User login
Readmission Rates and Mortality Measures
The Centers for Medicare & Medicaid Services (CMS) have sought to reduce readmissions in the 30 days following hospital discharge through penalties applied to hospitals with readmission rates that are higher than expected. Expected readmission rates for Medicare fee‐for‐service beneficiaries are calculated from models that use patient‐level administrative data to account for patient morbidities. Readmitted patients are defined as those who are discharged from the hospital alive and then rehospitalized at any acute care facility within 30 days of discharge. These models explicitly exclude sociodemographic variables that may impact quality of and access to outpatient care. Specific exclusions are also applied based on diagnosis codes so as to avoid penalizing hospitals for rehospitalizations that are likely to have been planned.
More recently, a hospital‐wide readmission measure has been developed, which seeks to provide a comprehensive view of each hospital's readmission rate by including the vast majority of Medicare patients. Like the condition‐specific readmission measures, the hospital‐wide readmission measure also excludes sociodemographic variables and incorporates specific condition‐based exclusions so as to avoid counting planned rehospitalizations (e.g., an admission for cholecystectomy following an admission for biliary sepsis). Although not currently used for pay‐for‐performance, this measure has been included in the CMS Star Report along with other readmission measures.[1] CMS does not currently disseminate a hospital‐wide mortality measure, but does disseminate hospital‐level adjusted 30‐day mortality rates for Medicare beneficiaries with discharge diagnoses of stroke, heart failure, myocardial infarction (MI), chronic obstructive pulmonary disease (COPD) and pneumonia, and principal procedure of coronary artery bypass grafting (CABG).
It is conceivable that aggressive efforts to reduce readmissions might delay life‐saving acute care in some scenarios,[2] and there is prior evidence that heart failure readmissions are inversely (but weakly) related to heart failure mortality.[3] It is also plausible that keeping tenuous patients alive until discharge might result in higher readmission rates. We sought to examine the relationship between hospital‐wide adjusted 30‐day readmissions and death rates across the acute care hospitals in the United States. Lacking a measure of hospital‐wide death rates, we examined the relation between hospital‐wide readmissions and each of the 6 condition‐specific mortality measures. For comparison, we also examined the relationships between condition‐specific readmission rates and mortality rates.
METHODS
We used publically available data published by CMS from July 1, 2011 through June 30, 2014.[4] These data are provided at the hospital level, without any patient‐level data. We included 4452 acute care facilities based on having hospital‐wide readmission rates, but not all facilities contributed data for each mortality measure. We excluded from analysis on a measure‐by‐measure basis those facilities for which outcomes were absent, without imputing missing outcome measures, because low volume of a given condition was the main reason for not reporting a measure. For each mortality measure, we constructed a logistic regression model to quantify the odds of performing in the lowest (best) mortality tertile as a function of hospital‐wide readmission tertile. To account for patient volumes, we included in each model the number of eligible patients at each hospital with the specified condition. We repeated these analyses using condition‐specific readmission rates (rather than the hospital‐wide readmission rates) as the independent variable. Specifications for CMS models for mortality and readmissions are publically available.[5]
RESULTS
After adjustment for patient volumes, hospitals in the highest hospital‐wide readmission tertile were more likely to perform in the lowest (best) mortality tertile for 3 of the 6 mortality measures: heart failure, COPD, and stroke (P < 0.001 for all). For MI, CABG and pneumonia, there was no significant association between high hospital‐wide readmission rates and low mortality (Table 1). Using condition‐specific readmission rates, there remained an inverse association between readmissions and mortality for heart failure and stroke, but not for COPD. In contrast, hospitals with the highest CABG‐specific readmission rates were significantly less likely to have low CABG‐specific mortality (P < 0.001).
Hospital‐Wide Readmission Rate Tertile [Range of Adjusted Readmission Rates, %]* | |||
---|---|---|---|
1st Tertile, n = 1359 [11.3%‐14.8%], Adjusted Odds Ratio (95% CI) |
2nd Tertile, n = 1785 [14.9%‐15.5%], Adjusted Odds Ratio (95% CI) |
3rd Tertile, n = 1308 [15.6%‐19.8%], Adjusted Odds Ratio (95% CI) |
|
| |||
Mortality measure (no. of hospitals reporting) | |||
Acute myocardial infarction (n = 2415) | 1.00 (referent) | 0.88 (0.711.09) | 1.02 (0.831.25) |
Pneumonia (n = 4067) | 1.00 (referent) | 0.83 (0.710.98) | 1.11 (0.941.31) |
Heart failure (n = 3668) | 1.00 (referent) | 1.21 (1.021.45) | 1.94 (1.632.30) |
Stroke (n = 2754) | 1.00 (referent) | 1.13 (0.931.38) | 1.48 (1.221.79) |
Chronic obstructive pulmonary disease (n = 3633) | 1.00 (referent) | 1.12 (0.951.33) | 1.73 (1.462.05) |
Coronary artery bypass (n = 1058) | 1.00 (referent) | 0.87 (0.631.19) | 0.99 (0.741.34) |
Condition‐specific readmission rate tertile | |||
Mortality measure | |||
Acute myocardial infarction | 1.00 (referent) | 0.88 (0.711.08) | 0.79 (0.640.99) |
Pneumonia | 1.00 (referent) | 0.91 (0.781.07) | 0.89 (0.761.04) |
Heart failure | 1.00 (referent) | 1.15 (0.961.36) | 1.56 (1.311.86) |
Stroke | 1.00 (referent) | 1.65 (1.342.03) | 1.70 (1.232.35) |
Chronic obstructive pulmonary disease | 1.00 (referent) | 0.83 (0.700.98) | 0.84 (0.710.99) |
Coronary artery bypass | 1.00 (referent) | 0.59 (0.440.80) | 0.47 (0.340.64) |
DISCUSSION
We found that higher hospital‐wide readmission rates were associated with lower mortality at the hospital level for 3 of the 6 mortality measures we examined. The findings for heart failure parallel the findings of Krumholz and colleagues who examined 3 of these 6 measures (MI, pneumonia, and heart failure) in relation to readmissions for these specific populations.[3] This prior analysis, however, did not include the 3 more recently reported mortality measures (COPD, stroke, and CABG) and did not use hospital‐wide readmissions.
Causal mechanisms underlying the associations between mortality and readmission at the hospital level deserve further exploration. It is certainly possible that global efforts to keep patients out of the hospital might, in some instances, place patients at risk by delaying necessary acute care.[2] It is also possible that unmeasured variables, particularly access to hospice and palliative care services that might facilitate good deaths, could be associated with both reduced readmissions and higher death rates. Additionally, because deceased patients cannot be readmitted, one might expect that readmissions and mortality might be inversely associated, particularly for conditions with a high postdischarge mortality rate. Similarly, a hospital that does a particularly good job keeping chronically ill patients alive until discharge might exhibit a higher readmission rate than a hospital that is less adept at keeping tenuous patients alive until discharge.
Regardless of the mechanisms of these findings, we present these data to raise the concern that using readmission rates, particularly hospital‐wide readmission rates, as a measure of hospital quality is inherently problematic. It is particularly problematic that CMS has applied equal weight to readmissions and mortality in the Star Report.[1] High readmission rates may result from complications and poor handoffs, but may also stem from the legitimate need to care for chronically ill patients in a high‐intensity setting, particularly fragile patients who have been kept alive against the odds. In conclusion, caution is warranted in viewing readmissions as a quality metric until the associations we describe are better explained using patient‐level data and more robust adjustment than is possible with these publically available data.
Disclosures: Dr. Daniel J. Brotman had full access to the data in the study and takes responsibility for the integrity of the data and the accuracy of the data analysis. There was no financial support for this work. Contributions of the authors are as follows: drafting manuscript (Brotman), revision of manuscript for important intellectual content (brotman, Hoyer, Lepley, Deutschendorf, Leung), acquisition of data (Deutschendorf, Leung, Lepley), interpretation of data (Brotman, Hoyer, Lepley, Deutschendorf, Leung), data analysis (Brotman, Hoyer).
- Centers for Medicare and Medicaid Services. Available at: https://www.cms.gov/Outreach-and-Education/Outreach/NPC/Downloads/2015-08-13-Star-Ratings-Presentation.pdf. Accessed September 2015.
- A comprehensive care management program to prevent chronic obstructive pulmonary disease hospitalizations: a randomized, controlled trial. Ann Intern Med. 2012;156(10):673–683. , , , et al.
- Relationship between hospital readmission and mortality rates for patients hospitalized with acute myocardial infarction, heart failure, or pneumonia. JAMA. 2013;309(6):587–593. , , , et al.
- Centers for Medicare and Medicaid Services. Hospital compare datasets. Available at: https://data.medicare.gov/data/hospital‐compare. Accessed September 2015.
- Centers for Medicare and Medicaid Services. Hospital quality initiative. Available at: https://www.cms.gov/Medicare/Quality‐Initiatives‐Patient‐Assessment‐Instruments/HospitalQualityInits. Accessed September 2015.
The Centers for Medicare & Medicaid Services (CMS) have sought to reduce readmissions in the 30 days following hospital discharge through penalties applied to hospitals with readmission rates that are higher than expected. Expected readmission rates for Medicare fee‐for‐service beneficiaries are calculated from models that use patient‐level administrative data to account for patient morbidities. Readmitted patients are defined as those who are discharged from the hospital alive and then rehospitalized at any acute care facility within 30 days of discharge. These models explicitly exclude sociodemographic variables that may impact quality of and access to outpatient care. Specific exclusions are also applied based on diagnosis codes so as to avoid penalizing hospitals for rehospitalizations that are likely to have been planned.
More recently, a hospital‐wide readmission measure has been developed, which seeks to provide a comprehensive view of each hospital's readmission rate by including the vast majority of Medicare patients. Like the condition‐specific readmission measures, the hospital‐wide readmission measure also excludes sociodemographic variables and incorporates specific condition‐based exclusions so as to avoid counting planned rehospitalizations (e.g., an admission for cholecystectomy following an admission for biliary sepsis). Although not currently used for pay‐for‐performance, this measure has been included in the CMS Star Report along with other readmission measures.[1] CMS does not currently disseminate a hospital‐wide mortality measure, but does disseminate hospital‐level adjusted 30‐day mortality rates for Medicare beneficiaries with discharge diagnoses of stroke, heart failure, myocardial infarction (MI), chronic obstructive pulmonary disease (COPD) and pneumonia, and principal procedure of coronary artery bypass grafting (CABG).
It is conceivable that aggressive efforts to reduce readmissions might delay life‐saving acute care in some scenarios,[2] and there is prior evidence that heart failure readmissions are inversely (but weakly) related to heart failure mortality.[3] It is also plausible that keeping tenuous patients alive until discharge might result in higher readmission rates. We sought to examine the relationship between hospital‐wide adjusted 30‐day readmissions and death rates across the acute care hospitals in the United States. Lacking a measure of hospital‐wide death rates, we examined the relation between hospital‐wide readmissions and each of the 6 condition‐specific mortality measures. For comparison, we also examined the relationships between condition‐specific readmission rates and mortality rates.
METHODS
We used publically available data published by CMS from July 1, 2011 through June 30, 2014.[4] These data are provided at the hospital level, without any patient‐level data. We included 4452 acute care facilities based on having hospital‐wide readmission rates, but not all facilities contributed data for each mortality measure. We excluded from analysis on a measure‐by‐measure basis those facilities for which outcomes were absent, without imputing missing outcome measures, because low volume of a given condition was the main reason for not reporting a measure. For each mortality measure, we constructed a logistic regression model to quantify the odds of performing in the lowest (best) mortality tertile as a function of hospital‐wide readmission tertile. To account for patient volumes, we included in each model the number of eligible patients at each hospital with the specified condition. We repeated these analyses using condition‐specific readmission rates (rather than the hospital‐wide readmission rates) as the independent variable. Specifications for CMS models for mortality and readmissions are publically available.[5]
RESULTS
After adjustment for patient volumes, hospitals in the highest hospital‐wide readmission tertile were more likely to perform in the lowest (best) mortality tertile for 3 of the 6 mortality measures: heart failure, COPD, and stroke (P < 0.001 for all). For MI, CABG and pneumonia, there was no significant association between high hospital‐wide readmission rates and low mortality (Table 1). Using condition‐specific readmission rates, there remained an inverse association between readmissions and mortality for heart failure and stroke, but not for COPD. In contrast, hospitals with the highest CABG‐specific readmission rates were significantly less likely to have low CABG‐specific mortality (P < 0.001).
Hospital‐Wide Readmission Rate Tertile [Range of Adjusted Readmission Rates, %]* | |||
---|---|---|---|
1st Tertile, n = 1359 [11.3%‐14.8%], Adjusted Odds Ratio (95% CI) |
2nd Tertile, n = 1785 [14.9%‐15.5%], Adjusted Odds Ratio (95% CI) |
3rd Tertile, n = 1308 [15.6%‐19.8%], Adjusted Odds Ratio (95% CI) |
|
| |||
Mortality measure (no. of hospitals reporting) | |||
Acute myocardial infarction (n = 2415) | 1.00 (referent) | 0.88 (0.711.09) | 1.02 (0.831.25) |
Pneumonia (n = 4067) | 1.00 (referent) | 0.83 (0.710.98) | 1.11 (0.941.31) |
Heart failure (n = 3668) | 1.00 (referent) | 1.21 (1.021.45) | 1.94 (1.632.30) |
Stroke (n = 2754) | 1.00 (referent) | 1.13 (0.931.38) | 1.48 (1.221.79) |
Chronic obstructive pulmonary disease (n = 3633) | 1.00 (referent) | 1.12 (0.951.33) | 1.73 (1.462.05) |
Coronary artery bypass (n = 1058) | 1.00 (referent) | 0.87 (0.631.19) | 0.99 (0.741.34) |
Condition‐specific readmission rate tertile | |||
Mortality measure | |||
Acute myocardial infarction | 1.00 (referent) | 0.88 (0.711.08) | 0.79 (0.640.99) |
Pneumonia | 1.00 (referent) | 0.91 (0.781.07) | 0.89 (0.761.04) |
Heart failure | 1.00 (referent) | 1.15 (0.961.36) | 1.56 (1.311.86) |
Stroke | 1.00 (referent) | 1.65 (1.342.03) | 1.70 (1.232.35) |
Chronic obstructive pulmonary disease | 1.00 (referent) | 0.83 (0.700.98) | 0.84 (0.710.99) |
Coronary artery bypass | 1.00 (referent) | 0.59 (0.440.80) | 0.47 (0.340.64) |
DISCUSSION
We found that higher hospital‐wide readmission rates were associated with lower mortality at the hospital level for 3 of the 6 mortality measures we examined. The findings for heart failure parallel the findings of Krumholz and colleagues who examined 3 of these 6 measures (MI, pneumonia, and heart failure) in relation to readmissions for these specific populations.[3] This prior analysis, however, did not include the 3 more recently reported mortality measures (COPD, stroke, and CABG) and did not use hospital‐wide readmissions.
Causal mechanisms underlying the associations between mortality and readmission at the hospital level deserve further exploration. It is certainly possible that global efforts to keep patients out of the hospital might, in some instances, place patients at risk by delaying necessary acute care.[2] It is also possible that unmeasured variables, particularly access to hospice and palliative care services that might facilitate good deaths, could be associated with both reduced readmissions and higher death rates. Additionally, because deceased patients cannot be readmitted, one might expect that readmissions and mortality might be inversely associated, particularly for conditions with a high postdischarge mortality rate. Similarly, a hospital that does a particularly good job keeping chronically ill patients alive until discharge might exhibit a higher readmission rate than a hospital that is less adept at keeping tenuous patients alive until discharge.
Regardless of the mechanisms of these findings, we present these data to raise the concern that using readmission rates, particularly hospital‐wide readmission rates, as a measure of hospital quality is inherently problematic. It is particularly problematic that CMS has applied equal weight to readmissions and mortality in the Star Report.[1] High readmission rates may result from complications and poor handoffs, but may also stem from the legitimate need to care for chronically ill patients in a high‐intensity setting, particularly fragile patients who have been kept alive against the odds. In conclusion, caution is warranted in viewing readmissions as a quality metric until the associations we describe are better explained using patient‐level data and more robust adjustment than is possible with these publically available data.
Disclosures: Dr. Daniel J. Brotman had full access to the data in the study and takes responsibility for the integrity of the data and the accuracy of the data analysis. There was no financial support for this work. Contributions of the authors are as follows: drafting manuscript (Brotman), revision of manuscript for important intellectual content (brotman, Hoyer, Lepley, Deutschendorf, Leung), acquisition of data (Deutschendorf, Leung, Lepley), interpretation of data (Brotman, Hoyer, Lepley, Deutschendorf, Leung), data analysis (Brotman, Hoyer).
The Centers for Medicare & Medicaid Services (CMS) have sought to reduce readmissions in the 30 days following hospital discharge through penalties applied to hospitals with readmission rates that are higher than expected. Expected readmission rates for Medicare fee‐for‐service beneficiaries are calculated from models that use patient‐level administrative data to account for patient morbidities. Readmitted patients are defined as those who are discharged from the hospital alive and then rehospitalized at any acute care facility within 30 days of discharge. These models explicitly exclude sociodemographic variables that may impact quality of and access to outpatient care. Specific exclusions are also applied based on diagnosis codes so as to avoid penalizing hospitals for rehospitalizations that are likely to have been planned.
More recently, a hospital‐wide readmission measure has been developed, which seeks to provide a comprehensive view of each hospital's readmission rate by including the vast majority of Medicare patients. Like the condition‐specific readmission measures, the hospital‐wide readmission measure also excludes sociodemographic variables and incorporates specific condition‐based exclusions so as to avoid counting planned rehospitalizations (e.g., an admission for cholecystectomy following an admission for biliary sepsis). Although not currently used for pay‐for‐performance, this measure has been included in the CMS Star Report along with other readmission measures.[1] CMS does not currently disseminate a hospital‐wide mortality measure, but does disseminate hospital‐level adjusted 30‐day mortality rates for Medicare beneficiaries with discharge diagnoses of stroke, heart failure, myocardial infarction (MI), chronic obstructive pulmonary disease (COPD) and pneumonia, and principal procedure of coronary artery bypass grafting (CABG).
It is conceivable that aggressive efforts to reduce readmissions might delay life‐saving acute care in some scenarios,[2] and there is prior evidence that heart failure readmissions are inversely (but weakly) related to heart failure mortality.[3] It is also plausible that keeping tenuous patients alive until discharge might result in higher readmission rates. We sought to examine the relationship between hospital‐wide adjusted 30‐day readmissions and death rates across the acute care hospitals in the United States. Lacking a measure of hospital‐wide death rates, we examined the relation between hospital‐wide readmissions and each of the 6 condition‐specific mortality measures. For comparison, we also examined the relationships between condition‐specific readmission rates and mortality rates.
METHODS
We used publically available data published by CMS from July 1, 2011 through June 30, 2014.[4] These data are provided at the hospital level, without any patient‐level data. We included 4452 acute care facilities based on having hospital‐wide readmission rates, but not all facilities contributed data for each mortality measure. We excluded from analysis on a measure‐by‐measure basis those facilities for which outcomes were absent, without imputing missing outcome measures, because low volume of a given condition was the main reason for not reporting a measure. For each mortality measure, we constructed a logistic regression model to quantify the odds of performing in the lowest (best) mortality tertile as a function of hospital‐wide readmission tertile. To account for patient volumes, we included in each model the number of eligible patients at each hospital with the specified condition. We repeated these analyses using condition‐specific readmission rates (rather than the hospital‐wide readmission rates) as the independent variable. Specifications for CMS models for mortality and readmissions are publically available.[5]
RESULTS
After adjustment for patient volumes, hospitals in the highest hospital‐wide readmission tertile were more likely to perform in the lowest (best) mortality tertile for 3 of the 6 mortality measures: heart failure, COPD, and stroke (P < 0.001 for all). For MI, CABG and pneumonia, there was no significant association between high hospital‐wide readmission rates and low mortality (Table 1). Using condition‐specific readmission rates, there remained an inverse association between readmissions and mortality for heart failure and stroke, but not for COPD. In contrast, hospitals with the highest CABG‐specific readmission rates were significantly less likely to have low CABG‐specific mortality (P < 0.001).
Hospital‐Wide Readmission Rate Tertile [Range of Adjusted Readmission Rates, %]* | |||
---|---|---|---|
1st Tertile, n = 1359 [11.3%‐14.8%], Adjusted Odds Ratio (95% CI) |
2nd Tertile, n = 1785 [14.9%‐15.5%], Adjusted Odds Ratio (95% CI) |
3rd Tertile, n = 1308 [15.6%‐19.8%], Adjusted Odds Ratio (95% CI) |
|
| |||
Mortality measure (no. of hospitals reporting) | |||
Acute myocardial infarction (n = 2415) | 1.00 (referent) | 0.88 (0.711.09) | 1.02 (0.831.25) |
Pneumonia (n = 4067) | 1.00 (referent) | 0.83 (0.710.98) | 1.11 (0.941.31) |
Heart failure (n = 3668) | 1.00 (referent) | 1.21 (1.021.45) | 1.94 (1.632.30) |
Stroke (n = 2754) | 1.00 (referent) | 1.13 (0.931.38) | 1.48 (1.221.79) |
Chronic obstructive pulmonary disease (n = 3633) | 1.00 (referent) | 1.12 (0.951.33) | 1.73 (1.462.05) |
Coronary artery bypass (n = 1058) | 1.00 (referent) | 0.87 (0.631.19) | 0.99 (0.741.34) |
Condition‐specific readmission rate tertile | |||
Mortality measure | |||
Acute myocardial infarction | 1.00 (referent) | 0.88 (0.711.08) | 0.79 (0.640.99) |
Pneumonia | 1.00 (referent) | 0.91 (0.781.07) | 0.89 (0.761.04) |
Heart failure | 1.00 (referent) | 1.15 (0.961.36) | 1.56 (1.311.86) |
Stroke | 1.00 (referent) | 1.65 (1.342.03) | 1.70 (1.232.35) |
Chronic obstructive pulmonary disease | 1.00 (referent) | 0.83 (0.700.98) | 0.84 (0.710.99) |
Coronary artery bypass | 1.00 (referent) | 0.59 (0.440.80) | 0.47 (0.340.64) |
DISCUSSION
We found that higher hospital‐wide readmission rates were associated with lower mortality at the hospital level for 3 of the 6 mortality measures we examined. The findings for heart failure parallel the findings of Krumholz and colleagues who examined 3 of these 6 measures (MI, pneumonia, and heart failure) in relation to readmissions for these specific populations.[3] This prior analysis, however, did not include the 3 more recently reported mortality measures (COPD, stroke, and CABG) and did not use hospital‐wide readmissions.
Causal mechanisms underlying the associations between mortality and readmission at the hospital level deserve further exploration. It is certainly possible that global efforts to keep patients out of the hospital might, in some instances, place patients at risk by delaying necessary acute care.[2] It is also possible that unmeasured variables, particularly access to hospice and palliative care services that might facilitate good deaths, could be associated with both reduced readmissions and higher death rates. Additionally, because deceased patients cannot be readmitted, one might expect that readmissions and mortality might be inversely associated, particularly for conditions with a high postdischarge mortality rate. Similarly, a hospital that does a particularly good job keeping chronically ill patients alive until discharge might exhibit a higher readmission rate than a hospital that is less adept at keeping tenuous patients alive until discharge.
Regardless of the mechanisms of these findings, we present these data to raise the concern that using readmission rates, particularly hospital‐wide readmission rates, as a measure of hospital quality is inherently problematic. It is particularly problematic that CMS has applied equal weight to readmissions and mortality in the Star Report.[1] High readmission rates may result from complications and poor handoffs, but may also stem from the legitimate need to care for chronically ill patients in a high‐intensity setting, particularly fragile patients who have been kept alive against the odds. In conclusion, caution is warranted in viewing readmissions as a quality metric until the associations we describe are better explained using patient‐level data and more robust adjustment than is possible with these publically available data.
Disclosures: Dr. Daniel J. Brotman had full access to the data in the study and takes responsibility for the integrity of the data and the accuracy of the data analysis. There was no financial support for this work. Contributions of the authors are as follows: drafting manuscript (Brotman), revision of manuscript for important intellectual content (brotman, Hoyer, Lepley, Deutschendorf, Leung), acquisition of data (Deutschendorf, Leung, Lepley), interpretation of data (Brotman, Hoyer, Lepley, Deutschendorf, Leung), data analysis (Brotman, Hoyer).
- Centers for Medicare and Medicaid Services. Available at: https://www.cms.gov/Outreach-and-Education/Outreach/NPC/Downloads/2015-08-13-Star-Ratings-Presentation.pdf. Accessed September 2015.
- A comprehensive care management program to prevent chronic obstructive pulmonary disease hospitalizations: a randomized, controlled trial. Ann Intern Med. 2012;156(10):673–683. , , , et al.
- Relationship between hospital readmission and mortality rates for patients hospitalized with acute myocardial infarction, heart failure, or pneumonia. JAMA. 2013;309(6):587–593. , , , et al.
- Centers for Medicare and Medicaid Services. Hospital compare datasets. Available at: https://data.medicare.gov/data/hospital‐compare. Accessed September 2015.
- Centers for Medicare and Medicaid Services. Hospital quality initiative. Available at: https://www.cms.gov/Medicare/Quality‐Initiatives‐Patient‐Assessment‐Instruments/HospitalQualityInits. Accessed September 2015.
- Centers for Medicare and Medicaid Services. Available at: https://www.cms.gov/Outreach-and-Education/Outreach/NPC/Downloads/2015-08-13-Star-Ratings-Presentation.pdf. Accessed September 2015.
- A comprehensive care management program to prevent chronic obstructive pulmonary disease hospitalizations: a randomized, controlled trial. Ann Intern Med. 2012;156(10):673–683. , , , et al.
- Relationship between hospital readmission and mortality rates for patients hospitalized with acute myocardial infarction, heart failure, or pneumonia. JAMA. 2013;309(6):587–593. , , , et al.
- Centers for Medicare and Medicaid Services. Hospital compare datasets. Available at: https://data.medicare.gov/data/hospital‐compare. Accessed September 2015.
- Centers for Medicare and Medicaid Services. Hospital quality initiative. Available at: https://www.cms.gov/Medicare/Quality‐Initiatives‐Patient‐Assessment‐Instruments/HospitalQualityInits. Accessed September 2015.
SCHOLAR Project
The structure and function of academic hospital medicine programs (AHPs) has evolved significantly with the growth of hospital medicine.[1, 2, 3, 4] Many AHPs formed in response to regulatory and financial changes, which drove demand for increased trainee oversight, improved clinical efficiency, and growth in nonteaching services staffed by hospitalists. Differences in local organizational contexts and needs have contributed to great variability in AHP program design and operations. As AHPs have become more established, the need to engage academic hospitalists in scholarship and activities that support professional development and promotion has been recognized. Defining sustainable and successful positions for academic hospitalists is a priority called for by leaders in the field.[5, 6]
In this rapidly evolving context, AHPs have employed a variety of approaches to organizing clinical and academic faculty roles, without guiding evidence or consensus‐based performance benchmarks. A number of AHPs have achieved success along traditional academic metrics of research, scholarship, and education. Currently, it is not known whether specific approaches to AHP organization, structure, or definition of faculty roles are associated with achievement of more traditional markers of academic success.
The Academic Committee of the Society of Hospital Medicine (SHM), and the Academic Hospitalist Task Force of the Society of General Internal Medicine (SGIM) had separately initiated projects to explore characteristics associated with success in AHPs. In 2012, these organizations combined efforts to jointly develop and implement the SCHOLAR (SuCcessful HOspitaLists in Academics and Research) project. The goals were to identify successful AHPs using objective criteria, and to then study those groups in greater detail to generate insights that would be broadly relevant to the field. Efforts to clarify the factors within AHPs linked to success by traditional academic metrics will benefit hospitalists, their leaders, and key stakeholders striving to achieve optimal balance between clinical and academic roles. We describe the initial work of the SCHOLAR project, our definitions of academic success in AHPs, and the characteristics of a cohort of exemplary AHPs who achieved the highest levels on these metrics.
METHODS
Defining Success
The 11 members of the SCHOLAR project held a variety of clinical and academic roles within a geographically diverse group of AHPs. We sought to create a functional definition of success applicable to AHPs. As no gold standard currently exists, we used a consensus process among task force members to arrive at a definition that was quantifiable, feasible, and meaningful. The first step was brainstorming on conference calls held 1 to 2 times monthly over 4 months. Potential defining characteristics that emerged from these discussions related to research, teaching, and administrative activities. When potential characteristics were proposed, we considered how to operationalize each one. Each characteristic was discussed until there was consensus from the entire group. Those around education and administration were the most complex, as many roles are locally driven and defined, and challenging to quantify. For this reason, we focused on promotion as a more global approach to assessing academic hospitalist success in these areas. Although criteria for academic advancement also vary across institutions, we felt that promotion generally reflected having met some threshold of academic success. We also wanted to recognize that scholarship occurs outside the context of funded research. Ultimately, 3 key domains emerged: research grant funding, faculty promotion, and scholarship.
After these 3 domains were identified, the group sought to define quantitative metrics to assess performance. These discussions occurred on subsequent calls over a 4‐month period. Between calls, group members gathered additional information to facilitate assessment of the feasibility of proposed metrics, reporting on progress via email. Again, group consensus was sought for each metric considered. Data on grant funding and successful promotions were available from a previous survey conducted through the SHM in 2011. Leaders from 170 AHPs were contacted, with 50 providing complete responses to the 21‐item questionnaire (see Supporting Information, Appendix 1, in the online version of this article). Results of the survey, heretofore referred to as the Leaders of Academic Hospitalist Programs survey (LAHP‐50), have been described elsewhere.[7] For the purposes of this study, we used the self‐reported data about grant funding and promotions contained in the survey to reflect the current state of the field. Although the survey response rate was approximately 30%, the survey was not anonymous, and many reputationally prominent academic hospitalist programs were represented. For these reasons, the group members felt that the survey results were relevant for the purposes of assessing academic success.
In the LAHP‐50, funding was defined as principal investigator or coinvestigator roles on federally and nonfederally funded research, clinical trials, internal grants, and any other extramurally funded projects. Mean and median funding for the overall sample was calculated. Through a separate question, each program's total faculty full‐time equivalent (FTE) count was reported, allowing us to adjust for group size by assessing both total funding per group and funding/FTE for each responding AHP.
Promotions were defined by the self‐reported number of faculty at each of the following ranks: instructor, assistant professor, associate professor, full professor, and professor above scale/emeritus. In addition, a category of nonacademic track (eg, adjunct faculty, clinical associate) was included to capture hospitalists that did not fit into the traditional promotions categories. We did not distinguish between tenure‐track and nontenure‐track academic ranks. LAHP‐50 survey respondents reported the number of faculty in their group at each academic rank. Given that the majority of academic hospitalists hold a rank of assistant professor or lower,[6, 8, 9] and that the number of full professors was only 3% in the LAHP‐50 cohort, we combined the faculty at the associate and full professor ranks, defining successfully promoted faculty as the percent of hospitalists above the rank of assistant professor.
We created a new metric to assess scholarly output. We had considerable discussion of ways to assess the numbers of peer‐reviewed manuscripts generated by AHPs. However, the group had concerns about the feasibility of identification and attribution of authors to specific AHPs through literature searches. We considered examining only publications in the Journal of Hospital Medicine and the Journal of General Internal Medicine, but felt that this would exclude significant work published by hospitalists in fields of medical education or health services research that would more likely appear in alternate journals. Instead, we quantified scholarship based on the number of abstracts presented at national meetings. We focused on meetings of the SHM and SGIM as the primary professional societies representing hospital medicine. The group felt that even work published outside of the journals of our professional societies would likely be presented at those meetings. We used the following strategy: We reviewed research abstracts accepted for presentation as posters or oral abstracts at the 2010 and 2011 SHM national meetings, and research abstracts with a primary or secondary category of hospital medicine at the 2010 and 2011 SGIM national meetings. By including submissions at both SGIM and SHM meetings, we accounted for the fact that some programs may gravitate more to one society meeting or another. We did not include abstracts in the clinical vignettes or innovations categories. We tallied the number of abstracts by group affiliation of the authors for each of the 4 meetings above and created a cumulative total per group for the 2‐year period. Abstracts with authors from different AHPs were counted once for each individual group. Members of the study group reviewed abstracts from each of the meetings in pairs. Reviewers worked separately and compared tallies of results to ensure consistent tabulations. Internet searches were conducted to identify or confirm author affiliations if it was not apparent in the abstract author list. Abstract tallies were compiled without regard to whether programs had completed the LAHP‐50 survey; thus, we collected data on programs that did not respond to the LAHP‐50 survey.
Identification of the SCHOLAR Cohort
To identify our cohort of top‐performing AHPs, we combined the funding and promotions data from the LAHP‐50 sample with the abstract data. We limited our sample to adult hospital medicine groups to reduce heterogeneity. We created rank lists of programs in each category (grant funding, successful promotions, and scholarship), using data from the LAHP‐50 survey to rank programs on funding and promotions, and data from our abstract counts to rank on scholarship. We limited the top‐performing list in each category to 10 institutions as a cutoff. Because we set a threshold of at least $1 million in total funding, we identified only 9 top performing AHPs with regard to grant funding. We also calculated mean funding/FTE. We chose to rank programs only by funding/FTE rather than total funding per program to better account for group size. For successful promotions, we ranked programs by the percentage of senior faculty. For abstract counts, we included programs whose faculty presented abstracts at a minimum of 2 separate meetings, and ranked programs based on the total number of abstracts per group.
