Hospitals. Published August 8, 2012. Available at: http://www.jointcommission.org/assets/1/18/SEA_49_opioids_8_2_12_final.pdf. Accessed March 4, 2013.
- Research gaps on use of opioids for chronic noncancer pain: findings from a review of the evidence for an American Pain Society and American Academy of Pain Medicine clinical practice guideline. J Pain. 2009;10(2):147–159. , , , , .
- Side effects of opioids during short‐term administration: effect of age, gender, and race. Clin Pharmacol Ther. 2003;74(2):102–112. , , , , , .
- Postoperative day one: a high‐risk period for respiratory events. Am J Surg. 2005;190(5):752–756. , , , .
Recent reports have drawn attention to the high and increasing rates of opioid prescribing and overdose‐related deaths in the United States.[1, 2, 3, 4, 5, 6, 7, 8, 9] These studies have focused on community‐based and emergency department prescribing, leaving prescribing practices in the inpatient setting unexamined. Given that pain is a frequent complaint in hospitalized patients, and that the Joint Commission mandates assessing pain as a vital sign, hospitalization is potentially a time of heightened use of such medications and could significantly contribute to nosocomial complications and subsequent outpatient use.[10] Variation in prescribing practices, unrelated to patient characteristics, could be a marker of inappropriate prescribing practices and poor quality of care.
Using a large, nationally representative cohort of admissions from July 2009 to June 2010, we sought to determine patterns and predictors of opioid utilization in nonsurgical admissions to US medical centers, hospital variation in use, and the association between hospital‐level use and the risk of opioid‐related adverse events. We hypothesized that hospitals with higher rates of opioid use would have an increased risk of an opioid‐related adverse event per patient exposed.
METHODS
Setting and Patients
We conducted a retrospective cohort study using data from 286 US nonfederal acute‐care facilities contributing to the database maintained by Premier (Premier Healthcare Solutions, Inc., Charlotte, NC). This database, created to measure healthcare utilization and quality of care, is drawn from voluntarily participating hospitals and contains data on approximately 1 in every 4 discharges nationwide.[11] Participating hospitals are similar in geographic distribution and metropolitan (urban/rural) status to hospitals nationwide, although large, nonteaching hospitals are slightly overrepresented in Premier. The database contains patient demographics, International Classification of Diseases, Ninth Revision, Clinical Modification (ICD‐9‐CM) codes, hospital demographics, and a date‐stamped log of all charges during the course of each hospitalization, including diagnostic tests, therapeutic treatments, and medications with dose and route of administration. The study was approved by the institutional review board at Beth Israel Deaconess Medical Center and granted a waiver of informed consent.
We studied a cohort of all adult nonsurgical admissions to participating hospitals from July 1, 2009, through June 30, 2010. We chose to study nonsurgical admissions, as patients undergoing surgical procedures have a clear indication for, and almost always receive, opioid pain medications. We defined a nonsurgical admission as an admission in which there were no charges for operating‐room procedures (including labor and delivery) and the attending of record was not a surgeon. We excluded admissions with unknown gender, since this is a key demographic variable, and admissions with a length of stay greater than 365 days, as these admissions are not representative of the typical admission to an acute‐care hospital. At the hospital level, we excluded hospitals contributing <100 admissions owing to resultant lack of precision in corresponding hospital prescribing rates, and hospitals that did not prescribe the full range of opioid medications (these hospitals had charges for codeine only), as these facilities seemed likely to have unusual limitations on prescribing or incomplete data capture.
Opioid Exposure
We defined opioid exposure as presence of 1 charge for an opioid medication during the admission. Opioid medications included morphine, hydrocodone, hydromorphone, oxycodone, fentanyl, meperidine, methadone, codeine, tramadol, buprenorphine, levorphanol, oxymorphone, pentazocine, propoxyphene, tapentadol, butorphanol, dezocine, and nalbuphine. We grouped the last 9 into an other category owing to infrequent use and/or differing characteristics from the main opioid drug types, such as synthetic, semisynthetic, and partial agonist qualities.
Severe Opioid‐Related Adverse Events
We defined severe opioid‐related adverse events as either naloxone exposure or an opioid‐related adverse drug event diagnosis code. Naloxone use in an adult patient exposed to opioids is one of the Institute for Healthcare Improvement's Trigger Tools for identifying adverse drug events[12] and previously has been demonstrated to have high positive predictive value for a confirmed adverse drug event.[13] We defined naloxone exposure as presence of 1 charge for naloxone. We excluded charges on hospital day 1 to focus on nosocomial events. We defined opioid‐related adverse drug events using ICD‐9‐CM diagnosis codes for poisoning by opioids (overdose, wrong substance given, or taken in error; ICD‐9‐CM 965.02, 965.09, E850.1, E850.2) and drugs causing adverse effects in therapeutic use (ICD‐9‐CM E935.1, E935.2), as specified in prior analyses by the Agency for Healthcare Research and Quality (AHRQ).[14, 15] To avoid capturing adverse events associated with outpatient use, we required the ICD‐9‐CM code to be qualified as not present on admission using the present on admission indicator required by the Centers for Medicare and Medicaid Services for all discharge diagnosis codes since 2008.[16]
Covariates of Interest
We were interested in the relationship between both patient and hospital characteristics and opioid exposure. Patient characteristics of interest included (1) demographic variables, such as age, sex, race (self‐reported by patients at the time of admission), marital status, and payer; (2) whether or not the patient spent any time in the intensive care unit (ICU); (3) comorbidities, identified via ICD‐9‐CM secondary diagnosis codes and diagnosis‐related groups using Healthcare Cost and Utilization Project Comorbidity Software, version 3.7, based on the work of Elixhauser et al[17, 18]; (4) primary ICD‐9‐CM discharge diagnosis groupings, selected based on hypothesized associations with receipt of opioids, and based on the Clinical Classifications Software (CCS)a diagnosis and procedure categorization scheme maintained by the AHRQ, and defined in the Appendix[19]; (5) and nonoperating‐room‐based procedures potentially necessitating opioids during the admission, selected from the 50 most common ICD‐9‐CM procedure codes in our cohort and grouped as cardiovascular procedures (catheterization and insertion of vascular stents), gastrointestinal procedures (upper and lower endoscopy), and mechanical ventilation, further defined in the Appendix. Hospital characteristics of interest included number of beds, population served (urban vs rural), teaching status, and US census region (Northeast, Midwest, South, West).
Statistical Analysis
We calculated the percent of admissions with exposure to any opioid and the percent exposed to each opioid, along with the total number of different opioid medications used during each admission. We also calculated the percent of admissions with parenteral administration and the percent of admissions with oral administration, among those exposed to the individual categories, and in aggregate. Because medications after discharge were unavailable in Premier's dataset, we report the percent of patients with a charge for opioids on the day of discharge.
We determined the daily dose of an opioid by taking the sum of the doses for that opioid charged on a given day. The average daily dose of an opioid was determined by taking the sum of the daily doses and dividing by the number of days on which 1 dose was charged. To facilitate comparison, all opioids, with the exception of those for which standard equivalences are unavailable (tramadol, other opioid category, oral fentanyl, epidural route for all), were converted to oral morphine equivalents using a standard equivalence conversion table.[20, 21] We excluded from our dosage calculations those charges for which standard morphine equivalence was unavailable, or for which dosage was missing. We also excluded from our dosage calculations any dose that was >3 standard deviations (SD) above the mean dose for that opioid, as such extreme values seemed physiologically implausible and more likely to be a data entry error that could lead to significant overestimation of the mean for that opioid.
All multivariable models used a generalized estimating equation (GEE) via the genmod procedure in SAS, with a Poisson distribution error term and a log link, controlling for repeated patient admissions with an autoregressive correlation structure.
To identify independent predictors of opioid receipt, we used a GEE model of opioid receipt where all patient and hospital characteristics listed in Table 1 were included as independent variables.
Patient characteristics, N=1,139,419 | N | % |
---|---|---|
| ||
Age group, y | ||
1824 | 37,464 | 3 |
2534 | 66,541 | 6 |
3544 | 102,701 | 9 |
4554 | 174,830 | 15 |
5564 | 192,570 | 17 |
6574 | 196,407 | 17 |
75+ | 368,906 | 32 |
Sex | ||
Male | 527,062 | 46 |
Female | 612,357 | 54 |
Race | ||
White | 711,993 | 62 |
Black | 176,993 | 16 |
Hispanic | 54,406 | 5 |
Other | 196,027 | 17 |
Marital status | ||
Married | 427,648 | 38 |
Single | 586,343 | 51 |
Unknown/other | 125,428 | 11 |
Primary insurance | ||
Private/commercial | 269,725 | 24 |
Medicare traditional | 502,301 | 44 |
Medicare managed care | 126,344 | 11 |
Medicaid | 125,025 | 11 |
Self‐pay/other | 116,024 | 10 |
ICU care | ||
No | 1,023,027 | 90 |
Yes | 116,392 | 10 |
Comorbidities | ||
AIDS | 5724 | 1 |
Alcohol abuse | 79,633 | 7 |
Deficiency anemias | 213,437 | 19 |
RA/collagen vascular disease | 35,210 | 3 |
Chronic blood‐loss anemia | 10,860 | 1 |
CHF | 190,085 | 17 |
Chronic pulmonary disease | 285,954 | 25 |
Coagulopathy | 48,513 | 4 |
Depression | 145,553 | 13 |
DM without chronic complications | 270,087 | 24 |
DM with chronic complications | 70,732 | 6 |
Drug abuse | 66,886 | 6 |
Hypertension | 696,299 | 61 |
Hypothyroidism | 146,136 | 13 |
Liver disease | 38,130 | 3 |
Lymphoma | 14,032 | 1 |
Fluid and electrolyte disorders | 326,576 | 29 |
Metastatic cancer | 33,435 | 3 |
Other neurological disorders | 124,195 | 11 |
Obesity | 118,915 | 10 |
Paralysis | 38,584 | 3 |
PVD | 77,334 | 7 |
Psychoses | 101,856 | 9 |
Pulmonary circulation disease | 52,106 | 5 |
Renal failure | 175,398 | 15 |
Solid tumor without metastasis | 29,594 | 3 |
Peptic ulcer disease excluding bleeding | 536 | 0 |
Valvular disease | 86,616 | 8 |
Weight loss | 45,132 | 4 |
Primary discharge diagnoses | ||
Cancer | 19,168 | 2 |
Musculoskeletal injuries | 16,798 | 1 |
Pain‐related diagnosesb | 101,533 | 9 |
Alcohol‐related disorders | 16,777 | 1 |
Substance‐related disorders | 13,697 | 1 |
Psychiatric disorders | 41,153 | 4 |
Mood disorders | 28,761 | 3 |
Schizophrenia and other psychotic disorders | 12,392 | 1 |
Procedures | ||
Cardiovascular procedures | 59,901 | 5 |
GI procedures | 31,224 | 3 |
Mechanical ventilation | 7853 | 1 |
Hospital characteristics, N=286 | ||
Number of beds | ||
<200 | 103 | 36 |
201300 | 63 | 22 |
301500 | 81 | 28 |
>500 | 39 | 14 |
Population served | ||
Urban | 225 | 79 |
Rural | 61 | 21 |
Teaching status | ||
Nonteaching | 207 | 72 |
Teaching | 79 | 28 |
US Census region | ||
Northeast | 47 | 16 |
Midwest | 63 | 22 |
South | 115 | 40 |
West | 61 | 21 |
To assess hospital variation in opioid prescribing after adjusting for patient characteristics, we used a GEE model of opioid receipt, controlling for all patient characteristics listed in Table 1. We then took the mean of the predicted probabilities of opioid receipt for the patients within each hospital in our cohort to derive the hospital prescribing rate adjusted for patient characteristics. We report the mean, SD, and range of the prescribing rates for the hospitals in our cohort before and after adjustment for patient characteristics.
To assess whether patients admitted to hospitals with higher rates of opioid prescribing have higher relative risk of severe opioid‐related adverse events, we stratified hospitals into opioid‐prescribing rate quartiles and compared the rates of opioid‐related adverse eventsboth overall and among opioid exposedbetween quartiles. To adjust for patient characteristics, we used a GEE model in which severe opioid‐related adverse event (yes/no) was the dependent variable and hospital‐prescribing rate quartile and all patient characteristics in Table 1 were independent variables. We also performed a sensitivity analysis in which we assessed the association between hospital prescribing‐rate quartile and the individual components of our composite outcome. Our results were qualitatively unchanged using this approach, and only the results of our main analysis are presented.
All analyses were carried out using SAS software, version 9.2 (SAS Institute Inc., Cary, NC).
RESULTS
Patient Admission Characteristics
There were 3,190,934 adult admissions to 300 acute‐care hospitals during our study period. After excluding admissions with a length of stay >365 days (n=25), missing patient sex (n=17), and charges for operating‐room procedures or a surgical attending of record (n=2,018,553), 1,172,339 admissions were available for analysis. There were 12 hospitals with incomplete opioid‐prescribing data (n=32,794) and 2 hospitals that contributed <100 admissions each (n=126), leaving 1,139,419 admissions in 286 hospitals in our analytic cohort. The median age of the cohort was 64 years (interquartile range, 4979 years), and 527,062 (46%) were men. Table 1 shows the characteristics of the admissions in the cohort.
Rate, Route, and Dose of Opioid Exposures
Overall, there were 576,373 (51%) admissions with charges for opioid medications. Among those exposed, 244,760 (43%) had charges for multiple opioids during the admission; 172,090 (30%) had charges for 2 different opioids; and 72,670 (13%) had charges for 3 different opioids. Table 2 shows the percent exposed to each opioid, the percent of exposed with parenteral and oral routes of administration, and the mean daily dose received in oral morphine equivalents.
Exposed | Parenteral Administration | Oral Administration | Dose Received, in Oral Morphine Equivalents | |||||
---|---|---|---|---|---|---|---|---|
N | %a | N | %b | N | %b | Mean | SDc | |
| ||||||||
All opioids | 576,373 | 51 | 378,771 | 66 | 371,796 | 65 | 68 | 185 |
Morphine | 224,811 | 20 | 209,040 | 93 | 21,645 | 10 | 40 | 121 |
Hydrocodone | 162,558 | 14 | 0 | 0 | 160,941 | 99 | 14 | 12 |
Hydromorphone | 146,236 | 13 | 137,936 | 94 | 16,052 | 11 | 113 | 274 |
Oxycodone | 126,733 | 11 | 0 | 0 | 125,033 | 99 | 26 | 37 |
Fentanyl | 105,052 | 9 | 103,113 | 98 | 641 | 1 | 64 | 75 |
Tramadol | 35,570 | 3 | 0 | 0 | 35,570 | 100 | ||
Meperidine | 24,850 | 2 | 24,398 | 98 | 515 | 2 | 36 | 34 |
Methadone | 15,302 | 1 | 370 | 2 | 14,781 | 97 | 337 | 384 |
Codeine | 22,818 | 2 | 178 | 1 | 22,183 | 97 | 9 | 15 |
Other | 45,469 | 4 | 5821 | 13 | 39,618 | 87 |
Among the medications/routes for which conversion to morphine equivalents was possible, dosage was missing in 39,728 out of 2,294,673 opioid charges (2%). The average daily dose received in oral morphine equivalents was 68 mg. A total dose of 50 mg per day was received in 39% of exposed, and a total dose of 100 mg per day was received in 23% of exposed. Among those exposed, 52% (26% of overall admissions) had charges for opioids on the day of discharge.
Rates of Opioid Use by Patient and Hospital Characteristics
Table 3 reports the association between admission characteristics and opioid use. Use was highest in patients between the ages of 25 and 54 years. Although use declined with age, 44% of admissions age 65 years had charges for opioid medication. After adjustment for patient demographics, comorbidities, and hospital characteristics, opioid use was more common in females than males, those age 2554 years compared with those older and younger, those of Caucasian race compared with non‐Caucasian race, and those with Medicare or Medicaid primary insurance. Among the primary discharge diagnoses, patients with musculoskeletal injuries, various specific and nonspecific pain‐related diagnoses, and cancer were significantly more likely to receive opioids than patients without these diagnoses, whereas patients with alcohol‐related disorders and psychiatric disorders were significantly less likely to receive opioids than patients without these diagnoses. Patients admitted to hospitals in the Midwest, South, and West were significantly more likely to receive opioid medications than patients in the Northeast.
Exposed, N=576,373 | Unexposed, N=563,046 | % Exposed | Adjusted RRa | 95% CI | |
---|---|---|---|---|---|
| |||||
Patient characteristics | |||||
Age group, y | |||||
1824 | 17,360 | 20,104 | 46 | (ref) | |
2534 | 37,793 | 28,748 | 57 | 1.17 | 1.16‐1.19 |
3544 | 60,712 | 41,989 | 59 | 1.16 | 1.15‐1.17 |
4554 | 103,798 | 71,032 | 59 | 1.11 | 1.09‐1.12 |
5564 | 108,256 | 84,314 | 56 | 1.00 | 0.98‐1.01 |
6574 | 98,110 | 98,297 | 50 | 0.84 | 0.83‐0.85 |
75+ | 150,344 | 218,562 | 41 | 0.71 | 0.70‐0.72 |
Sex | |||||
Male | 255,315 | 271,747 | 48 | (ref) | |
Female | 321,058 | 291,299 | 52 | 1.11 | 1.10‐1.11 |
Race | |||||
White | 365,107 | 346,886 | 51 | (ref) | |
Black | 92,013 | 84,980 | 52 | 0.93 | 0.92‐0.93 |
Hispanic | 27,592 | 26,814 | 51 | 0.94 | 0.93‐0.94 |
Other | 91,661 | 104,366 | 47 | 0.93 | 0.92‐0.93 |
Marital status | |||||
Married | 222,912 | 204,736 | 52 | (ref) | |
Single | 297,742 | 288,601 | 51 | 1.00 | 0.99‐1.01 |
Unknown/other | 55,719 | 69,709 | 44 | 0.94 | 0.93‐0.95 |
Primary insurance | |||||
Private/commercial | 143,954 | 125,771 | 53 | (ref) | |
Medicare traditional | 236,114 | 266,187 | 47 | 1.10 | 1.09‐1.10 |
Medicare managed care | 59,104 | 67,240 | 47 | 1.11 | 1.11‐1.12 |
Medicaid | 73,583 | 51,442 | 59 | 1.13 | 1.12‐1.13 |
Self‐pay/other | 63,618 | 52,406 | 55 | 1.03 | 1.02‐1.04 |
ICU care | |||||
No | 510,654 | 512,373 | 50 | (ref) | |
Yes | 65,719 | 50,673 | 56 | 1.02 | 1.01‐1.03 |
Comorbiditiesb | |||||
AIDS | 3655 | 2069 | 64 | 1.09 | 1.07‐1.12 |
Alcohol abuse | 35,112 | 44,521 | 44 | 0.92 | 0.91‐0.93 |
Deficiency anemias | 115,842 | 97,595 | 54 | 1.08 | 1.08‐1.09 |
RA/collagen vascular disease | 22,519 | 12,691 | 64 | 1.22 | 1.21‐1.23 |
Chronic blood‐loss anemia | 6444 | 4416 | 59 | 1.04 | 1.02‐1.05 |
CHF | 88,895 | 101,190 | 47 | 0.99 | 0.98‐0.99 |
Chronic pulmonary disease | 153,667 | 132,287 | 54 | 1.08 | 1.08‐1.08 |
Coagulopathy | 25,802 | 22,711 | 53 | 1.03 | 1.02‐1.04 |
Depression | 83,051 | 62,502 | 57 | 1.08 | 1.08‐1.09 |
DM without chronic complications | 136,184 | 133,903 | 50 | 0.99 | 0.99‐0.99 |
DM with chronic complications | 38,696 | 32,036 | 55 | 1.04 | 1.03‐1.05 |
Drug abuse | 37,202 | 29,684 | 56 | 1.14 | 1.13‐1.15 |
Hypertension | 344,718 | 351,581 | 50 | 0.98 | 0.97‐0.98 |
Hypothyroidism | 70,786 | 75,350 | 48 | 0.99 | 0.99‐0.99 |