This process resulted in separate lists of top performing programs in each of the 3 domains we associated with academic success, arranged in descending order by grant dollars/FTE, percent of senior faculty, and abstract counts (Table 1). Seventeen different programs were represented across these 3 top 10 lists. One program appeared on all 3 lists, 8 programs appeared on 2 lists, and the remainder appeared on a single list (Table 2). Seven of these programs were identified solely based on abstract presentations, diversifying our top groups beyond only those who completed the LAHP‐50 survey. We considered all of these programs to represent high performance in academic hospital medicine. The group selected this inclusive approach because we recognized that any 1 metric was potentially limited, and we sought to identify diverse pathways to success.
Funding | Promotions | Scholarship | |
---|---|---|---|
Grant $/FTE | Total Grant $ | Senior Faculty, No. (%) | Total Abstract Count |
| |||
$1,409,090 | $15,500,000 | 3 (60%) | 23 |
$1,000,000 | $9,000,000 | 3 (60%) | 21 |
$750,000 | $8,000,000 | 4 (57%) | 20 |
$478,609 | $6,700,535 | 9 (53%) | 15 |
$347,826 | $3,000,000 | 8 (44%) | 11 |
$86,956 | $3,000,000 | 14 (41%) | 11 |
$66,666 | $2,000,000 | 17 (36%) | 10 |
$46,153 | $1,500,000 | 9 (33%) | 10 |
$38,461 | $1,000,000 | 2 (33%) | 9 |
4 (31%) | 9 |
Selection Criteria for SCHOLAR Cohort | No. of Programs |
---|---|
| |
Abstracts, funding, and promotions | 1 |
Abstracts plus promotions | 4 |
Abstracts plus funding | 3 |
Funding plus promotion | 1 |
Funding only | 1 |
Abstract only | 7 |
Total | 17 |
Top 10 abstract count | |
4 meetings | 2 |
3 meetings | 2 |
2 meetings | 6 |
The 17 unique adult AHPs appearing on at least 1 of the top 10 lists comprised the SCHOLAR cohort of programs that we studied in greater detail. Data reflecting program demographics were solicited directly from leaders of the AHPs identified in the SCHOLAR cohort, including size and age of program, reporting structure, number of faculty at various academic ranks (for programs that did not complete the LAHP‐50 survey), and number of faculty with fellowship training (defined as any postresidency fellowship program).
Subsequently, we performed comparative analyses between the programs in the SCHOLAR cohort to the general population of AHPs reflected by the LAHP‐50 sample. Because abstract presentations were not recorded in the original LAHP‐50 survey instrument, it was not possible to perform a benchmarking comparison for the scholarship domain.
Data Analysis
To measure the success of the SCHOLAR cohort we compared the grant funding and proportion of successfully promoted faculty at the SCHOLAR programs to those in the overall LAHP‐50 sample. Differences in mean and median grant funding were compared using t tests and Mann‐Whitney rank sum tests. Proportion of promoted faculty were compared using 2 tests. A 2‐tailed of 0.05 was used to test significance of differences.
RESULTS
Demographics
Among the AHPs in the SCHOLAR cohort, the mean program age was 13.2 years (range, 618 years), and the mean program size was 36 faculty (range, 1895; median, 28). On average, 15% of faculty members at SCHOLAR programs were fellowship trained (range, 0%37%). Reporting structure among the SCHOLAR programs was as follows: 53% were an independent division or section of the department of medicine; 29% were a section within general internal medicine, and 18% were an independent clinical group.
Grant Funding
Table 3 compares grant funding in the SCHOLAR programs to programs in the overall LAHP‐50 sample. Mean funding per group and mean funding per FTE were significantly higher in the SCHOLAR group than in the overall sample.
Funding (Millions) | ||
---|---|---|
LAHP‐50 Overall Sample | SCHOLAR | |
| ||
Median grant funding/AHP | 0.060 | 1.500* |
Mean grant funding/AHP | 1.147 (015) | 3.984* (015) |
Median grant funding/FTE | 0.004 | 0.038* |
Mean grant funding/FTE | 0.095 (01.4) | 0.364* (01.4) |
Thirteen of the SCHOLAR programs were represented in the initial LAHP‐50, but 2 did not report a dollar amount for grants and contracts. Therefore, data for total grant funding were available for only 65% (11 of 17) of the programs in the SCHOLAR cohort. Of note, 28% of AHPs in the overall LAHP‐50 sample reported no external funding sources.
Faculty Promotion
Figure 1 demonstrates the proportion of faculty at various academic ranks. The percent of faculty above the rank of assistant professor in the SCHOLAR programs exceeded those in the overall LAHP‐50 by 5% (17.9% vs 12.8%, P = 0.01). Of note, 6% of the hospitalists at AHPs in the SCHOLAR programs were on nonfaculty tracks.
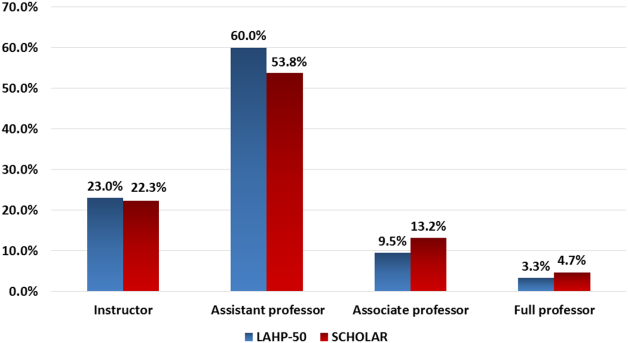
Scholarship
Mean abstract output over the 2‐year period measured was 10.8 (range, 323) in the SCHOLAR cohort. Because we did not collect these data for the LAHP‐50 group, comparative analyses were not possible.
DISCUSSION
Using a definition of academic success that incorporated metrics of grant funding, faculty promotion, and scholarly output, we identified a unique subset of successful AHPsthe SCHOLAR cohort. The programs represented in the SCHOLAR cohort were generally large and relatively mature. Despite this, the cohort consisted of mostly junior faculty, had a paucity of fellowship‐trained hospitalists, and not all reported grant funding.
Prior published work reported complementary findings.[6, 8, 9] A survey of 20 large, well‐established academic hospitalist programs in 2008 found that the majority of hospitalists were junior faculty with a limited publication portfolio. Of the 266 respondents in that study, 86% reported an academic rank at or below assistant professor; funding was not explored.[9] Our similar findings 4 years later add to this work by demonstrating trends over time, and suggest that progress toward creating successful pathways for academic advancement has been slow. In a 2012 survey of the SHM membership, 28% of hospitalists with academic appointments reported no current or future plans to engage in research.[8] These findings suggest that faculty in AHPs may define scholarship through nontraditional pathways, or in some cases choose not to pursue or prioritize scholarship altogether.
Our findings also add to the literature with regard to our assessment of funding, which was variable across the SCHOLAR group. The broad range of funding in the SCHOLAR programs for which we have data (grant dollars $0$15 million per program) suggests that opportunities to improve supported scholarship remain, even among a selected cohort of successful AHPs. The predominance of junior faculty in the SCHOLAR programs may be a reason for this variation. Junior faculty may be engaged in research with funding directed to senior mentors outside their AHP. Alternatively, they may pursue meaningful local hospital quality improvement or educational innovations not supported by external grants, or hold leadership roles in education, quality, or information technology that allow for advancement and promotion without external grant funding. As the scope and impact of these roles increases, senior leaders with alternate sources of support may rely less on research funds; this too may explain some of the differences. Our findings are congruent with results of a study that reviewed original research published by hospitalists, and concluded that the majority of hospitalist research was not externally funded.[8] Our approach for assessing grant funding by adjusting for FTE had the potential to inadvertently favor smaller well‐funded groups over larger ones; however, programs in our sample were similarly represented when ranked by funding/FTE or total grant dollars. As many successful AHPs do concentrate their research funding among a core of focused hospitalist researchers, our definition may not be the ideal metric for some programs.
We chose to define scholarship based on abstract output, rather than peer‐reviewed publications. Although this choice was necessary from a feasibility perspective, it may have excluded programs that prioritize peer‐reviewed publications over abstracts. Although we were unable to incorporate a search strategy to accurately and comprehensively track the publication output attributed specifically to hospitalist researchers and quantify it by program, others have since defined such an approach.[8] However, tracking abstracts theoretically allowed insights into a larger volume of innovative and creative work generated by top AHPs by potentially including work in the earlier stages of development.
We used a consensus‐based definition of success to define our SCHOLAR cohort. There are other ways to measure academic success, which if applied, may have yielded a different sample of programs. For example, over half of the original research articles published in the Journal of Hospital Medicine over a 7‐year span were generated from 5 academic centers.[8] This definition of success may be equally credible, though we note that 4 of these 5 programs were also included in the SCHOLAR cohort. We feel our broader approach was more reflective of the variety of pathways to success available to academic hospitalists. Before our metrics are applied as a benchmarking tool, however, they should ideally be combined with factors not measured in our study to ensure a more comprehensive or balanced reflection of academic success. Factors such as mentorship, level of hospitalist engagement,[10] prevalence of leadership opportunities, operational and fiscal infrastructure, and the impact of local quality, safety, and value efforts should be considered.
Comparison of successfully promoted faculty at AHPs across the country is inherently limited by the wide variation in promotion standards across different institutions; controlling for such differences was not possible with our methodology. For example, it appears that several programs with relatively few senior faculty may have met metrics leading to their inclusion in the SCHOLAR group because of their small program size. Future benchmarking efforts for promotion at AHPs should take scaling into account and consider both total number as well as percentage of senior faculty when evaluating success.
Our methodology has several limitations. Survey data were self‐reported and not independently validated, and as such are subject to recall and reporting biases. Response bias inherently excluded some AHPs that may have met our grant funding or promotions criteria had they participated in the initial LAHP‐50 survey, though we identified and included additional programs through our scholarship metric, increasing the representativeness of the SCHOLAR cohort. Given the dynamic nature of the field, the age of the data we relied upon for analysis limits the generalizability of our specific benchmarks to current practice. However, the development of academic success occurs over the long‐term, and published data on academic hospitalist productivity are consistent with this slower time course.[8] Despite these limitations, our data inform the general topic of gauging performance of AHPs, underscoring the challenges of developing and applying metrics of success, and highlight the variability of performance on selected metrics even among a relatively small group of 17 programs.
In conclusion, we have created a method to quantify academic success that may be useful to academic hospitalists and their group leaders as they set targets for improvement in the field. Even among our SCHOLAR cohort, room for ongoing improvement in development of funded scholarship and a core of senior faculty exists. Further investigation into the unique features of successful groups will offer insight to leaders in academic hospital medicine regarding infrastructure and processes that should be embraced to raise the bar for all AHPs. In addition, efforts to further define and validate nontraditional approaches to scholarship that allow for successful promotion at AHPs would be informative. We view our work less as a singular approach to benchmarking standards for AHPs, and more a call to action to continue efforts to balance scholarly activity and broad professional development of academic hospitalists with increasing clinical demands.
Acknowledgements
The authors thank all of the AHP leaders who participated in the SCHOLAR project. They also thank the Society of Hospital Medicine and Society of General Internal Medicine and the SHM Academic Committee and SGIM Academic Hospitalist Task Force for their support of this work.
Disclosures
The work reported here was supported by the Department of Veterans Affairs, Veterans Health Administration, South Texas Veterans Health Care System. The views expressed in this article are those of the authors and do not necessarily reflect the position or policy of the Department of Veterans Affairs. The authors report no conflicts of interest.
- Characteristics of primary care providers who adopted the hospitalist model from 2001 to 2009. J Hosp Med. 2015;10(2):75–82. , , , , .
- Growth in the care of older patients by hospitalists in the United States. N Engl J Med. 2009;360(11):1102–1112. , , , .
- Updating threshold‐based identification of hospitalists in 2012 Medicare pay data. J Hosp Med. 2016;11(1):45–47. , , , , .
- Use of hospitalists by Medicare beneficiaries: a national picture. Medicare Medicaid Res Rev. 2014;4(2). , , , .
- Challenges and opportunities in Academic Hospital Medicine: report from the Academic Hospital Medicine Summit. J Hosp Med. 2009;4(4):240–246. , , , , , .
- Survey of US academic hospitalist leaders about mentorship and academic activities in hospitalist groups. J Hosp Med. 2011;6(1):5–9. , , , .
- The structure of hospital medicine programs at academic medical centers [abstract]. J Hosp Med. 2012;7(suppl 2):s92. , , , , , .
- Research and publication trends in hospital medicine. J Hosp Med. 2014;9(3):148–154. , , , , .
- Mentorship, productivity, and promotion among academic hospitalists. J Gen Intern Med. 2012;27(1):23–27. , , , , , .
- The key principles and characteristics of an effective hospital medicine group: an assessment guide for hospitals and hospitalists. J Hosp Med. 2014;9(2):123–128. , , , et al.
The structure and function of academic hospital medicine programs (AHPs) has evolved significantly with the growth of hospital medicine.[1, 2, 3, 4] Many AHPs formed in response to regulatory and financial changes, which drove demand for increased trainee oversight, improved clinical efficiency, and growth in nonteaching services staffed by hospitalists. Differences in local organizational contexts and needs have contributed to great variability in AHP program design and operations. As AHPs have become more established, the need to engage academic hospitalists in scholarship and activities that support professional development and promotion has been recognized. Defining sustainable and successful positions for academic hospitalists is a priority called for by leaders in the field.[5, 6]
In this rapidly evolving context, AHPs have employed a variety of approaches to organizing clinical and academic faculty roles, without guiding evidence or consensus‐based performance benchmarks. A number of AHPs have achieved success along traditional academic metrics of research, scholarship, and education. Currently, it is not known whether specific approaches to AHP organization, structure, or definition of faculty roles are associated with achievement of more traditional markers of academic success.
The Academic Committee of the Society of Hospital Medicine (SHM), and the Academic Hospitalist Task Force of the Society of General Internal Medicine (SGIM) had separately initiated projects to explore characteristics associated with success in AHPs. In 2012, these organizations combined efforts to jointly develop and implement the SCHOLAR (SuCcessful HOspitaLists in Academics and Research) project. The goals were to identify successful AHPs using objective criteria, and to then study those groups in greater detail to generate insights that would be broadly relevant to the field. Efforts to clarify the factors within AHPs linked to success by traditional academic metrics will benefit hospitalists, their leaders, and key stakeholders striving to achieve optimal balance between clinical and academic roles. We describe the initial work of the SCHOLAR project, our definitions of academic success in AHPs, and the characteristics of a cohort of exemplary AHPs who achieved the highest levels on these metrics.
METHODS
Defining Success
The 11 members of the SCHOLAR project held a variety of clinical and academic roles within a geographically diverse group of AHPs. We sought to create a functional definition of success applicable to AHPs. As no gold standard currently exists, we used a consensus process among task force members to arrive at a definition that was quantifiable, feasible, and meaningful. The first step was brainstorming on conference calls held 1 to 2 times monthly over 4 months. Potential defining characteristics that emerged from these discussions related to research, teaching, and administrative activities. When potential characteristics were proposed, we considered how to operationalize each one. Each characteristic was discussed until there was consensus from the entire group. Those around education and administration were the most complex, as many roles are locally driven and defined, and challenging to quantify. For this reason, we focused on promotion as a more global approach to assessing academic hospitalist success in these areas. Although criteria for academic advancement also vary across institutions, we felt that promotion generally reflected having met some threshold of academic success. We also wanted to recognize that scholarship occurs outside the context of funded research. Ultimately, 3 key domains emerged: research grant funding, faculty promotion, and scholarship.
After these 3 domains were identified, the group sought to define quantitative metrics to assess performance. These discussions occurred on subsequent calls over a 4‐month period. Between calls, group members gathered additional information to facilitate assessment of the feasibility of proposed metrics, reporting on progress via email. Again, group consensus was sought for each metric considered. Data on grant funding and successful promotions were available from a previous survey conducted through the SHM in 2011. Leaders from 170 AHPs were contacted, with 50 providing complete responses to the 21‐item questionnaire (see Supporting Information, Appendix 1, in the online version of this article). Results of the survey, heretofore referred to as the Leaders of Academic Hospitalist Programs survey (LAHP‐50), have been described elsewhere.[7] For the purposes of this study, we used the self‐reported data about grant funding and promotions contained in the survey to reflect the current state of the field. Although the survey response rate was approximately 30%, the survey was not anonymous, and many reputationally prominent academic hospitalist programs were represented. For these reasons, the group members felt that the survey results were relevant for the purposes of assessing academic success.
In the LAHP‐50, funding was defined as principal investigator or coinvestigator roles on federally and nonfederally funded research, clinical trials, internal grants, and any other extramurally funded projects. Mean and median funding for the overall sample was calculated. Through a separate question, each program's total faculty full‐time equivalent (FTE) count was reported, allowing us to adjust for group size by assessing both total funding per group and funding/FTE for each responding AHP.
Promotions were defined by the self‐reported number of faculty at each of the following ranks: instructor, assistant professor, associate professor, full professor, and professor above scale/emeritus. In addition, a category of nonacademic track (eg, adjunct faculty, clinical associate) was included to capture hospitalists that did not fit into the traditional promotions categories. We did not distinguish between tenure‐track and nontenure‐track academic ranks. LAHP‐50 survey respondents reported the number of faculty in their group at each academic rank. Given that the majority of academic hospitalists hold a rank of assistant professor or lower,[6, 8, 9] and that the number of full professors was only 3% in the LAHP‐50 cohort, we combined the faculty at the associate and full professor ranks, defining successfully promoted faculty as the percent of hospitalists above the rank of assistant professor.
We created a new metric to assess scholarly output. We had considerable discussion of ways to assess the numbers of peer‐reviewed manuscripts generated by AHPs. However, the group had concerns about the feasibility of identification and attribution of authors to specific AHPs through literature searches. We considered examining only publications in the Journal of Hospital Medicine and the Journal of General Internal Medicine, but felt that this would exclude significant work published by hospitalists in fields of medical education or health services research that would more likely appear in alternate journals. Instead, we quantified scholarship based on the number of abstracts presented at national meetings. We focused on meetings of the SHM and SGIM as the primary professional societies representing hospital medicine. The group felt that even work published outside of the journals of our professional societies would likely be presented at those meetings. We used the following strategy: We reviewed research abstracts accepted for presentation as posters or oral abstracts at the 2010 and 2011 SHM national meetings, and research abstracts with a primary or secondary category of hospital medicine at the 2010 and 2011 SGIM national meetings. By including submissions at both SGIM and SHM meetings, we accounted for the fact that some programs may gravitate more to one society meeting or another. We did not include abstracts in the clinical vignettes or innovations categories. We tallied the number of abstracts by group affiliation of the authors for each of the 4 meetings above and created a cumulative total per group for the 2‐year period. Abstracts with authors from different AHPs were counted once for each individual group. Members of the study group reviewed abstracts from each of the meetings in pairs. Reviewers worked separately and compared tallies of results to ensure consistent tabulations. Internet searches were conducted to identify or confirm author affiliations if it was not apparent in the abstract author list. Abstract tallies were compiled without regard to whether programs had completed the LAHP‐50 survey; thus, we collected data on programs that did not respond to the LAHP‐50 survey.
Identification of the SCHOLAR Cohort
To identify our cohort of top‐performing AHPs, we combined the funding and promotions data from the LAHP‐50 sample with the abstract data. We limited our sample to adult hospital medicine groups to reduce heterogeneity. We created rank lists of programs in each category (grant funding, successful promotions, and scholarship), using data from the LAHP‐50 survey to rank programs on funding and promotions, and data from our abstract counts to rank on scholarship. We limited the top‐performing list in each category to 10 institutions as a cutoff. Because we set a threshold of at least $1 million in total funding, we identified only 9 top performing AHPs with regard to grant funding. We also calculated mean funding/FTE. We chose to rank programs only by funding/FTE rather than total funding per program to better account for group size. For successful promotions, we ranked programs by the percentage of senior faculty. For abstract counts, we included programs whose faculty presented abstracts at a minimum of 2 separate meetings, and ranked programs based on the total number of abstracts per group.
This process resulted in separate lists of top performing programs in each of the 3 domains we associated with academic success, arranged in descending order by grant dollars/FTE, percent of senior faculty, and abstract counts (Table 1). Seventeen different programs were represented across these 3 top 10 lists. One program appeared on all 3 lists, 8 programs appeared on 2 lists, and the remainder appeared on a single list (Table 2). Seven of these programs were identified solely based on abstract presentations, diversifying our top groups beyond only those who completed the LAHP‐50 survey. We considered all of these programs to represent high performance in academic hospital medicine. The group selected this inclusive approach because we recognized that any 1 metric was potentially limited, and we sought to identify diverse pathways to success.
Funding | Promotions | Scholarship | |
---|---|---|---|
Grant $/FTE | Total Grant $ | Senior Faculty, No. (%) | Total Abstract Count |
| |||
$1,409,090 | $15,500,000 | 3 (60%) | 23 |
$1,000,000 | $9,000,000 | 3 (60%) | 21 |
$750,000 | $8,000,000 | 4 (57%) | 20 |
$478,609 | $6,700,535 | 9 (53%) | 15 |
$347,826 | $3,000,000 | 8 (44%) | 11 |
$86,956 | $3,000,000 | 14 (41%) | 11 |
$66,666 | $2,000,000 | 17 (36%) | 10 |
$46,153 | $1,500,000 | 9 (33%) | 10 |
$38,461 | $1,000,000 | 2 (33%) | 9 |
4 (31%) | 9 |
Selection Criteria for SCHOLAR Cohort | No. of Programs |
---|---|
| |
Abstracts, funding, and promotions | 1 |
Abstracts plus promotions | 4 |
Abstracts plus funding | 3 |
Funding plus promotion | 1 |
Funding only | 1 |
Abstract only | 7 |
Total | 17 |
Top 10 abstract count | |
4 meetings | 2 |
3 meetings | 2 |
2 meetings | 6 |
The 17 unique adult AHPs appearing on at least 1 of the top 10 lists comprised the SCHOLAR cohort of programs that we studied in greater detail. Data reflecting program demographics were solicited directly from leaders of the AHPs identified in the SCHOLAR cohort, including size and age of program, reporting structure, number of faculty at various academic ranks (for programs that did not complete the LAHP‐50 survey), and number of faculty with fellowship training (defined as any postresidency fellowship program).
Subsequently, we performed comparative analyses between the programs in the SCHOLAR cohort to the general population of AHPs reflected by the LAHP‐50 sample. Because abstract presentations were not recorded in the original LAHP‐50 survey instrument, it was not possible to perform a benchmarking comparison for the scholarship domain.
Data Analysis
To measure the success of the SCHOLAR cohort we compared the grant funding and proportion of successfully promoted faculty at the SCHOLAR programs to those in the overall LAHP‐50 sample. Differences in mean and median grant funding were compared using t tests and Mann‐Whitney rank sum tests. Proportion of promoted faculty were compared using 2 tests. A 2‐tailed of 0.05 was used to test significance of differences.
RESULTS
Demographics
Among the AHPs in the SCHOLAR cohort, the mean program age was 13.2 years (range, 618 years), and the mean program size was 36 faculty (range, 1895; median, 28). On average, 15% of faculty members at SCHOLAR programs were fellowship trained (range, 0%37%). Reporting structure among the SCHOLAR programs was as follows: 53% were an independent division or section of the department of medicine; 29% were a section within general internal medicine, and 18% were an independent clinical group.
Grant Funding
Table 3 compares grant funding in the SCHOLAR programs to programs in the overall LAHP‐50 sample. Mean funding per group and mean funding per FTE were significantly higher in the SCHOLAR group than in the overall sample.
Funding (Millions) | ||
---|---|---|
LAHP‐50 Overall Sample | SCHOLAR | |
| ||
Median grant funding/AHP | 0.060 | 1.500* |
Mean grant funding/AHP | 1.147 (015) | 3.984* (015) |
Median grant funding/FTE | 0.004 | 0.038* |
Mean grant funding/FTE | 0.095 (01.4) | 0.364* (01.4) |
Thirteen of the SCHOLAR programs were represented in the initial LAHP‐50, but 2 did not report a dollar amount for grants and contracts. Therefore, data for total grant funding were available for only 65% (11 of 17) of the programs in the SCHOLAR cohort. Of note, 28% of AHPs in the overall LAHP‐50 sample reported no external funding sources.
Faculty Promotion
Figure 1 demonstrates the proportion of faculty at various academic ranks. The percent of faculty above the rank of assistant professor in the SCHOLAR programs exceeded those in the overall LAHP‐50 by 5% (17.9% vs 12.8%, P = 0.01). Of note, 6% of the hospitalists at AHPs in the SCHOLAR programs were on nonfaculty tracks.
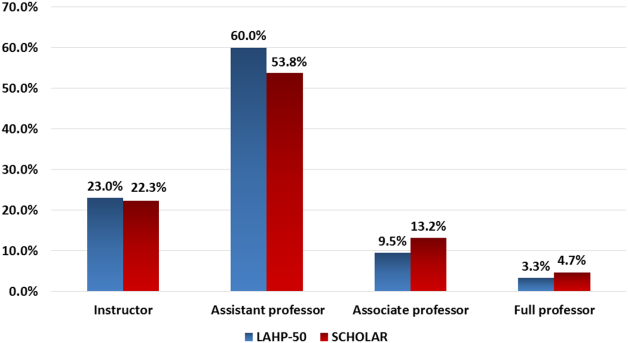
Scholarship
Mean abstract output over the 2‐year period measured was 10.8 (range, 323) in the SCHOLAR cohort. Because we did not collect these data for the LAHP‐50 group, comparative analyses were not possible.
DISCUSSION
Using a definition of academic success that incorporated metrics of grant funding, faculty promotion, and scholarly output, we identified a unique subset of successful AHPsthe SCHOLAR cohort. The programs represented in the SCHOLAR cohort were generally large and relatively mature. Despite this, the cohort consisted of mostly junior faculty, had a paucity of fellowship‐trained hospitalists, and not all reported grant funding.
Prior published work reported complementary findings.[6, 8, 9] A survey of 20 large, well‐established academic hospitalist programs in 2008 found that the majority of hospitalists were junior faculty with a limited publication portfolio. Of the 266 respondents in that study, 86% reported an academic rank at or below assistant professor; funding was not explored.[9] Our similar findings 4 years later add to this work by demonstrating trends over time, and suggest that progress toward creating successful pathways for academic advancement has been slow. In a 2012 survey of the SHM membership, 28% of hospitalists with academic appointments reported no current or future plans to engage in research.[8] These findings suggest that faculty in AHPs may define scholarship through nontraditional pathways, or in some cases choose not to pursue or prioritize scholarship altogether.
Our findings also add to the literature with regard to our assessment of funding, which was variable across the SCHOLAR group. The broad range of funding in the SCHOLAR programs for which we have data (grant dollars $0$15 million per program) suggests that opportunities to improve supported scholarship remain, even among a selected cohort of successful AHPs. The predominance of junior faculty in the SCHOLAR programs may be a reason for this variation. Junior faculty may be engaged in research with funding directed to senior mentors outside their AHP. Alternatively, they may pursue meaningful local hospital quality improvement or educational innovations not supported by external grants, or hold leadership roles in education, quality, or information technology that allow for advancement and promotion without external grant funding. As the scope and impact of these roles increases, senior leaders with alternate sources of support may rely less on research funds; this too may explain some of the differences. Our findings are congruent with results of a study that reviewed original research published by hospitalists, and concluded that the majority of hospitalist research was not externally funded.[8] Our approach for assessing grant funding by adjusting for FTE had the potential to inadvertently favor smaller well‐funded groups over larger ones; however, programs in our sample were similarly represented when ranked by funding/FTE or total grant dollars. As many successful AHPs do concentrate their research funding among a core of focused hospitalist researchers, our definition may not be the ideal metric for some programs.
We chose to define scholarship based on abstract output, rather than peer‐reviewed publications. Although this choice was necessary from a feasibility perspective, it may have excluded programs that prioritize peer‐reviewed publications over abstracts. Although we were unable to incorporate a search strategy to accurately and comprehensively track the publication output attributed specifically to hospitalist researchers and quantify it by program, others have since defined such an approach.[8] However, tracking abstracts theoretically allowed insights into a larger volume of innovative and creative work generated by top AHPs by potentially including work in the earlier stages of development.
We used a consensus‐based definition of success to define our SCHOLAR cohort. There are other ways to measure academic success, which if applied, may have yielded a different sample of programs. For example, over half of the original research articles published in the Journal of Hospital Medicine over a 7‐year span were generated from 5 academic centers.[8] This definition of success may be equally credible, though we note that 4 of these 5 programs were also included in the SCHOLAR cohort. We feel our broader approach was more reflective of the variety of pathways to success available to academic hospitalists. Before our metrics are applied as a benchmarking tool, however, they should ideally be combined with factors not measured in our study to ensure a more comprehensive or balanced reflection of academic success. Factors such as mentorship, level of hospitalist engagement,[10] prevalence of leadership opportunities, operational and fiscal infrastructure, and the impact of local quality, safety, and value efforts should be considered.
Comparison of successfully promoted faculty at AHPs across the country is inherently limited by the wide variation in promotion standards across different institutions; controlling for such differences was not possible with our methodology. For example, it appears that several programs with relatively few senior faculty may have met metrics leading to their inclusion in the SCHOLAR group because of their small program size. Future benchmarking efforts for promotion at AHPs should take scaling into account and consider both total number as well as percentage of senior faculty when evaluating success.
Our methodology has several limitations. Survey data were self‐reported and not independently validated, and as such are subject to recall and reporting biases. Response bias inherently excluded some AHPs that may have met our grant funding or promotions criteria had they participated in the initial LAHP‐50 survey, though we identified and included additional programs through our scholarship metric, increasing the representativeness of the SCHOLAR cohort. Given the dynamic nature of the field, the age of the data we relied upon for analysis limits the generalizability of our specific benchmarks to current practice. However, the development of academic success occurs over the long‐term, and published data on academic hospitalist productivity are consistent with this slower time course.[8] Despite these limitations, our data inform the general topic of gauging performance of AHPs, underscoring the challenges of developing and applying metrics of success, and highlight the variability of performance on selected metrics even among a relatively small group of 17 programs.
In conclusion, we have created a method to quantify academic success that may be useful to academic hospitalists and their group leaders as they set targets for improvement in the field. Even among our SCHOLAR cohort, room for ongoing improvement in development of funded scholarship and a core of senior faculty exists. Further investigation into the unique features of successful groups will offer insight to leaders in academic hospital medicine regarding infrastructure and processes that should be embraced to raise the bar for all AHPs. In addition, efforts to further define and validate nontraditional approaches to scholarship that allow for successful promotion at AHPs would be informative. We view our work less as a singular approach to benchmarking standards for AHPs, and more a call to action to continue efforts to balance scholarly activity and broad professional development of academic hospitalists with increasing clinical demands.
Acknowledgements
The authors thank all of the AHP leaders who participated in the SCHOLAR project. They also thank the Society of Hospital Medicine and Society of General Internal Medicine and the SHM Academic Committee and SGIM Academic Hospitalist Task Force for their support of this work.
Disclosures
The work reported here was supported by the Department of Veterans Affairs, Veterans Health Administration, South Texas Veterans Health Care System. The views expressed in this article are those of the authors and do not necessarily reflect the position or policy of the Department of Veterans Affairs. The authors report no conflicts of interest.
The structure and function of academic hospital medicine programs (AHPs) has evolved significantly with the growth of hospital medicine.[1, 2, 3, 4] Many AHPs formed in response to regulatory and financial changes, which drove demand for increased trainee oversight, improved clinical efficiency, and growth in nonteaching services staffed by hospitalists. Differences in local organizational contexts and needs have contributed to great variability in AHP program design and operations. As AHPs have become more established, the need to engage academic hospitalists in scholarship and activities that support professional development and promotion has been recognized. Defining sustainable and successful positions for academic hospitalists is a priority called for by leaders in the field.[5, 6]
In this rapidly evolving context, AHPs have employed a variety of approaches to organizing clinical and academic faculty roles, without guiding evidence or consensus‐based performance benchmarks. A number of AHPs have achieved success along traditional academic metrics of research, scholarship, and education. Currently, it is not known whether specific approaches to AHP organization, structure, or definition of faculty roles are associated with achievement of more traditional markers of academic success.
The Academic Committee of the Society of Hospital Medicine (SHM), and the Academic Hospitalist Task Force of the Society of General Internal Medicine (SGIM) had separately initiated projects to explore characteristics associated with success in AHPs. In 2012, these organizations combined efforts to jointly develop and implement the SCHOLAR (SuCcessful HOspitaLists in Academics and Research) project. The goals were to identify successful AHPs using objective criteria, and to then study those groups in greater detail to generate insights that would be broadly relevant to the field. Efforts to clarify the factors within AHPs linked to success by traditional academic metrics will benefit hospitalists, their leaders, and key stakeholders striving to achieve optimal balance between clinical and academic roles. We describe the initial work of the SCHOLAR project, our definitions of academic success in AHPs, and the characteristics of a cohort of exemplary AHPs who achieved the highest levels on these metrics.
METHODS
Defining Success
The 11 members of the SCHOLAR project held a variety of clinical and academic roles within a geographically diverse group of AHPs. We sought to create a functional definition of success applicable to AHPs. As no gold standard currently exists, we used a consensus process among task force members to arrive at a definition that was quantifiable, feasible, and meaningful. The first step was brainstorming on conference calls held 1 to 2 times monthly over 4 months. Potential defining characteristics that emerged from these discussions related to research, teaching, and administrative activities. When potential characteristics were proposed, we considered how to operationalize each one. Each characteristic was discussed until there was consensus from the entire group. Those around education and administration were the most complex, as many roles are locally driven and defined, and challenging to quantify. For this reason, we focused on promotion as a more global approach to assessing academic hospitalist success in these areas. Although criteria for academic advancement also vary across institutions, we felt that promotion generally reflected having met some threshold of academic success. We also wanted to recognize that scholarship occurs outside the context of funded research. Ultimately, 3 key domains emerged: research grant funding, faculty promotion, and scholarship.