Liver disease | 24,067 | 14,063 | 63 | 1.15 | 1.14‐1.16 |
Lymphoma | 7727 | 6305 | 55 | 1.16 | 1.14‐1.17 |
Fluid and electrolyte disorders | 168,814 | 157,762 | 52 | 1.04 | 1.03‐1.04 |
Metastatic cancer | 23,920 | 9515 | 72 | 1.40 | 1.39‐1.42 |
Other neurological disorders | 51,091 | 73,104 | 41 | 0.87 | 0.86‐0.87 |
Obesity | 69,584 | 49,331 | 59 | 1.05 | 1.04‐1.05 |
Paralysis | 17,497 | 21,087 | 45 | 0.97 | 0.96‐0.98 |
PVD | 42,176 | 35,158 | 55 | 1.11 | 1.11‐1.12 |
Psychoses | 38,638 | 63,218 | 38 | 0.91 | 0.90‐0.92 |
Pulmonary circulation disease | 26,656 | 25,450 | 51 | 1.05 | 1.04‐1.06 |
Renal failure | 86,565 | 88,833 | 49 | 1.01 | 1.01‐1.02 |
Solid tumor without metastasis | 16,258 | 13,336 | 55 | 1.14 | 1.13‐1.15 |
Peptic ulcer disease excluding bleeding | 376 | 160 | 70 | 1.12 | 1.07‐1.18 |
Valvular disease | 38,396 | 48,220 | 44 | 0.93 | 0.92‐0.94 |
Weight loss | 25,724 | 19,408 | 57 | 1.09 | 1.08‐1.10 |
Primary discharge diagnosesb | |||||
Cancer | 13,986 | 5182 | 73 | 1.20 | 1.19‐1.21 |
Musculoskeletal injuries | 14,638 | 2160 | 87 | 2.02 | 2.002.04 |
Pain‐related diagnosesc | 64,656 | 36,877 | 64 | 1.20 | 1.20‐1.21 |
Alcohol‐related disorders | 3425 | 13,352 | 20 | 0.46 | 0.44‐0.47 |
Substance‐related disorders | 8680 | 5017 | 63 | 1.03 | 1.01‐1.04 |
Psychiatric disorders | 7253 | 33,900 | 18 | 0.37 | 0.36‐0.38 |
Mood disorders | 5943 | 22,818 | 21 | ||
Schizophrenia and other psychotic disorders | 1310 | 11,082 | 11 | ||
Proceduresb | |||||
Cardiovascular procedures | 50,997 | 8904 | 85 | 1.80 | 1.79‐1.81 |
GI procedures | 27,206 | 4018 | 87 | 1.70 | 1.69‐1.71 |
Mechanical ventilation | 5341 | 2512 | 68 | 1.37 | 1.34‐1.39 |
Hospital characteristics | |||||
Number of beds | |||||
<200 | 100,900 | 88,439 | 53 | (ref) | |
201300 | 104,213 | 99,995 | 51 | 0.95 | 0.95‐0.96 |
301500 | 215,340 | 209,104 | 51 | 0.94 | 0.94‐0.95 |
>500 | 155,920 | 165,508 | 49 | 0.96 | 0.95‐0.96 |
Population served | |||||
Urban | 511,727 | 506,803 | 50 | (ref) | |
Rural | 64,646 | 56,243 | 53 | 0.98 | 0.97‐0.99 |
Teaching status | |||||
Nonteaching | 366,623 | 343,581 | 52 | (ref) | |
Teaching | 209,750 | 219,465 | 49 | 1.00 | 0.99‐1.01 |
US Census region | |||||
Northeast | 99,377 | 149,446 | 40 | (ref) | |
Midwest | 123,194 | 120,322 | 51 | 1.26 | (1.25‐1.27) |
South | 251,624 | 213,029 | 54 | 1.33 | (1.33‐1.34) |
West | 102,178 | 80,249 | 56 | 1.37 | (1.36‐1.38) |
Variation in Opioid Prescribing
Figure 1 shows the histograms of hospital opioid prescribing rate for the 286 hospitals in our cohort (A) before and (B) after adjustment for patient characteristics. The observed rates ranged from 5% in the lowest‐prescribing hospital to 72% in the highest‐prescribing hospital, with a mean (SD) of 51% (10%). After adjusting for patient characteristics, the adjusted opioid‐prescribing rates ranged from 33% to 64%, with a mean (SD) of 50% (4%).
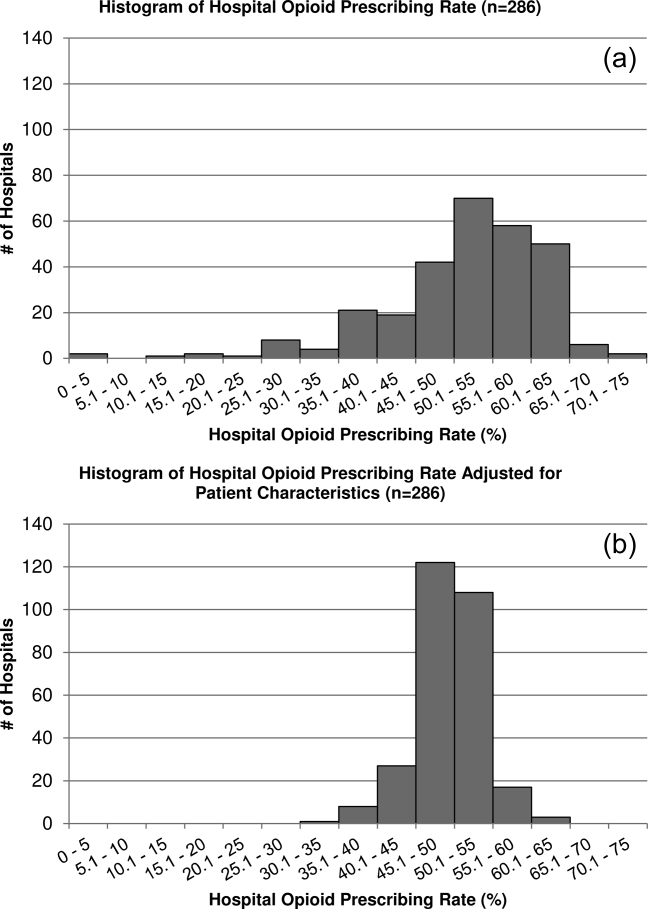
Severe Opioid‐Related Adverse Events
Among admissions with opioid exposure (n=576,373), naloxone use occurred in 2345 (0.41%) and opioid‐related adverse drug events in 1174 (0.20%), for a total of 3441 (0.60%) severe opioid‐related adverse events (some patients experienced both). Table 4 reports the opioid exposure and severe opioid‐related adverse‐event rates within hospital opioid‐prescribing rate quartiles, along with the adjusted association between the hospital opioid‐prescribing rate quartile and severe opioid‐related adverse events. After adjusting for patient characteristics, the relative risk of a severe opioid‐related adverse event was significantly greater in hospitals with higher opioid‐prescribing rates, both overall and among opioid exposed.
Quartile | No. of Patients | Opioid Exposed, n (%) | Opioid‐Related Adverse Events, n (%) | Adjusted RR in All Patients, RR (95% CI), N=1,139,419a | Adjusted RR in Opioid Exposed, RR (95% CI), N=576,373a |
---|---|---|---|---|---|
| |||||
1 | 349,747 | 132,824 (38) | 719 (0.21) | (ref) | (ref) |
2 | 266,652 | 134,590 (50) | 729 (0.27) | 1.31 (1.17‐1.45) | 1.07 (0.96‐1.18) |
3 | 251,042 | 139,770 (56) | 922 (0.37) | 1.72 (1.56‐1.90) | 1.31 (1.19‐1.44) |
4 | 271,978 | 169,189 (62) | 1071 (0.39) | 1.73 (1.57‐1.90) | 1.23 (1.12‐1.35) |
DISCUSSION
In this analysis of a large cohort of hospitalized nonsurgical patients, we found that more than half of all patients received opioids, with 43% of those exposed receiving multiple opioids during their admission and 52% receiving opioids on the day of discharge. Considerable hospital variation in opioid use was evident, and this was not fully explained by patient characteristics. Severe opioid‐related adverse events occurred more frequently at hospitals with higher opioid‐prescribing rates, and the relative risk of a severe adverse event per patient prescribed opioids was also higher in these hospitals. To our knowledge, this is the first study to describe the scope of opioid utilization and the relationship between utilization and severe opioid‐related adverse events in a sample of nonsurgical patients in US acute‐care facilities.
Our use of naloxone charges and opioid‐specific ICD‐9‐CM coding to define an opioid‐related adverse event was intended to capture only the most severe opioid‐related adverse events. We chose to focus on these events in our analysis to maximize the specificity of our outcome definition and thereby minimize confounding in our observed associations. The rate of less‐severe opioid‐related adverse events, such as nausea, constipation, and pruritis, is likely much higher and not captured in our outcome definition. Prior analyses have found variable rates of opioid‐related adverse events of approximately 1.8% to 13.6% of exposed patients.[22, 23, 24] However, these analyses focused on surgical patients and included less‐severe events. To our knowledge, ours is the first analysis of severe opioid‐related adverse events in nonsurgical patients.
Our finding that severe opioid‐related adverse events increase as opioid prescribing increases is consistent with that which has been demonstrated in the community setting, where rates of opioid‐related adverse events and mortality are higher in communities with higher levels of opioid prescribing.[2, 8, 25] This finding is expected, as greater use of a class of medications with known side effects would be expected to result in a higher overall rate of adverse events. More concerning, however, is the fact that this relationship persists when focusing exclusively on opioid‐exposed patients. Among similar patients receiving opioids at different hospitals, those hospitalized in facilities with higher opioid‐prescribing rates have higher rates of severe opioid‐related adverse events. This suggests that hospitals that use opioids more frequently do not do so more safely. Rather, the increased overall prescribing rates are associated with heightened risk for a serious adverse event per patient exposed and may reflect unsafe prescribing practices.