After these 3 domains were identified, the group sought to define quantitative metrics to assess performance. These discussions occurred on subsequent calls over a 4‐month period. Between calls, group members gathered additional information to facilitate assessment of the feasibility of proposed metrics, reporting on progress via email. Again, group consensus was sought for each metric considered. Data on grant funding and successful promotions were available from a previous survey conducted through the SHM in 2011. Leaders from 170 AHPs were contacted, with 50 providing complete responses to the 21‐item questionnaire (see Supporting Information, Appendix 1, in the online version of this article). Results of the survey, heretofore referred to as the Leaders of Academic Hospitalist Programs survey (LAHP‐50), have been described elsewhere.[7] For the purposes of this study, we used the self‐reported data about grant funding and promotions contained in the survey to reflect the current state of the field. Although the survey response rate was approximately 30%, the survey was not anonymous, and many reputationally prominent academic hospitalist programs were represented. For these reasons, the group members felt that the survey results were relevant for the purposes of assessing academic success.
In the LAHP‐50, funding was defined as principal investigator or coinvestigator roles on federally and nonfederally funded research, clinical trials, internal grants, and any other extramurally funded projects. Mean and median funding for the overall sample was calculated. Through a separate question, each program's total faculty full‐time equivalent (FTE) count was reported, allowing us to adjust for group size by assessing both total funding per group and funding/FTE for each responding AHP.
Promotions were defined by the self‐reported number of faculty at each of the following ranks: instructor, assistant professor, associate professor, full professor, and professor above scale/emeritus. In addition, a category of nonacademic track (eg, adjunct faculty, clinical associate) was included to capture hospitalists that did not fit into the traditional promotions categories. We did not distinguish between tenure‐track and nontenure‐track academic ranks. LAHP‐50 survey respondents reported the number of faculty in their group at each academic rank. Given that the majority of academic hospitalists hold a rank of assistant professor or lower,[6, 8, 9] and that the number of full professors was only 3% in the LAHP‐50 cohort, we combined the faculty at the associate and full professor ranks, defining successfully promoted faculty as the percent of hospitalists above the rank of assistant professor.
We created a new metric to assess scholarly output. We had considerable discussion of ways to assess the numbers of peer‐reviewed manuscripts generated by AHPs. However, the group had concerns about the feasibility of identification and attribution of authors to specific AHPs through literature searches. We considered examining only publications in the Journal of Hospital Medicine and the Journal of General Internal Medicine, but felt that this would exclude significant work published by hospitalists in fields of medical education or health services research that would more likely appear in alternate journals. Instead, we quantified scholarship based on the number of abstracts presented at national meetings. We focused on meetings of the SHM and SGIM as the primary professional societies representing hospital medicine. The group felt that even work published outside of the journals of our professional societies would likely be presented at those meetings. We used the following strategy: We reviewed research abstracts accepted for presentation as posters or oral abstracts at the 2010 and 2011 SHM national meetings, and research abstracts with a primary or secondary category of hospital medicine at the 2010 and 2011 SGIM national meetings. By including submissions at both SGIM and SHM meetings, we accounted for the fact that some programs may gravitate more to one society meeting or another. We did not include abstracts in the clinical vignettes or innovations categories. We tallied the number of abstracts by group affiliation of the authors for each of the 4 meetings above and created a cumulative total per group for the 2‐year period. Abstracts with authors from different AHPs were counted once for each individual group. Members of the study group reviewed abstracts from each of the meetings in pairs. Reviewers worked separately and compared tallies of results to ensure consistent tabulations. Internet searches were conducted to identify or confirm author affiliations if it was not apparent in the abstract author list. Abstract tallies were compiled without regard to whether programs had completed the LAHP‐50 survey; thus, we collected data on programs that did not respond to the LAHP‐50 survey.
Identification of the SCHOLAR Cohort
To identify our cohort of top‐performing AHPs, we combined the funding and promotions data from the LAHP‐50 sample with the abstract data. We limited our sample to adult hospital medicine groups to reduce heterogeneity. We created rank lists of programs in each category (grant funding, successful promotions, and scholarship), using data from the LAHP‐50 survey to rank programs on funding and promotions, and data from our abstract counts to rank on scholarship. We limited the top‐performing list in each category to 10 institutions as a cutoff. Because we set a threshold of at least $1 million in total funding, we identified only 9 top performing AHPs with regard to grant funding. We also calculated mean funding/FTE. We chose to rank programs only by funding/FTE rather than total funding per program to better account for group size. For successful promotions, we ranked programs by the percentage of senior faculty. For abstract counts, we included programs whose faculty presented abstracts at a minimum of 2 separate meetings, and ranked programs based on the total number of abstracts per group.
This process resulted in separate lists of top performing programs in each of the 3 domains we associated with academic success, arranged in descending order by grant dollars/FTE, percent of senior faculty, and abstract counts (Table 1). Seventeen different programs were represented across these 3 top 10 lists. One program appeared on all 3 lists, 8 programs appeared on 2 lists, and the remainder appeared on a single list (Table 2). Seven of these programs were identified solely based on abstract presentations, diversifying our top groups beyond only those who completed the LAHP‐50 survey. We considered all of these programs to represent high performance in academic hospital medicine. The group selected this inclusive approach because we recognized that any 1 metric was potentially limited, and we sought to identify diverse pathways to success.
Funding | Promotions | Scholarship | |
---|---|---|---|
Grant $/FTE | Total Grant $ | Senior Faculty, No. (%) | Total Abstract Count |
| |||
$1,409,090 | $15,500,000 | 3 (60%) | 23 |
$1,000,000 | $9,000,000 | 3 (60%) | 21 |
$750,000 | $8,000,000 | 4 (57%) | 20 |
$478,609 | $6,700,535 | 9 (53%) | 15 |
$347,826 | $3,000,000 | 8 (44%) | 11 |
$86,956 | $3,000,000 | 14 (41%) | 11 |
$66,666 | $2,000,000 | 17 (36%) | 10 |
$46,153 | $1,500,000 | 9 (33%) | 10 |
$38,461 | $1,000,000 | 2 (33%) | 9 |
4 (31%) | 9 |
Selection Criteria for SCHOLAR Cohort | No. of Programs |
---|---|
| |
Abstracts, funding, and promotions | 1 |
Abstracts plus promotions | 4 |
Abstracts plus funding | 3 |
Funding plus promotion | 1 |
Funding only | 1 |
Abstract only | 7 |
Total | 17 |
Top 10 abstract count | |
4 meetings | 2 |
3 meetings | 2 |
2 meetings | 6 |
The 17 unique adult AHPs appearing on at least 1 of the top 10 lists comprised the SCHOLAR cohort of programs that we studied in greater detail. Data reflecting program demographics were solicited directly from leaders of the AHPs identified in the SCHOLAR cohort, including size and age of program, reporting structure, number of faculty at various academic ranks (for programs that did not complete the LAHP‐50 survey), and number of faculty with fellowship training (defined as any postresidency fellowship program).
Subsequently, we performed comparative analyses between the programs in the SCHOLAR cohort to the general population of AHPs reflected by the LAHP‐50 sample. Because abstract presentations were not recorded in the original LAHP‐50 survey instrument, it was not possible to perform a benchmarking comparison for the scholarship domain.
Data Analysis
To measure the success of the SCHOLAR cohort we compared the grant funding and proportion of successfully promoted faculty at the SCHOLAR programs to those in the overall LAHP‐50 sample. Differences in mean and median grant funding were compared using t tests and Mann‐Whitney rank sum tests. Proportion of promoted faculty were compared using 2 tests. A 2‐tailed of 0.05 was used to test significance of differences.
RESULTS
Demographics
Among the AHPs in the SCHOLAR cohort, the mean program age was 13.2 years (range, 618 years), and the mean program size was 36 faculty (range, 1895; median, 28). On average, 15% of faculty members at SCHOLAR programs were fellowship trained (range, 0%37%). Reporting structure among the SCHOLAR programs was as follows: 53% were an independent division or section of the department of medicine; 29% were a section within general internal medicine, and 18% were an independent clinical group.
Grant Funding
Table 3 compares grant funding in the SCHOLAR programs to programs in the overall LAHP‐50 sample. Mean funding per group and mean funding per FTE were significantly higher in the SCHOLAR group than in the overall sample.
Funding (Millions) | ||
---|---|---|
LAHP‐50 Overall Sample | SCHOLAR | |
| ||
Median grant funding/AHP | 0.060 | 1.500* |
Mean grant funding/AHP | 1.147 (015) | 3.984* (015) |
Median grant funding/FTE | 0.004 | 0.038* |
Mean grant funding/FTE | 0.095 (01.4) | 0.364* (01.4) |
Thirteen of the SCHOLAR programs were represented in the initial LAHP‐50, but 2 did not report a dollar amount for grants and contracts. Therefore, data for total grant funding were available for only 65% (11 of 17) of the programs in the SCHOLAR cohort. Of note, 28% of AHPs in the overall LAHP‐50 sample reported no external funding sources.
Faculty Promotion
Figure 1 demonstrates the proportion of faculty at various academic ranks. The percent of faculty above the rank of assistant professor in the SCHOLAR programs exceeded those in the overall LAHP‐50 by 5% (17.9% vs 12.8%, P = 0.01). Of note, 6% of the hospitalists at AHPs in the SCHOLAR programs were on nonfaculty tracks.
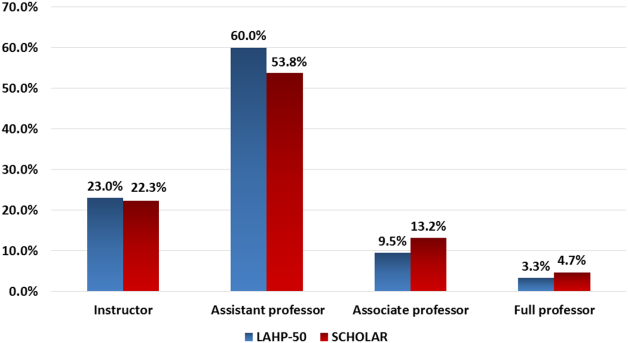
Scholarship
Mean abstract output over the 2‐year period measured was 10.8 (range, 323) in the SCHOLAR cohort. Because we did not collect these data for the LAHP‐50 group, comparative analyses were not possible.
DISCUSSION
Using a definition of academic success that incorporated metrics of grant funding, faculty promotion, and scholarly output, we identified a unique subset of successful AHPsthe SCHOLAR cohort. The programs represented in the SCHOLAR cohort were generally large and relatively mature. Despite this, the cohort consisted of mostly junior faculty, had a paucity of fellowship‐trained hospitalists, and not all reported grant funding.
Prior published work reported complementary findings.[6, 8, 9] A survey of 20 large, well‐established academic hospitalist programs in 2008 found that the majority of hospitalists were junior faculty with a limited publication portfolio. Of the 266 respondents in that study, 86% reported an academic rank at or below assistant professor; funding was not explored.[9] Our similar findings 4 years later add to this work by demonstrating trends over time, and suggest that progress toward creating successful pathways for academic advancement has been slow. In a 2012 survey of the SHM membership, 28% of hospitalists with academic appointments reported no current or future plans to engage in research.[8] These findings suggest that faculty in AHPs may define scholarship through nontraditional pathways, or in some cases choose not to pursue or prioritize scholarship altogether.
Our findings also add to the literature with regard to our assessment of funding, which was variable across the SCHOLAR group. The broad range of funding in the SCHOLAR programs for which we have data (grant dollars $0$15 million per program) suggests that opportunities to improve supported scholarship remain, even among a selected cohort of successful AHPs. The predominance of junior faculty in the SCHOLAR programs may be a reason for this variation. Junior faculty may be engaged in research with funding directed to senior mentors outside their AHP. Alternatively, they may pursue meaningful local hospital quality improvement or educational innovations not supported by external grants, or hold leadership roles in education, quality, or information technology that allow for advancement and promotion without external grant funding. As the scope and impact of these roles increases, senior leaders with alternate sources of support may rely less on research funds; this too may explain some of the differences. Our findings are congruent with results of a study that reviewed original research published by hospitalists, and concluded that the majority of hospitalist research was not externally funded.[8] Our approach for assessing grant funding by adjusting for FTE had the potential to inadvertently favor smaller well‐funded groups over larger ones; however, programs in our sample were similarly represented when ranked by funding/FTE or total grant dollars. As many successful AHPs do concentrate their research funding among a core of focused hospitalist researchers, our definition may not be the ideal metric for some programs.
We chose to define scholarship based on abstract output, rather than peer‐reviewed publications. Although this choice was necessary from a feasibility perspective, it may have excluded programs that prioritize peer‐reviewed publications over abstracts. Although we were unable to incorporate a search strategy to accurately and comprehensively track the publication output attributed specifically to hospitalist researchers and quantify it by program, others have since defined such an approach.[8] However, tracking abstracts theoretically allowed insights into a larger volume of innovative and creative work generated by top AHPs by potentially including work in the earlier stages of development.
We used a consensus‐based definition of success to define our SCHOLAR cohort. There are other ways to measure academic success, which if applied, may have yielded a different sample of programs. For example, over half of the original research articles published in the Journal of Hospital Medicine over a 7‐year span were generated from 5 academic centers.[8] This definition of success may be equally credible, though we note that 4 of these 5 programs were also included in the SCHOLAR cohort. We feel our broader approach was more reflective of the variety of pathways to success available to academic hospitalists. Before our metrics are applied as a benchmarking tool, however, they should ideally be combined with factors not measured in our study to ensure a more comprehensive or balanced reflection of academic success. Factors such as mentorship, level of hospitalist engagement,[10] prevalence of leadership opportunities, operational and fiscal infrastructure, and the impact of local quality, safety, and value efforts should be considered.
Comparison of successfully promoted faculty at AHPs across the country is inherently limited by the wide variation in promotion standards across different institutions; controlling for such differences was not possible with our methodology. For example, it appears that several programs with relatively few senior faculty may have met metrics leading to their inclusion in the SCHOLAR group because of their small program size. Future benchmarking efforts for promotion at AHPs should take scaling into account and consider both total number as well as percentage of senior faculty when evaluating success.
Our methodology has several limitations. Survey data were self‐reported and not independently validated, and as such are subject to recall and reporting biases. Response bias inherently excluded some AHPs that may have met our grant funding or promotions criteria had they participated in the initial LAHP‐50 survey, though we identified and included additional programs through our scholarship metric, increasing the representativeness of the SCHOLAR cohort. Given the dynamic nature of the field, the age of the data we relied upon for analysis limits the generalizability of our specific benchmarks to current practice. However, the development of academic success occurs over the long‐term, and published data on academic hospitalist productivity are consistent with this slower time course.[8] Despite these limitations, our data inform the general topic of gauging performance of AHPs, underscoring the challenges of developing and applying metrics of success, and highlight the variability of performance on selected metrics even among a relatively small group of 17 programs.
In conclusion, we have created a method to quantify academic success that may be useful to academic hospitalists and their group leaders as they set targets for improvement in the field. Even among our SCHOLAR cohort, room for ongoing improvement in development of funded scholarship and a core of senior faculty exists. Further investigation into the unique features of successful groups will offer insight to leaders in academic hospital medicine regarding infrastructure and processes that should be embraced to raise the bar for all AHPs. In addition, efforts to further define and validate nontraditional approaches to scholarship that allow for successful promotion at AHPs would be informative. We view our work less as a singular approach to benchmarking standards for AHPs, and more a call to action to continue efforts to balance scholarly activity and broad professional development of academic hospitalists with increasing clinical demands.
Acknowledgements
The authors thank all of the AHP leaders who participated in the SCHOLAR project. They also thank the Society of Hospital Medicine and Society of General Internal Medicine and the SHM Academic Committee and SGIM Academic Hospitalist Task Force for their support of this work.
Disclosures
The work reported here was supported by the Department of Veterans Affairs, Veterans Health Administration, South Texas Veterans Health Care System. The views expressed in this article are those of the authors and do not necessarily reflect the position or policy of the Department of Veterans Affairs. The authors report no conflicts of interest.
- Characteristics of primary care providers who adopted the hospitalist model from 2001 to 2009. J Hosp Med. 2015;10(2):75–82. , , , , .
- Growth in the care of older patients by hospitalists in the United States. N Engl J Med. 2009;360(11):1102–1112. , , , .
- Updating threshold‐based identification of hospitalists in 2012 Medicare pay data. J Hosp Med. 2016;11(1):45–47. , , , , .
- Use of hospitalists by Medicare beneficiaries: a national picture. Medicare Medicaid Res Rev. 2014;4(2). , , , .
- Challenges and opportunities in Academic Hospital Medicine: report from the Academic Hospital Medicine Summit. J Hosp Med. 2009;4(4):240–246. , , , , , .
- Survey of US academic hospitalist leaders about mentorship and academic activities in hospitalist groups. J Hosp Med. 2011;6(1):5–9. , , , .
- The structure of hospital medicine programs at academic medical centers [abstract]. J Hosp Med. 2012;7(suppl 2):s92. , , , , , .
- Research and publication trends in hospital medicine. J Hosp Med. 2014;9(3):148–154. , , , , .
- Mentorship, productivity, and promotion among academic hospitalists. J Gen Intern Med. 2012;27(1):23–27. , , , , , .
- The key principles and characteristics of an effective hospital medicine group: an assessment guide for hospitals and hospitalists. J Hosp Med. 2014;9(2):123–128. , , , et al.
- Characteristics of primary care providers who adopted the hospitalist model from 2001 to 2009. J Hosp Med. 2015;10(2):75–82. , , , , .
- Growth in the care of older patients by hospitalists in the United States. N Engl J Med. 2009;360(11):1102–1112. , , , .
- Updating threshold‐based identification of hospitalists in 2012 Medicare pay data. J Hosp Med. 2016;11(1):45–47. , , , , .
- Use of hospitalists by Medicare beneficiaries: a national picture. Medicare Medicaid Res Rev. 2014;4(2). , , , .
- Challenges and opportunities in Academic Hospital Medicine: report from the Academic Hospital Medicine Summit. J Hosp Med. 2009;4(4):240–246. , , , , , .
- Survey of US academic hospitalist leaders about mentorship and academic activities in hospitalist groups. J Hosp Med. 2011;6(1):5–9. , , , .
- The structure of hospital medicine programs at academic medical centers [abstract]. J Hosp Med. 2012;7(suppl 2):s92. , , , , , .
- Research and publication trends in hospital medicine. J Hosp Med. 2014;9(3):148–154. , , , , .
- Mentorship, productivity, and promotion among academic hospitalists. J Gen Intern Med. 2012;27(1):23–27. , , , , , .
- The key principles and characteristics of an effective hospital medicine group: an assessment guide for hospitals and hospitalists. J Hosp Med. 2014;9(2):123–128. , , , et al.
Discharge Summaries and Readmissions
Across the continuum of care, the discharge summary is a critical tool for communication among care providers.[1] In the United States, the Joint Commission policies mandate that all hospital providers complete a discharge summary for patients with specific components to foster effective communication with future providers.[2] Because outpatient providers and emergency physicians rely on clinical information in the discharge summary to ensure appropriate postdischarge continuity of care, timely documentation is potentially an essential aspect of readmission reduction initiatives.[3, 4, 5] Prior reports indicate that poor discharge documentation of follow‐up plan‐of‐care increases the risk of hospitalization, whereas structured instructions, patient education, and direct communications with primary care physicians (PCPs) reduce repeat hospital visits.[6, 7, 8, 9] However, the current literature is limited in its narrow focus on the contents of discharge summaries, considered only same‐hospital readmissions, or considered readmissions within 3 months of discharge.[10, 11, 12, 13] Moreover, some prior research has suggested no association between discharge summary timeliness with readmission,[12, 13, 14] whereas another study did find a relationship,[15] hence the need to study this further is important. Filling this gap in knowledge could provide an avenue to track and improve quality of patient care, as delays in discharge summaries have been linked with pot‐discharge adverse outcomes and patient safety concerns.[15, 16, 17, 18] Because readmissions often occur soon after discharge, having timely discharge summaries may be particularly important to outcomes.[19, 20]
This research began under the framework of evaluating a bundle of care coordination strategies that were implemented at the Johns Hopkins Health System. These strategies were informed by the early Centers for Medicare and Medicaid Services (CMS) demonstration projects and other best practices that have been documented in the literature to improve utilization and improve communication during transitions of care.[21, 22, 23, 24, 25] Later they were augmented through a contract with the Center of Medicare and Medicaid Innovation to improve access to healthcare services and improve patient outcomes through improved care coordination processes. One of the domains our institution has increased efforts to improve is in provider handoffs. Toward that goal, we have worked to disentangle the effects of different factors of provider‐to‐provider communication that may influence readmissions.[26] For example, effective written provider handoffs in the form of accurate and timely discharge summaries was considered a key care coordination component of this program, but there was institutional resistance to endorsing an expectation that discharge summary turnaround should be shortened. To build a case for this concept, we sought to test the hypothesis that, at our hospital, longer time to complete hospital discharge summaries was associated with increased readmission rates. Unique to this analysis is that, in the state of Maryland, there is statewide reporting of readmissions, so we were able to account for intra‐ and interhospital readmissions for an all‐payer population. The authors anticipated that findings from this study would help inform discharge quality‐improvement initiatives and reemphasize the importance of timely discharge documentation across all disciplines as part of quality patient care.
METHODS
Study Population and Setting
We conducted a single‐center, retrospective cohort study of 87,994 consecutive patients discharged from Johns Hopkins Hospital, which is a 1000‐bed, tertiary academic medical center in Baltimore, Maryland between January 1, 2013 and December 31, 2014. One thousand ninety‐three (1.2%) of the records on days to complete the discharge summary were missing and were excluded from the analysis.
Data Source and Covariates
Data were derived from several sources. The Johns Hopkins Hospital data mart financial database, used for mandatory reporting to the State of Maryland, provided the following patient data: age, gender, race/ethnicity, payer (Medicare, Medicaid, and other) as a proxy for socioeconomic status,[27] hospital service prior to discharge (gynecologyobstetrics, medicine, neurosciences, oncology, pediatrics, and surgical sciences), hospital length of stay (LOS) prior to discharge, Agency for Healthcare Research and Quality (AHRQ) Comorbidity Index (which is an update to the original Elixhauser methodology[28]), and all‐payerrefined diagnosis‐related group (APRDRG) and severity of illness (SOI) combinations (a tool to group patients into clinically comparable disease and SOI categories expected to use similar resources and experience similar outcomes). The Health Services Cost Review Commission (HSCRC) in Maryland provided the observed readmission rate in Maryland for each APRDRG‐SOI combination and served as an expected readmission rate. This risk stratification methodology is similar to the approach used in previous studies.[26, 29] Discharge summary turnaround time was obtained from institutional administrative databases used to track compliance with discharge summary completion. Discharge location (home, facility, home with homecare or hospice, or other) was obtained from Curaspan databases (Curaspan Health Group, Inc., Newton, MA).
Primary Outcome: 30‐Day Readmission
The primary outcome was unplanned rehospitalizations to an acute care hospital in Maryland within 30 days of discharge from Johns Hopkins Hospital. This was as defined by the Maryland HSCRC using an algorithm to exclude readmissions that were likely to be scheduled, as defined by the index admission diagnosis and readmission diagnosis; this algorithm is updated based on the CMS all‐cause readmission algorithm.[30, 31]
Primary Exposure: Days to Complete the Discharge Summary
Discharge summary completion time was defined as the date when the discharge attending physician electronically signs the discharge summary. At our institution, an auto‐fax system sends documents (eg, discharge summaries, clinic notes) to linked providers (eg, primary care providers) shortly after midnight from the day the document is signed by an attending physician. During the period of the project, the policy for discharge summaries at the Johns Hopkins Hospital went from requiring them to be completed within 30 days to 14 days, and we were hoping to use our analyses to inform decision makers why this was important. To emphasize the need for timely completion of discharge summaries, we dichotomized the number of days to complete the discharge summary into >3 versus 3 days (20th percentile cutoff) and modeled it as a continuous variable (per 3‐day increase in days to complete the discharge summary).
Statistical Analysis
To evaluate differences in patient characteristics by readmission status, analysis of variance and 2 tests were used for continuous and dichotomous variables, respectively. Logistic regression was used to evaluate the association between days to complete the discharge summary >3 days and readmission status, adjusting for potentially confounding variables. Before inclusion in the logistic regression model, we confirmed a lack of multicollinearity in the multivariable regression model using variance inflation factors. We evaluated residual versus predicted value plots and residual versus fitted value plots with a locally weighted scatterplot smoothing line. In a sensitivity analysis we evaluated the association between readmission status and different cutoffs (>8 days, 50th percentile; and >14 days, 70% percentile). In a separate analysis, we used interaction terms to test whether the association between the association between days to complete the discharge summary >3 days and hospital readmission varied by the covariates in the analysis (age, sex, race, payer, hospital service, discharge location, LOS, APRDRG‐SOI expected readmission rate, and AHRQ Comorbidity Index). We observed a significant interaction between 30‐day readmission and days to complete the discharge summary >3 days by hospital service. Hence, we separately calculated the adjusted mean readmission rates separately for each hospital service using the least squared means method for the multivariable logistic regression analysis and adjusting for the previously mentioned covariates. In a separate analysis, we used linear regression to evaluate the association between LOS and days to complete the discharge summary, adjusting for potentially confounding variables. Statistical significance was defined as a 2‐sided P < 0.05. Data were analyzed with R (version 2.15.0; R Foundation for Statistical Computing, Vienna, Austria;
RESULTS
Readmitted Patients
In the study period, 14,248 out of 87,994 (16.2%) consecutive eligible patients were readmitted to a hospital in Maryland from patients discharged from Johns Hopkins Hospital between January 1, 2013 and December 31, 2014. A total of 11,027 (77.4%) of the readmissions were back to Johns Hopkins Hospital. Table 1 compares characteristics of readmitted versus nonreadmitted patients, with the following variables being significantly different between these patient groups: age, gender, healthcare payer, hospital service, discharge location, length of stay expected readmission rate, AHRQ Comorbidity Index, and days to complete inpatient discharge summary.
Characteristics | All Patients, N = 87,994 | Not Readmitted, N = 73,746 | Readmitted, N = 14,248 | P Value |
---|---|---|---|---|
| ||||
Age, y | 42.1 (25.1) | 41.3 (25.4) | 46.4 (23.1) | <0.001 |
Male | 43,210 (49.1%) | 35,851 (48.6%) | 7,359 (51.6%) | <0.001 |
Race | <0.001 | |||
Caucasian | 45,705 (51.9%) | 3,8661 (52.4%) | 7,044 (49.4%) | |
African American | 32,777 (37.2%) | 2,6841 (36.4%) | 5,936 (41.7%) | |
Other | 9,512 (10.8%) | 8,244 (11.2%) | 1,268 (8.9%) | |
Payer | <0.001 | |||
Medicare | 22,345 (25.4%) | 17,614 (23.9%) | 4,731 (33.2%) | |
Medicaid | 24,080 (27.4%) | 20,100 (27.3%) | 3,980 (27.9%) | |
Other | 41,569 (47.2%) | 36,032 (48.9%) | 5,537 (38.9%) | |
Hospital service | <0.001 | |||
Gynecologyobstetrics | 9,299 (10.6%) | 8,829 (12.0%) | 470 (3.3%) | |
Medicine | 26,036 (29.6%) | 20,069 (27.2%) | 5,967 (41.9%) | |
Neurosciences | 8,269 (9.4%) | 7,331 (9.9%) | 938 (6.6%) | |
Oncology | 5,222 (5.9%) | 3,898 (5.3%) | 1,324 (9.3%) | |
Pediatrics | 17,029 (19.4%) | 14,684 (19.9%) | 2,345 (16.5%) | |
Surgical sciences | 22,139 (25.2%) | 18,935 (25.7%) | 3,204 (22.5%) | |
Discharge location | <0.001 | |||
Home | 65,478 (74.4%) | 56,359 (76.4%) | 9,119 (64.0%) | |
Home with homecare or hospice | 9,524 (10.8%) | 7,440 (10.1%) | 2,084 (14.6%) | |
Facility (SNF, rehabilitation facility) | 5,398 (6.1%) | 4,131 (5.6%) | 1,267 (8.9%) | |
Other | 7,594 (8.6%) | 5,816 (7.9%) | 1,778 (12.5%) | |
Length of stay, d | 5.5 (8.6) | 5.1 (7.8) | 7.5 (11.6) | <0.001 |
APRDRG‐SOI Expected Readmission Rate, % | 14.4 (9.5) | 13.3 (9.2) | 20.1 (9.0) | <0.001 |
AHRQ Comorbidity Index (1 point) | 2.5 (1.4) | 2.4 (1.4) | 3.0 (1.8) | <0.001 |
Discharge summary completed >3 days | 66,242 (75.3%) | 55,329 (75.0%) | 10,913 (76.6%) | <0.001 |
Association Between Days to Complete the Discharge Summary and Readmission
After hospital discharge, median (IQR) number of days to complete discharge summaries was 8 (416) days. After hospital discharge, median (IQR) number of days to complete discharge summaries and the number of days from discharge to readmission was 8 (416) and 11 (519) days, respectively (P < 0.001). Six thousand one hundred one patients (42.8%) were readmitted before their discharge summary was completed. The median (IQR) days to complete discharge summaries by hospital service in order from shortest to longest was: oncology, 6 (212) days; surgical sciences, 6 (312) days; pediatrics, 7 (315) days; gynecologyobstetrics, 8 (415) days; medicine, 9 (420) days; neurosciences, 12 (621) days.
When we divided the number of days to complete the discharge summary into deciles (02, 2.13, 3.14, 4.16, 6.18, 8.210, 10.114, 14.119, 19.130, >30), a longer number of days to complete discharge summaries had higher unadjusted and adjusted readmission rates (Figure 1). In unadjusted analysis, Table 2 shows that older age, male sex, African American race, oncological versus medicine hospital service, discharge location, longer LOS, higher APRDRG‐SOI expected readmission rate, and higher AHRQ Comorbidity Index were associated with readmission. Days to complete the discharge summary >3 days versus 3 days was associated with a higher readmission rate, with an unadjusted odds ratio (OR) and 95% confidence interval (CI) of 1.09 (95% CI: 1.04 to 1.13, P < 0.001).
Characteristic | Bivariable Analysis* | Multivariable Analysis* | ||
---|---|---|---|---|
OR (95% CI) | P Value | OR (95% CI) | P Value | |
| ||||
Age, 10 y | 1.09 (1.08 to 1.09) | <0.001 | 0.97 (0.95 to 0.98) | <0.001 |
Male | 1.13 (1.09 to 1.17) | <0.001 | 1.01 (0.97 to 1.05) | 0.76 |
Race | ||||
Caucasian | Referent | Referent | ||
African American | 1.21 (1.17 to 1.26) | <0.001 | 1.01 (0.96 to 1.05) | 0.74 |
Other | 0.84 (0.79 to 0.90) | <0.001 | 0.92 (0.86 to 0.98) | 0.01 |
Payer | ||||
Medicare | Referent | Referent | ||
Medicaid | 0.74 (0.70 to 0.77) | <0.001 | 1.03 (0.97 to 1.09) | 0.42 |
Other | 0.57 (0.55 to 0.60) | <0.001 | 0.86 (0.82 to 0.91) | <0.001 |
Hospital service | ||||
Medicine | Referent | Referent | ||
Gynecologyobstetrics | 0.18 (0.16 to 0.20) | <0.001 | 0.50 (0.45 to 0.56) | <0.001 |
Neurosciences | 0.43 (0.40 to 0.46) | <0.001 | 0.76 (0.70 to 0.82) | <0.001 |
Oncology | 1.14 (1.07 to 1.22) | <0.001 | 1.18 (1.10 to 1.28) | <0.001 |
Pediatrics | 0.54 (0.51 to 0.57) | <0.001 | 0.77 (0.71 to 0.83) | <0.001 |
Surgical sciences | 0.57 (0.54 to 0.60) | <0.001 | 0.92 (0.87 to 0.97) | 0.002 |
Discharge location | ||||
Home | Referent | |||
Facility (SNF, rehabilitation facility) | 1.90 (1.77 to 2.03) | <0.001 | 1.11 (1.02 to 1.19) | 0.009 |
Home with homecare or hospice | 1.73 (1.64 to 1.83) | <0.001 | 1.26 (1.19 to 1.34) | <0.001 |
Other | 1.89 (1.78 to 2.00) | <0.001 | 1.25 (1.18 to 1.34) | <0.001 |
Length of stay, d | 1.03 (1.02 to 1.03) | <0.001 | 1.00 (1.00 to 1.01) | <0.001 |
APRDRG‐SOI expected readmission rate, % | 1.08 (1.07 to 1.08) | <0.001 | 1.06 (1.06 to 1.06) | <0.001 |
AHRQ Comorbidity Index (1 point) | 1.27 (1.26 to 1.28) | <0.001 | 1.11 (1.09 to 1.12) | <0.001 |
Discharge summary completed >3 days | 1.09 (1.04 to 1.14) | <0.001 | 1.09 (1.05 to 1.14) | <0.001 |
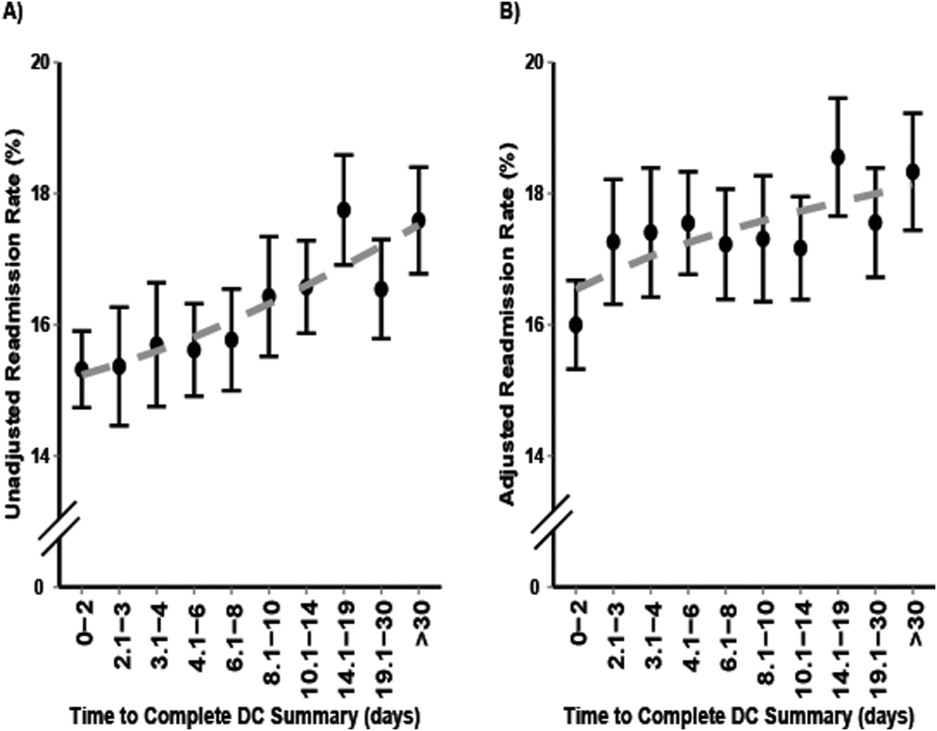
Multivariable and Secondary Analyses
In adjusted analysis (Table 2), patients discharged from an oncologic service relative to a medicine hospital service (OR: 1.19, 95% CI: 1.10 to 1.28, P < 0.001), patients discharged to a facility, home with homecare or hospice, or other location compared to home (facility OR: 1.11, 95% CI: 1.02 to 1.19, P = 0.009; home with homecare or hospice OR: 1.26, 95% CI: 1.19 to 1.34, P < 0.001; other OR: 1.25, 95% CI: 1.18 to 1.34, P < 0.001), patients with longer LOS (OR: 1.11 per day, 95% CI: 1.10 to 1.12, P < 0.001), patients with a higher expected readmission rates (OR: 1.01 per percent, 95% CI: 1.00 to 1.01, P < 0.001), and patients with a higher AHRQ comorbidity index (OR: 1.06 per 1 point, 95% CI: 1.06 to 1.06, P < 0.001) had higher 30‐day readmission rates. Overall, days to complete the discharge summary >3 days versus 3 days was associated with a higher readmission rate (OR: 1.09, 95% CI: 1.05 to 1.14, P < 0.001).
In a sensitivity analysis, discharge summary completion >8 days (median) versus 8 days was associated with higher unadjusted readmission rate (OR: 1.11, 95% CI: 1.07 to 1.15, P < 0.001) and a higher adjusted readmission rate (OR: 1.06, 95% CI: 1.02 to 1.10, P < 0.001). Discharge summary completion >14 days (70th percentile) versus 14 days was also associated with higher unadjusted readmission rate (OR: 1.15, 95% CI: 1.08 to 1.21, P < 0.001) and a higher adjusted readmission rate (OR: 1.09, 95% CI: 1.02 to 1.16, P = 0.008). The association between days to complete the discharge summary >3 days and readmissions was found to vary significantly by hospital service (P = 0.03). For comparing days to complete the discharge summary >3 versus 3 days, Table 3 shows that neurosciences, pediatrics, oncology, and medicine hospital services were associated with significantly increased adjusted mean readmission rates. Additionally, when days to complete the discharge summary was modeled as a continuous variable, we found that for every 3 days the odds of readmission increased by 1% (OR: 1.01, 95% CI: 1.00 to 1.01, P < 0.001).
Days to Complete Discharge Summary by Hospital Service | Adjusted Mean Readmission Rate (95% CI)* | P Value |
---|---|---|
| ||
Gynecologyobstetrics | 0.30 | |
03 days, n = 1,792 | 5.4 (4.1 to 6.7) | |
>3 days, n = 7,507 | 6.0 (4.9 to 7.0) | |
Medicine | 0.04 | |
03 days, n = 6,137 | 21.1 (20.0 to 22.3) | |
>3 days, n = 19,899 | 22.4 (21.6 to 23.2) | |
Neurosciences | 0.02 | |
03 days, n = 1,116 | 10.1 (8.2 to 12.1) | |
>3 days, n = 7,153 | 12.5 (11.6 to 13.5) | |
Oncology | 0.01 | |
03 days, n = 1,885 | 25.0 (22.6 to 27.4) | |
>3 days, n = 3,337 | 28.2 (26.6 to 30.2) | |
Pediatrics | 0.001 | |
03 days, n = 4,561 | 9.5 (6.9 to 12.2) | |
>3 days, n = 12,468 | 11.4 (8.9 to 13.9) | |
Surgical sciences | 0.89 | |
03 days, n = 6,261 | 15.2 (14.2 to 16.1) | |
>3 days, n = 15,878 | 15.1 (14.4 to 15.8) |
In an unadjusted analysis, we found that the relationship between LOS and days to complete the discharge summary was not significant ( coefficient and 95% CI:, 0.01, 0.02 to 0.00, P = 0.20). However, we found a small but significant relationship in our multivariable analysis, such that each hospitalization day was associated with a 0.01 (95% CI: 0.00 to 0.02, P = 0.03) increase in days to complete the discharge summary.
DISCUSSION
In this single‐center retrospective analysis, the number of days to complete the discharge summary was significantly associated with readmissions after hospitalization. This association was independent of age, gender, comorbidity index, payer, discharge location, length of hospital stay, expected readmission rate based on diagnosis and severity of illness, and all hospital services. The odds of readmission for patients with delayed discharge summaries was small but significant. This is important in the current landscape of readmissions, particularly for institutions who are challenged to reduce readmission rates, and a small relative difference in readmissions may be the difference between getting penalized or not. In the context of prior studies, the results highlight the role of timely discharge summary as an under‐recognized metric, which may be a valid litmus test for care coordination. The findings also emphasize the potential of early summaries to expedite communication and to help facilitate quality of patient care. Hence, the study results extend the literature examining the relationship of delay in discharge summary with unfavorable patient outcomes.[15, 32]
In contrast to prior reports with limited focus on same‐hospital readmissions,[18, 33, 34, 35] readmissions beyond 30 days,[12] or focused on a specific patient population,[13, 36] this study evaluates both intra‐ and interhospital 30‐day readmissions in Maryland in an all‐payer, multi‐institution, diverse patient population. Additionally, prior research is conflicting with respect to whether timely discharges summaries are significantly associated with increased hospital readmissions.[12, 13, 14, 15] Although it is not surprising that inadequate care during hospitalization could result in readmissions, the role of discharge summaries remain underappreciated. Having a timely discharge summary may not always prevent readmissions, but our study showed that 43% of readmission occurred before the discharge summary completion. Not having a completed discharge summary at the time of readmission may have been a driver for the positive association between timely completion and 30‐day readmission we observed. This study highlights that delay in the discharge summary could be a marker of poor transitions of care, because suboptimal dissemination of critical information to care providers may result in discontinuity of patient care posthospitalization.
A plausible mechanism of the association between discharge summary delays and readmissions could be the provision of collateral information, which may potentially alter the threshold for readmissions. For example, in the emergency room/emergency department (ER/ED) setting, discharge summaries may help with preventable readmissions. For patients who present repeatedly with the same complaint, timely summaries to ER/ED providers may help reframe the patient complaints, such as patient has concern X, which was previously identified to be related to diagnosis Y. As others have shown, the content of discharge summaries, format, and accessibility (electronic vs paper chart), as well as timely distribution of summaries, are key factors that impact quality outcomes.[2, 12, 15, 37, 38] By detailing prior hospital information (ie, discharge medications, prior presentations, tests completed), summaries could help prevent errors in medication dosing, reduce unnecessary testing, and help facilitate admission triage. Summaries may have information regarding a new diagnosis such as the results of an endoscopic evaluation that revealed the source of occult gastrointestinal bleeding, which could help contextualize a complaint of repeat melena and redirect goals of care. Discussions of goals of care in the discharge summary may guide primary providers in continued care management plans.
Our study findings underscore a positive correlation between late discharge summaries and readmissions. However, the extent that this is a causal relationship is unclear; the association of delay in days to complete the discharge summary with readmission may be an epiphenomenon related to processes related to quality of clinical care. For example, delays in discharge summary completion could be a marker of other system issues, such as a stressed work environment. It is possible that providers who fail to complete timely discharge summaries may also fail to do other important functions related to transitions of care and care coordination. However, even if this is so, timely discharge summaries could become a focal point for discussion for optimization of care transitions. A discharge summary could be delayed because the patient has already been readmitted before the summary was distributed, thus making that original summary less relevant. Delays could also be a reflection of the data complexity for patients with longer hospital stays. This is supported by the small but significant relationship between LOS and days to complete the discharge summary in this study. Lastly, delays in discharge summary completion may also be a proxy of provider communication and can reflect the culture of communication at the institution.
Although unplanned hospital readmission is an important outcome, many readmissions may be related to other factors such as disease progression, rather than late summaries or the lack of postdischarge communication. For instance, prior reports did not find any association between the PCP seeing the discharge summaries or direct communications with the PCP and 30‐day clinical outcomes for readmission and death.[26, 39] However, these studies were limited in their use of self‐reported handoffs, did not measure quality of information transfer, and failed to capture a broader audience beyond the PCP, such as ED physicians or specialists.
Our results suggest that the relationship between days to complete discharge summaries and 30‐day readmissions may vary depending on whether the hospitalization is primarily surgical/procedural versus medical treatment. A recent study found that most readmissions after surgery were associated with new complications related to the procedure and not exacerbation of prior index hospitalization complications.[40] Hence, treatment for common causes of hospital readmissions after surgical or gynecological procedures, such as wound infections, acute anemia, ileus, or dehydration, may not necessarily require a completed discharge summary for appropriate management. However, we caution extending this finding to clinical practice before further studies are conducted on specific procedures and in different clinical settings.
Results from this study also support institutional policies that specify the need for practitioners to complete discharge summaries contemporaneously, such as at the time of discharge or within a couple of days. Unlike other forms of communication that are optional, discharge summaries are required, so we recommend that practitioners be held accountable for short turnaround times. For example, providers could be graded and rated on timely completions of discharge summaries, among other performance variables. Anecdotally at our institutions, we have heard from practitioners that it takes less time to complete them when you do them on the day of discharge, because the hospitalization course is fresher in their mind and they have to wade through less information in the medical record to complete an accurate discharge summary. To this point, a barrier to on‐time completion is that providers may have misconceptions about what is really vital information to convey to the next provider. In agreement with past research and in the era of the electronic medical record system, we recommend that the discharge summary should be a quick synthesis of key findings that incorporates only the important elements, such as why the patient was hospitalized, what were key findings and key responses to therapy, what is pending at the time of discharge, what medications the patient is currently taking, and what are the follow‐up plans, rather than a lengthy expose of all the findings.[13, 36, 41, 42]
Lastly, our study results should be taken in the context of its limitations. As a single‐center study, findings may lack generalizability. In particular, the results may not generalize to hospitals that lack access to statewide reporting. We were also not able to assess readmission for patients who may have been readmitted to a hospital outside of Maryland. Although we adjusted for pertinent variables such as age, gender, healthcare payer, hospital service, comorbidity index, discharge location, LOS, and expected readmission rates, there may be other relevant confounders that we failed to capture or measure optimally. Median days to complete the discharge summary in this study was 8 days, which is longer than practices at other institutions, and may also limit this study's generalizability.[15, 36, 42] However, prior research supports our findings,[15] and a systematic review found that only 29% and 52% of discharge summaries were completed by 2 weeks and 4 weeks, respectively.[9] Finally, as noted above and perhaps most important, it is possible that discharge summary turnaround time does not in itself causally impact readmissions, but rather reflects an underlying commitment of the inpatient team to effectively coordinate care following hospital discharge.
CONCLUSION
In sum, this study delineates an underappreciated but important relationship of timely discharge summary completion and readmission outcomes. The discharge summary may be a relevant metric reflecting quality of patient care. Healthcare providers may begin to target timely discharge summaries as a potential focal point of quality‐improvement projects with the goal to facilitate better patient outcomes.
Disclosures
The authors certify that no party having a direct interest in the results of the research supporting this article has or will confer a benefit on us or on any organization with which we are associated, and, if applicable, the authors certify that all financial and material support for this research (eg, Centers for Medicare and Medicaid Services, National Institutes of Health, or National Health Service grants) and work are clearly identified. This study was supported by funding opportunity, number CMS‐1C1‐12‐0001, from the Centers for Medicare and Medicaid Services and Center for Medicare and Medicaid Innovation. Its contents are solely the responsibility of the authors and do not necessarily represent the official views of the Department of Health and Human Services or any of its agencies.
- Development and sustainability of an inpatient‐to‐outpatient discharge handoff tool: a quality improvement project. Jt Comm J Qual Patient Saf. 2014;40(5):219–227. , , , et al.
- Documentation of mandated discharge summary components in transitions from acute to subacute care. In: Henriksen K, Battles JB, Keyes MA, et al., eds. Advances in Patient Safety: New Directions and Alternative Approaches. Vol. 2. Culture and Redesign. Rockville, MD: Agency for Healthcare Research and Quality; 2008. , , , , , .
- Better transitions: improving comprehension of discharge instructions. Front Health Serv Manage. 2009;25(3):11–32. , , , .
- Structured discharge education improves early outcome in orthopedic patients. Int J Orthop Trauma Nurs. 2010;14(2):66–74. , , , .
- Hospital discharge documentation and risk of rehospitalisation. BMJ Qual Saf. 2011;20(9):773–778. , , , et al.
- The hospital discharge: a review of a high risk care transition with highlights of a reengineered discharge process. J Patient Saf. 2007;3(2):97–106. , , .
- Interventions to reduce 30‐day rehospitalization: a systematic review. Ann Intern Med. 2011;155(8):520–528. , , , , .
- Effect of hospital follow‐up appointment on clinical event outcomes and mortality. Arch Intern Med. 2010;170(11):955–960. , , , , , .
- Deficits in communication and information transfer between hospital‐based and primary care physicians: implications for patient safety and continuity of care. JAMA. 2007;297(8):831–841. , , , , , .
- Provider characteristics, clinical‐work processes and their relationship to discharge summary quality for sub‐acute care patients. J Gen Intern Med. 2012;27(1):78–84. , , , , .
- Contemporary evidence about hospital strategies for reducing 30‐day readmissions: a national study. J Am Coll Cardiol. 2012;60(7):607–614. , , , et al.
- Effect of discharge summary availability during post‐discharge visits on hospital readmission. J Gen Intern Med. 2002;17(3):186–192. , , , .
- Association of discharge summary quality with readmission risk for patients hospitalized with heart failure exacerbation. Circ Cardiovasc Qual Outcomes. 2015;8(1):109–111. , , , et al.
- The independent association of provider and information continuity on outcomes after hospital discharge: implications for hospitalists. J Hosp Med. 2010;5(7):398–405. , , , et al.
- Timeliness in discharge summary dissemination is associated with patients' clinical outcomes. J Eval Clin Pract. 2013;19(1):76–79. , , , , .
- Communication and information deficits in patients discharged to rehabilitation facilities: an evaluation of five acute care hospitals. J Hosp Med. 2009;4(8):E28–E33. , , , et al.
- Preventing readmissions through comprehensive discharge planning. Prof Case Manag. 2013;18(2):56–63; quiz 64–65. , , .
- Effect of a postdischarge virtual ward on readmission or death for high‐risk patients: a randomized clinical trial. JAMA. 2014;312(13):1305–1312.. , , , et al.
- Risk factors for early unplanned hospital readmission in the elderly. J Gen Intern Med. 1991;6(3):223–228. , , .
- Differences between early and late readmissions among patients: a cohort study. Ann Intern Med. 2015;162(11):741–749. , , , , .
- Post‐acute care payment reform demonstration report to congress supplement‐interim report. Centers for Medicare 14(3):1–14; quiz 88–89. , , , et al.
- Comprehensive discharge planning and home follow‐up of hospitalized elders: a randomized clinical trial. JAMA. 1999;281(7):613–620. , , , et al.
- Posthospital care transitions: patterns, complications, and risk identification. Health Serv Res. 2004;39(5):1449–1465. , , , .
- Transitions of care consensus policy statement: American College of Physicians, Society of General Internal Medicine, Society of Hospital Medicine, American Geriatrics Society, American College of Emergency Physicians, and Society for Academic Emergency Medicine. J Hosp Med. 2009;4(6):364–370. , , , et al.
- Association of self‐reported hospital discharge handoffs with 30‐day readmissions. JAMA Intern Med. 2013;173(8):624–629. , , , et al.
- Socioeconomic disparities in health: pathways and policies. Health Aff (Millwood). 2002;21(2):60–76. , .
- Comorbidity measures for use with administrative data. Med Care. 1998;36(1):8–27. , , , .
- Functional status impairment is associated with unplanned readmissions. Arch Phys Med Rehabil. 2013;94(10):1951–1958. , , , , , .
- Centers for Medicare 35(10):1044–1059.
- Understanding and execution of discharge instructions. Am J Med Qual. 2013;28(5):383–391. , , , et al.
- Beyond the hospital gates: elucidating the interactive association of social support, depressive symptoms, and physical function with 30‐day readmissions. Am J Phys Med Rehabil. 2015;94(7):555–567. , , , , .
- Improving the discharge process by embedding a discharge facilitator in a resident team. J Hosp Med. 2011;6(9):494–500. , , , et al.
- Hospital variation in quality of discharge summaries for patients hospitalized with heart failure exacerbation. Circ Cardiovasc Qual Outcomes. 2015;8(1):77–86 , , , et al.
- Addressing the business of discharge: building a case for an electronic discharge summary. J Hosp Med. 2011;6(1):37–42. , , , .
- Joint commission requirements for discharge instructions in patients with heart failure: is understanding important for preventing readmissions? J Card Fail. 2014;20(9):641–649. , , , , .
- Association of communication between hospital‐based physicians and primary care providers with patient outcomes. J Gen Intern Med. 2009;24(3):381–386. , , , et al.
- Underlying reasons associated with hospital readmission following surgery in the united states. JAMA. 2015;313(5):483–495. , , , et al.
- Assessing quality and efficiency of discharge summaries. Am J Med Qual. 2005;20(6):337–343. , , , , .
- Comprehensive quality of discharge summaries at an academic medical center. J Hosp Med. 2013;8(8):436–443. , , , et al.
Across the continuum of care, the discharge summary is a critical tool for communication among care providers.[1] In the United States, the Joint Commission policies mandate that all hospital providers complete a discharge summary for patients with specific components to foster effective communication with future providers.[2] Because outpatient providers and emergency physicians rely on clinical information in the discharge summary to ensure appropriate postdischarge continuity of care, timely documentation is potentially an essential aspect of readmission reduction initiatives.[3, 4, 5] Prior reports indicate that poor discharge documentation of follow‐up plan‐of‐care increases the risk of hospitalization, whereas structured instructions, patient education, and direct communications with primary care physicians (PCPs) reduce repeat hospital visits.[6, 7, 8, 9] However, the current literature is limited in its narrow focus on the contents of discharge summaries, considered only same‐hospital readmissions, or considered readmissions within 3 months of discharge.[10, 11, 12, 13] Moreover, some prior research has suggested no association between discharge summary timeliness with readmission,[12, 13, 14] whereas another study did find a relationship,[15] hence the need to study this further is important. Filling this gap in knowledge could provide an avenue to track and improve quality of patient care, as delays in discharge summaries have been linked with pot‐discharge adverse outcomes and patient safety concerns.[15, 16, 17, 18] Because readmissions often occur soon after discharge, having timely discharge summaries may be particularly important to outcomes.[19, 20]
This research began under the framework of evaluating a bundle of care coordination strategies that were implemented at the Johns Hopkins Health System. These strategies were informed by the early Centers for Medicare and Medicaid Services (CMS) demonstration projects and other best practices that have been documented in the literature to improve utilization and improve communication during transitions of care.[21, 22, 23, 24, 25] Later they were augmented through a contract with the Center of Medicare and Medicaid Innovation to improve access to healthcare services and improve patient outcomes through improved care coordination processes. One of the domains our institution has increased efforts to improve is in provider handoffs. Toward that goal, we have worked to disentangle the effects of different factors of provider‐to‐provider communication that may influence readmissions.[26] For example, effective written provider handoffs in the form of accurate and timely discharge summaries was considered a key care coordination component of this program, but there was institutional resistance to endorsing an expectation that discharge summary turnaround should be shortened. To build a case for this concept, we sought to test the hypothesis that, at our hospital, longer time to complete hospital discharge summaries was associated with increased readmission rates. Unique to this analysis is that, in the state of Maryland, there is statewide reporting of readmissions, so we were able to account for intra‐ and interhospital readmissions for an all‐payer population. The authors anticipated that findings from this study would help inform discharge quality‐improvement initiatives and reemphasize the importance of timely discharge documentation across all disciplines as part of quality patient care.
METHODS
Study Population and Setting
We conducted a single‐center, retrospective cohort study of 87,994 consecutive patients discharged from Johns Hopkins Hospital, which is a 1000‐bed, tertiary academic medical center in Baltimore, Maryland between January 1, 2013 and December 31, 2014. One thousand ninety‐three (1.2%) of the records on days to complete the discharge summary were missing and were excluded from the analysis.
Data Source and Covariates
Data were derived from several sources. The Johns Hopkins Hospital data mart financial database, used for mandatory reporting to the State of Maryland, provided the following patient data: age, gender, race/ethnicity, payer (Medicare, Medicaid, and other) as a proxy for socioeconomic status,[27] hospital service prior to discharge (gynecologyobstetrics, medicine, neurosciences, oncology, pediatrics, and surgical sciences), hospital length of stay (LOS) prior to discharge, Agency for Healthcare Research and Quality (AHRQ) Comorbidity Index (which is an update to the original Elixhauser methodology[28]), and all‐payerrefined diagnosis‐related group (APRDRG) and severity of illness (SOI) combinations (a tool to group patients into clinically comparable disease and SOI categories expected to use similar resources and experience similar outcomes). The Health Services Cost Review Commission (HSCRC) in Maryland provided the observed readmission rate in Maryland for each APRDRG‐SOI combination and served as an expected readmission rate. This risk stratification methodology is similar to the approach used in previous studies.[26, 29] Discharge summary turnaround time was obtained from institutional administrative databases used to track compliance with discharge summary completion. Discharge location (home, facility, home with homecare or hospice, or other) was obtained from Curaspan databases (Curaspan Health Group, Inc., Newton, MA).
Primary Outcome: 30‐Day Readmission
The primary outcome was unplanned rehospitalizations to an acute care hospital in Maryland within 30 days of discharge from Johns Hopkins Hospital. This was as defined by the Maryland HSCRC using an algorithm to exclude readmissions that were likely to be scheduled, as defined by the index admission diagnosis and readmission diagnosis; this algorithm is updated based on the CMS all‐cause readmission algorithm.[30, 31]
Primary Exposure: Days to Complete the Discharge Summary
Discharge summary completion time was defined as the date when the discharge attending physician electronically signs the discharge summary. At our institution, an auto‐fax system sends documents (eg, discharge summaries, clinic notes) to linked providers (eg, primary care providers) shortly after midnight from the day the document is signed by an attending physician. During the period of the project, the policy for discharge summaries at the Johns Hopkins Hospital went from requiring them to be completed within 30 days to 14 days, and we were hoping to use our analyses to inform decision makers why this was important. To emphasize the need for timely completion of discharge summaries, we dichotomized the number of days to complete the discharge summary into >3 versus 3 days (20th percentile cutoff) and modeled it as a continuous variable (per 3‐day increase in days to complete the discharge summary).
Statistical Analysis
To evaluate differences in patient characteristics by readmission status, analysis of variance and 2 tests were used for continuous and dichotomous variables, respectively. Logistic regression was used to evaluate the association between days to complete the discharge summary >3 days and readmission status, adjusting for potentially confounding variables. Before inclusion in the logistic regression model, we confirmed a lack of multicollinearity in the multivariable regression model using variance inflation factors. We evaluated residual versus predicted value plots and residual versus fitted value plots with a locally weighted scatterplot smoothing line. In a sensitivity analysis we evaluated the association between readmission status and different cutoffs (>8 days, 50th percentile; and >14 days, 70% percentile). In a separate analysis, we used interaction terms to test whether the association between the association between days to complete the discharge summary >3 days and hospital readmission varied by the covariates in the analysis (age, sex, race, payer, hospital service, discharge location, LOS, APRDRG‐SOI expected readmission rate, and AHRQ Comorbidity Index). We observed a significant interaction between 30‐day readmission and days to complete the discharge summary >3 days by hospital service. Hence, we separately calculated the adjusted mean readmission rates separately for each hospital service using the least squared means method for the multivariable logistic regression analysis and adjusting for the previously mentioned covariates. In a separate analysis, we used linear regression to evaluate the association between LOS and days to complete the discharge summary, adjusting for potentially confounding variables. Statistical significance was defined as a 2‐sided P < 0.05. Data were analyzed with R (version 2.15.0; R Foundation for Statistical Computing, Vienna, Austria;
RESULTS
Readmitted Patients
In the study period, 14,248 out of 87,994 (16.2%) consecutive eligible patients were readmitted to a hospital in Maryland from patients discharged from Johns Hopkins Hospital between January 1, 2013 and December 31, 2014. A total of 11,027 (77.4%) of the readmissions were back to Johns Hopkins Hospital. Table 1 compares characteristics of readmitted versus nonreadmitted patients, with the following variables being significantly different between these patient groups: age, gender, healthcare payer, hospital service, discharge location, length of stay expected readmission rate, AHRQ Comorbidity Index, and days to complete inpatient discharge summary.
Characteristics | All Patients, N = 87,994 | Not Readmitted, N = 73,746 | Readmitted, N = 14,248 | P Value |
---|---|---|---|---|
| ||||
Age, y | 42.1 (25.1) | 41.3 (25.4) | 46.4 (23.1) | <0.001 |
Male | 43,210 (49.1%) | 35,851 (48.6%) | 7,359 (51.6%) | <0.001 |
Race | <0.001 | |||
Caucasian | 45,705 (51.9%) | 3,8661 (52.4%) | 7,044 (49.4%) | |
African American | 32,777 (37.2%) | 2,6841 (36.4%) | 5,936 (41.7%) | |
Other | 9,512 (10.8%) | 8,244 (11.2%) | 1,268 (8.9%) | |
Payer | <0.001 | |||
Medicare | 22,345 (25.4%) | 17,614 (23.9%) | 4,731 (33.2%) | |
Medicaid | 24,080 (27.4%) | 20,100 (27.3%) | 3,980 (27.9%) | |
Other | 41,569 (47.2%) | 36,032 (48.9%) | 5,537 (38.9%) | |
Hospital service | <0.001 | |||
Gynecologyobstetrics | 9,299 (10.6%) | 8,829 (12.0%) | 470 (3.3%) | |
Medicine | 26,036 (29.6%) | 20,069 (27.2%) | 5,967 (41.9%) | |
Neurosciences | 8,269 (9.4%) | 7,331 (9.9%) | 938 (6.6%) | |
Oncology | 5,222 (5.9%) | 3,898 (5.3%) | 1,324 (9.3%) | |
Pediatrics | 17,029 (19.4%) | 14,684 (19.9%) | 2,345 (16.5%) | |
Surgical sciences | 22,139 (25.2%) | 18,935 (25.7%) | 3,204 (22.5%) | |
Discharge location | <0.001 | |||
Home | 65,478 (74.4%) | 56,359 (76.4%) | 9,119 (64.0%) | |
Home with homecare or hospice | 9,524 (10.8%) | 7,440 (10.1%) | 2,084 (14.6%) | |
Facility (SNF, rehabilitation facility) | 5,398 (6.1%) | 4,131 (5.6%) | 1,267 (8.9%) | |
Other | 7,594 (8.6%) | 5,816 (7.9%) | 1,778 (12.5%) | |
Length of stay, d | 5.5 (8.6) | 5.1 (7.8) | 7.5 (11.6) | <0.001 |
APRDRG‐SOI Expected Readmission Rate, % | 14.4 (9.5) | 13.3 (9.2) | 20.1 (9.0) | <0.001 |
AHRQ Comorbidity Index (1 point) | 2.5 (1.4) | 2.4 (1.4) | 3.0 (1.8) | <0.001 |
Discharge summary completed >3 days | 66,242 (75.3%) | 55,329 (75.0%) | 10,913 (76.6%) | <0.001 |
Association Between Days to Complete the Discharge Summary and Readmission
After hospital discharge, median (IQR) number of days to complete discharge summaries was 8 (416) days. After hospital discharge, median (IQR) number of days to complete discharge summaries and the number of days from discharge to readmission was 8 (416) and 11 (519) days, respectively (P < 0.001). Six thousand one hundred one patients (42.8%) were readmitted before their discharge summary was completed. The median (IQR) days to complete discharge summaries by hospital service in order from shortest to longest was: oncology, 6 (212) days; surgical sciences, 6 (312) days; pediatrics, 7 (315) days; gynecologyobstetrics, 8 (415) days; medicine, 9 (420) days; neurosciences, 12 (621) days.
When we divided the number of days to complete the discharge summary into deciles (02, 2.13, 3.14, 4.16, 6.18, 8.210, 10.114, 14.119, 19.130, >30), a longer number of days to complete discharge summaries had higher unadjusted and adjusted readmission rates (Figure 1). In unadjusted analysis, Table 2 shows that older age, male sex, African American race, oncological versus medicine hospital service, discharge location, longer LOS, higher APRDRG‐SOI expected readmission rate, and higher AHRQ Comorbidity Index were associated with readmission. Days to complete the discharge summary >3 days versus 3 days was associated with a higher readmission rate, with an unadjusted odds ratio (OR) and 95% confidence interval (CI) of 1.09 (95% CI: 1.04 to 1.13, P < 0.001).
Characteristic | Bivariable Analysis* | Multivariable Analysis* | ||
---|---|---|---|---|
OR (95% CI) | P Value | OR (95% CI) | P Value | |
| ||||
Age, 10 y | 1.09 (1.08 to 1.09) | <0.001 | 0.97 (0.95 to 0.98) | <0.001 |
Male | 1.13 (1.09 to 1.17) | <0.001 | 1.01 (0.97 to 1.05) | 0.76 |
Race | ||||
Caucasian | Referent | Referent | ||
African American | 1.21 (1.17 to 1.26) | <0.001 | 1.01 (0.96 to 1.05) | 0.74 |
Other | 0.84 (0.79 to 0.90) | <0.001 | 0.92 (0.86 to 0.98) | 0.01 |
Payer | ||||
Medicare | Referent | Referent | ||
Medicaid | 0.74 (0.70 to 0.77) | <0.001 | 1.03 (0.97 to 1.09) | 0.42 |
Other | 0.57 (0.55 to 0.60) | <0.001 | 0.86 (0.82 to 0.91) | <0.001 |
Hospital service | ||||
Medicine | Referent | Referent | ||
Gynecologyobstetrics | 0.18 (0.16 to 0.20) | <0.001 | 0.50 (0.45 to 0.56) | <0.001 |
Neurosciences | 0.43 (0.40 to 0.46) | <0.001 | 0.76 (0.70 to 0.82) | <0.001 |
Oncology | 1.14 (1.07 to 1.22) | <0.001 | 1.18 (1.10 to 1.28) | <0.001 |
Pediatrics | 0.54 (0.51 to 0.57) | <0.001 | 0.77 (0.71 to 0.83) | <0.001 |
Surgical sciences | 0.57 (0.54 to 0.60) | <0.001 | 0.92 (0.87 to 0.97) | 0.002 |
Discharge location | ||||
Home | Referent | |||
Facility (SNF, rehabilitation facility) | 1.90 (1.77 to 2.03) | <0.001 | 1.11 (1.02 to 1.19) | 0.009 |
Home with homecare or hospice | 1.73 (1.64 to 1.83) | <0.001 | 1.26 (1.19 to 1.34) | <0.001 |
Other | 1.89 (1.78 to 2.00) | <0.001 | 1.25 (1.18 to 1.34) | <0.001 |
Length of stay, d | 1.03 (1.02 to 1.03) | <0.001 | 1.00 (1.00 to 1.01) | <0.001 |
APRDRG‐SOI expected readmission rate, % | 1.08 (1.07 to 1.08) | <0.001 | 1.06 (1.06 to 1.06) | <0.001 |
AHRQ Comorbidity Index (1 point) | 1.27 (1.26 to 1.28) | <0.001 | 1.11 (1.09 to 1.12) | <0.001 |
Discharge summary completed >3 days | 1.09 (1.04 to 1.14) | <0.001 | 1.09 (1.05 to 1.14) | <0.001 |
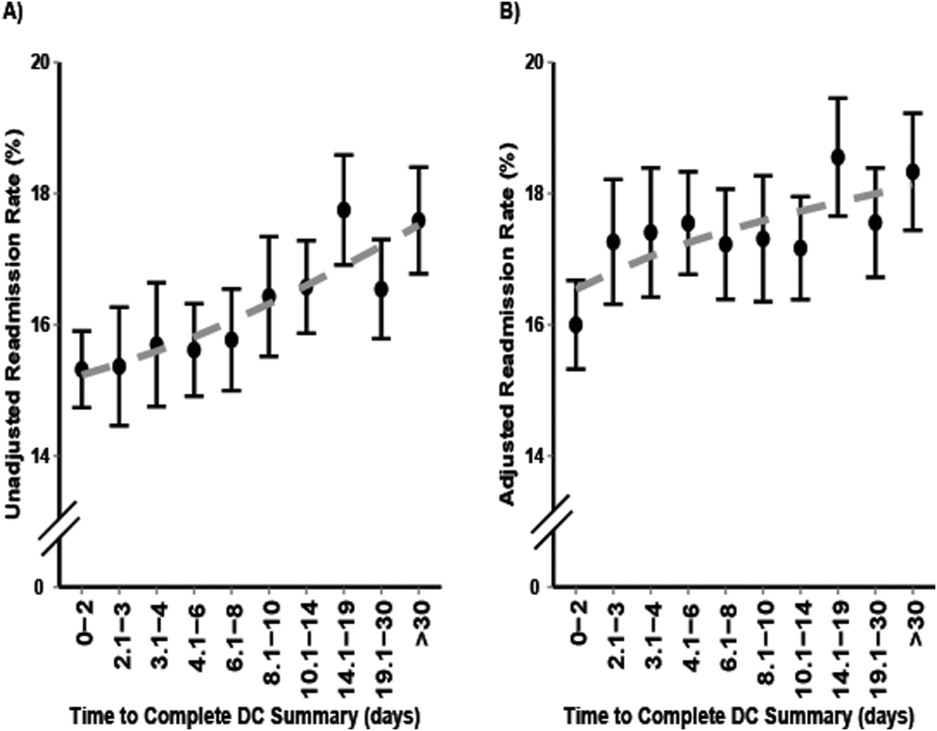
Multivariable and Secondary Analyses
In adjusted analysis (Table 2), patients discharged from an oncologic service relative to a medicine hospital service (OR: 1.19, 95% CI: 1.10 to 1.28, P < 0.001), patients discharged to a facility, home with homecare or hospice, or other location compared to home (facility OR: 1.11, 95% CI: 1.02 to 1.19, P = 0.009; home with homecare or hospice OR: 1.26, 95% CI: 1.19 to 1.34, P < 0.001; other OR: 1.25, 95% CI: 1.18 to 1.34, P < 0.001), patients with longer LOS (OR: 1.11 per day, 95% CI: 1.10 to 1.12, P < 0.001), patients with a higher expected readmission rates (OR: 1.01 per percent, 95% CI: 1.00 to 1.01, P < 0.001), and patients with a higher AHRQ comorbidity index (OR: 1.06 per 1 point, 95% CI: 1.06 to 1.06, P < 0.001) had higher 30‐day readmission rates. Overall, days to complete the discharge summary >3 days versus 3 days was associated with a higher readmission rate (OR: 1.09, 95% CI: 1.05 to 1.14, P < 0.001).