Furthermore, our results demonstrate both regional and hospital variation in use of opioids not fully explained by patient characteristics, similar to that which has been demonstrated for other drugs and heathcare services.[26, 27, 28, 29, 30] The implications of these findings are limited by our lack of information on pain severity or prior outpatient treatment, and our resultant inability to evaluate the appropriateness of opioid use in this analysis. Additionally, although we controlled for a large number of patient and hospital characteristics, there could be other significant predictors of use not accounted for in our analysis. However, it seems unlikely that differential pain severity or patient characteristics between patients in different regions of the country could fully explain a 37% relative difference in prescribing between the lowest‐ and highest‐prescribing regions, after accounting for the 44 patient‐level variables in our models. Whereas variation in use unrelated to patient factors could represent inappropriate prescribing practices, it could also be a marker of uncertainty regarding what constitutes appropriate prescribing and high‐quality care in this realm. Although guidelines advocate for standard pain assessments and a step‐up approach to treatment,[31, 32, 33] the lack of objective measures of pain severity and lack of evidence‐based recommendations on the use of opioids for noncancer pain[34] will almost certainly lead to persistent variation in opioid prescribing despite guideline‐driven care.
Nonetheless, our findings suggest that opportunities exist to make opioid prescribing safer in hospitalized patients. Studies aimed at elucidating the source of regional and hospital variation are necessary. Additionally, efforts should focus on identifying patient and prescribing characteristics associated with heightened risk of opioid‐related adverse events. Prior studies have demonstrated that the risks of opioid medications increase with increasing age of the patient.[35, 36] Although opioid use in our cohort declined with age, 44% of admissions age 65 years had charges for opioid medications. Studies in outpatients have also demonstrated that the risks of opioid overdose and overdose‐related death increase with dose.[5, 7] One study demonstrated a 3.7‐fold increased risk of overdose at doses of 5099 mg/day in oral morphine equivalents, and an 8.9‐fold increased risk at doses of 100 mg/day, compared with doses of 20 mg/day.[7] The prevalence of high dose exposure observed in our cohort, coupled with the older age of hospitalized patients, suggests potential targets for promoting safer use in hospitalized patients through interventions such as computerized decision support and enhanced monitoring in those at highest risk.
Because medications after discharge were unavailable in our dataset, the percentage of patients given a prescription for opioid medication on discharge is unknown. However, given that opioids are often tapered rather than abruptly discontinued, our finding that 26% of hospitalized nonsurgical patients received opioids on the day of discharge suggests that a substantial proportion of patients may be discharged with a prescription for opioid medication. Given the possibility of coexistent outpatient opioid prescriptions, these findings draw attention to the importance of assuring development and streamlined accessibility of data from state prescription drug monitoring programs and suggest that increased attention should be paid to the role that inpatient opioid prescribing plays in the increased rates of chronic opioid use and overdose‐related deaths in the United States.
There are additional limitations to our analysis. First, although the database used for this analysis captures a large proportion of admissions to US acute‐care facilities and is similar in composition, it is possible that participating medical centers differ from nonparticipating medical centers in ways that could be associated with opioid prescribing. Additionally, although Premier performs extensive validation and correction processes to assure the quality of their data, there is still likely to be a small amount of random error in the database, which could particularly impact dosage calculations. The lack of preadmission medications in our database precluded identification of the proportion of patients newly started on opioid medications. Lastly, it is possible that the hospital prescribing‐rate quartile is associated with patient characteristics unaccounted for in our analysis, and, therefore, the possibility of residual confounding still exists.
In conclusion, the majority of hospitalized nonsurgical patients are exposed to opioid medications during the course of their hospitalizations, often at high doses. More than half of those exposed are still receiving these medications on the day of discharge. We found hospital and regional variation in opioid use that was not fully explained by patient characteristics, and higher levels of hospital use were associated with higher risk of severe opioid‐related adverse events in opioid‐exposed patients. Further research is necessary to investigate the appropriateness of opioid use in this patient population, the sources of variation in use, and the predictors of opioid‐related adverse events in hospitalized patients to allow development of interventions to make hospital use safer.
Disclosures
Disclosures: Dr. Herzig had full access to all of the data in the study and takes responsibility for the integrity of the data and the accuracy of the data analysis. Dr. Herzig was funded by grant no. K23AG042459 from the National Institute on Aging. Dr. Marcantonio was funded by grant nos. P01AG031720, R01AG030618, R03AG028189, and K24AG035075 from the National Institute on Aging. The funding organization had no involvement in any aspect of the study, including design, conduct, and reporting of the study. None of the authors have any conflicts of interest to disclose.
Recent reports have drawn attention to the high and increasing rates of opioid prescribing and overdose‐related deaths in the United States.[1, 2, 3, 4, 5, 6, 7, 8, 9] These studies have focused on community‐based and emergency department prescribing, leaving prescribing practices in the inpatient setting unexamined. Given that pain is a frequent complaint in hospitalized patients, and that the Joint Commission mandates assessing pain as a vital sign, hospitalization is potentially a time of heightened use of such medications and could significantly contribute to nosocomial complications and subsequent outpatient use.[10] Variation in prescribing practices, unrelated to patient characteristics, could be a marker of inappropriate prescribing practices and poor quality of care.
Using a large, nationally representative cohort of admissions from July 2009 to June 2010, we sought to determine patterns and predictors of opioid utilization in nonsurgical admissions to US medical centers, hospital variation in use, and the association between hospital‐level use and the risk of opioid‐related adverse events. We hypothesized that hospitals with higher rates of opioid use would have an increased risk of an opioid‐related adverse event per patient exposed.
METHODS
Setting and Patients
We conducted a retrospective cohort study using data from 286 US nonfederal acute‐care facilities contributing to the database maintained by Premier (Premier Healthcare Solutions, Inc., Charlotte, NC). This database, created to measure healthcare utilization and quality of care, is drawn from voluntarily participating hospitals and contains data on approximately 1 in every 4 discharges nationwide.[11] Participating hospitals are similar in geographic distribution and metropolitan (urban/rural) status to hospitals nationwide, although large, nonteaching hospitals are slightly overrepresented in Premier. The database contains patient demographics, International Classification of Diseases, Ninth Revision, Clinical Modification (ICD‐9‐CM) codes, hospital demographics, and a date‐stamped log of all charges during the course of each hospitalization, including diagnostic tests, therapeutic treatments, and medications with dose and route of administration. The study was approved by the institutional review board at Beth Israel Deaconess Medical Center and granted a waiver of informed consent.
We studied a cohort of all adult nonsurgical admissions to participating hospitals from July 1, 2009, through June 30, 2010. We chose to study nonsurgical admissions, as patients undergoing surgical procedures have a clear indication for, and almost always receive, opioid pain medications. We defined a nonsurgical admission as an admission in which there were no charges for operating‐room procedures (including labor and delivery) and the attending of record was not a surgeon. We excluded admissions with unknown gender, since this is a key demographic variable, and admissions with a length of stay greater than 365 days, as these admissions are not representative of the typical admission to an acute‐care hospital. At the hospital level, we excluded hospitals contributing <100 admissions owing to resultant lack of precision in corresponding hospital prescribing rates, and hospitals that did not prescribe the full range of opioid medications (these hospitals had charges for codeine only), as these facilities seemed likely to have unusual limitations on prescribing or incomplete data capture.
Opioid Exposure
We defined opioid exposure as presence of 1 charge for an opioid medication during the admission. Opioid medications included morphine, hydrocodone, hydromorphone, oxycodone, fentanyl, meperidine, methadone, codeine, tramadol, buprenorphine, levorphanol, oxymorphone, pentazocine, propoxyphene, tapentadol, butorphanol, dezocine, and nalbuphine. We grouped the last 9 into an other category owing to infrequent use and/or differing characteristics from the main opioid drug types, such as synthetic, semisynthetic, and partial agonist qualities.
Severe Opioid‐Related Adverse Events
We defined severe opioid‐related adverse events as either naloxone exposure or an opioid‐related adverse drug event diagnosis code. Naloxone use in an adult patient exposed to opioids is one of the Institute for Healthcare Improvement's Trigger Tools for identifying adverse drug events[12] and previously has been demonstrated to have high positive predictive value for a confirmed adverse drug event.[13] We defined naloxone exposure as presence of 1 charge for naloxone. We excluded charges on hospital day 1 to focus on nosocomial events. We defined opioid‐related adverse drug events using ICD‐9‐CM diagnosis codes for poisoning by opioids (overdose, wrong substance given, or taken in error; ICD‐9‐CM 965.02, 965.09, E850.1, E850.2) and drugs causing adverse effects in therapeutic use (ICD‐9‐CM E935.1, E935.2), as specified in prior analyses by the Agency for Healthcare Research and Quality (AHRQ).[14, 15] To avoid capturing adverse events associated with outpatient use, we required the ICD‐9‐CM code to be qualified as not present on admission using the present on admission indicator required by the Centers for Medicare and Medicaid Services for all discharge diagnosis codes since 2008.[16]
Covariates of Interest
We were interested in the relationship between both patient and hospital characteristics and opioid exposure. Patient characteristics of interest included (1) demographic variables, such as age, sex, race (self‐reported by patients at the time of admission), marital status, and payer; (2) whether or not the patient spent any time in the intensive care unit (ICU); (3) comorbidities, identified via ICD‐9‐CM secondary diagnosis codes and diagnosis‐related groups using Healthcare Cost and Utilization Project Comorbidity Software, version 3.7, based on the work of Elixhauser et al[17, 18]; (4) primary ICD‐9‐CM discharge diagnosis groupings, selected based on hypothesized associations with receipt of opioids, and based on the Clinical Classifications Software (CCS)a diagnosis and procedure categorization scheme maintained by the AHRQ, and defined in the Appendix[19]; (5) and nonoperating‐room‐based procedures potentially necessitating opioids during the admission, selected from the 50 most common ICD‐9‐CM procedure codes in our cohort and grouped as cardiovascular procedures (catheterization and insertion of vascular stents), gastrointestinal procedures (upper and lower endoscopy), and mechanical ventilation, further defined in the Appendix. Hospital characteristics of interest included number of beds, population served (urban vs rural), teaching status, and US census region (Northeast, Midwest, South, West).
Statistical Analysis
We calculated the percent of admissions with exposure to any opioid and the percent exposed to each opioid, along with the total number of different opioid medications used during each admission. We also calculated the percent of admissions with parenteral administration and the percent of admissions with oral administration, among those exposed to the individual categories, and in aggregate. Because medications after discharge were unavailable in Premier's dataset, we report the percent of patients with a charge for opioids on the day of discharge.
We determined the daily dose of an opioid by taking the sum of the doses for that opioid charged on a given day. The average daily dose of an opioid was determined by taking the sum of the daily doses and dividing by the number of days on which 1 dose was charged. To facilitate comparison, all opioids, with the exception of those for which standard equivalences are unavailable (tramadol, other opioid category, oral fentanyl, epidural route for all), were converted to oral morphine equivalents using a standard equivalence conversion table.[20, 21] We excluded from our dosage calculations those charges for which standard morphine equivalence was unavailable, or for which dosage was missing. We also excluded from our dosage calculations any dose that was >3 standard deviations (SD) above the mean dose for that opioid, as such extreme values seemed physiologically implausible and more likely to be a data entry error that could lead to significant overestimation of the mean for that opioid.
All multivariable models used a generalized estimating equation (GEE) via the genmod procedure in SAS, with a Poisson distribution error term and a log link, controlling for repeated patient admissions with an autoregressive correlation structure.
To identify independent predictors of opioid receipt, we used a GEE model of opioid receipt where all patient and hospital characteristics listed in Table 1 were included as independent variables.
Patient characteristics, N=1,139,419 | N | % |
---|---|---|
| ||
Age group, y | ||
1824 | 37,464 | 3 |
2534 | 66,541 | 6 |
3544 | 102,701 | 9 |
4554 | 174,830 | 15 |
5564 | 192,570 | 17 |
6574 | 196,407 | 17 |
75+ | 368,906 | 32 |
Sex | ||
Male | 527,062 | 46 |
Female | 612,357 | 54 |
Race | ||
White | 711,993 | 62 |
Black | 176,993 | 16 |
Hispanic | 54,406 | 5 |
Other | 196,027 | 17 |
Marital status | ||
Married | 427,648 | 38 |
Single | 586,343 | 51 |
Unknown/other | 125,428 | 11 |
Primary insurance | ||
Private/commercial | 269,725 | 24 |
Medicare traditional | 502,301 | 44 |
Medicare managed care | 126,344 | 11 |
Medicaid | 125,025 | 11 |
Self‐pay/other | 116,024 | 10 |
ICU care | ||
No | 1,023,027 | 90 |
Yes | 116,392 | 10 |
Comorbidities | ||
AIDS | 5724 | 1 |
Alcohol abuse | 79,633 | 7 |
Deficiency anemias | 213,437 | 19 |
RA/collagen vascular disease | 35,210 | 3 |
Chronic blood‐loss anemia | 10,860 | 1 |
CHF | 190,085 | 17 |
Chronic pulmonary disease | 285,954 | 25 |
Coagulopathy | 48,513 | 4 |
Depression | 145,553 | 13 |
DM without chronic complications | 270,087 | 24 |
DM with chronic complications | 70,732 | 6 |
Drug abuse | 66,886 | 6 |
Hypertension | 696,299 | 61 |
Hypothyroidism | 146,136 | 13 |
Liver disease | 38,130 | 3 |
Lymphoma | 14,032 | 1 |
Fluid and electrolyte disorders | 326,576 | 29 |
Metastatic cancer | 33,435 | 3 |
Other neurological disorders | 124,195 | 11 |
Obesity | 118,915 | 10 |
Paralysis | 38,584 | 3 |
PVD | 77,334 | 7 |
Psychoses | 101,856 | 9 |
Pulmonary circulation disease | 52,106 | 5 |
Renal failure | 175,398 | 15 |
Solid tumor without metastasis | 29,594 | 3 |
Peptic ulcer disease excluding bleeding | 536 | 0 |
Valvular disease | 86,616 | 8 |
Weight loss | 45,132 | 4 |
Primary discharge diagnoses | ||
Cancer | 19,168 | 2 |
Musculoskeletal injuries | 16,798 | 1 |
Pain‐related diagnosesb | 101,533 | 9 |
Alcohol‐related disorders | 16,777 | 1 |
Substance‐related disorders | 13,697 | 1 |
Psychiatric disorders | 41,153 | 4 |
Mood disorders | 28,761 | 3 |
Schizophrenia and other psychotic disorders | 12,392 | 1 |
Procedures | ||
Cardiovascular procedures | 59,901 | 5 |
GI procedures | 31,224 | 3 |
Mechanical ventilation | 7853 | 1 |
Hospital characteristics, N=286 | ||
Number of beds | ||
<200 | 103 | 36 |
201300 | 63 | 22 |
301500 | 81 | 28 |
>500 | 39 | 14 |
Population served | ||
Urban | 225 | 79 |
Rural | 61 | 21 |
Teaching status | ||
Nonteaching | 207 | 72 |
Teaching | 79 | 28 |
US Census region | ||
Northeast | 47 | 16 |
Midwest | 63 | 22 |
South | 115 | 40 |
West | 61 | 21 |
To assess hospital variation in opioid prescribing after adjusting for patient characteristics, we used a GEE model of opioid receipt, controlling for all patient characteristics listed in Table 1. We then took the mean of the predicted probabilities of opioid receipt for the patients within each hospital in our cohort to derive the hospital prescribing rate adjusted for patient characteristics. We report the mean, SD, and range of the prescribing rates for the hospitals in our cohort before and after adjustment for patient characteristics.
To assess whether patients admitted to hospitals with higher rates of opioid prescribing have higher relative risk of severe opioid‐related adverse events, we stratified hospitals into opioid‐prescribing rate quartiles and compared the rates of opioid‐related adverse eventsboth overall and among opioid exposedbetween quartiles. To adjust for patient characteristics, we used a GEE model in which severe opioid‐related adverse event (yes/no) was the dependent variable and hospital‐prescribing rate quartile and all patient characteristics in Table 1 were independent variables. We also performed a sensitivity analysis in which we assessed the association between hospital prescribing‐rate quartile and the individual components of our composite outcome. Our results were qualitatively unchanged using this approach, and only the results of our main analysis are presented.
All analyses were carried out using SAS software, version 9.2 (SAS Institute Inc., Cary, NC).
RESULTS
Patient Admission Characteristics
There were 3,190,934 adult admissions to 300 acute‐care hospitals during our study period. After excluding admissions with a length of stay >365 days (n=25), missing patient sex (n=17), and charges for operating‐room procedures or a surgical attending of record (n=2,018,553), 1,172,339 admissions were available for analysis. There were 12 hospitals with incomplete opioid‐prescribing data (n=32,794) and 2 hospitals that contributed <100 admissions each (n=126), leaving 1,139,419 admissions in 286 hospitals in our analytic cohort. The median age of the cohort was 64 years (interquartile range, 4979 years), and 527,062 (46%) were men. Table 1 shows the characteristics of the admissions in the cohort.
Rate, Route, and Dose of Opioid Exposures
Overall, there were 576,373 (51%) admissions with charges for opioid medications. Among those exposed, 244,760 (43%) had charges for multiple opioids during the admission; 172,090 (30%) had charges for 2 different opioids; and 72,670 (13%) had charges for 3 different opioids. Table 2 shows the percent exposed to each opioid, the percent of exposed with parenteral and oral routes of administration, and the mean daily dose received in oral morphine equivalents.
Exposed | Parenteral Administration | Oral Administration | Dose Received, in Oral Morphine Equivalents | |||||
---|---|---|---|---|---|---|---|---|
N | %a | N | %b | N | %b | Mean | SDc | |
| ||||||||
All opioids | 576,373 | 51 | 378,771 | 66 | 371,796 | 65 | 68 | 185 |
Morphine | 224,811 | 20 | 209,040 | 93 | 21,645 | 10 | 40 | 121 |
Hydrocodone | 162,558 | 14 | 0 | 0 | 160,941 | 99 | 14 | 12 |
Hydromorphone | 146,236 | 13 | 137,936 | 94 | 16,052 | 11 | 113 | 274 |
Oxycodone | 126,733 | 11 | 0 | 0 | 125,033 | 99 | 26 | 37 |
Fentanyl | 105,052 | 9 | 103,113 | 98 | 641 | 1 | 64 | 75 |
Tramadol | 35,570 | 3 | 0 | 0 | 35,570 | 100 | ||
Meperidine | 24,850 | 2 | 24,398 | 98 | 515 | 2 | 36 | 34 |
Methadone | 15,302 | 1 | 370 | 2 | 14,781 | 97 | 337 | 384 |
Codeine | 22,818 | 2 | 178 | 1 | 22,183 | 97 | 9 | 15 |
Other | 45,469 | 4 | 5821 | 13 | 39,618 | 87 |
Among the medications/routes for which conversion to morphine equivalents was possible, dosage was missing in 39,728 out of 2,294,673 opioid charges (2%). The average daily dose received in oral morphine equivalents was 68 mg. A total dose of 50 mg per day was received in 39% of exposed, and a total dose of 100 mg per day was received in 23% of exposed. Among those exposed, 52% (26% of overall admissions) had charges for opioids on the day of discharge.