In a sensitivity analysis, discharge summary completion >8 days (median) versus 8 days was associated with higher unadjusted readmission rate (OR: 1.11, 95% CI: 1.07 to 1.15, P < 0.001) and a higher adjusted readmission rate (OR: 1.06, 95% CI: 1.02 to 1.10, P < 0.001). Discharge summary completion >14 days (70th percentile) versus 14 days was also associated with higher unadjusted readmission rate (OR: 1.15, 95% CI: 1.08 to 1.21, P < 0.001) and a higher adjusted readmission rate (OR: 1.09, 95% CI: 1.02 to 1.16, P = 0.008). The association between days to complete the discharge summary >3 days and readmissions was found to vary significantly by hospital service (P = 0.03). For comparing days to complete the discharge summary >3 versus 3 days, Table 3 shows that neurosciences, pediatrics, oncology, and medicine hospital services were associated with significantly increased adjusted mean readmission rates. Additionally, when days to complete the discharge summary was modeled as a continuous variable, we found that for every 3 days the odds of readmission increased by 1% (OR: 1.01, 95% CI: 1.00 to 1.01, P < 0.001).
Days to Complete Discharge Summary by Hospital Service | Adjusted Mean Readmission Rate (95% CI)* | P Value |
---|---|---|
| ||
Gynecologyobstetrics | 0.30 | |
03 days, n = 1,792 | 5.4 (4.1 to 6.7) | |
>3 days, n = 7,507 | 6.0 (4.9 to 7.0) | |
Medicine | 0.04 | |
03 days, n = 6,137 | 21.1 (20.0 to 22.3) | |
>3 days, n = 19,899 | 22.4 (21.6 to 23.2) | |
Neurosciences | 0.02 | |
03 days, n = 1,116 | 10.1 (8.2 to 12.1) | |
>3 days, n = 7,153 | 12.5 (11.6 to 13.5) | |
Oncology | 0.01 | |
03 days, n = 1,885 | 25.0 (22.6 to 27.4) | |
>3 days, n = 3,337 | 28.2 (26.6 to 30.2) | |
Pediatrics | 0.001 | |
03 days, n = 4,561 | 9.5 (6.9 to 12.2) | |
>3 days, n = 12,468 | 11.4 (8.9 to 13.9) | |
Surgical sciences | 0.89 | |
03 days, n = 6,261 | 15.2 (14.2 to 16.1) | |
>3 days, n = 15,878 | 15.1 (14.4 to 15.8) |
In an unadjusted analysis, we found that the relationship between LOS and days to complete the discharge summary was not significant ( coefficient and 95% CI:, 0.01, 0.02 to 0.00, P = 0.20). However, we found a small but significant relationship in our multivariable analysis, such that each hospitalization day was associated with a 0.01 (95% CI: 0.00 to 0.02, P = 0.03) increase in days to complete the discharge summary.
DISCUSSION
In this single‐center retrospective analysis, the number of days to complete the discharge summary was significantly associated with readmissions after hospitalization. This association was independent of age, gender, comorbidity index, payer, discharge location, length of hospital stay, expected readmission rate based on diagnosis and severity of illness, and all hospital services. The odds of readmission for patients with delayed discharge summaries was small but significant. This is important in the current landscape of readmissions, particularly for institutions who are challenged to reduce readmission rates, and a small relative difference in readmissions may be the difference between getting penalized or not. In the context of prior studies, the results highlight the role of timely discharge summary as an under‐recognized metric, which may be a valid litmus test for care coordination. The findings also emphasize the potential of early summaries to expedite communication and to help facilitate quality of patient care. Hence, the study results extend the literature examining the relationship of delay in discharge summary with unfavorable patient outcomes.[15, 32]
In contrast to prior reports with limited focus on same‐hospital readmissions,[18, 33, 34, 35] readmissions beyond 30 days,[12] or focused on a specific patient population,[13, 36] this study evaluates both intra‐ and interhospital 30‐day readmissions in Maryland in an all‐payer, multi‐institution, diverse patient population. Additionally, prior research is conflicting with respect to whether timely discharges summaries are significantly associated with increased hospital readmissions.[12, 13, 14, 15] Although it is not surprising that inadequate care during hospitalization could result in readmissions, the role of discharge summaries remain underappreciated. Having a timely discharge summary may not always prevent readmissions, but our study showed that 43% of readmission occurred before the discharge summary completion. Not having a completed discharge summary at the time of readmission may have been a driver for the positive association between timely completion and 30‐day readmission we observed. This study highlights that delay in the discharge summary could be a marker of poor transitions of care, because suboptimal dissemination of critical information to care providers may result in discontinuity of patient care posthospitalization.
A plausible mechanism of the association between discharge summary delays and readmissions could be the provision of collateral information, which may potentially alter the threshold for readmissions. For example, in the emergency room/emergency department (ER/ED) setting, discharge summaries may help with preventable readmissions. For patients who present repeatedly with the same complaint, timely summaries to ER/ED providers may help reframe the patient complaints, such as patient has concern X, which was previously identified to be related to diagnosis Y. As others have shown, the content of discharge summaries, format, and accessibility (electronic vs paper chart), as well as timely distribution of summaries, are key factors that impact quality outcomes.[2, 12, 15, 37, 38] By detailing prior hospital information (ie, discharge medications, prior presentations, tests completed), summaries could help prevent errors in medication dosing, reduce unnecessary testing, and help facilitate admission triage. Summaries may have information regarding a new diagnosis such as the results of an endoscopic evaluation that revealed the source of occult gastrointestinal bleeding, which could help contextualize a complaint of repeat melena and redirect goals of care. Discussions of goals of care in the discharge summary may guide primary providers in continued care management plans.
Our study findings underscore a positive correlation between late discharge summaries and readmissions. However, the extent that this is a causal relationship is unclear; the association of delay in days to complete the discharge summary with readmission may be an epiphenomenon related to processes related to quality of clinical care. For example, delays in discharge summary completion could be a marker of other system issues, such as a stressed work environment. It is possible that providers who fail to complete timely discharge summaries may also fail to do other important functions related to transitions of care and care coordination. However, even if this is so, timely discharge summaries could become a focal point for discussion for optimization of care transitions. A discharge summary could be delayed because the patient has already been readmitted before the summary was distributed, thus making that original summary less relevant. Delays could also be a reflection of the data complexity for patients with longer hospital stays. This is supported by the small but significant relationship between LOS and days to complete the discharge summary in this study. Lastly, delays in discharge summary completion may also be a proxy of provider communication and can reflect the culture of communication at the institution.
Although unplanned hospital readmission is an important outcome, many readmissions may be related to other factors such as disease progression, rather than late summaries or the lack of postdischarge communication. For instance, prior reports did not find any association between the PCP seeing the discharge summaries or direct communications with the PCP and 30‐day clinical outcomes for readmission and death.[26, 39] However, these studies were limited in their use of self‐reported handoffs, did not measure quality of information transfer, and failed to capture a broader audience beyond the PCP, such as ED physicians or specialists.
Our results suggest that the relationship between days to complete discharge summaries and 30‐day readmissions may vary depending on whether the hospitalization is primarily surgical/procedural versus medical treatment. A recent study found that most readmissions after surgery were associated with new complications related to the procedure and not exacerbation of prior index hospitalization complications.[40] Hence, treatment for common causes of hospital readmissions after surgical or gynecological procedures, such as wound infections, acute anemia, ileus, or dehydration, may not necessarily require a completed discharge summary for appropriate management. However, we caution extending this finding to clinical practice before further studies are conducted on specific procedures and in different clinical settings.
Results from this study also support institutional policies that specify the need for practitioners to complete discharge summaries contemporaneously, such as at the time of discharge or within a couple of days. Unlike other forms of communication that are optional, discharge summaries are required, so we recommend that practitioners be held accountable for short turnaround times. For example, providers could be graded and rated on timely completions of discharge summaries, among other performance variables. Anecdotally at our institutions, we have heard from practitioners that it takes less time to complete them when you do them on the day of discharge, because the hospitalization course is fresher in their mind and they have to wade through less information in the medical record to complete an accurate discharge summary. To this point, a barrier to on‐time completion is that providers may have misconceptions about what is really vital information to convey to the next provider. In agreement with past research and in the era of the electronic medical record system, we recommend that the discharge summary should be a quick synthesis of key findings that incorporates only the important elements, such as why the patient was hospitalized, what were key findings and key responses to therapy, what is pending at the time of discharge, what medications the patient is currently taking, and what are the follow‐up plans, rather than a lengthy expose of all the findings.[13, 36, 41, 42]
Lastly, our study results should be taken in the context of its limitations. As a single‐center study, findings may lack generalizability. In particular, the results may not generalize to hospitals that lack access to statewide reporting. We were also not able to assess readmission for patients who may have been readmitted to a hospital outside of Maryland. Although we adjusted for pertinent variables such as age, gender, healthcare payer, hospital service, comorbidity index, discharge location, LOS, and expected readmission rates, there may be other relevant confounders that we failed to capture or measure optimally. Median days to complete the discharge summary in this study was 8 days, which is longer than practices at other institutions, and may also limit this study's generalizability.[15, 36, 42] However, prior research supports our findings,[15] and a systematic review found that only 29% and 52% of discharge summaries were completed by 2 weeks and 4 weeks, respectively.[9] Finally, as noted above and perhaps most important, it is possible that discharge summary turnaround time does not in itself causally impact readmissions, but rather reflects an underlying commitment of the inpatient team to effectively coordinate care following hospital discharge.
CONCLUSION
In sum, this study delineates an underappreciated but important relationship of timely discharge summary completion and readmission outcomes. The discharge summary may be a relevant metric reflecting quality of patient care. Healthcare providers may begin to target timely discharge summaries as a potential focal point of quality‐improvement projects with the goal to facilitate better patient outcomes.
Disclosures
The authors certify that no party having a direct interest in the results of the research supporting this article has or will confer a benefit on us or on any organization with which we are associated, and, if applicable, the authors certify that all financial and material support for this research (eg, Centers for Medicare and Medicaid Services, National Institutes of Health, or National Health Service grants) and work are clearly identified. This study was supported by funding opportunity, number CMS‐1C1‐12‐0001, from the Centers for Medicare and Medicaid Services and Center for Medicare and Medicaid Innovation. Its contents are solely the responsibility of the authors and do not necessarily represent the official views of the Department of Health and Human Services or any of its agencies.
Across the continuum of care, the discharge summary is a critical tool for communication among care providers.[1] In the United States, the Joint Commission policies mandate that all hospital providers complete a discharge summary for patients with specific components to foster effective communication with future providers.[2] Because outpatient providers and emergency physicians rely on clinical information in the discharge summary to ensure appropriate postdischarge continuity of care, timely documentation is potentially an essential aspect of readmission reduction initiatives.[3, 4, 5] Prior reports indicate that poor discharge documentation of follow‐up plan‐of‐care increases the risk of hospitalization, whereas structured instructions, patient education, and direct communications with primary care physicians (PCPs) reduce repeat hospital visits.[6, 7, 8, 9] However, the current literature is limited in its narrow focus on the contents of discharge summaries, considered only same‐hospital readmissions, or considered readmissions within 3 months of discharge.[10, 11, 12, 13] Moreover, some prior research has suggested no association between discharge summary timeliness with readmission,[12, 13, 14] whereas another study did find a relationship,[15] hence the need to study this further is important. Filling this gap in knowledge could provide an avenue to track and improve quality of patient care, as delays in discharge summaries have been linked with pot‐discharge adverse outcomes and patient safety concerns.[15, 16, 17, 18] Because readmissions often occur soon after discharge, having timely discharge summaries may be particularly important to outcomes.[19, 20]
This research began under the framework of evaluating a bundle of care coordination strategies that were implemented at the Johns Hopkins Health System. These strategies were informed by the early Centers for Medicare and Medicaid Services (CMS) demonstration projects and other best practices that have been documented in the literature to improve utilization and improve communication during transitions of care.[21, 22, 23, 24, 25] Later they were augmented through a contract with the Center of Medicare and Medicaid Innovation to improve access to healthcare services and improve patient outcomes through improved care coordination processes. One of the domains our institution has increased efforts to improve is in provider handoffs. Toward that goal, we have worked to disentangle the effects of different factors of provider‐to‐provider communication that may influence readmissions.[26] For example, effective written provider handoffs in the form of accurate and timely discharge summaries was considered a key care coordination component of this program, but there was institutional resistance to endorsing an expectation that discharge summary turnaround should be shortened. To build a case for this concept, we sought to test the hypothesis that, at our hospital, longer time to complete hospital discharge summaries was associated with increased readmission rates. Unique to this analysis is that, in the state of Maryland, there is statewide reporting of readmissions, so we were able to account for intra‐ and interhospital readmissions for an all‐payer population. The authors anticipated that findings from this study would help inform discharge quality‐improvement initiatives and reemphasize the importance of timely discharge documentation across all disciplines as part of quality patient care.
METHODS
Study Population and Setting
We conducted a single‐center, retrospective cohort study of 87,994 consecutive patients discharged from Johns Hopkins Hospital, which is a 1000‐bed, tertiary academic medical center in Baltimore, Maryland between January 1, 2013 and December 31, 2014. One thousand ninety‐three (1.2%) of the records on days to complete the discharge summary were missing and were excluded from the analysis.
Data Source and Covariates
Data were derived from several sources. The Johns Hopkins Hospital data mart financial database, used for mandatory reporting to the State of Maryland, provided the following patient data: age, gender, race/ethnicity, payer (Medicare, Medicaid, and other) as a proxy for socioeconomic status,[27] hospital service prior to discharge (gynecologyobstetrics, medicine, neurosciences, oncology, pediatrics, and surgical sciences), hospital length of stay (LOS) prior to discharge, Agency for Healthcare Research and Quality (AHRQ) Comorbidity Index (which is an update to the original Elixhauser methodology[28]), and all‐payerrefined diagnosis‐related group (APRDRG) and severity of illness (SOI) combinations (a tool to group patients into clinically comparable disease and SOI categories expected to use similar resources and experience similar outcomes). The Health Services Cost Review Commission (HSCRC) in Maryland provided the observed readmission rate in Maryland for each APRDRG‐SOI combination and served as an expected readmission rate. This risk stratification methodology is similar to the approach used in previous studies.[26, 29] Discharge summary turnaround time was obtained from institutional administrative databases used to track compliance with discharge summary completion. Discharge location (home, facility, home with homecare or hospice, or other) was obtained from Curaspan databases (Curaspan Health Group, Inc., Newton, MA).
Primary Outcome: 30‐Day Readmission
The primary outcome was unplanned rehospitalizations to an acute care hospital in Maryland within 30 days of discharge from Johns Hopkins Hospital. This was as defined by the Maryland HSCRC using an algorithm to exclude readmissions that were likely to be scheduled, as defined by the index admission diagnosis and readmission diagnosis; this algorithm is updated based on the CMS all‐cause readmission algorithm.[30, 31]
Primary Exposure: Days to Complete the Discharge Summary
Discharge summary completion time was defined as the date when the discharge attending physician electronically signs the discharge summary. At our institution, an auto‐fax system sends documents (eg, discharge summaries, clinic notes) to linked providers (eg, primary care providers) shortly after midnight from the day the document is signed by an attending physician. During the period of the project, the policy for discharge summaries at the Johns Hopkins Hospital went from requiring them to be completed within 30 days to 14 days, and we were hoping to use our analyses to inform decision makers why this was important. To emphasize the need for timely completion of discharge summaries, we dichotomized the number of days to complete the discharge summary into >3 versus 3 days (20th percentile cutoff) and modeled it as a continuous variable (per 3‐day increase in days to complete the discharge summary).
Statistical Analysis
To evaluate differences in patient characteristics by readmission status, analysis of variance and 2 tests were used for continuous and dichotomous variables, respectively. Logistic regression was used to evaluate the association between days to complete the discharge summary >3 days and readmission status, adjusting for potentially confounding variables. Before inclusion in the logistic regression model, we confirmed a lack of multicollinearity in the multivariable regression model using variance inflation factors. We evaluated residual versus predicted value plots and residual versus fitted value plots with a locally weighted scatterplot smoothing line. In a sensitivity analysis we evaluated the association between readmission status and different cutoffs (>8 days, 50th percentile; and >14 days, 70% percentile). In a separate analysis, we used interaction terms to test whether the association between the association between days to complete the discharge summary >3 days and hospital readmission varied by the covariates in the analysis (age, sex, race, payer, hospital service, discharge location, LOS, APRDRG‐SOI expected readmission rate, and AHRQ Comorbidity Index). We observed a significant interaction between 30‐day readmission and days to complete the discharge summary >3 days by hospital service. Hence, we separately calculated the adjusted mean readmission rates separately for each hospital service using the least squared means method for the multivariable logistic regression analysis and adjusting for the previously mentioned covariates. In a separate analysis, we used linear regression to evaluate the association between LOS and days to complete the discharge summary, adjusting for potentially confounding variables. Statistical significance was defined as a 2‐sided P < 0.05. Data were analyzed with R (version 2.15.0; R Foundation for Statistical Computing, Vienna, Austria;
RESULTS
Readmitted Patients
In the study period, 14,248 out of 87,994 (16.2%) consecutive eligible patients were readmitted to a hospital in Maryland from patients discharged from Johns Hopkins Hospital between January 1, 2013 and December 31, 2014. A total of 11,027 (77.4%) of the readmissions were back to Johns Hopkins Hospital. Table 1 compares characteristics of readmitted versus nonreadmitted patients, with the following variables being significantly different between these patient groups: age, gender, healthcare payer, hospital service, discharge location, length of stay expected readmission rate, AHRQ Comorbidity Index, and days to complete inpatient discharge summary.
Characteristics | All Patients, N = 87,994 | Not Readmitted, N = 73,746 | Readmitted, N = 14,248 | P Value |
---|---|---|---|---|
| ||||
Age, y | 42.1 (25.1) | 41.3 (25.4) | 46.4 (23.1) | <0.001 |
Male | 43,210 (49.1%) | 35,851 (48.6%) | 7,359 (51.6%) | <0.001 |
Race | <0.001 | |||
Caucasian | 45,705 (51.9%) | 3,8661 (52.4%) | 7,044 (49.4%) | |
African American | 32,777 (37.2%) | 2,6841 (36.4%) | 5,936 (41.7%) | |
Other | 9,512 (10.8%) | 8,244 (11.2%) | 1,268 (8.9%) | |
Payer | <0.001 | |||
Medicare | 22,345 (25.4%) | 17,614 (23.9%) | 4,731 (33.2%) | |
Medicaid | 24,080 (27.4%) | 20,100 (27.3%) | 3,980 (27.9%) | |
Other | 41,569 (47.2%) | 36,032 (48.9%) | 5,537 (38.9%) | |
Hospital service | <0.001 | |||
Gynecologyobstetrics | 9,299 (10.6%) | 8,829 (12.0%) | 470 (3.3%) | |
Medicine | 26,036 (29.6%) | 20,069 (27.2%) | 5,967 (41.9%) | |
Neurosciences | 8,269 (9.4%) | 7,331 (9.9%) | 938 (6.6%) | |
Oncology | 5,222 (5.9%) | 3,898 (5.3%) | 1,324 (9.3%) | |
Pediatrics | 17,029 (19.4%) | 14,684 (19.9%) | 2,345 (16.5%) | |
Surgical sciences | 22,139 (25.2%) | 18,935 (25.7%) | 3,204 (22.5%) | |
Discharge location | <0.001 | |||
Home | 65,478 (74.4%) | 56,359 (76.4%) | 9,119 (64.0%) | |
Home with homecare or hospice | 9,524 (10.8%) | 7,440 (10.1%) | 2,084 (14.6%) | |
Facility (SNF, rehabilitation facility) | 5,398 (6.1%) | 4,131 (5.6%) | 1,267 (8.9%) | |
Other | 7,594 (8.6%) | 5,816 (7.9%) | 1,778 (12.5%) | |
Length of stay, d | 5.5 (8.6) | 5.1 (7.8) | 7.5 (11.6) | <0.001 |
APRDRG‐SOI Expected Readmission Rate, % | 14.4 (9.5) | 13.3 (9.2) | 20.1 (9.0) | <0.001 |
AHRQ Comorbidity Index (1 point) | 2.5 (1.4) | 2.4 (1.4) | 3.0 (1.8) | <0.001 |
Discharge summary completed >3 days | 66,242 (75.3%) | 55,329 (75.0%) | 10,913 (76.6%) | <0.001 |
Association Between Days to Complete the Discharge Summary and Readmission
After hospital discharge, median (IQR) number of days to complete discharge summaries was 8 (416) days. After hospital discharge, median (IQR) number of days to complete discharge summaries and the number of days from discharge to readmission was 8 (416) and 11 (519) days, respectively (P < 0.001). Six thousand one hundred one patients (42.8%) were readmitted before their discharge summary was completed. The median (IQR) days to complete discharge summaries by hospital service in order from shortest to longest was: oncology, 6 (212) days; surgical sciences, 6 (312) days; pediatrics, 7 (315) days; gynecologyobstetrics, 8 (415) days; medicine, 9 (420) days; neurosciences, 12 (621) days.
When we divided the number of days to complete the discharge summary into deciles (02, 2.13, 3.14, 4.16, 6.18, 8.210, 10.114, 14.119, 19.130, >30), a longer number of days to complete discharge summaries had higher unadjusted and adjusted readmission rates (Figure 1). In unadjusted analysis, Table 2 shows that older age, male sex, African American race, oncological versus medicine hospital service, discharge location, longer LOS, higher APRDRG‐SOI expected readmission rate, and higher AHRQ Comorbidity Index were associated with readmission. Days to complete the discharge summary >3 days versus 3 days was associated with a higher readmission rate, with an unadjusted odds ratio (OR) and 95% confidence interval (CI) of 1.09 (95% CI: 1.04 to 1.13, P < 0.001).
Characteristic | Bivariable Analysis* | Multivariable Analysis* | ||
---|---|---|---|---|
OR (95% CI) | P Value | OR (95% CI) | P Value | |
| ||||
Age, 10 y | 1.09 (1.08 to 1.09) | <0.001 | 0.97 (0.95 to 0.98) | <0.001 |
Male | 1.13 (1.09 to 1.17) | <0.001 | 1.01 (0.97 to 1.05) | 0.76 |
Race | ||||
Caucasian | Referent | Referent | ||
African American | 1.21 (1.17 to 1.26) | <0.001 | 1.01 (0.96 to 1.05) | 0.74 |
Other | 0.84 (0.79 to 0.90) | <0.001 | 0.92 (0.86 to 0.98) | 0.01 |
Payer | ||||
Medicare | Referent | Referent | ||
Medicaid | 0.74 (0.70 to 0.77) | <0.001 | 1.03 (0.97 to 1.09) | 0.42 |
Other | 0.57 (0.55 to 0.60) | <0.001 | 0.86 (0.82 to 0.91) | <0.001 |
Hospital service | ||||
Medicine | Referent | Referent | ||
Gynecologyobstetrics | 0.18 (0.16 to 0.20) | <0.001 | 0.50 (0.45 to 0.56) | <0.001 |
Neurosciences | 0.43 (0.40 to 0.46) | <0.001 | 0.76 (0.70 to 0.82) | <0.001 |
Oncology | 1.14 (1.07 to 1.22) | <0.001 | 1.18 (1.10 to 1.28) | <0.001 |
Pediatrics | 0.54 (0.51 to 0.57) | <0.001 | 0.77 (0.71 to 0.83) | <0.001 |
Surgical sciences | 0.57 (0.54 to 0.60) | <0.001 | 0.92 (0.87 to 0.97) | 0.002 |
Discharge location | ||||
Home | Referent | |||
Facility (SNF, rehabilitation facility) | 1.90 (1.77 to 2.03) | <0.001 | 1.11 (1.02 to 1.19) | 0.009 |
Home with homecare or hospice | 1.73 (1.64 to 1.83) | <0.001 | 1.26 (1.19 to 1.34) | <0.001 |
Other | 1.89 (1.78 to 2.00) | <0.001 | 1.25 (1.18 to 1.34) | <0.001 |
Length of stay, d | 1.03 (1.02 to 1.03) | <0.001 | 1.00 (1.00 to 1.01) | <0.001 |
APRDRG‐SOI expected readmission rate, % | 1.08 (1.07 to 1.08) | <0.001 | 1.06 (1.06 to 1.06) | <0.001 |
AHRQ Comorbidity Index (1 point) | 1.27 (1.26 to 1.28) | <0.001 | 1.11 (1.09 to 1.12) | <0.001 |
Discharge summary completed >3 days | 1.09 (1.04 to 1.14) | <0.001 | 1.09 (1.05 to 1.14) | <0.001 |
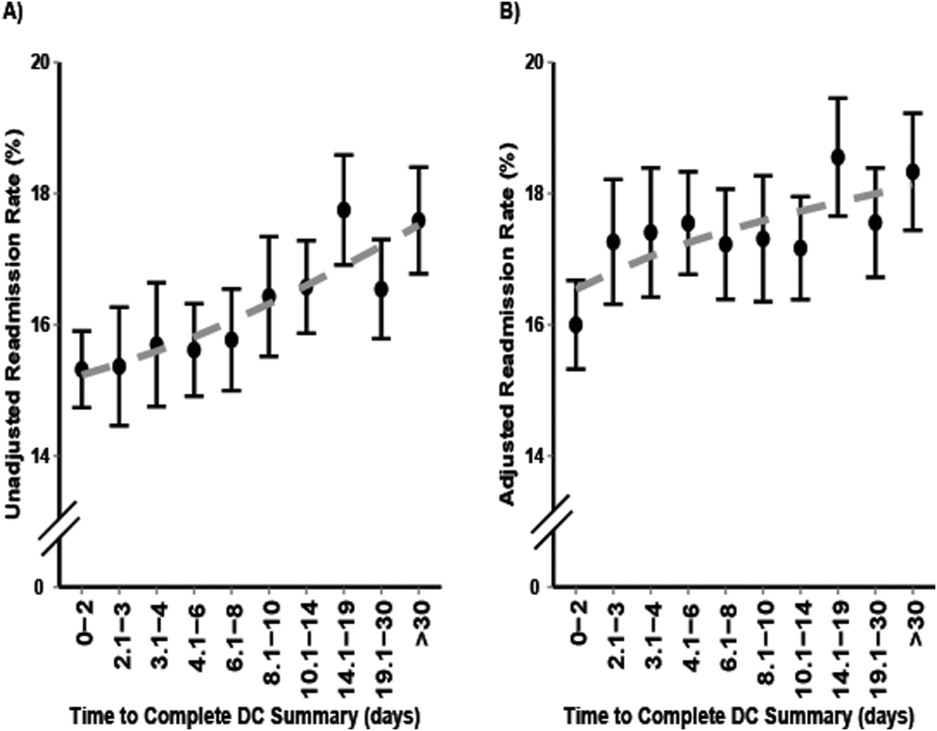
Multivariable and Secondary Analyses
In adjusted analysis (Table 2), patients discharged from an oncologic service relative to a medicine hospital service (OR: 1.19, 95% CI: 1.10 to 1.28, P < 0.001), patients discharged to a facility, home with homecare or hospice, or other location compared to home (facility OR: 1.11, 95% CI: 1.02 to 1.19, P = 0.009; home with homecare or hospice OR: 1.26, 95% CI: 1.19 to 1.34, P < 0.001; other OR: 1.25, 95% CI: 1.18 to 1.34, P < 0.001), patients with longer LOS (OR: 1.11 per day, 95% CI: 1.10 to 1.12, P < 0.001), patients with a higher expected readmission rates (OR: 1.01 per percent, 95% CI: 1.00 to 1.01, P < 0.001), and patients with a higher AHRQ comorbidity index (OR: 1.06 per 1 point, 95% CI: 1.06 to 1.06, P < 0.001) had higher 30‐day readmission rates. Overall, days to complete the discharge summary >3 days versus 3 days was associated with a higher readmission rate (OR: 1.09, 95% CI: 1.05 to 1.14, P < 0.001).
In a sensitivity analysis, discharge summary completion >8 days (median) versus 8 days was associated with higher unadjusted readmission rate (OR: 1.11, 95% CI: 1.07 to 1.15, P < 0.001) and a higher adjusted readmission rate (OR: 1.06, 95% CI: 1.02 to 1.10, P < 0.001). Discharge summary completion >14 days (70th percentile) versus 14 days was also associated with higher unadjusted readmission rate (OR: 1.15, 95% CI: 1.08 to 1.21, P < 0.001) and a higher adjusted readmission rate (OR: 1.09, 95% CI: 1.02 to 1.16, P = 0.008). The association between days to complete the discharge summary >3 days and readmissions was found to vary significantly by hospital service (P = 0.03). For comparing days to complete the discharge summary >3 versus 3 days, Table 3 shows that neurosciences, pediatrics, oncology, and medicine hospital services were associated with significantly increased adjusted mean readmission rates. Additionally, when days to complete the discharge summary was modeled as a continuous variable, we found that for every 3 days the odds of readmission increased by 1% (OR: 1.01, 95% CI: 1.00 to 1.01, P < 0.001).
Days to Complete Discharge Summary by Hospital Service | Adjusted Mean Readmission Rate (95% CI)* | P Value |
---|---|---|
| ||
Gynecologyobstetrics | 0.30 | |
03 days, n = 1,792 | 5.4 (4.1 to 6.7) | |
>3 days, n = 7,507 | 6.0 (4.9 to 7.0) | |
Medicine | 0.04 | |
03 days, n = 6,137 | 21.1 (20.0 to 22.3) | |
>3 days, n = 19,899 | 22.4 (21.6 to 23.2) | |
Neurosciences | 0.02 | |
03 days, n = 1,116 | 10.1 (8.2 to 12.1) | |
>3 days, n = 7,153 | 12.5 (11.6 to 13.5) | |
Oncology | 0.01 | |
03 days, n = 1,885 | 25.0 (22.6 to 27.4) | |
>3 days, n = 3,337 | 28.2 (26.6 to 30.2) | |
Pediatrics | 0.001 | |
03 days, n = 4,561 | 9.5 (6.9 to 12.2) | |
>3 days, n = 12,468 | 11.4 (8.9 to 13.9) | |
Surgical sciences | 0.89 | |
03 days, n = 6,261 | 15.2 (14.2 to 16.1) | |
>3 days, n = 15,878 | 15.1 (14.4 to 15.8) |
In an unadjusted analysis, we found that the relationship between LOS and days to complete the discharge summary was not significant ( coefficient and 95% CI:, 0.01, 0.02 to 0.00, P = 0.20). However, we found a small but significant relationship in our multivariable analysis, such that each hospitalization day was associated with a 0.01 (95% CI: 0.00 to 0.02, P = 0.03) increase in days to complete the discharge summary.