Rates of Opioid Use by Patient and Hospital Characteristics
Table 3 reports the association between admission characteristics and opioid use. Use was highest in patients between the ages of 25 and 54 years. Although use declined with age, 44% of admissions age 65 years had charges for opioid medication. After adjustment for patient demographics, comorbidities, and hospital characteristics, opioid use was more common in females than males, those age 2554 years compared with those older and younger, those of Caucasian race compared with non‐Caucasian race, and those with Medicare or Medicaid primary insurance. Among the primary discharge diagnoses, patients with musculoskeletal injuries, various specific and nonspecific pain‐related diagnoses, and cancer were significantly more likely to receive opioids than patients without these diagnoses, whereas patients with alcohol‐related disorders and psychiatric disorders were significantly less likely to receive opioids than patients without these diagnoses. Patients admitted to hospitals in the Midwest, South, and West were significantly more likely to receive opioid medications than patients in the Northeast.
Exposed, N=576,373 | Unexposed, N=563,046 | % Exposed | Adjusted RRa | 95% CI | |
---|---|---|---|---|---|
| |||||
Patient characteristics | |||||
Age group, y | |||||
1824 | 17,360 | 20,104 | 46 | (ref) | |
2534 | 37,793 | 28,748 | 57 | 1.17 | 1.16‐1.19 |
3544 | 60,712 | 41,989 | 59 | 1.16 | 1.15‐1.17 |
4554 | 103,798 | 71,032 | 59 | 1.11 | 1.09‐1.12 |
5564 | 108,256 | 84,314 | 56 | 1.00 | 0.98‐1.01 |
6574 | 98,110 | 98,297 | 50 | 0.84 | 0.83‐0.85 |
75+ | 150,344 | 218,562 | 41 | 0.71 | 0.70‐0.72 |
Sex | |||||
Male | 255,315 | 271,747 | 48 | (ref) | |
Female | 321,058 | 291,299 | 52 | 1.11 | 1.10‐1.11 |
Race | |||||
White | 365,107 | 346,886 | 51 | (ref) | |
Black | 92,013 | 84,980 | 52 | 0.93 | 0.92‐0.93 |
Hispanic | 27,592 | 26,814 | 51 | 0.94 | 0.93‐0.94 |
Other | 91,661 | 104,366 | 47 | 0.93 | 0.92‐0.93 |
Marital status | |||||
Married | 222,912 | 204,736 | 52 | (ref) | |
Single | 297,742 | 288,601 | 51 | 1.00 | 0.99‐1.01 |
Unknown/other | 55,719 | 69,709 | 44 | 0.94 | 0.93‐0.95 |
Primary insurance | |||||
Private/commercial | 143,954 | 125,771 | 53 | (ref) | |
Medicare traditional | 236,114 | 266,187 | 47 | 1.10 | 1.09‐1.10 |
Medicare managed care | 59,104 | 67,240 | 47 | 1.11 | 1.11‐1.12 |
Medicaid | 73,583 | 51,442 | 59 | 1.13 | 1.12‐1.13 |
Self‐pay/other | 63,618 | 52,406 | 55 | 1.03 | 1.02‐1.04 |
ICU care | |||||
No | 510,654 | 512,373 | 50 | (ref) | |
Yes | 65,719 | 50,673 | 56 | 1.02 | 1.01‐1.03 |
Comorbiditiesb | |||||
AIDS | 3655 | 2069 | 64 | 1.09 | 1.07‐1.12 |
Alcohol abuse | 35,112 | 44,521 | 44 | 0.92 | 0.91‐0.93 |
Deficiency anemias | 115,842 | 97,595 | 54 | 1.08 | 1.08‐1.09 |
RA/collagen vascular disease | 22,519 | 12,691 | 64 | 1.22 | 1.21‐1.23 |
Chronic blood‐loss anemia | 6444 | 4416 | 59 | 1.04 | 1.02‐1.05 |
CHF | 88,895 | 101,190 | 47 | 0.99 | 0.98‐0.99 |
Chronic pulmonary disease | 153,667 | 132,287 | 54 | 1.08 | 1.08‐1.08 |
Coagulopathy | 25,802 | 22,711 | 53 | 1.03 | 1.02‐1.04 |
Depression | 83,051 | 62,502 | 57 | 1.08 | 1.08‐1.09 |
DM without chronic complications | 136,184 | 133,903 | 50 | 0.99 | 0.99‐0.99 |
DM with chronic complications | 38,696 | 32,036 | 55 | 1.04 | 1.03‐1.05 |
Drug abuse | 37,202 | 29,684 | 56 | 1.14 | 1.13‐1.15 |
Hypertension | 344,718 | 351,581 | 50 | 0.98 | 0.97‐0.98 |
Hypothyroidism | 70,786 | 75,350 | 48 | 0.99 | 0.99‐0.99 |
Liver disease | 24,067 | 14,063 | 63 | 1.15 | 1.14‐1.16 |
Lymphoma | 7727 | 6305 | 55 | 1.16 | 1.14‐1.17 |
Fluid and electrolyte disorders | 168,814 | 157,762 | 52 | 1.04 | 1.03‐1.04 |
Metastatic cancer | 23,920 | 9515 | 72 | 1.40 | 1.39‐1.42 |
Other neurological disorders | 51,091 | 73,104 | 41 | 0.87 | 0.86‐0.87 |
Obesity | 69,584 | 49,331 | 59 | 1.05 | 1.04‐1.05 |
Paralysis | 17,497 | 21,087 | 45 | 0.97 | 0.96‐0.98 |
PVD | 42,176 | 35,158 | 55 | 1.11 | 1.11‐1.12 |
Psychoses | 38,638 | 63,218 | 38 | 0.91 | 0.90‐0.92 |
Pulmonary circulation disease | 26,656 | 25,450 | 51 | 1.05 | 1.04‐1.06 |
Renal failure | 86,565 | 88,833 | 49 | 1.01 | 1.01‐1.02 |
Solid tumor without metastasis | 16,258 | 13,336 | 55 | 1.14 | 1.13‐1.15 |
Peptic ulcer disease excluding bleeding | 376 | 160 | 70 | 1.12 | 1.07‐1.18 |
Valvular disease | 38,396 | 48,220 | 44 | 0.93 | 0.92‐0.94 |
Weight loss | 25,724 | 19,408 | 57 | 1.09 | 1.08‐1.10 |
Primary discharge diagnosesb | |||||
Cancer | 13,986 | 5182 | 73 | 1.20 | 1.19‐1.21 |
Musculoskeletal injuries | 14,638 | 2160 | 87 | 2.02 | 2.002.04 |
Pain‐related diagnosesc | 64,656 | 36,877 | 64 | 1.20 | 1.20‐1.21 |
Alcohol‐related disorders | 3425 | 13,352 | 20 | 0.46 | 0.44‐0.47 |
Substance‐related disorders | 8680 | 5017 | 63 | 1.03 | 1.01‐1.04 |
Psychiatric disorders | 7253 | 33,900 | 18 | 0.37 | 0.36‐0.38 |
Mood disorders | 5943 | 22,818 | 21 | ||
Schizophrenia and other psychotic disorders | 1310 | 11,082 | 11 | ||
Proceduresb | |||||
Cardiovascular procedures | 50,997 | 8904 | 85 | 1.80 | 1.79‐1.81 |
GI procedures | 27,206 | 4018 | 87 | 1.70 | 1.69‐1.71 |
Mechanical ventilation | 5341 | 2512 | 68 | 1.37 | 1.34‐1.39 |
Hospital characteristics | |||||
Number of beds | |||||
<200 | 100,900 | 88,439 | 53 | (ref) | |
201300 | 104,213 | 99,995 | 51 | 0.95 | 0.95‐0.96 |
301500 | 215,340 | 209,104 | 51 | 0.94 | 0.94‐0.95 |
>500 | 155,920 | 165,508 | 49 | 0.96 | 0.95‐0.96 |
Population served | |||||
Urban | 511,727 | 506,803 | 50 | (ref) | |
Rural | 64,646 | 56,243 | 53 | 0.98 | 0.97‐0.99 |
Teaching status | |||||
Nonteaching | 366,623 | 343,581 | 52 | (ref) | |
Teaching | 209,750 | 219,465 | 49 | 1.00 | 0.99‐1.01 |
US Census region | |||||
Northeast | 99,377 | 149,446 | 40 | (ref) | |
Midwest | 123,194 | 120,322 | 51 | 1.26 | (1.25‐1.27) |
South | 251,624 | 213,029 | 54 | 1.33 | (1.33‐1.34) |
West | 102,178 | 80,249 | 56 | 1.37 | (1.36‐1.38) |
Variation in Opioid Prescribing
Figure 1 shows the histograms of hospital opioid prescribing rate for the 286 hospitals in our cohort (A) before and (B) after adjustment for patient characteristics. The observed rates ranged from 5% in the lowest‐prescribing hospital to 72% in the highest‐prescribing hospital, with a mean (SD) of 51% (10%). After adjusting for patient characteristics, the adjusted opioid‐prescribing rates ranged from 33% to 64%, with a mean (SD) of 50% (4%).
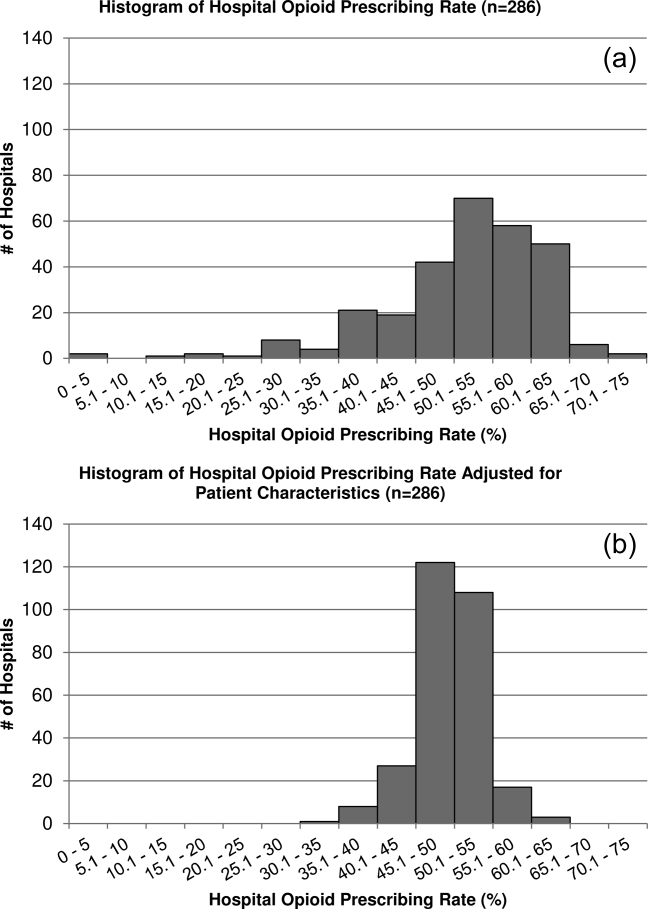
Severe Opioid‐Related Adverse Events
Among admissions with opioid exposure (n=576,373), naloxone use occurred in 2345 (0.41%) and opioid‐related adverse drug events in 1174 (0.20%), for a total of 3441 (0.60%) severe opioid‐related adverse events (some patients experienced both). Table 4 reports the opioid exposure and severe opioid‐related adverse‐event rates within hospital opioid‐prescribing rate quartiles, along with the adjusted association between the hospital opioid‐prescribing rate quartile and severe opioid‐related adverse events. After adjusting for patient characteristics, the relative risk of a severe opioid‐related adverse event was significantly greater in hospitals with higher opioid‐prescribing rates, both overall and among opioid exposed.
Quartile | No. of Patients | Opioid Exposed, n (%) | Opioid‐Related Adverse Events, n (%) | Adjusted RR in All Patients, RR (95% CI), N=1,139,419a | Adjusted RR in Opioid Exposed, RR (95% CI), N=576,373a |
---|---|---|---|---|---|
| |||||
1 | 349,747 | 132,824 (38) | 719 (0.21) | (ref) | (ref) |
2 | 266,652 | 134,590 (50) | 729 (0.27) | 1.31 (1.17‐1.45) | 1.07 (0.96‐1.18) |
3 | 251,042 | 139,770 (56) | 922 (0.37) | 1.72 (1.56‐1.90) | 1.31 (1.19‐1.44) |
4 | 271,978 | 169,189 (62) | 1071 (0.39) | 1.73 (1.57‐1.90) | 1.23 (1.12‐1.35) |
DISCUSSION
In this analysis of a large cohort of hospitalized nonsurgical patients, we found that more than half of all patients received opioids, with 43% of those exposed receiving multiple opioids during their admission and 52% receiving opioids on the day of discharge. Considerable hospital variation in opioid use was evident, and this was not fully explained by patient characteristics. Severe opioid‐related adverse events occurred more frequently at hospitals with higher opioid‐prescribing rates, and the relative risk of a severe adverse event per patient prescribed opioids was also higher in these hospitals. To our knowledge, this is the first study to describe the scope of opioid utilization and the relationship between utilization and severe opioid‐related adverse events in a sample of nonsurgical patients in US acute‐care facilities.
Our use of naloxone charges and opioid‐specific ICD‐9‐CM coding to define an opioid‐related adverse event was intended to capture only the most severe opioid‐related adverse events. We chose to focus on these events in our analysis to maximize the specificity of our outcome definition and thereby minimize confounding in our observed associations. The rate of less‐severe opioid‐related adverse events, such as nausea, constipation, and pruritis, is likely much higher and not captured in our outcome definition. Prior analyses have found variable rates of opioid‐related adverse events of approximately 1.8% to 13.6% of exposed patients.[22, 23, 24] However, these analyses focused on surgical patients and included less‐severe events. To our knowledge, ours is the first analysis of severe opioid‐related adverse events in nonsurgical patients.
Our finding that severe opioid‐related adverse events increase as opioid prescribing increases is consistent with that which has been demonstrated in the community setting, where rates of opioid‐related adverse events and mortality are higher in communities with higher levels of opioid prescribing.[2, 8, 25] This finding is expected, as greater use of a class of medications with known side effects would be expected to result in a higher overall rate of adverse events. More concerning, however, is the fact that this relationship persists when focusing exclusively on opioid‐exposed patients. Among similar patients receiving opioids at different hospitals, those hospitalized in facilities with higher opioid‐prescribing rates have higher rates of severe opioid‐related adverse events. This suggests that hospitals that use opioids more frequently do not do so more safely. Rather, the increased overall prescribing rates are associated with heightened risk for a serious adverse event per patient exposed and may reflect unsafe prescribing practices.
Furthermore, our results demonstrate both regional and hospital variation in use of opioids not fully explained by patient characteristics, similar to that which has been demonstrated for other drugs and heathcare services.[26, 27, 28, 29, 30] The implications of these findings are limited by our lack of information on pain severity or prior outpatient treatment, and our resultant inability to evaluate the appropriateness of opioid use in this analysis. Additionally, although we controlled for a large number of patient and hospital characteristics, there could be other significant predictors of use not accounted for in our analysis. However, it seems unlikely that differential pain severity or patient characteristics between patients in different regions of the country could fully explain a 37% relative difference in prescribing between the lowest‐ and highest‐prescribing regions, after accounting for the 44 patient‐level variables in our models. Whereas variation in use unrelated to patient factors could represent inappropriate prescribing practices, it could also be a marker of uncertainty regarding what constitutes appropriate prescribing and high‐quality care in this realm. Although guidelines advocate for standard pain assessments and a step‐up approach to treatment,[31, 32, 33] the lack of objective measures of pain severity and lack of evidence‐based recommendations on the use of opioids for noncancer pain[34] will almost certainly lead to persistent variation in opioid prescribing despite guideline‐driven care.
Nonetheless, our findings suggest that opportunities exist to make opioid prescribing safer in hospitalized patients. Studies aimed at elucidating the source of regional and hospital variation are necessary. Additionally, efforts should focus on identifying patient and prescribing characteristics associated with heightened risk of opioid‐related adverse events. Prior studies have demonstrated that the risks of opioid medications increase with increasing age of the patient.[35, 36] Although opioid use in our cohort declined with age, 44% of admissions age 65 years had charges for opioid medications. Studies in outpatients have also demonstrated that the risks of opioid overdose and overdose‐related death increase with dose.[5, 7] One study demonstrated a 3.7‐fold increased risk of overdose at doses of 5099 mg/day in oral morphine equivalents, and an 8.9‐fold increased risk at doses of 100 mg/day, compared with doses of 20 mg/day.[7] The prevalence of high dose exposure observed in our cohort, coupled with the older age of hospitalized patients, suggests potential targets for promoting safer use in hospitalized patients through interventions such as computerized decision support and enhanced monitoring in those at highest risk.
Because medications after discharge were unavailable in our dataset, the percentage of patients given a prescription for opioid medication on discharge is unknown. However, given that opioids are often tapered rather than abruptly discontinued, our finding that 26% of hospitalized nonsurgical patients received opioids on the day of discharge suggests that a substantial proportion of patients may be discharged with a prescription for opioid medication. Given the possibility of coexistent outpatient opioid prescriptions, these findings draw attention to the importance of assuring development and streamlined accessibility of data from state prescription drug monitoring programs and suggest that increased attention should be paid to the role that inpatient opioid prescribing plays in the increased rates of chronic opioid use and overdose‐related deaths in the United States.
There are additional limitations to our analysis. First, although the database used for this analysis captures a large proportion of admissions to US acute‐care facilities and is similar in composition, it is possible that participating medical centers differ from nonparticipating medical centers in ways that could be associated with opioid prescribing. Additionally, although Premier performs extensive validation and correction processes to assure the quality of their data, there is still likely to be a small amount of random error in the database, which could particularly impact dosage calculations. The lack of preadmission medications in our database precluded identification of the proportion of patients newly started on opioid medications. Lastly, it is possible that the hospital prescribing‐rate quartile is associated with patient characteristics unaccounted for in our analysis, and, therefore, the possibility of residual confounding still exists.
In conclusion, the majority of hospitalized nonsurgical patients are exposed to opioid medications during the course of their hospitalizations, often at high doses. More than half of those exposed are still receiving these medications on the day of discharge. We found hospital and regional variation in opioid use that was not fully explained by patient characteristics, and higher levels of hospital use were associated with higher risk of severe opioid‐related adverse events in opioid‐exposed patients. Further research is necessary to investigate the appropriateness of opioid use in this patient population, the sources of variation in use, and the predictors of opioid‐related adverse events in hospitalized patients to allow development of interventions to make hospital use safer.
Disclosures
Disclosures: Dr. Herzig had full access to all of the data in the study and takes responsibility for the integrity of the data and the accuracy of the data analysis. Dr. Herzig was funded by grant no. K23AG042459 from the National Institute on Aging. Dr. Marcantonio was funded by grant nos. P01AG031720, R01AG030618, R03AG028189, and K24AG035075 from the National Institute on Aging. The funding organization had no involvement in any aspect of the study, including design, conduct, and reporting of the study. None of the authors have any conflicts of interest to disclose.
- A flood of opioids, a rising tide of deaths. N Engl J Med. 2010;363(21):1981–1985. .
- Increasing deaths from opioid analgesics in the United States. Pharmacoepidemiol Drug Saf. 2006;15(9):618–627. , , .
- Trends in opioid prescribing by race/ethnicity for patients seeking care in US emergency departments. JAMA. 2008;299(1):70–78. , , , .
- Trends in medical use and abuse of opioid analgesics. JAMA. 2000;283(13):1710–1714. , , , .
- Association between opioid prescribing patterns and opioid overdose‐related deaths. JAMA. 2011;305(13):1315–1321. , , , et al.
- Prescription opioid mortality trends in New York City, 1990–2006: examining the emergence of an epidemic. Drug Alcohol Depend. 2013;132(1‐2):53–62. , , , et al.
- Opioid prescriptions for chronic pain and overdose: a cohort study. Ann Intern Med. 2010;152(2):85–92. , , , et al.
- Relationship of opioid prescription sales and overdoses, North Carolina. Drug Alcohol Depend. 2013;132(1‐2):81–86. , , , et al.