DISCUSSION
In this single‐center retrospective analysis, the number of days to complete the discharge summary was significantly associated with readmissions after hospitalization. This association was independent of age, gender, comorbidity index, payer, discharge location, length of hospital stay, expected readmission rate based on diagnosis and severity of illness, and all hospital services. The odds of readmission for patients with delayed discharge summaries was small but significant. This is important in the current landscape of readmissions, particularly for institutions who are challenged to reduce readmission rates, and a small relative difference in readmissions may be the difference between getting penalized or not. In the context of prior studies, the results highlight the role of timely discharge summary as an under‐recognized metric, which may be a valid litmus test for care coordination. The findings also emphasize the potential of early summaries to expedite communication and to help facilitate quality of patient care. Hence, the study results extend the literature examining the relationship of delay in discharge summary with unfavorable patient outcomes.[15, 32]
In contrast to prior reports with limited focus on same‐hospital readmissions,[18, 33, 34, 35] readmissions beyond 30 days,[12] or focused on a specific patient population,[13, 36] this study evaluates both intra‐ and interhospital 30‐day readmissions in Maryland in an all‐payer, multi‐institution, diverse patient population. Additionally, prior research is conflicting with respect to whether timely discharges summaries are significantly associated with increased hospital readmissions.[12, 13, 14, 15] Although it is not surprising that inadequate care during hospitalization could result in readmissions, the role of discharge summaries remain underappreciated. Having a timely discharge summary may not always prevent readmissions, but our study showed that 43% of readmission occurred before the discharge summary completion. Not having a completed discharge summary at the time of readmission may have been a driver for the positive association between timely completion and 30‐day readmission we observed. This study highlights that delay in the discharge summary could be a marker of poor transitions of care, because suboptimal dissemination of critical information to care providers may result in discontinuity of patient care posthospitalization.
A plausible mechanism of the association between discharge summary delays and readmissions could be the provision of collateral information, which may potentially alter the threshold for readmissions. For example, in the emergency room/emergency department (ER/ED) setting, discharge summaries may help with preventable readmissions. For patients who present repeatedly with the same complaint, timely summaries to ER/ED providers may help reframe the patient complaints, such as patient has concern X, which was previously identified to be related to diagnosis Y. As others have shown, the content of discharge summaries, format, and accessibility (electronic vs paper chart), as well as timely distribution of summaries, are key factors that impact quality outcomes.[2, 12, 15, 37, 38] By detailing prior hospital information (ie, discharge medications, prior presentations, tests completed), summaries could help prevent errors in medication dosing, reduce unnecessary testing, and help facilitate admission triage. Summaries may have information regarding a new diagnosis such as the results of an endoscopic evaluation that revealed the source of occult gastrointestinal bleeding, which could help contextualize a complaint of repeat melena and redirect goals of care. Discussions of goals of care in the discharge summary may guide primary providers in continued care management plans.
Our study findings underscore a positive correlation between late discharge summaries and readmissions. However, the extent that this is a causal relationship is unclear; the association of delay in days to complete the discharge summary with readmission may be an epiphenomenon related to processes related to quality of clinical care. For example, delays in discharge summary completion could be a marker of other system issues, such as a stressed work environment. It is possible that providers who fail to complete timely discharge summaries may also fail to do other important functions related to transitions of care and care coordination. However, even if this is so, timely discharge summaries could become a focal point for discussion for optimization of care transitions. A discharge summary could be delayed because the patient has already been readmitted before the summary was distributed, thus making that original summary less relevant. Delays could also be a reflection of the data complexity for patients with longer hospital stays. This is supported by the small but significant relationship between LOS and days to complete the discharge summary in this study. Lastly, delays in discharge summary completion may also be a proxy of provider communication and can reflect the culture of communication at the institution.
Although unplanned hospital readmission is an important outcome, many readmissions may be related to other factors such as disease progression, rather than late summaries or the lack of postdischarge communication. For instance, prior reports did not find any association between the PCP seeing the discharge summaries or direct communications with the PCP and 30‐day clinical outcomes for readmission and death.[26, 39] However, these studies were limited in their use of self‐reported handoffs, did not measure quality of information transfer, and failed to capture a broader audience beyond the PCP, such as ED physicians or specialists.
Our results suggest that the relationship between days to complete discharge summaries and 30‐day readmissions may vary depending on whether the hospitalization is primarily surgical/procedural versus medical treatment. A recent study found that most readmissions after surgery were associated with new complications related to the procedure and not exacerbation of prior index hospitalization complications.[40] Hence, treatment for common causes of hospital readmissions after surgical or gynecological procedures, such as wound infections, acute anemia, ileus, or dehydration, may not necessarily require a completed discharge summary for appropriate management. However, we caution extending this finding to clinical practice before further studies are conducted on specific procedures and in different clinical settings.
Results from this study also support institutional policies that specify the need for practitioners to complete discharge summaries contemporaneously, such as at the time of discharge or within a couple of days. Unlike other forms of communication that are optional, discharge summaries are required, so we recommend that practitioners be held accountable for short turnaround times. For example, providers could be graded and rated on timely completions of discharge summaries, among other performance variables. Anecdotally at our institutions, we have heard from practitioners that it takes less time to complete them when you do them on the day of discharge, because the hospitalization course is fresher in their mind and they have to wade through less information in the medical record to complete an accurate discharge summary. To this point, a barrier to on‐time completion is that providers may have misconceptions about what is really vital information to convey to the next provider. In agreement with past research and in the era of the electronic medical record system, we recommend that the discharge summary should be a quick synthesis of key findings that incorporates only the important elements, such as why the patient was hospitalized, what were key findings and key responses to therapy, what is pending at the time of discharge, what medications the patient is currently taking, and what are the follow‐up plans, rather than a lengthy expose of all the findings.[13, 36, 41, 42]
Lastly, our study results should be taken in the context of its limitations. As a single‐center study, findings may lack generalizability. In particular, the results may not generalize to hospitals that lack access to statewide reporting. We were also not able to assess readmission for patients who may have been readmitted to a hospital outside of Maryland. Although we adjusted for pertinent variables such as age, gender, healthcare payer, hospital service, comorbidity index, discharge location, LOS, and expected readmission rates, there may be other relevant confounders that we failed to capture or measure optimally. Median days to complete the discharge summary in this study was 8 days, which is longer than practices at other institutions, and may also limit this study's generalizability.[15, 36, 42] However, prior research supports our findings,[15] and a systematic review found that only 29% and 52% of discharge summaries were completed by 2 weeks and 4 weeks, respectively.[9] Finally, as noted above and perhaps most important, it is possible that discharge summary turnaround time does not in itself causally impact readmissions, but rather reflects an underlying commitment of the inpatient team to effectively coordinate care following hospital discharge.
CONCLUSION
In sum, this study delineates an underappreciated but important relationship of timely discharge summary completion and readmission outcomes. The discharge summary may be a relevant metric reflecting quality of patient care. Healthcare providers may begin to target timely discharge summaries as a potential focal point of quality‐improvement projects with the goal to facilitate better patient outcomes.
Disclosures
The authors certify that no party having a direct interest in the results of the research supporting this article has or will confer a benefit on us or on any organization with which we are associated, and, if applicable, the authors certify that all financial and material support for this research (eg, Centers for Medicare and Medicaid Services, National Institutes of Health, or National Health Service grants) and work are clearly identified. This study was supported by funding opportunity, number CMS‐1C1‐12‐0001, from the Centers for Medicare and Medicaid Services and Center for Medicare and Medicaid Innovation. Its contents are solely the responsibility of the authors and do not necessarily represent the official views of the Department of Health and Human Services or any of its agencies.
- Development and sustainability of an inpatient‐to‐outpatient discharge handoff tool: a quality improvement project. Jt Comm J Qual Patient Saf. 2014;40(5):219–227. , , , et al.
- Documentation of mandated discharge summary components in transitions from acute to subacute care. In: Henriksen K, Battles JB, Keyes MA, et al., eds. Advances in Patient Safety: New Directions and Alternative Approaches. Vol. 2. Culture and Redesign. Rockville, MD: Agency for Healthcare Research and Quality; 2008. , , , , , .
- Better transitions: improving comprehension of discharge instructions. Front Health Serv Manage. 2009;25(3):11–32. , , , .
- Structured discharge education improves early outcome in orthopedic patients. Int J Orthop Trauma Nurs. 2010;14(2):66–74. , , , .
- Hospital discharge documentation and risk of rehospitalisation. BMJ Qual Saf. 2011;20(9):773–778. , , , et al.
- The hospital discharge: a review of a high risk care transition with highlights of a reengineered discharge process. J Patient Saf. 2007;3(2):97–106. , , .
- Interventions to reduce 30‐day rehospitalization: a systematic review. Ann Intern Med. 2011;155(8):520–528. , , , , .
- Effect of hospital follow‐up appointment on clinical event outcomes and mortality. Arch Intern Med. 2010;170(11):955–960. , , , , , .
- Deficits in communication and information transfer between hospital‐based and primary care physicians: implications for patient safety and continuity of care. JAMA. 2007;297(8):831–841. , , , , , .
- Provider characteristics, clinical‐work processes and their relationship to discharge summary quality for sub‐acute care patients. J Gen Intern Med. 2012;27(1):78–84. , , , , .
- Contemporary evidence about hospital strategies for reducing 30‐day readmissions: a national study. J Am Coll Cardiol. 2012;60(7):607–614. , , , et al.
- Effect of discharge summary availability during post‐discharge visits on hospital readmission. J Gen Intern Med. 2002;17(3):186–192. , , , .
- Association of discharge summary quality with readmission risk for patients hospitalized with heart failure exacerbation. Circ Cardiovasc Qual Outcomes. 2015;8(1):109–111. , , , et al.
- The independent association of provider and information continuity on outcomes after hospital discharge: implications for hospitalists. J Hosp Med. 2010;5(7):398–405. , , , et al.
- Timeliness in discharge summary dissemination is associated with patients' clinical outcomes. J Eval Clin Pract. 2013;19(1):76–79. , , , , .
- Communication and information deficits in patients discharged to rehabilitation facilities: an evaluation of five acute care hospitals. J Hosp Med. 2009;4(8):E28–E33. , , , et al.
- Preventing readmissions through comprehensive discharge planning. Prof Case Manag. 2013;18(2):56–63; quiz 64–65. , , .
- Effect of a postdischarge virtual ward on readmission or death for high‐risk patients: a randomized clinical trial. JAMA. 2014;312(13):1305–1312.. , , , et al.
- Risk factors for early unplanned hospital readmission in the elderly. J Gen Intern Med. 1991;6(3):223–228. , , .
- Differences between early and late readmissions among patients: a cohort study. Ann Intern Med. 2015;162(11):741–749. , , , , .
- Post‐acute care payment reform demonstration report to congress supplement‐interim report. Centers for Medicare 14(3):1–14; quiz 88–89. , , , et al.
- Comprehensive discharge planning and home follow‐up of hospitalized elders: a randomized clinical trial. JAMA. 1999;281(7):613–620. , , , et al.
- Posthospital care transitions: patterns, complications, and risk identification. Health Serv Res. 2004;39(5):1449–1465. , , , .
- Transitions of care consensus policy statement: American College of Physicians, Society of General Internal Medicine, Society of Hospital Medicine, American Geriatrics Society, American College of Emergency Physicians, and Society for Academic Emergency Medicine. J Hosp Med. 2009;4(6):364–370. , , , et al.
- Association of self‐reported hospital discharge handoffs with 30‐day readmissions. JAMA Intern Med. 2013;173(8):624–629. , , , et al.
- Socioeconomic disparities in health: pathways and policies. Health Aff (Millwood). 2002;21(2):60–76. , .
- Comorbidity measures for use with administrative data. Med Care. 1998;36(1):8–27. , , , .
- Functional status impairment is associated with unplanned readmissions. Arch Phys Med Rehabil. 2013;94(10):1951–1958. , , , , , .
- Centers for Medicare 35(10):1044–1059.
- Understanding and execution of discharge instructions. Am J Med Qual. 2013;28(5):383–391. , , , et al.
- Beyond the hospital gates: elucidating the interactive association of social support, depressive symptoms, and physical function with 30‐day readmissions. Am J Phys Med Rehabil. 2015;94(7):555–567. , , , , .
- Improving the discharge process by embedding a discharge facilitator in a resident team. J Hosp Med. 2011;6(9):494–500. , , , et al.
- Hospital variation in quality of discharge summaries for patients hospitalized with heart failure exacerbation. Circ Cardiovasc Qual Outcomes. 2015;8(1):77–86 , , , et al.
- Addressing the business of discharge: building a case for an electronic discharge summary. J Hosp Med. 2011;6(1):37–42. , , , .
- Joint commission requirements for discharge instructions in patients with heart failure: is understanding important for preventing readmissions? J Card Fail. 2014;20(9):641–649. , , , , .
- Association of communication between hospital‐based physicians and primary care providers with patient outcomes. J Gen Intern Med. 2009;24(3):381–386. , , , et al.
- Underlying reasons associated with hospital readmission following surgery in the united states. JAMA. 2015;313(5):483–495. , , , et al.
- Assessing quality and efficiency of discharge summaries. Am J Med Qual. 2005;20(6):337–343. , , , , .
- Comprehensive quality of discharge summaries at an academic medical center. J Hosp Med. 2013;8(8):436–443. , , , et al.
- Development and sustainability of an inpatient‐to‐outpatient discharge handoff tool: a quality improvement project. Jt Comm J Qual Patient Saf. 2014;40(5):219–227. , , , et al.
- Documentation of mandated discharge summary components in transitions from acute to subacute care. In: Henriksen K, Battles JB, Keyes MA, et al., eds. Advances in Patient Safety: New Directions and Alternative Approaches. Vol. 2. Culture and Redesign. Rockville, MD: Agency for Healthcare Research and Quality; 2008. , , , , , .
- Better transitions: improving comprehension of discharge instructions. Front Health Serv Manage. 2009;25(3):11–32. , , , .
- Structured discharge education improves early outcome in orthopedic patients. Int J Orthop Trauma Nurs. 2010;14(2):66–74. , , , .
- Hospital discharge documentation and risk of rehospitalisation. BMJ Qual Saf. 2011;20(9):773–778. , , , et al.
- The hospital discharge: a review of a high risk care transition with highlights of a reengineered discharge process. J Patient Saf. 2007;3(2):97–106. , , .
- Interventions to reduce 30‐day rehospitalization: a systematic review. Ann Intern Med. 2011;155(8):520–528. , , , , .
- Effect of hospital follow‐up appointment on clinical event outcomes and mortality. Arch Intern Med. 2010;170(11):955–960. , , , , , .
- Deficits in communication and information transfer between hospital‐based and primary care physicians: implications for patient safety and continuity of care. JAMA. 2007;297(8):831–841. , , , , , .
- Provider characteristics, clinical‐work processes and their relationship to discharge summary quality for sub‐acute care patients. J Gen Intern Med. 2012;27(1):78–84. , , , , .
- Contemporary evidence about hospital strategies for reducing 30‐day readmissions: a national study. J Am Coll Cardiol. 2012;60(7):607–614. , , , et al.
- Effect of discharge summary availability during post‐discharge visits on hospital readmission. J Gen Intern Med. 2002;17(3):186–192. , , , .
- Association of discharge summary quality with readmission risk for patients hospitalized with heart failure exacerbation. Circ Cardiovasc Qual Outcomes. 2015;8(1):109–111. , , , et al.
- The independent association of provider and information continuity on outcomes after hospital discharge: implications for hospitalists. J Hosp Med. 2010;5(7):398–405. , , , et al.
- Timeliness in discharge summary dissemination is associated with patients' clinical outcomes. J Eval Clin Pract. 2013;19(1):76–79. , , , , .
- Communication and information deficits in patients discharged to rehabilitation facilities: an evaluation of five acute care hospitals. J Hosp Med. 2009;4(8):E28–E33. , , , et al.
- Preventing readmissions through comprehensive discharge planning. Prof Case Manag. 2013;18(2):56–63; quiz 64–65. , , .
- Effect of a postdischarge virtual ward on readmission or death for high‐risk patients: a randomized clinical trial. JAMA. 2014;312(13):1305–1312.. , , , et al.
- Risk factors for early unplanned hospital readmission in the elderly. J Gen Intern Med. 1991;6(3):223–228. , , .
- Differences between early and late readmissions among patients: a cohort study. Ann Intern Med. 2015;162(11):741–749. , , , , .
- Post‐acute care payment reform demonstration report to congress supplement‐interim report. Centers for Medicare 14(3):1–14; quiz 88–89. , , , et al.
- Comprehensive discharge planning and home follow‐up of hospitalized elders: a randomized clinical trial. JAMA. 1999;281(7):613–620. , , , et al.
- Posthospital care transitions: patterns, complications, and risk identification. Health Serv Res. 2004;39(5):1449–1465. , , , .
- Transitions of care consensus policy statement: American College of Physicians, Society of General Internal Medicine, Society of Hospital Medicine, American Geriatrics Society, American College of Emergency Physicians, and Society for Academic Emergency Medicine. J Hosp Med. 2009;4(6):364–370. , , , et al.
- Association of self‐reported hospital discharge handoffs with 30‐day readmissions. JAMA Intern Med. 2013;173(8):624–629. , , , et al.
- Socioeconomic disparities in health: pathways and policies. Health Aff (Millwood). 2002;21(2):60–76. , .
- Comorbidity measures for use with administrative data. Med Care. 1998;36(1):8–27. , , , .
- Functional status impairment is associated with unplanned readmissions. Arch Phys Med Rehabil. 2013;94(10):1951–1958. , , , , , .
- Centers for Medicare 35(10):1044–1059.
- Understanding and execution of discharge instructions. Am J Med Qual. 2013;28(5):383–391. , , , et al.
- Beyond the hospital gates: elucidating the interactive association of social support, depressive symptoms, and physical function with 30‐day readmissions. Am J Phys Med Rehabil. 2015;94(7):555–567. , , , , .
- Improving the discharge process by embedding a discharge facilitator in a resident team. J Hosp Med. 2011;6(9):494–500. , , , et al.
- Hospital variation in quality of discharge summaries for patients hospitalized with heart failure exacerbation. Circ Cardiovasc Qual Outcomes. 2015;8(1):77–86 , , , et al.
- Addressing the business of discharge: building a case for an electronic discharge summary. J Hosp Med. 2011;6(1):37–42. , , , .
- Joint commission requirements for discharge instructions in patients with heart failure: is understanding important for preventing readmissions? J Card Fail. 2014;20(9):641–649. , , , , .
- Association of communication between hospital‐based physicians and primary care providers with patient outcomes. J Gen Intern Med. 2009;24(3):381–386. , , , et al.
- Underlying reasons associated with hospital readmission following surgery in the united states. JAMA. 2015;313(5):483–495. , , , et al.
- Assessing quality and efficiency of discharge summaries. Am J Med Qual. 2005;20(6):337–343. , , , , .
- Comprehensive quality of discharge summaries at an academic medical center. J Hosp Med. 2013;8(8):436–443. , , , et al.
© 2016 Society of Hospital Medicine
Promoting Mobility and Reducing LOS
Annually, more than 35 million patients are hospitalized in the United States, with many experiencing hospital‐acquired impairments in physical functioning during their in‐patient stay.[1, 2, 3, 4] Such impairments include difficulties performing basic activities of daily living, such as rising from a chair, toileting, or ambulating. This functional decline may result in increased length of stay (LOS), nursing home placement, and decreased mobility and participation in community activities even years after hospitalization.[1, 2, 3, 5, 6, 7] Ameliorating this hospital‐acquired functional impairment is important to improving patient outcomes and reducing healthcare utilization. Even the sickest hospitalized patients (eg, those in the intensive care unit [ICU]), can safely and feasibly benefit from early mobilization.[6, 8, 9, 10, 11] In the non‐ICU setting there is also evidence that patient mobilization reduces LOS and hospital costs, while improving patient satisfaction and physical and psychological outcomes.[12, 13, 14, 15, 16] These studies are, however, difficult to replicate as part of routine clinical care, because they often do not present the details of how early mobility was incorporated into daily practice, require additional hospital resources (eg, specially trained providers or additional staff), or are focused only on a select patient population.
The Johns Hopkins medical ICU started early rehabilitation quality‐improvement (QI) work in 2007, which has demonstrated ongoing reductions in LOS and been transformative in terms of helping to foster a culture of mobility at our institution. Previous research suggests that ICU‐based rehabilitation interventions are often not carried over to the ward setting, even in post‐ICU patients.[17] Moreover, trends for sicker patients being admitted in our general medicine units,[18] growing reports of patients spending most of their time in bed,[2, 19, 20] and healthcare policies emphasizing the importance of improving inpatient outcomes motivated the need for QI to improve patient mobility in this setting. Experience from the medical ICU‐based early rehabilitation program helped drive multidisciplinary collaboration of stakeholders to develop this nurse‐driven, mobility promotion QI project on 2 general medicine hospital units. The main goals of the project were to see whether a QI framework can be used in a general medicine setting to increase patient mobility and reduce LOS.[21, 22]
METHODS
Overview of Project
Mobility, for this project, was defined as a patient getting out of bed (eg, sitting out of bed, toileting at bedside commode or bathroom, standing, and ambulating). We aimed to increase patient mobility using preexisting unit staffing ratios of clinicians and support staff. This project was reported in accordance with the SQUIRE (Standards for QUality Improvement Reporting Excellence) guidelines and used a structured QI model that had been used to successfully promote early mobility in the intensive care unit.[21, 23, 24, 25] The planning phase of the QI project began in spring 2012, with initiation of the 12‐month project on March 1, 2013. During the 12‐month QI period, prospective collection of mobility status occurred for all patients, with no exclusions based on patient characteristics.
Setting
The QI project setting was 2, 24‐bed, general medicine units at the Johns Hopkins Hospital, a large academic medical center located in Baltimore, Maryland.
QI Process
The primary goals of the QI project were to mobilize patients 3 times daily, quantify and document the mobility of the patients, set daily goals to increase mobility (eg, move up 1 step on the scale today), and standardize the description of patient mobility across all hospital staff. We used a structured QI model that that has been used to implement an early mobility program in a medical ICU at our institution[21, 22, 24] (see Supporting Information, Appendix, in the online version of this article). At a programmatic level, we involved key stakeholders (nurses, physicians, rehabilitation therapists, administrators) in the QI project team, we identified local barriers to implementation through team meetings as well as a survey tool to identify perceived barriers,[26] and we developed a scale (the Johns Hopkins Highest Level of Mobility [JH‐HLM]) to document mobility. The JH‐HLM is an 8‐point ordinal scale that captures mobility milestones, where 1 = only lying, 2 = bed activities, 3 = sit at edge of bed, 4 = transfer to chair/commode, 5 = standing for 1 minute, 6 = walking 10+ steps, 7 = walking 25+ feet, and 8 = walking 250+ feet (see Supporting Information, Appendix and Supporting Figure 1, in the online version of this article for additional information on the JH‐HLM scale).
The 12‐month QI project was characterized by several phases and milestones and involved a number of intervention components. During the first 4 months (ramp‐up phase), nurses received education in the form of unit‐based presentations, hands‐on‐training, and online education modules. On a 5‐times weekly basis, nurses met with rehabilitation therapists for unit‐based huddles to discuss baseline patient mobility, current patient mobility levels, barriers to mobilizing patients, and daily goals to progress mobility. Mobility levels were included on daily nursing report sheets to facilitate communication with subsequent shifts. Discussion of JH‐HLM scores also occurred during daily unit‐based care‐coordination meetings of the nurses, physicians, and social‐workers to address barriers to mobilizing patients, such as optimizing pain control, facilitating discharge location planning, and expediting physician consultation with physical and occupational therapy for appropriate patients. Audit and feedback from huddles and care‐coordination rounds resulted in improved nurse attendance and engagement during these meetings. Nurses were expected to document patient mobility scores using the JH‐HLM 3 times daily in the patient medical record. On the fourth month, reports on JH‐HLM scores and documentation compliance were available to nurse managers, champions, and unit staff. Via twice‐monthly meetings with the units and quarterly meetings with hospital leadership and administration, problems arising during the QI intervention were evaluated and resolved on a timely basis. Seven months after project execution started, educational sessions were repeated to all staff, and feedback was provided based on the data collected, such as documentation compliance rates and patient mobility levels, and nurse champions presented the project during an American Nurses Credentialing Center magnet recognition program visit. Lastly, mobility scores and documentation compliance were continually assessed for 4 months after the project completion to determine sustainability of the intervention. Additional details of the QI project implementation are provided in the Supporting Information, Appendix, in the online version of this article.
Data Sources and Covariates for Project Evaluation
The Sunrise Clinical Manager system (Allscripts Healthcare Solutions Inc., Chicago, IL) was used to document and extract nursing‐documented JH‐HLM scores. The Johns Hopkins Hospital Datamart financial database, used for mandatory reporting to the State of Maryland, provided data on LOS, age, sex, race (white, black, other), payer (Medicare, Medicaid, other), primary admission diagnosis, and comorbidity index using Agency for Healthcare Research and Quality (AHRQ) methodology.[27] Expected LOS was calculated using the risk adjustment method developed by the University Health System Consortium (UHC).[28] This calculation uses a combination of the Diagnostic‐Related Group grouper and the Sachs Complication Profiler[29] in conjunction with data on specific patient characteristics (age, sex, urgency of admission, payer category) to construct risk‐adjustment regression models that assign expected values for LOS, and is not based on actual LOS.[28] The databases were linked at the patient level using the patient's medical record and unique admission record number.
Outcome Measures
Two functional outcome measures were based on daily JH‐HLM scores, which frequently occurred several times on each patient‐day: (1) the maximum daily JH‐HLM scores for each patient‐day during hospitalization, and (2) the intrapatient change in JH‐HLM scores between the maximum JH‐HLM score within 24 hours of hospital admission and 24 hours before discharge for all patients who were on the unit >48 hours. We also compared the mean LOS during the 12‐month QI project versus the 12‐months prior so we could more accurately address seasonal differences.[30, 31, 32, 33, 34, 35] Lastly, because the perception of increased falls was an important barrier to address in the QI process, we compared the rate of injurious falls between the QI period and 12‐months prior.
Statistical Analysis
To evaluate changes in the percent of ambulatory patients (JH‐HLM 6), we compared the initial 4 months of the QI project (ramp‐up phase) with the same 4‐month period occurring immediately after project completion (post‐QI phase) using generalized estimating equations to account for clustering at the patient‐level. This test was also used to evaluate changes in documentation compliance rates between the 2 phases, with compliance defined as at least 1 instance of JH‐HLM documentation per day, excluding the day of admission and discharge. To evaluate if improved JH‐HLM results were driven by improved documentation compliance rates over time, we performed a sensitivity analysis by imputing a JH‐HLM score of 6 (ambulate 10+ steps) for any missing daily maximum JH‐HLM scores.
To assess unadjusted changes in LOS during the 12‐month QI project versus the same period 1 year earlier, we compared mean and median LOS using a t test and Wilcoxon rank sum test, respectively. We used a multivariable linear regression model to estimate the change (expressed in days) in adjusted median LOS comparing the project months (March 2013March 2014) with 12 months prior (March 2012March 2013). The model adjusted for age, gender, race, payer, admission diagnostic category, UHC expected LOS, and AHRQ comorbidity index. We confirmed a lack of multicollinearity in the multivariable regression model using variance inflation factors. We evaluated residual versus predicted value plots and residual versus fitted value plots with a locally weighted scatterplot smoothing line to confirm model fit. P values are reported from the test of the null hypothesis that the change in adjusted median LOS is the same comparing the QI project months versus 12 months prior. Separate models estimated and tested the change in adjusted median LOS by tertiles of expected LOS (<4, 47, and >7 days). Lastly, we compared the rate of injurious falls (the number of injurious falls by total patient‐days) between the QI period and 12 months prior using an exact Poisson method.[36] Statistical significance was defined as a 2‐sided P < 0.05. Statistical analyses were conducted using R (version 3.1.0; The R Foundation for Statistical Computing, Vienna, Austria;
RESULTS
During the QI project period, 3352 patients were admitted to the 2 general medicine units. Twelve (0.4%) patients expired on the units, but their data were retained in the analysis. Mean (standard deviation [SD]) age of the patients was 54.4 (18.3) years, with 47% male, and 54% African American. A total of 1896 of 6654 (28%) patients on the QI units were 65 years old. Patient characteristics were similar during the QI period versus 12 months prior (Table 1).
Characteristics | Comparison Period, March 2012March 2013, N = 3,302 | QI Period, March 2013March 2014, N = 3,352 |
---|---|---|
| ||
Age, y | 53.3 (17.8) | 54.4 (18.3) |
Male | 1467 (44%) | 1569 (47%) |
Race | ||
African American | 1883 (57%) | 1809 (54%) |
Caucasian | 1269 (38%) | 1348 (40%) |
Other | 150 (5%) | 195 (6%) |
Payer | ||
Medicare | 1310 (40%) | 1470 (44%) |
Medicaid | 1015 (31%) | 925 (28%) |
Other | 977 (30%) | 957 (29%) |
Admission diagnostic category | ||
Infectious disease | 579 (18%) | 629 (19%) |
Pulmonary | 519 (16%) | 559 (17%) |
Gastrointestinal | 535 (16%) | 494 (15%) |
Cardiovascular | 410 (12%) | 405 (12%) |
Hematologic | 199 (6%) | 195 (6%) |
Renal | 220 (7%) | 205 (6%) |
Other | 840 (25%) | 865 (26%) |
UHC expected length of stay, d | 5.5 (3.3) | 5.3 (3.2) |
AHRQ comorbidity index | 3.3 (1.7) | 3.5 (1.8) |
During the 12‐month QI project, there were a total of 13,815 patient‐days of documented mobility data and the median (interquartile range [IQR]) number of days of documentation for each hospital admission was 3 (25) days. Compliance with daily documentation of JH‐HLM was 85.0% over the entire 12‐month QI project. Documentation compliance started at 83% during the ramp‐up phase and increased to 89% during the last 4 months of the project (late‐QI phase, P < 0.001).
Comparing the ramp‐up phase versus post‐QI phase, the percentage of patient‐days in which patients ambulated (JH‐HLM 6) increased from 43% to 70% (P < 0.001), and the percentage of patients who experienced an improvement in their mobility scores between admission and discharge increased from 32% to 45% (P < 0.001), as shown in Table 2. In the sensitivity analysis imputing missing daily JH‐HLM scores and comparing the ramp‐up versus post‐QI phases, the results were similar to the primary analysis; the percent of patient‐days where patients ambulated increased from 60% to 78% (P < 0.001), and the percent of patients who experienced an improvement in their mobility scores increased from 26% to 48% (P < 0.001).
JH‐HLM Category | Ramp‐up Phase, March 1, 2013 June 30, 2013, n = 4,649 | Late‐QI Phase, November 1, 2013February 28, 2013, n = 4,515 | Post‐QI Phase, March 1, 2014 June 30, 2014, n = 4,298 |
---|---|---|---|
Change in Mobility (Admission Versus Discharge) | Ramp‐up Phase, March 1, 2013June 30, 2013, n = 968 | Late‐QI Phase, November 1, 2013February 28, 2013, n = 893 | Post‐QI Phase, March 1, 2014 June 30, 2014, n = 834 |
| |||
Walk (JH‐HLM = 6, 7, or 8) | 1,994 (43) | 3,430 (76) | 2,986 (70) |
Stand/chair (JH‐HLM = 4 or 5) | 1,772 (38) | 488 (10) | 511 (12) |
Bed (JH‐HLM = 1, 2, or 3) | 883 (19) | 597 (13) | 801 (19) |
Improved | 305 (32) | 392 (44) | 379 (45) |
No change | 512 (53) | 428 (48) | 386 (46) |
Declined | 151 (16) | 73 (8) | 69 (8) |
LOS during the 12‐month QI project versus the 12‐months immediately prior was shorter (Table 3), with an unadjusted median (IQR) LOS of 3 (26) versus 4 (27) days (P < 0.001) and an unadjusted mean (SD) LOS of 5.1 (5.6) versus 6.0 (7.6) (P < 0.001).
Adjusted Median LOS, d | Absolute Change in Adjusted Median LOS (95% CI), d | P Value | ||
---|---|---|---|---|
12 Months Prior | QI Project Months | |||
| ||||
All patients | 6.01 | 5.61 | 0.40 (0.57 to 0.21), N = 4,411 | <0.001 |
Subgroups by ELOS | ||||
ELOS <4 days | 4.68 | 4.77 | 0.09 (0.13 to 0.32), N = 1,357 | 0.42 |
ELOS 47 days | 5.68 | 5.38 | 0.30 (0.57 to 0.01), N = 1,509 | 0.04 |
ELOS >7 days | 8.07 | 6.96 | 1.11 (1.53 to 0.65), N = 1,545 | <0.001 |
Table 3 displays the change in adjusted median LOS for the project months versus the 12 months prior among the QI units. We found that for all patients, there was an overall reduction in adjusted median LOS of 0.40 (95% confidence interval [CI]: 0.57 to 0.21, P<0.001) days. When we divided patients into tertiles based on their UHC expected LOS (ELOS), we observed that patients with longer ELOS had greater reductions in adjusted median LOS. Patients on the QI units with ELOS <4 days (lowest tertile) did not show a significant reduction in adjusted median LOS (0.09 days, 95% CI: 0.13 to 0.32, P = 0.42); however, patients with UHC ELOS 4 to 7 days (middle tertile) and ELOS >7 days (highest tertile) had a significant reduction in adjusted median LOS by 0.30 (95% CI: 0.57 to 0.01, P = 0.04) and 1.11 (95% CI: 1.53 to 0.65, P < 0.001) days during the QI project versus 12 months prior, respectively.
Lastly, we found that there was no difference in the rate of injurious falls on the QI units during QI period compared to 12 months prior (QI: 0.34 per 1000 patient‐days versus 12 months prior: 0.48 per 1000 patient‐days, P = 0.73).
DISCUSSION
We conducted a nurse‐driven, multidisciplinary mobility promotion QI project on 2 general medicine units at a large teaching hospital. The 12‐month QI project, conducted between March 1, 2013 and February 28, 2014, was associated with patients ambulating more frequently, with improved mobility status between hospital admission and discharge. These improvements in mobility were not associated with increased rates of injurious falls, and were sustained for at least 4 months after project completion. The QI project was associated with overall significant reduction in LOS for more complex patients with longer expected LOS (4 days or longer). Hence, such QI efforts may be important for maintaining or improving patients' functional status during hospitalization in a safe and cost‐effective manner.
Our findings are consistent with previous studies showing that mobility promotion in the acute hospital setting is feasible, can reduce length of stay, and can be applied to a diverse population including vulnerable medical patients with multiple comorbidities and the elderly.[12, 16, 37, 38, 39, 40, 41, 42] These studies provide valuable evidence of the benefits of mobility promotion; however, it is difficult to translate these prior results into routine clinical practice because they used specially trained staff to mobilize patients, focused on a select patient population, or did not specify how the mobility intervention was delivered within daily clinical workflows. Research in the medical ICU at our institution has previously described the use of a structured QI model to successfully implement an early rehabilitation program.[22, 24] Here, we successfully adapted the same QI framework to a general medicine setting. Hence, our study contributes to the literature with respect to (1) use of a structured QI framework to develop a successful patient mobility program in a general medicine patient population, and (2) sharing best practices from 1 clinical setting, such as the ICU, as a source of learning and knowledge translation for other care settings, with the addition of novel tools, such as the JH‐HLM scale.
There may have been several factors that contributed to shorter stays in the hospital we observed during the QI project. First, we increased the number of ambulatory patient‐days, which may have helped prevent physiological complications of bed rest, such as muscle weakness, atelectasis, insulin resistance, vascular dysfunction, contractures, and pressure ulcers.[43] As such, mobility promotion has been associated with reduced rates of other hospital‐acquired complications, such as deep venous thrombosis, pneumonia, and delirium.[44, 45, 46] In our study, we saw the greatest LOS reduction in more complex patients who were expected to spend a longer time in the hospital and are at greater risk of developing complications from bed rest. Second, our early mobility project may have had a direct impact on care‐coordination processes as reported in prior studies.[47, 48, 49] An important component of our intervention was incorporating functional status into multidisciplinary discussions, either through nurse‐to‐therapist huddles or care‐coordination rounds between nurses, therapists, physicians, social workers, and case managers. During care‐coordination rounds, JH‐HLM scores were reported to expedite appropriate physical and occupational therapy consultations and assist in determining appropriate discharge location. During the QI project, we transitioned from a unit‐based daily huddle between nursing and rehabilitation therapists to a system where mobility status was discussed primarily during care coordination rounds 5 times per week. We saw that mobility scores were maintained after QI project completion, suggesting that reporting on patient function in a multidisciplinary setting is a potentially sustainable mechanism to improve care‐coordination processes that are affected by functional status.
Our study has several potential limitations. First, this is a single‐site study in 2 general medicine units of a large academic hospital. Further research is needed to determine if this structured QI intervention and its benefits can be generalized to different settings and different patient populations. Second, because the documentation was initially an optional element in the electronic medical record system, we observed higher rates of missing documentation during the first 4 months of the project versus the comparison period at 4 months after project completion. However, a sensitivity analysis conducted of these missing data demonstrated similar results to our primary analysis. Third, our nonrandomized pre‐post study design does not allow us to conclude a direct cause‐and‐effect relationship between our intervention and increased mobility and reduced LOS. Although patient characteristics were similar between the 2 periods and adjusted for in our multivariable regression analysis, we cannot rule out the possibility of secular trends in LOS on the project units and that broader QI efforts at our institution also contributed to reduction in LOS. Fourth, we do not have data on 30‐day readmissions and discharge location. Future studies should explore the impact of hospital‐based mobility interventions on these outcomes.[50] Fifth, although nurses consistently documented the highest level of mobility on a daily basis, these data did not capture other potentially important information about patient mobility such as the daily frequency that patients were mobilized, the length of time a patient was engaged in a mobility event (ie, number of hours sitting in a chair), or the mobility that occurred during physical therapy or occupational therapy sessions. Hence, although we used JH‐HLM as a marker of improved mobility during our QI project it is likely that our data cannot fully describe the total mobility and activity that patients experienced during hospitalization. Lastly, although the front‐line staff and QI team found the JH‐HLM scale to be a useful tool to measure and advance patient mobility, further studies are needed to evaluate the reliability and validity of this scale.
CONCLUSION
A structured QI process can improve patient mobility and may contribute to reduction in LOS, particularly for more complex patients in this setting. Active prevention of decline in physical function that commonly occurs during hospitalization may prove valuable for improving patient outcomes and reducing healthcare resource utilization.
Disclosures
The authors certify that no party having a direct interest in the results of the research supporting this article has or will confer a benefit on us or on any organization with which we are associated. The authors report no conflicts of interest.
- Loss of independence in activities of daily living in older adults hospitalized with medical illnesses: increased vulnerability with age. J Am Geriatr Soc. 2003;51(4):451–458. , , , et al.
- Prevalence and outcomes of low mobility in hospitalized older patients. J Am Geriatr Soc. 2004;52(8):1263–1270. , , .
- Trajectories of life‐space mobility after hospitalization. Ann Intern Med. 2009;150(6):372–378. , , , , , .
- Hospitalization‐associated disability: “She was probably able to ambulate, but I'm not sure”. JAMA. 2011;306(16):1782–1793. , , .
- Physical fitness and all‐cause mortality. A prospective study of healthy men and women. JAMA. 1989;262(17):2395–2401. , , , , , .
- Mobilizing patients in the intensive care unit: improving neuromuscular weakness and physical function. JAMA. 2008;300(14):1685–1690. .
- Mobility limitation in the older patient: a clinical review. JAMA. 2013;310(11):1168–1177. , .
- Early physical and occupational therapy in mechanically ventilated, critically ill patients: a randomised controlled trial. Lancet. 2009;373(9678):1874–1882. , , , et al.
- Technology to enhance physical rehabilitation of critically ill patients. Crit Care Med. 2009;37(10 suppl):S436–S441. , , .
- Receiving early mobility during an intensive care unit admission is a predictor of improved outcomes in acute respiratory failure. Am J Med Sci. 2011;341(5):373–377. , , , et al.
- Physiotherapy in intensive care: an updated systematic review. Chest. 2013;144(3):825–847. .
- Exercise for acutely hospitalised older medical patients. Cochrane Database Syst Rev. 2007;(1):CD005955. , , .
- Extra physical therapy reduces patient length of stay and improves functional outcomes and quality of life in people with acute or subacute conditions: a systematic review. Arch Phys Med Rehabil. 2011;92(9):1490–1500. , , .
- Impact of early mobilization protocol on the medical‐surgical inpatient population: an integrated review of literature. Clin Nurse Spec. 2012;26(2):87–94. , .
- Outcomes of inpatient mobilization: a literature review. J Clin Nurs. 2014;23(11–12):1486–1501. , , .
- The early mobility bundle: a simple enhancement of therapy which may reduce incidence of hospital‐acquired pneumonia and length of hospital stay. J Hosp Infect. 2014;88(1):34–39. , , , et al.
- Physical therapy on the wards after early physical activity and mobility in the intensive care unit. Phys Ther. 2012;92(12):1518–1523. , , , , .
- Impact of hospital variables on case mix index as a marker of disease severity. Popul Health Manag. 2014;17(1):28–34. , , , .
- Frequency of hallway ambulation by hospitalized older adults on medical units of an academic hospital. Geriatr Nurs. 2004;25(4):212–217. , , , , .
- Activity level of hospital medical inpatients: an observational study. Arch Gerontol Geriatr. 2012;55(2):417–421. , , .
- Translating evidence into practice: a model for large scale knowledge translation. BMJ. 2008;337:a1714. , , .
- Early physical medicine and rehabilitation for patients with acute respiratory failure: a quality improvement project. Arch Phys Med Rehabil. 2010;91(4):536–542. , , , et al.
- SQUIRE development group. Publication guidelines for quality improvement studies in health care: evolution of the SQUIRE project. BMJ. 2009;338:a3152. , , , , ;
- Rehabilitation quality improvement in an intensive care unit setting: implementation of a quality improvement model. Top Stroke Rehabil. 2010;17(4):271–281. , .
- ICU early mobilization: from recommendation to implementation at three medical centers. Crit Care Med. 2013;41(9 suppl 1):S69–S80. , , , .
- Barriers to early mobility of hospitalized general medicine patients: survey development and results. Am J Phys Med Rehabil. 2015;94(4):304–312. , , , .
- Comorbidity measures for use with administrative data. Med Care. 1998;36(1):8–27. , , , .
- UHC Clinical Information Management Risk Adjustment of the UHC Clinical Data Base. Chicago, IL: University HealthSystem Consortium; 1998.
- Sachs Complications Profiler, Version 1.0, User's Guide. Evanston, IL: Sachs Group; 1995.
- Assessing hospital‐associated deaths from discharge data. The role of length of stay and comorbidities. JAMA. 1988;260(15):2240–2246. , , .
- Annual rates of admission and seasonal variations in hospitalizations for heart failure. Eur J Heart Fail. 2002;4(6):779–786. , , , et al.
- Regional and seasonal variation in the length of hospital stay for chronic obstructive pulmonary disease in Finland. Int J Circumpolar Health. 2002;61(2):131–135. , , , .
- Canadian CABG Surgery Quality Indicator Consensus Panel. The identification and development of canadian coronary artery bypass graft surgery quality indicators. J Thorac Cardiovasc Surg. 2005;130(5):1257. , , , , , ;
- Quality of care and length of hospital stay among patients with stroke. Med Care. 2009;47(5):575–582. , , , .
- A systematic review of outcomes and quality measures in adult patients cared for by hospitalists vs nonhospitalists. Mayo Clin Proc. 2009;84(3):248–254. .
- Confidence intervals that match Fisher's exact or Blaker's exact tests. Biostatistics. 2010;11(2):373–374. .
- A multicomponent intervention to prevent delirium in hospitalized older patients. N Engl J Med. 1999;340(9):669–676. , , , et al.
- Early mobilization of patients hospitalized with community‐acquired pneumonia. Chest. 2003;124(3):883–889. , , , , .
- Effects of a walking intervention on fatigue‐related experiences of hospitalized acute myelogenous leukemia patients undergoing chemotherapy: a randomized controlled trial. J Pain Symptom Manage. 2008;35(5):524–534. , , , et al.
- Early ambulation and length of stay in older adults hospitalized for acute illness. Arch Intern Med. 2010;170(21):1942–1943. , , , , .
- Impact of a nurse‐driven mobility protocol on functional decline in hospitalized older adults. J Nurs Care Qual. 2009;24(4):325–331. , , .
- Exercising body and mind: an integrated approach to functional independence in hospitalized older people. J Am Geriatr Soc. 2008;56(4):630–635. , , .
- Consequences of bed rest. Crit Care Med. 2009;37(10 suppl):S422–S428. .
- Time to ambulation after hip fracture surgery: relation to hospitalization outcomes. J Gerontol A Biol Sci Med Sci. 2003;58(11):M1042–M1045. , , , , .
- Early mobilization after total knee replacement reduces the incidence of deep venous thrombosis. ANZ J Surg. 2009;79(7–8):526–529. , , , .
- Efficacy and safety of postoperative early mobilization for chronic subdural hematoma in elderly patients. Acta Neurochir (Wien). 2010;152(7):1171–1174. , , , .
- Impact of relational coordination on quality of care, postoperative pain and functioning, and length of stay: a nine‐hospital study of surgical patients. Med Care. 2000;38(8):807–819. , , , et al.
- Care coordination cuts admissions, ED visits, LOS. Hosp Case Manag. 2013;21(5):67–68.
- A heart failure initiative to reduce the length of stay and readmission rates. Prof Case Manag. 2014;19(6):276–284. , .
- Association of impaired functional status at hospital discharge and subsequent rehospitalization. J Hosp Med. 2014;9(5):277–282. , , , , , .
Annually, more than 35 million patients are hospitalized in the United States, with many experiencing hospital‐acquired impairments in physical functioning during their in‐patient stay.[1, 2, 3, 4] Such impairments include difficulties performing basic activities of daily living, such as rising from a chair, toileting, or ambulating. This functional decline may result in increased length of stay (LOS), nursing home placement, and decreased mobility and participation in community activities even years after hospitalization.[1, 2, 3, 5, 6, 7] Ameliorating this hospital‐acquired functional impairment is important to improving patient outcomes and reducing healthcare utilization. Even the sickest hospitalized patients (eg, those in the intensive care unit [ICU]), can safely and feasibly benefit from early mobilization.[6, 8, 9, 10, 11] In the non‐ICU setting there is also evidence that patient mobilization reduces LOS and hospital costs, while improving patient satisfaction and physical and psychological outcomes.[12, 13, 14, 15, 16] These studies are, however, difficult to replicate as part of routine clinical care, because they often do not present the details of how early mobility was incorporated into daily practice, require additional hospital resources (eg, specially trained providers or additional staff), or are focused only on a select patient population.
The Johns Hopkins medical ICU started early rehabilitation quality‐improvement (QI) work in 2007, which has demonstrated ongoing reductions in LOS and been transformative in terms of helping to foster a culture of mobility at our institution. Previous research suggests that ICU‐based rehabilitation interventions are often not carried over to the ward setting, even in post‐ICU patients.[17] Moreover, trends for sicker patients being admitted in our general medicine units,[18] growing reports of patients spending most of their time in bed,[2, 19, 20] and healthcare policies emphasizing the importance of improving inpatient outcomes motivated the need for QI to improve patient mobility in this setting. Experience from the medical ICU‐based early rehabilitation program helped drive multidisciplinary collaboration of stakeholders to develop this nurse‐driven, mobility promotion QI project on 2 general medicine hospital units. The main goals of the project were to see whether a QI framework can be used in a general medicine setting to increase patient mobility and reduce LOS.[21, 22]
METHODS
Overview of Project
Mobility, for this project, was defined as a patient getting out of bed (eg, sitting out of bed, toileting at bedside commode or bathroom, standing, and ambulating). We aimed to increase patient mobility using preexisting unit staffing ratios of clinicians and support staff. This project was reported in accordance with the SQUIRE (Standards for QUality Improvement Reporting Excellence) guidelines and used a structured QI model that had been used to successfully promote early mobility in the intensive care unit.[21, 23, 24, 25] The planning phase of the QI project began in spring 2012, with initiation of the 12‐month project on March 1, 2013. During the 12‐month QI period, prospective collection of mobility status occurred for all patients, with no exclusions based on patient characteristics.
Setting
The QI project setting was 2, 24‐bed, general medicine units at the Johns Hopkins Hospital, a large academic medical center located in Baltimore, Maryland.
QI Process
The primary goals of the QI project were to mobilize patients 3 times daily, quantify and document the mobility of the patients, set daily goals to increase mobility (eg, move up 1 step on the scale today), and standardize the description of patient mobility across all hospital staff. We used a structured QI model that that has been used to implement an early mobility program in a medical ICU at our institution[21, 22, 24] (see Supporting Information, Appendix, in the online version of this article). At a programmatic level, we involved key stakeholders (nurses, physicians, rehabilitation therapists, administrators) in the QI project team, we identified local barriers to implementation through team meetings as well as a survey tool to identify perceived barriers,[26] and we developed a scale (the Johns Hopkins Highest Level of Mobility [JH‐HLM]) to document mobility. The JH‐HLM is an 8‐point ordinal scale that captures mobility milestones, where 1 = only lying, 2 = bed activities, 3 = sit at edge of bed, 4 = transfer to chair/commode, 5 = standing for 1 minute, 6 = walking 10+ steps, 7 = walking 25+ feet, and 8 = walking 250+ feet (see Supporting Information, Appendix and Supporting Figure 1, in the online version of this article for additional information on the JH‐HLM scale).
The 12‐month QI project was characterized by several phases and milestones and involved a number of intervention components. During the first 4 months (ramp‐up phase), nurses received education in the form of unit‐based presentations, hands‐on‐training, and online education modules. On a 5‐times weekly basis, nurses met with rehabilitation therapists for unit‐based huddles to discuss baseline patient mobility, current patient mobility levels, barriers to mobilizing patients, and daily goals to progress mobility. Mobility levels were included on daily nursing report sheets to facilitate communication with subsequent shifts. Discussion of JH‐HLM scores also occurred during daily unit‐based care‐coordination meetings of the nurses, physicians, and social‐workers to address barriers to mobilizing patients, such as optimizing pain control, facilitating discharge location planning, and expediting physician consultation with physical and occupational therapy for appropriate patients. Audit and feedback from huddles and care‐coordination rounds resulted in improved nurse attendance and engagement during these meetings. Nurses were expected to document patient mobility scores using the JH‐HLM 3 times daily in the patient medical record. On the fourth month, reports on JH‐HLM scores and documentation compliance were available to nurse managers, champions, and unit staff. Via twice‐monthly meetings with the units and quarterly meetings with hospital leadership and administration, problems arising during the QI intervention were evaluated and resolved on a timely basis. Seven months after project execution started, educational sessions were repeated to all staff, and feedback was provided based on the data collected, such as documentation compliance rates and patient mobility levels, and nurse champions presented the project during an American Nurses Credentialing Center magnet recognition program visit. Lastly, mobility scores and documentation compliance were continually assessed for 4 months after the project completion to determine sustainability of the intervention. Additional details of the QI project implementation are provided in the Supporting Information, Appendix, in the online version of this article.
Data Sources and Covariates for Project Evaluation
The Sunrise Clinical Manager system (Allscripts Healthcare Solutions Inc., Chicago, IL) was used to document and extract nursing‐documented JH‐HLM scores. The Johns Hopkins Hospital Datamart financial database, used for mandatory reporting to the State of Maryland, provided data on LOS, age, sex, race (white, black, other), payer (Medicare, Medicaid, other), primary admission diagnosis, and comorbidity index using Agency for Healthcare Research and Quality (AHRQ) methodology.[27] Expected LOS was calculated using the risk adjustment method developed by the University Health System Consortium (UHC).[28] This calculation uses a combination of the Diagnostic‐Related Group grouper and the Sachs Complication Profiler[29] in conjunction with data on specific patient characteristics (age, sex, urgency of admission, payer category) to construct risk‐adjustment regression models that assign expected values for LOS, and is not based on actual LOS.[28] The databases were linked at the patient level using the patient's medical record and unique admission record number.
Outcome Measures
Two functional outcome measures were based on daily JH‐HLM scores, which frequently occurred several times on each patient‐day: (1) the maximum daily JH‐HLM scores for each patient‐day during hospitalization, and (2) the intrapatient change in JH‐HLM scores between the maximum JH‐HLM score within 24 hours of hospital admission and 24 hours before discharge for all patients who were on the unit >48 hours. We also compared the mean LOS during the 12‐month QI project versus the 12‐months prior so we could more accurately address seasonal differences.[30, 31, 32, 33, 34, 35] Lastly, because the perception of increased falls was an important barrier to address in the QI process, we compared the rate of injurious falls between the QI period and 12‐months prior.
Statistical Analysis
To evaluate changes in the percent of ambulatory patients (JH‐HLM 6), we compared the initial 4 months of the QI project (ramp‐up phase) with the same 4‐month period occurring immediately after project completion (post‐QI phase) using generalized estimating equations to account for clustering at the patient‐level. This test was also used to evaluate changes in documentation compliance rates between the 2 phases, with compliance defined as at least 1 instance of JH‐HLM documentation per day, excluding the day of admission and discharge. To evaluate if improved JH‐HLM results were driven by improved documentation compliance rates over time, we performed a sensitivity analysis by imputing a JH‐HLM score of 6 (ambulate 10+ steps) for any missing daily maximum JH‐HLM scores.
To assess unadjusted changes in LOS during the 12‐month QI project versus the same period 1 year earlier, we compared mean and median LOS using a t test and Wilcoxon rank sum test, respectively. We used a multivariable linear regression model to estimate the change (expressed in days) in adjusted median LOS comparing the project months (March 2013March 2014) with 12 months prior (March 2012March 2013). The model adjusted for age, gender, race, payer, admission diagnostic category, UHC expected LOS, and AHRQ comorbidity index. We confirmed a lack of multicollinearity in the multivariable regression model using variance inflation factors. We evaluated residual versus predicted value plots and residual versus fitted value plots with a locally weighted scatterplot smoothing line to confirm model fit. P values are reported from the test of the null hypothesis that the change in adjusted median LOS is the same comparing the QI project months versus 12 months prior. Separate models estimated and tested the change in adjusted median LOS by tertiles of expected LOS (<4, 47, and >7 days). Lastly, we compared the rate of injurious falls (the number of injurious falls by total patient‐days) between the QI period and 12 months prior using an exact Poisson method.[36] Statistical significance was defined as a 2‐sided P < 0.05. Statistical analyses were conducted using R (version 3.1.0; The R Foundation for Statistical Computing, Vienna, Austria;
RESULTS
During the QI project period, 3352 patients were admitted to the 2 general medicine units. Twelve (0.4%) patients expired on the units, but their data were retained in the analysis. Mean (standard deviation [SD]) age of the patients was 54.4 (18.3) years, with 47% male, and 54% African American. A total of 1896 of 6654 (28%) patients on the QI units were 65 years old. Patient characteristics were similar during the QI period versus 12 months prior (Table 1).
Characteristics | Comparison Period, March 2012March 2013, N = 3,302 | QI Period, March 2013March 2014, N = 3,352 |
---|---|---|
| ||
Age, y | 53.3 (17.8) | 54.4 (18.3) |
Male | 1467 (44%) | 1569 (47%) |
Race | ||
African American | 1883 (57%) | 1809 (54%) |
Caucasian | 1269 (38%) | 1348 (40%) |
Other | 150 (5%) | 195 (6%) |
Payer | ||
Medicare | 1310 (40%) | 1470 (44%) |
Medicaid | 1015 (31%) | 925 (28%) |
Other | 977 (30%) | 957 (29%) |
Admission diagnostic category | ||
Infectious disease | 579 (18%) | 629 (19%) |
Pulmonary | 519 (16%) | 559 (17%) |
Gastrointestinal | 535 (16%) | 494 (15%) |
Cardiovascular | 410 (12%) | 405 (12%) |
Hematologic | 199 (6%) | 195 (6%) |
Renal | 220 (7%) | 205 (6%) |
Other | 840 (25%) | 865 (26%) |
UHC expected length of stay, d | 5.5 (3.3) | 5.3 (3.2) |
AHRQ comorbidity index | 3.3 (1.7) | 3.5 (1.8) |
During the 12‐month QI project, there were a total of 13,815 patient‐days of documented mobility data and the median (interquartile range [IQR]) number of days of documentation for each hospital admission was 3 (25) days. Compliance with daily documentation of JH‐HLM was 85.0% over the entire 12‐month QI project. Documentation compliance started at 83% during the ramp‐up phase and increased to 89% during the last 4 months of the project (late‐QI phase, P < 0.001).
Comparing the ramp‐up phase versus post‐QI phase, the percentage of patient‐days in which patients ambulated (JH‐HLM 6) increased from 43% to 70% (P < 0.001), and the percentage of patients who experienced an improvement in their mobility scores between admission and discharge increased from 32% to 45% (P < 0.001), as shown in Table 2. In the sensitivity analysis imputing missing daily JH‐HLM scores and comparing the ramp‐up versus post‐QI phases, the results were similar to the primary analysis; the percent of patient‐days where patients ambulated increased from 60% to 78% (P < 0.001), and the percent of patients who experienced an improvement in their mobility scores increased from 26% to 48% (P < 0.001).
JH‐HLM Category | Ramp‐up Phase, March 1, 2013 June 30, 2013, n = 4,649 | Late‐QI Phase, November 1, 2013February 28, 2013, n = 4,515 | Post‐QI Phase, March 1, 2014 June 30, 2014, n = 4,298 |
---|---|---|---|
Change in Mobility (Admission Versus Discharge) | Ramp‐up Phase, March 1, 2013June 30, 2013, n = 968 | Late‐QI Phase, November 1, 2013February 28, 2013, n = 893 | Post‐QI Phase, March 1, 2014 June 30, 2014, n = 834 |
| |||
Walk (JH‐HLM = 6, 7, or 8) | 1,994 (43) | 3,430 (76) | 2,986 (70) |
Stand/chair (JH‐HLM = 4 or 5) | 1,772 (38) | 488 (10) | 511 (12) |
Bed (JH‐HLM = 1, 2, or 3) | 883 (19) | 597 (13) | 801 (19) |
Improved | 305 (32) | 392 (44) | 379 (45) |
No change | 512 (53) | 428 (48) | 386 (46) |
Declined | 151 (16) | 73 (8) | 69 (8) |
LOS during the 12‐month QI project versus the 12‐months immediately prior was shorter (Table 3), with an unadjusted median (IQR) LOS of 3 (26) versus 4 (27) days (P < 0.001) and an unadjusted mean (SD) LOS of 5.1 (5.6) versus 6.0 (7.6) (P < 0.001).
Adjusted Median LOS, d | Absolute Change in Adjusted Median LOS (95% CI), d | P Value | ||
---|---|---|---|---|
12 Months Prior | QI Project Months | |||
| ||||
All patients | 6.01 | 5.61 | 0.40 (0.57 to 0.21), N = 4,411 | <0.001 |
Subgroups by ELOS | ||||
ELOS <4 days | 4.68 | 4.77 | 0.09 (0.13 to 0.32), N = 1,357 | 0.42 |
ELOS 47 days | 5.68 | 5.38 | 0.30 (0.57 to 0.01), N = 1,509 | 0.04 |
ELOS >7 days | 8.07 | 6.96 | 1.11 (1.53 to 0.65), N = 1,545 | <0.001 |
Table 3 displays the change in adjusted median LOS for the project months versus the 12 months prior among the QI units. We found that for all patients, there was an overall reduction in adjusted median LOS of 0.40 (95% confidence interval [CI]: 0.57 to 0.21, P<0.001) days. When we divided patients into tertiles based on their UHC expected LOS (ELOS), we observed that patients with longer ELOS had greater reductions in adjusted median LOS. Patients on the QI units with ELOS <4 days (lowest tertile) did not show a significant reduction in adjusted median LOS (0.09 days, 95% CI: 0.13 to 0.32, P = 0.42); however, patients with UHC ELOS 4 to 7 days (middle tertile) and ELOS >7 days (highest tertile) had a significant reduction in adjusted median LOS by 0.30 (95% CI: 0.57 to 0.01, P = 0.04) and 1.11 (95% CI: 1.53 to 0.65, P < 0.001) days during the QI project versus 12 months prior, respectively.
Lastly, we found that there was no difference in the rate of injurious falls on the QI units during QI period compared to 12 months prior (QI: 0.34 per 1000 patient‐days versus 12 months prior: 0.48 per 1000 patient‐days, P = 0.73).
DISCUSSION
We conducted a nurse‐driven, multidisciplinary mobility promotion QI project on 2 general medicine units at a large teaching hospital. The 12‐month QI project, conducted between March 1, 2013 and February 28, 2014, was associated with patients ambulating more frequently, with improved mobility status between hospital admission and discharge. These improvements in mobility were not associated with increased rates of injurious falls, and were sustained for at least 4 months after project completion. The QI project was associated with overall significant reduction in LOS for more complex patients with longer expected LOS (4 days or longer). Hence, such QI efforts may be important for maintaining or improving patients' functional status during hospitalization in a safe and cost‐effective manner.
Our findings are consistent with previous studies showing that mobility promotion in the acute hospital setting is feasible, can reduce length of stay, and can be applied to a diverse population including vulnerable medical patients with multiple comorbidities and the elderly.[12, 16, 37, 38, 39, 40, 41, 42] These studies provide valuable evidence of the benefits of mobility promotion; however, it is difficult to translate these prior results into routine clinical practice because they used specially trained staff to mobilize patients, focused on a select patient population, or did not specify how the mobility intervention was delivered within daily clinical workflows. Research in the medical ICU at our institution has previously described the use of a structured QI model to successfully implement an early rehabilitation program.[22, 24] Here, we successfully adapted the same QI framework to a general medicine setting. Hence, our study contributes to the literature with respect to (1) use of a structured QI framework to develop a successful patient mobility program in a general medicine patient population, and (2) sharing best practices from 1 clinical setting, such as the ICU, as a source of learning and knowledge translation for other care settings, with the addition of novel tools, such as the JH‐HLM scale.
There may have been several factors that contributed to shorter stays in the hospital we observed during the QI project. First, we increased the number of ambulatory patient‐days, which may have helped prevent physiological complications of bed rest, such as muscle weakness, atelectasis, insulin resistance, vascular dysfunction, contractures, and pressure ulcers.[43] As such, mobility promotion has been associated with reduced rates of other hospital‐acquired complications, such as deep venous thrombosis, pneumonia, and delirium.[44, 45, 46] In our study, we saw the greatest LOS reduction in more complex patients who were expected to spend a longer time in the hospital and are at greater risk of developing complications from bed rest. Second, our early mobility project may have had a direct impact on care‐coordination processes as reported in prior studies.[47, 48, 49] An important component of our intervention was incorporating functional status into multidisciplinary discussions, either through nurse‐to‐therapist huddles or care‐coordination rounds between nurses, therapists, physicians, social workers, and case managers. During care‐coordination rounds, JH‐HLM scores were reported to expedite appropriate physical and occupational therapy consultations and assist in determining appropriate discharge location. During the QI project, we transitioned from a unit‐based daily huddle between nursing and rehabilitation therapists to a system where mobility status was discussed primarily during care coordination rounds 5 times per week. We saw that mobility scores were maintained after QI project completion, suggesting that reporting on patient function in a multidisciplinary setting is a potentially sustainable mechanism to improve care‐coordination processes that are affected by functional status.
Our study has several potential limitations. First, this is a single‐site study in 2 general medicine units of a large academic hospital. Further research is needed to determine if this structured QI intervention and its benefits can be generalized to different settings and different patient populations. Second, because the documentation was initially an optional element in the electronic medical record system, we observed higher rates of missing documentation during the first 4 months of the project versus the comparison period at 4 months after project completion. However, a sensitivity analysis conducted of these missing data demonstrated similar results to our primary analysis. Third, our nonrandomized pre‐post study design does not allow us to conclude a direct cause‐and‐effect relationship between our intervention and increased mobility and reduced LOS. Although patient characteristics were similar between the 2 periods and adjusted for in our multivariable regression analysis, we cannot rule out the possibility of secular trends in LOS on the project units and that broader QI efforts at our institution also contributed to reduction in LOS. Fourth, we do not have data on 30‐day readmissions and discharge location. Future studies should explore the impact of hospital‐based mobility interventions on these outcomes.[50] Fifth, although nurses consistently documented the highest level of mobility on a daily basis, these data did not capture other potentially important information about patient mobility such as the daily frequency that patients were mobilized, the length of time a patient was engaged in a mobility event (ie, number of hours sitting in a chair), or the mobility that occurred during physical therapy or occupational therapy sessions. Hence, although we used JH‐HLM as a marker of improved mobility during our QI project it is likely that our data cannot fully describe the total mobility and activity that patients experienced during hospitalization. Lastly, although the front‐line staff and QI team found the JH‐HLM scale to be a useful tool to measure and advance patient mobility, further studies are needed to evaluate the reliability and validity of this scale.
CONCLUSION
A structured QI process can improve patient mobility and may contribute to reduction in LOS, particularly for more complex patients in this setting. Active prevention of decline in physical function that commonly occurs during hospitalization may prove valuable for improving patient outcomes and reducing healthcare resource utilization.
Disclosures
The authors certify that no party having a direct interest in the results of the research supporting this article has or will confer a benefit on us or on any organization with which we are associated. The authors report no conflicts of interest.
Annually, more than 35 million patients are hospitalized in the United States, with many experiencing hospital‐acquired impairments in physical functioning during their in‐patient stay.[1, 2, 3, 4] Such impairments include difficulties performing basic activities of daily living, such as rising from a chair, toileting, or ambulating. This functional decline may result in increased length of stay (LOS), nursing home placement, and decreased mobility and participation in community activities even years after hospitalization.[1, 2, 3, 5, 6, 7] Ameliorating this hospital‐acquired functional impairment is important to improving patient outcomes and reducing healthcare utilization. Even the sickest hospitalized patients (eg, those in the intensive care unit [ICU]), can safely and feasibly benefit from early mobilization.[6, 8, 9, 10, 11] In the non‐ICU setting there is also evidence that patient mobilization reduces LOS and hospital costs, while improving patient satisfaction and physical and psychological outcomes.[12, 13, 14, 15, 16] These studies are, however, difficult to replicate as part of routine clinical care, because they often do not present the details of how early mobility was incorporated into daily practice, require additional hospital resources (eg, specially trained providers or additional staff), or are focused only on a select patient population.