- Deaths from prescription opioids soar in New York. BMJ. 2013;346:f921.
- Mortality in elderly dementia patients treated with risperidone. J Clin Psychopharmacol. 2006;26(6):566–570. , , .
- Medication reconciliation: a practical tool to reduce the risk of medication errors. J Crit Care. 2003;18(4):201–205. , , , et al.
- Adverse drug event trigger tool: a practical methodology for measuring medication related harm. Qual Saf Health Care. 2003;12(3):194–200. , , .
- Improvement in the detection of adverse drug events by the use of electronic health and prescription records: an evaluation of two trigger tools. Eur J Clin Pharmacol. 2013;69(2):255–259. , , , , .
- Adverse Drug Events in U.S. Hospitals, 2004. Rockville, MD: Agency for Healthcare Research and Quality; April 2007. Statistical Brief 29. , .
- Medication‐Related Adverse Outcomes in U.S. Hospitals and Emergency Departments, 2008. Rockville, MD: Agency for Healthcare Research and Quality; April 2011. Statistical Brief 109. , , .
- US Centers for Medicare and Medicaid Services. Hospital‐Acquired Conditions (Present on Admission Indicator). Available at: http://www.cms.hhs.gov/HospitalAcqCond/. Accessed August 16, 2011. Updated September 20, 2012.
- Fracture risk associated with the use of morphine and opiates. J Intern Med. 2006;260(1):76–87. , , .
- Comorbidity measures for use with administrative data. Med Care. 1998;36(1):8–27. , , , .
- Clinical Classifications Software, Healthcare Cost and Utilization Project (HCUP). Rockville, MD; Agency for Healthcare Research and Quality; December 2009.
- Clinical application of opioid equianalgesic data. Clin J Pain. 2003;19(5)286–297. , , , , .
- Choosing the unit of measurement counts: the use of oral morphine equivalents in studies of opioid consumption is a useful addition to defined daily doses. Palliat Med. 2011;25(7):725–732. , , , , , .
- Cost and quality implications of opioid‐based postsurgical pain control using administrative claims data from a large health system: opioid‐related adverse events and their impact on clinical and economic outcomes. Pharmacotherapy. 2013;33(4):383–391. , , , .
- Opioid‐related adverse drug events in surgical hospitalizations: impact on costs and length of stay. Ann Pharmacother. 2007;41(3):400–406. , , , et al.
- Cost of opioid‐related adverse drug events in surgical patients. J Pain Symptom Manage. 2003;25(3):276–283. , , , et al.
- Opioid analgesics and rates of fatal drug poisoning in the United States. Am J Prev Med. 2006;31(6):506–511. , .
- Geographic variation in the treatment of acute myocardial infarction: the Cooperative Cardiovascular Project. JAMA. 1999;281(7):627–633. , , , et al.
- GUSTO‐1 Investigators. Regional variation across the United States in the management of acute myocardial infarction. Global Utilization of Streptokinase and Tissue Plasminogen Activator for Occluded Coronary Arteries. N Engl J Med. 1995;333(9):565–572. , , , et al;
- Predictors of broad‐spectrum antibiotic prescribing for acute respiratory tract infections in adult primary care. JAMA. 2003;289(6):719–725. , , .
- Geographic variation in Medicare drug spending. N Engl J Med. 2010;363(5):405–409. , , .
- Geographic variation in outpatient antibiotic prescribing among older adults. Arch Intern Med. 2012;172(19):1465–1471. , , .
- Clinical policy: critical issues in the prescribing of opioids for adult patients in the emergency department. Ann Emerg Med. 2012;60(4):499–525. , , , et al.
- The Joint Commission. Facts About Pain Management. Available at: http://www.jointcommission.org/pain_management/. Accessed July 23, 2012.
- The Joint Commission. Sentinel Event Alert: Safe Use of Opioids in Hospitals. Published August 8, 2012. Available at: http://www.jointcommission.org/assets/1/18/SEA_49_opioids_8_2_12_final.pdf. Accessed March 4, 2013.
- Research gaps on use of opioids for chronic noncancer pain: findings from a review of the evidence for an American Pain Society and American Academy of Pain Medicine clinical practice guideline. J Pain. 2009;10(2):147–159. , , , , .
- Side effects of opioids during short‐term administration: effect of age, gender, and race. Clin Pharmacol Ther. 2003;74(2):102–112. , , , , , .
- Postoperative day one: a high‐risk period for respiratory events. Am J Surg. 2005;190(5):752–756. , , , .
- A flood of opioids, a rising tide of deaths. N Engl J Med. 2010;363(21):1981–1985. .
- Increasing deaths from opioid analgesics in the United States. Pharmacoepidemiol Drug Saf. 2006;15(9):618–627. , , .
- Trends in opioid prescribing by race/ethnicity for patients seeking care in US emergency departments. JAMA. 2008;299(1):70–78. , , , .
- Trends in medical use and abuse of opioid analgesics. JAMA. 2000;283(13):1710–1714. , , , .
- Association between opioid prescribing patterns and opioid overdose‐related deaths. JAMA. 2011;305(13):1315–1321. , , , et al.
- Prescription opioid mortality trends in New York City, 1990–2006: examining the emergence of an epidemic. Drug Alcohol Depend. 2013;132(1‐2):53–62. , , , et al.
- Opioid prescriptions for chronic pain and overdose: a cohort study. Ann Intern Med. 2010;152(2):85–92. , , , et al.
- Relationship of opioid prescription sales and overdoses, North Carolina. Drug Alcohol Depend. 2013;132(1‐2):81–86. , , , et al.
- Deaths from prescription opioids soar in New York. BMJ. 2013;346:f921.
- Mortality in elderly dementia patients treated with risperidone. J Clin Psychopharmacol. 2006;26(6):566–570. , , .
- Medication reconciliation: a practical tool to reduce the risk of medication errors. J Crit Care. 2003;18(4):201–205. , , , et al.
- Adverse drug event trigger tool: a practical methodology for measuring medication related harm. Qual Saf Health Care. 2003;12(3):194–200. , , .
- Improvement in the detection of adverse drug events by the use of electronic health and prescription records: an evaluation of two trigger tools. Eur J Clin Pharmacol. 2013;69(2):255–259. , , , , .
- Adverse Drug Events in U.S. Hospitals, 2004. Rockville, MD: Agency for Healthcare Research and Quality; April 2007. Statistical Brief 29. , .
- Medication‐Related Adverse Outcomes in U.S. Hospitals and Emergency Departments, 2008. Rockville, MD: Agency for Healthcare Research and Quality; April 2011. Statistical Brief 109. , , .
- US Centers for Medicare and Medicaid Services. Hospital‐Acquired Conditions (Present on Admission Indicator). Available at: http://www.cms.hhs.gov/HospitalAcqCond/. Accessed August 16, 2011. Updated September 20, 2012.
- Fracture risk associated with the use of morphine and opiates. J Intern Med. 2006;260(1):76–87. , , .
- Comorbidity measures for use with administrative data. Med Care. 1998;36(1):8–27. , , , .
- Clinical Classifications Software, Healthcare Cost and Utilization Project (HCUP). Rockville, MD; Agency for Healthcare Research and Quality; December 2009.
- Clinical application of opioid equianalgesic data. Clin J Pain. 2003;19(5)286–297. , , , , .
- Choosing the unit of measurement counts: the use of oral morphine equivalents in studies of opioid consumption is a useful addition to defined daily doses. Palliat Med. 2011;25(7):725–732. , , , , , .
- Cost and quality implications of opioid‐based postsurgical pain control using administrative claims data from a large health system: opioid‐related adverse events and their impact on clinical and economic outcomes. Pharmacotherapy. 2013;33(4):383–391. , , , .
- Opioid‐related adverse drug events in surgical hospitalizations: impact on costs and length of stay. Ann Pharmacother. 2007;41(3):400–406. , , , et al.
- Cost of opioid‐related adverse drug events in surgical patients. J Pain Symptom Manage. 2003;25(3):276–283. , , , et al.
- Opioid analgesics and rates of fatal drug poisoning in the United States. Am J Prev Med. 2006;31(6):506–511. , .
- Geographic variation in the treatment of acute myocardial infarction: the Cooperative Cardiovascular Project. JAMA. 1999;281(7):627–633. , , , et al.
- GUSTO‐1 Investigators. Regional variation across the United States in the management of acute myocardial infarction. Global Utilization of Streptokinase and Tissue Plasminogen Activator for Occluded Coronary Arteries. N Engl J Med. 1995;333(9):565–572. , , , et al;
- Predictors of broad‐spectrum antibiotic prescribing for acute respiratory tract infections in adult primary care. JAMA. 2003;289(6):719–725. , , .
- Geographic variation in Medicare drug spending. N Engl J Med. 2010;363(5):405–409. , , .
- Geographic variation in outpatient antibiotic prescribing among older adults. Arch Intern Med. 2012;172(19):1465–1471. , , .
- Clinical policy: critical issues in the prescribing of opioids for adult patients in the emergency department. Ann Emerg Med. 2012;60(4):499–525. , , , et al.
- The Joint Commission. Facts About Pain Management. Available at: http://www.jointcommission.org/pain_management/. Accessed July 23, 2012.
- The Joint Commission. Sentinel Event Alert: Safe Use of Opioids in Hospitals. Published August 8, 2012. Available at: http://www.jointcommission.org/assets/1/18/SEA_49_opioids_8_2_12_final.pdf. Accessed March 4, 2013.
- Research gaps on use of opioids for chronic noncancer pain: findings from a review of the evidence for an American Pain Society and American Academy of Pain Medicine clinical practice guideline. J Pain. 2009;10(2):147–159. , , , , .
- Side effects of opioids during short‐term administration: effect of age, gender, and race. Clin Pharmacol Ther. 2003;74(2):102–112. , , , , , .
- Postoperative day one: a high‐risk period for respiratory events. Am J Surg. 2005;190(5):752–756. , , , .
© 2013 Society of Hospital Medicine