The Johns Hopkins medical ICU started early rehabilitation quality‐improvement (QI) work in 2007, which has demonstrated ongoing reductions in LOS and been transformative in terms of helping to foster a culture of mobility at our institution. Previous research suggests that ICU‐based rehabilitation interventions are often not carried over to the ward setting, even in post‐ICU patients.[17] Moreover, trends for sicker patients being admitted in our general medicine units,[18] growing reports of patients spending most of their time in bed,[2, 19, 20] and healthcare policies emphasizing the importance of improving inpatient outcomes motivated the need for QI to improve patient mobility in this setting. Experience from the medical ICU‐based early rehabilitation program helped drive multidisciplinary collaboration of stakeholders to develop this nurse‐driven, mobility promotion QI project on 2 general medicine hospital units. The main goals of the project were to see whether a QI framework can be used in a general medicine setting to increase patient mobility and reduce LOS.[21, 22]
METHODS
Overview of Project
Mobility, for this project, was defined as a patient getting out of bed (eg, sitting out of bed, toileting at bedside commode or bathroom, standing, and ambulating). We aimed to increase patient mobility using preexisting unit staffing ratios of clinicians and support staff. This project was reported in accordance with the SQUIRE (Standards for QUality Improvement Reporting Excellence) guidelines and used a structured QI model that had been used to successfully promote early mobility in the intensive care unit.[21, 23, 24, 25] The planning phase of the QI project began in spring 2012, with initiation of the 12‐month project on March 1, 2013. During the 12‐month QI period, prospective collection of mobility status occurred for all patients, with no exclusions based on patient characteristics.
Setting
The QI project setting was 2, 24‐bed, general medicine units at the Johns Hopkins Hospital, a large academic medical center located in Baltimore, Maryland.
QI Process
The primary goals of the QI project were to mobilize patients 3 times daily, quantify and document the mobility of the patients, set daily goals to increase mobility (eg, move up 1 step on the scale today), and standardize the description of patient mobility across all hospital staff. We used a structured QI model that that has been used to implement an early mobility program in a medical ICU at our institution[21, 22, 24] (see Supporting Information, Appendix, in the online version of this article). At a programmatic level, we involved key stakeholders (nurses, physicians, rehabilitation therapists, administrators) in the QI project team, we identified local barriers to implementation through team meetings as well as a survey tool to identify perceived barriers,[26] and we developed a scale (the Johns Hopkins Highest Level of Mobility [JH‐HLM]) to document mobility. The JH‐HLM is an 8‐point ordinal scale that captures mobility milestones, where 1 = only lying, 2 = bed activities, 3 = sit at edge of bed, 4 = transfer to chair/commode, 5 = standing for 1 minute, 6 = walking 10+ steps, 7 = walking 25+ feet, and 8 = walking 250+ feet (see Supporting Information, Appendix and Supporting Figure 1, in the online version of this article for additional information on the JH‐HLM scale).
The 12‐month QI project was characterized by several phases and milestones and involved a number of intervention components. During the first 4 months (ramp‐up phase), nurses received education in the form of unit‐based presentations, hands‐on‐training, and online education modules. On a 5‐times weekly basis, nurses met with rehabilitation therapists for unit‐based huddles to discuss baseline patient mobility, current patient mobility levels, barriers to mobilizing patients, and daily goals to progress mobility. Mobility levels were included on daily nursing report sheets to facilitate communication with subsequent shifts. Discussion of JH‐HLM scores also occurred during daily unit‐based care‐coordination meetings of the nurses, physicians, and social‐workers to address barriers to mobilizing patients, such as optimizing pain control, facilitating discharge location planning, and expediting physician consultation with physical and occupational therapy for appropriate patients. Audit and feedback from huddles and care‐coordination rounds resulted in improved nurse attendance and engagement during these meetings. Nurses were expected to document patient mobility scores using the JH‐HLM 3 times daily in the patient medical record. On the fourth month, reports on JH‐HLM scores and documentation compliance were available to nurse managers, champions, and unit staff. Via twice‐monthly meetings with the units and quarterly meetings with hospital leadership and administration, problems arising during the QI intervention were evaluated and resolved on a timely basis. Seven months after project execution started, educational sessions were repeated to all staff, and feedback was provided based on the data collected, such as documentation compliance rates and patient mobility levels, and nurse champions presented the project during an American Nurses Credentialing Center magnet recognition program visit. Lastly, mobility scores and documentation compliance were continually assessed for 4 months after the project completion to determine sustainability of the intervention. Additional details of the QI project implementation are provided in the Supporting Information, Appendix, in the online version of this article.
Data Sources and Covariates for Project Evaluation
The Sunrise Clinical Manager system (Allscripts Healthcare Solutions Inc., Chicago, IL) was used to document and extract nursing‐documented JH‐HLM scores. The Johns Hopkins Hospital Datamart financial database, used for mandatory reporting to the State of Maryland, provided data on LOS, age, sex, race (white, black, other), payer (Medicare, Medicaid, other), primary admission diagnosis, and comorbidity index using Agency for Healthcare Research and Quality (AHRQ) methodology.[27] Expected LOS was calculated using the risk adjustment method developed by the University Health System Consortium (UHC).[28] This calculation uses a combination of the Diagnostic‐Related Group grouper and the Sachs Complication Profiler[29] in conjunction with data on specific patient characteristics (age, sex, urgency of admission, payer category) to construct risk‐adjustment regression models that assign expected values for LOS, and is not based on actual LOS.[28] The databases were linked at the patient level using the patient's medical record and unique admission record number.
Outcome Measures
Two functional outcome measures were based on daily JH‐HLM scores, which frequently occurred several times on each patient‐day: (1) the maximum daily JH‐HLM scores for each patient‐day during hospitalization, and (2) the intrapatient change in JH‐HLM scores between the maximum JH‐HLM score within 24 hours of hospital admission and 24 hours before discharge for all patients who were on the unit >48 hours. We also compared the mean LOS during the 12‐month QI project versus the 12‐months prior so we could more accurately address seasonal differences.[30, 31, 32, 33, 34, 35] Lastly, because the perception of increased falls was an important barrier to address in the QI process, we compared the rate of injurious falls between the QI period and 12‐months prior.
Statistical Analysis
To evaluate changes in the percent of ambulatory patients (JH‐HLM 6), we compared the initial 4 months of the QI project (ramp‐up phase) with the same 4‐month period occurring immediately after project completion (post‐QI phase) using generalized estimating equations to account for clustering at the patient‐level. This test was also used to evaluate changes in documentation compliance rates between the 2 phases, with compliance defined as at least 1 instance of JH‐HLM documentation per day, excluding the day of admission and discharge. To evaluate if improved JH‐HLM results were driven by improved documentation compliance rates over time, we performed a sensitivity analysis by imputing a JH‐HLM score of 6 (ambulate 10+ steps) for any missing daily maximum JH‐HLM scores.
To assess unadjusted changes in LOS during the 12‐month QI project versus the same period 1 year earlier, we compared mean and median LOS using a t test and Wilcoxon rank sum test, respectively. We used a multivariable linear regression model to estimate the change (expressed in days) in adjusted median LOS comparing the project months (March 2013March 2014) with 12 months prior (March 2012March 2013). The model adjusted for age, gender, race, payer, admission diagnostic category, UHC expected LOS, and AHRQ comorbidity index. We confirmed a lack of multicollinearity in the multivariable regression model using variance inflation factors. We evaluated residual versus predicted value plots and residual versus fitted value plots with a locally weighted scatterplot smoothing line to confirm model fit. P values are reported from the test of the null hypothesis that the change in adjusted median LOS is the same comparing the QI project months versus 12 months prior. Separate models estimated and tested the change in adjusted median LOS by tertiles of expected LOS (<4, 47, and >7 days). Lastly, we compared the rate of injurious falls (the number of injurious falls by total patient‐days) between the QI period and 12 months prior using an exact Poisson method.[36] Statistical significance was defined as a 2‐sided P < 0.05. Statistical analyses were conducted using R (version 3.1.0; The R Foundation for Statistical Computing, Vienna, Austria;
RESULTS
During the QI project period, 3352 patients were admitted to the 2 general medicine units. Twelve (0.4%) patients expired on the units, but their data were retained in the analysis. Mean (standard deviation [SD]) age of the patients was 54.4 (18.3) years, with 47% male, and 54% African American. A total of 1896 of 6654 (28%) patients on the QI units were 65 years old. Patient characteristics were similar during the QI period versus 12 months prior (Table 1).
Characteristics | Comparison Period, March 2012March 2013, N = 3,302 | QI Period, March 2013March 2014, N = 3,352 |
---|---|---|
| ||
Age, y | 53.3 (17.8) | 54.4 (18.3) |
Male | 1467 (44%) | 1569 (47%) |
Race | ||
African American | 1883 (57%) | 1809 (54%) |
Caucasian | 1269 (38%) | 1348 (40%) |
Other | 150 (5%) | 195 (6%) |
Payer | ||
Medicare | 1310 (40%) | 1470 (44%) |
Medicaid | 1015 (31%) | 925 (28%) |
Other | 977 (30%) | 957 (29%) |
Admission diagnostic category | ||
Infectious disease | 579 (18%) | 629 (19%) |
Pulmonary | 519 (16%) | 559 (17%) |
Gastrointestinal | 535 (16%) | 494 (15%) |
Cardiovascular | 410 (12%) | 405 (12%) |
Hematologic | 199 (6%) | 195 (6%) |
Renal | 220 (7%) | 205 (6%) |
Other | 840 (25%) | 865 (26%) |
UHC expected length of stay, d | 5.5 (3.3) | 5.3 (3.2) |
AHRQ comorbidity index | 3.3 (1.7) | 3.5 (1.8) |
During the 12‐month QI project, there were a total of 13,815 patient‐days of documented mobility data and the median (interquartile range [IQR]) number of days of documentation for each hospital admission was 3 (25) days. Compliance with daily documentation of JH‐HLM was 85.0% over the entire 12‐month QI project. Documentation compliance started at 83% during the ramp‐up phase and increased to 89% during the last 4 months of the project (late‐QI phase, P < 0.001).
Comparing the ramp‐up phase versus post‐QI phase, the percentage of patient‐days in which patients ambulated (JH‐HLM 6) increased from 43% to 70% (P < 0.001), and the percentage of patients who experienced an improvement in their mobility scores between admission and discharge increased from 32% to 45% (P < 0.001), as shown in Table 2. In the sensitivity analysis imputing missing daily JH‐HLM scores and comparing the ramp‐up versus post‐QI phases, the results were similar to the primary analysis; the percent of patient‐days where patients ambulated increased from 60% to 78% (P < 0.001), and the percent of patients who experienced an improvement in their mobility scores increased from 26% to 48% (P < 0.001).
JH‐HLM Category | Ramp‐up Phase, March 1, 2013 June 30, 2013, n = 4,649 | Late‐QI Phase, November 1, 2013February 28, 2013, n = 4,515 | Post‐QI Phase, March 1, 2014 June 30, 2014, n = 4,298 |
---|---|---|---|
Change in Mobility (Admission Versus Discharge) | Ramp‐up Phase, March 1, 2013June 30, 2013, n = 968 | Late‐QI Phase, November 1, 2013February 28, 2013, n = 893 | Post‐QI Phase, March 1, 2014 June 30, 2014, n = 834 |
| |||
Walk (JH‐HLM = 6, 7, or 8) | 1,994 (43) | 3,430 (76) | 2,986 (70) |
Stand/chair (JH‐HLM = 4 or 5) | 1,772 (38) | 488 (10) | 511 (12) |
Bed (JH‐HLM = 1, 2, or 3) | 883 (19) | 597 (13) | 801 (19) |
Improved | 305 (32) | 392 (44) | 379 (45) |
No change | 512 (53) | 428 (48) | 386 (46) |
Declined | 151 (16) | 73 (8) | 69 (8) |
LOS during the 12‐month QI project versus the 12‐months immediately prior was shorter (Table 3), with an unadjusted median (IQR) LOS of 3 (26) versus 4 (27) days (P < 0.001) and an unadjusted mean (SD) LOS of 5.1 (5.6) versus 6.0 (7.6) (P < 0.001).
Adjusted Median LOS, d | Absolute Change in Adjusted Median LOS (95% CI), d | P Value | ||
---|---|---|---|---|
12 Months Prior | QI Project Months | |||
| ||||
All patients | 6.01 | 5.61 | 0.40 (0.57 to 0.21), N = 4,411 | <0.001 |
Subgroups by ELOS | ||||
ELOS <4 days | 4.68 | 4.77 | 0.09 (0.13 to 0.32), N = 1,357 | 0.42 |
ELOS 47 days | 5.68 | 5.38 | 0.30 (0.57 to 0.01), N = 1,509 | 0.04 |
ELOS >7 days | 8.07 | 6.96 | 1.11 (1.53 to 0.65), N = 1,545 | <0.001 |
Table 3 displays the change in adjusted median LOS for the project months versus the 12 months prior among the QI units. We found that for all patients, there was an overall reduction in adjusted median LOS of 0.40 (95% confidence interval [CI]: 0.57 to 0.21, P<0.001) days. When we divided patients into tertiles based on their UHC expected LOS (ELOS), we observed that patients with longer ELOS had greater reductions in adjusted median LOS. Patients on the QI units with ELOS <4 days (lowest tertile) did not show a significant reduction in adjusted median LOS (0.09 days, 95% CI: 0.13 to 0.32, P = 0.42); however, patients with UHC ELOS 4 to 7 days (middle tertile) and ELOS >7 days (highest tertile) had a significant reduction in adjusted median LOS by 0.30 (95% CI: 0.57 to 0.01, P = 0.04) and 1.11 (95% CI: 1.53 to 0.65, P < 0.001) days during the QI project versus 12 months prior, respectively.
Lastly, we found that there was no difference in the rate of injurious falls on the QI units during QI period compared to 12 months prior (QI: 0.34 per 1000 patient‐days versus 12 months prior: 0.48 per 1000 patient‐days, P = 0.73).
DISCUSSION
We conducted a nurse‐driven, multidisciplinary mobility promotion QI project on 2 general medicine units at a large teaching hospital. The 12‐month QI project, conducted between March 1, 2013 and February 28, 2014, was associated with patients ambulating more frequently, with improved mobility status between hospital admission and discharge. These improvements in mobility were not associated with increased rates of injurious falls, and were sustained for at least 4 months after project completion. The QI project was associated with overall significant reduction in LOS for more complex patients with longer expected LOS (4 days or longer). Hence, such QI efforts may be important for maintaining or improving patients' functional status during hospitalization in a safe and cost‐effective manner.
Our findings are consistent with previous studies showing that mobility promotion in the acute hospital setting is feasible, can reduce length of stay, and can be applied to a diverse population including vulnerable medical patients with multiple comorbidities and the elderly.[12, 16, 37, 38, 39, 40, 41, 42] These studies provide valuable evidence of the benefits of mobility promotion; however, it is difficult to translate these prior results into routine clinical practice because they used specially trained staff to mobilize patients, focused on a select patient population, or did not specify how the mobility intervention was delivered within daily clinical workflows. Research in the medical ICU at our institution has previously described the use of a structured QI model to successfully implement an early rehabilitation program.[22, 24] Here, we successfully adapted the same QI framework to a general medicine setting. Hence, our study contributes to the literature with respect to (1) use of a structured QI framework to develop a successful patient mobility program in a general medicine patient population, and (2) sharing best practices from 1 clinical setting, such as the ICU, as a source of learning and knowledge translation for other care settings, with the addition of novel tools, such as the JH‐HLM scale.
There may have been several factors that contributed to shorter stays in the hospital we observed during the QI project. First, we increased the number of ambulatory patient‐days, which may have helped prevent physiological complications of bed rest, such as muscle weakness, atelectasis, insulin resistance, vascular dysfunction, contractures, and pressure ulcers.[43] As such, mobility promotion has been associated with reduced rates of other hospital‐acquired complications, such as deep venous thrombosis, pneumonia, and delirium.[44, 45, 46] In our study, we saw the greatest LOS reduction in more complex patients who were expected to spend a longer time in the hospital and are at greater risk of developing complications from bed rest. Second, our early mobility project may have had a direct impact on care‐coordination processes as reported in prior studies.[47, 48, 49] An important component of our intervention was incorporating functional status into multidisciplinary discussions, either through nurse‐to‐therapist huddles or care‐coordination rounds between nurses, therapists, physicians, social workers, and case managers. During care‐coordination rounds, JH‐HLM scores were reported to expedite appropriate physical and occupational therapy consultations and assist in determining appropriate discharge location. During the QI project, we transitioned from a unit‐based daily huddle between nursing and rehabilitation therapists to a system where mobility status was discussed primarily during care coordination rounds 5 times per week. We saw that mobility scores were maintained after QI project completion, suggesting that reporting on patient function in a multidisciplinary setting is a potentially sustainable mechanism to improve care‐coordination processes that are affected by functional status.
Our study has several potential limitations. First, this is a single‐site study in 2 general medicine units of a large academic hospital. Further research is needed to determine if this structured QI intervention and its benefits can be generalized to different settings and different patient populations. Second, because the documentation was initially an optional element in the electronic medical record system, we observed higher rates of missing documentation during the first 4 months of the project versus the comparison period at 4 months after project completion. However, a sensitivity analysis conducted of these missing data demonstrated similar results to our primary analysis. Third, our nonrandomized pre‐post study design does not allow us to conclude a direct cause‐and‐effect relationship between our intervention and increased mobility and reduced LOS. Although patient characteristics were similar between the 2 periods and adjusted for in our multivariable regression analysis, we cannot rule out the possibility of secular trends in LOS on the project units and that broader QI efforts at our institution also contributed to reduction in LOS. Fourth, we do not have data on 30‐day readmissions and discharge location. Future studies should explore the impact of hospital‐based mobility interventions on these outcomes.[50] Fifth, although nurses consistently documented the highest level of mobility on a daily basis, these data did not capture other potentially important information about patient mobility such as the daily frequency that patients were mobilized, the length of time a patient was engaged in a mobility event (ie, number of hours sitting in a chair), or the mobility that occurred during physical therapy or occupational therapy sessions. Hence, although we used JH‐HLM as a marker of improved mobility during our QI project it is likely that our data cannot fully describe the total mobility and activity that patients experienced during hospitalization. Lastly, although the front‐line staff and QI team found the JH‐HLM scale to be a useful tool to measure and advance patient mobility, further studies are needed to evaluate the reliability and validity of this scale.
CONCLUSION
A structured QI process can improve patient mobility and may contribute to reduction in LOS, particularly for more complex patients in this setting. Active prevention of decline in physical function that commonly occurs during hospitalization may prove valuable for improving patient outcomes and reducing healthcare resource utilization.
Disclosures
The authors certify that no party having a direct interest in the results of the research supporting this article has or will confer a benefit on us or on any organization with which we are associated. The authors report no conflicts of interest.
- Loss of independence in activities of daily living in older adults hospitalized with medical illnesses: increased vulnerability with age. J Am Geriatr Soc. 2003;51(4):451–458. , , , et al.
- Prevalence and outcomes of low mobility in hospitalized older patients. J Am Geriatr Soc. 2004;52(8):1263–1270. , , .
- Trajectories of life‐space mobility after hospitalization. Ann Intern Med. 2009;150(6):372–378. , , , , , .
- Hospitalization‐associated disability: “She was probably able to ambulate, but I'm not sure”. JAMA. 2011;306(16):1782–1793. , , .
- Physical fitness and all‐cause mortality. A prospective study of healthy men and women. JAMA. 1989;262(17):2395–2401. , , , , , .
- Mobilizing patients in the intensive care unit: improving neuromuscular weakness and physical function. JAMA. 2008;300(14):1685–1690. .
- Mobility limitation in the older patient: a clinical review. JAMA. 2013;310(11):1168–1177. , .
- Early physical and occupational therapy in mechanically ventilated, critically ill patients: a randomised controlled trial. Lancet. 2009;373(9678):1874–1882. , , , et al.
- Technology to enhance physical rehabilitation of critically ill patients. Crit Care Med. 2009;37(10 suppl):S436–S441. , , .
- Receiving early mobility during an intensive care unit admission is a predictor of improved outcomes in acute respiratory failure. Am J Med Sci. 2011;341(5):373–377. , , , et al.
- Physiotherapy in intensive care: an updated systematic review. Chest. 2013;144(3):825–847. .
- Exercise for acutely hospitalised older medical patients. Cochrane Database Syst Rev. 2007;(1):CD005955. , , .
- Extra physical therapy reduces patient length of stay and improves functional outcomes and quality of life in people with acute or subacute conditions: a systematic review. Arch Phys Med Rehabil. 2011;92(9):1490–1500. , , .
- Impact of early mobilization protocol on the medical‐surgical inpatient population: an integrated review of literature. Clin Nurse Spec. 2012;26(2):87–94. , .
- Outcomes of inpatient mobilization: a literature review. J Clin Nurs. 2014;23(11–12):1486–1501. , , .
- The early mobility bundle: a simple enhancement of therapy which may reduce incidence of hospital‐acquired pneumonia and length of hospital stay. J Hosp Infect. 2014;88(1):34–39. , , , et al.
- Physical therapy on the wards after early physical activity and mobility in the intensive care unit. Phys Ther. 2012;92(12):1518–1523. , , , , .
- Impact of hospital variables on case mix index as a marker of disease severity. Popul Health Manag. 2014;17(1):28–34. , , , .
- Frequency of hallway ambulation by hospitalized older adults on medical units of an academic hospital. Geriatr Nurs. 2004;25(4):212–217. , , , , .
- Activity level of hospital medical inpatients: an observational study. Arch Gerontol Geriatr. 2012;55(2):417–421. , , .
- Translating evidence into practice: a model for large scale knowledge translation. BMJ. 2008;337:a1714. , , .
- Early physical medicine and rehabilitation for patients with acute respiratory failure: a quality improvement project. Arch Phys Med Rehabil. 2010;91(4):536–542. , , , et al.
- SQUIRE development group. Publication guidelines for quality improvement studies in health care: evolution of the SQUIRE project. BMJ. 2009;338:a3152. , , , , ;
- Rehabilitation quality improvement in an intensive care unit setting: implementation of a quality improvement model. Top Stroke Rehabil. 2010;17(4):271–281. , .
- ICU early mobilization: from recommendation to implementation at three medical centers. Crit Care Med. 2013;41(9 suppl 1):S69–S80. , , , .
- Barriers to early mobility of hospitalized general medicine patients: survey development and results. Am J Phys Med Rehabil. 2015;94(4):304–312. , , , .
- Comorbidity measures for use with administrative data. Med Care. 1998;36(1):8–27. , , , .
- UHC Clinical Information Management Risk Adjustment of the UHC Clinical Data Base. Chicago, IL: University HealthSystem Consortium; 1998.
- Sachs Complications Profiler, Version 1.0, User's Guide. Evanston, IL: Sachs Group; 1995.
- Assessing hospital‐associated deaths from discharge data. The role of length of stay and comorbidities. JAMA. 1988;260(15):2240–2246. , , .
- Annual rates of admission and seasonal variations in hospitalizations for heart failure. Eur J Heart Fail. 2002;4(6):779–786. , , , et al.
- Regional and seasonal variation in the length of hospital stay for chronic obstructive pulmonary disease in Finland. Int J Circumpolar Health. 2002;61(2):131–135. , , , .
- Canadian CABG Surgery Quality Indicator Consensus Panel. The identification and development of canadian coronary artery bypass graft surgery quality indicators. J Thorac Cardiovasc Surg. 2005;130(5):1257. , , , , , ;
- Quality of care and length of hospital stay among patients with stroke. Med Care. 2009;47(5):575–582. , , , .
- A systematic review of outcomes and quality measures in adult patients cared for by hospitalists vs nonhospitalists. Mayo Clin Proc. 2009;84(3):248–254. .
- Confidence intervals that match Fisher's exact or Blaker's exact tests. Biostatistics. 2010;11(2):373–374. .
- A multicomponent intervention to prevent delirium in hospitalized older patients. N Engl J Med. 1999;340(9):669–676. , , , et al.
- Early mobilization of patients hospitalized with community‐acquired pneumonia. Chest. 2003;124(3):883–889. , , , , .
- Effects of a walking intervention on fatigue‐related experiences of hospitalized acute myelogenous leukemia patients undergoing chemotherapy: a randomized controlled trial. J Pain Symptom Manage. 2008;35(5):524–534. , , , et al.
- Early ambulation and length of stay in older adults hospitalized for acute illness. Arch Intern Med. 2010;170(21):1942–1943. , , , , .
- Impact of a nurse‐driven mobility protocol on functional decline in hospitalized older adults. J Nurs Care Qual. 2009;24(4):325–331. , , .
- Exercising body and mind: an integrated approach to functional independence in hospitalized older people. J Am Geriatr Soc. 2008;56(4):630–635. , , .
- Consequences of bed rest. Crit Care Med. 2009;37(10 suppl):S422–S428. .
- Time to ambulation after hip fracture surgery: relation to hospitalization outcomes. J Gerontol A Biol Sci Med Sci. 2003;58(11):M1042–M1045. , , , , .
- Early mobilization after total knee replacement reduces the incidence of deep venous thrombosis. ANZ J Surg. 2009;79(7–8):526–529. , , , .
- Efficacy and safety of postoperative early mobilization for chronic subdural hematoma in elderly patients. Acta Neurochir (Wien). 2010;152(7):1171–1174. , , , .
- Impact of relational coordination on quality of care, postoperative pain and functioning, and length of stay: a nine‐hospital study of surgical patients. Med Care. 2000;38(8):807–819. , , , et al.
- Care coordination cuts admissions, ED visits, LOS. Hosp Case Manag. 2013;21(5):67–68.
- A heart failure initiative to reduce the length of stay and readmission rates. Prof Case Manag. 2014;19(6):276–284. , .
- Association of impaired functional status at hospital discharge and subsequent rehospitalization. J Hosp Med. 2014;9(5):277–282. , , , , , .
- Loss of independence in activities of daily living in older adults hospitalized with medical illnesses: increased vulnerability with age. J Am Geriatr Soc. 2003;51(4):451–458. , , , et al.
- Prevalence and outcomes of low mobility in hospitalized older patients. J Am Geriatr Soc. 2004;52(8):1263–1270. , , .
- Trajectories of life‐space mobility after hospitalization. Ann Intern Med. 2009;150(6):372–378. , , , , , .
- Hospitalization‐associated disability: “She was probably able to ambulate, but I'm not sure”. JAMA. 2011;306(16):1782–1793. , , .
- Physical fitness and all‐cause mortality. A prospective study of healthy men and women. JAMA. 1989;262(17):2395–2401. , , , , , .
- Mobilizing patients in the intensive care unit: improving neuromuscular weakness and physical function. JAMA. 2008;300(14):1685–1690. .
- Mobility limitation in the older patient: a clinical review. JAMA. 2013;310(11):1168–1177. , .
- Early physical and occupational therapy in mechanically ventilated, critically ill patients: a randomised controlled trial. Lancet. 2009;373(9678):1874–1882. , , , et al.
- Technology to enhance physical rehabilitation of critically ill patients. Crit Care Med. 2009;37(10 suppl):S436–S441. , , .
- Receiving early mobility during an intensive care unit admission is a predictor of improved outcomes in acute respiratory failure. Am J Med Sci. 2011;341(5):373–377. , , , et al.
- Physiotherapy in intensive care: an updated systematic review. Chest. 2013;144(3):825–847. .
- Exercise for acutely hospitalised older medical patients. Cochrane Database Syst Rev. 2007;(1):CD005955. , , .
- Extra physical therapy reduces patient length of stay and improves functional outcomes and quality of life in people with acute or subacute conditions: a systematic review. Arch Phys Med Rehabil. 2011;92(9):1490–1500. , , .
- Impact of early mobilization protocol on the medical‐surgical inpatient population: an integrated review of literature. Clin Nurse Spec. 2012;26(2):87–94. , .
- Outcomes of inpatient mobilization: a literature review. J Clin Nurs. 2014;23(11–12):1486–1501. , , .
- The early mobility bundle: a simple enhancement of therapy which may reduce incidence of hospital‐acquired pneumonia and length of hospital stay. J Hosp Infect. 2014;88(1):34–39. , , , et al.
- Physical therapy on the wards after early physical activity and mobility in the intensive care unit. Phys Ther. 2012;92(12):1518–1523. , , , , .
- Impact of hospital variables on case mix index as a marker of disease severity. Popul Health Manag. 2014;17(1):28–34. , , , .
- Frequency of hallway ambulation by hospitalized older adults on medical units of an academic hospital. Geriatr Nurs. 2004;25(4):212–217. , , , , .
- Activity level of hospital medical inpatients: an observational study. Arch Gerontol Geriatr. 2012;55(2):417–421. , , .
- Translating evidence into practice: a model for large scale knowledge translation. BMJ. 2008;337:a1714. , , .
- Early physical medicine and rehabilitation for patients with acute respiratory failure: a quality improvement project. Arch Phys Med Rehabil. 2010;91(4):536–542. , , , et al.
- SQUIRE development group. Publication guidelines for quality improvement studies in health care: evolution of the SQUIRE project. BMJ. 2009;338:a3152. , , , , ;
- Rehabilitation quality improvement in an intensive care unit setting: implementation of a quality improvement model. Top Stroke Rehabil. 2010;17(4):271–281. , .
- ICU early mobilization: from recommendation to implementation at three medical centers. Crit Care Med. 2013;41(9 suppl 1):S69–S80. , , , .
- Barriers to early mobility of hospitalized general medicine patients: survey development and results. Am J Phys Med Rehabil. 2015;94(4):304–312. , , , .
- Comorbidity measures for use with administrative data. Med Care. 1998;36(1):8–27. , , , .
- UHC Clinical Information Management Risk Adjustment of the UHC Clinical Data Base. Chicago, IL: University HealthSystem Consortium; 1998.
- Sachs Complications Profiler, Version 1.0, User's Guide. Evanston, IL: Sachs Group; 1995.
- Assessing hospital‐associated deaths from discharge data. The role of length of stay and comorbidities. JAMA. 1988;260(15):2240–2246. , , .
- Annual rates of admission and seasonal variations in hospitalizations for heart failure. Eur J Heart Fail. 2002;4(6):779–786. , , , et al.
- Regional and seasonal variation in the length of hospital stay for chronic obstructive pulmonary disease in Finland. Int J Circumpolar Health. 2002;61(2):131–135. , , , .
- Canadian CABG Surgery Quality Indicator Consensus Panel. The identification and development of canadian coronary artery bypass graft surgery quality indicators. J Thorac Cardiovasc Surg. 2005;130(5):1257. , , , , , ;
- Quality of care and length of hospital stay among patients with stroke. Med Care. 2009;47(5):575–582. , , , .
- A systematic review of outcomes and quality measures in adult patients cared for by hospitalists vs nonhospitalists. Mayo Clin Proc. 2009;84(3):248–254. .
- Confidence intervals that match Fisher's exact or Blaker's exact tests. Biostatistics. 2010;11(2):373–374. .
- A multicomponent intervention to prevent delirium in hospitalized older patients. N Engl J Med. 1999;340(9):669–676. , , , et al.
- Early mobilization of patients hospitalized with community‐acquired pneumonia. Chest. 2003;124(3):883–889. , , , , .
- Effects of a walking intervention on fatigue‐related experiences of hospitalized acute myelogenous leukemia patients undergoing chemotherapy: a randomized controlled trial. J Pain Symptom Manage. 2008;35(5):524–534. , , , et al.
- Early ambulation and length of stay in older adults hospitalized for acute illness. Arch Intern Med. 2010;170(21):1942–1943. , , , , .
- Impact of a nurse‐driven mobility protocol on functional decline in hospitalized older adults. J Nurs Care Qual. 2009;24(4):325–331. , , .
- Exercising body and mind: an integrated approach to functional independence in hospitalized older people. J Am Geriatr Soc. 2008;56(4):630–635. , , .
- Consequences of bed rest. Crit Care Med. 2009;37(10 suppl):S422–S428. .
- Time to ambulation after hip fracture surgery: relation to hospitalization outcomes. J Gerontol A Biol Sci Med Sci. 2003;58(11):M1042–M1045. , , , , .
- Early mobilization after total knee replacement reduces the incidence of deep venous thrombosis. ANZ J Surg. 2009;79(7–8):526–529. , , , .
- Efficacy and safety of postoperative early mobilization for chronic subdural hematoma in elderly patients. Acta Neurochir (Wien). 2010;152(7):1171–1174. , , , .
- Impact of relational coordination on quality of care, postoperative pain and functioning, and length of stay: a nine‐hospital study of surgical patients. Med Care. 2000;38(8):807–819. , , , et al.
- Care coordination cuts admissions, ED visits, LOS. Hosp Case Manag. 2013;21(5):67–68.
- A heart failure initiative to reduce the length of stay and readmission rates. Prof Case Manag. 2014;19(6):276–284. , .
- Association of impaired functional status at hospital discharge and subsequent rehospitalization. J Hosp Med. 2014;9(5):277–282. , , , , , .
© 2016 Society of Hospital Medicine
Letter to the Editor
We thank Mr. Zilm and colleagues for their interest in our work.[1] Certainly, we did not intend to imply that well‐designed buildings have little value in the efficient and patient‐centered delivery of healthcare. Our main goal was to highlight (1) that patients can distinguish between facility features and actual care delivery, and poor facilities alone should not be an excuse for poor patient satisfaction; and (2) that global evaluations are more dependent on perceived quality of care than on facility features. Furthermore, we agree with many of the points raised. Certainly, patient satisfaction is but 1 measure of successful facility design, and the delivery of modern healthcare requires updated facilities. However, based on our results, we think that healthcare administrators and designers should consider the return on investment on the costly features that are incorporated purely to improve patient satisfaction rather than for safety and staff effectiveness.
Referral patterns and patient expectations are likely very different for a tertiary care hospital like ours. A different relationship between facility design and patient satisfaction may indeed exist for community hospitals. However, we would caution against making this assumption without supportive evidence. Furthermore, it is difficult to attribute lack of improvement of physician scores in our study because of a ceiling effect. The baseline scores were certainly not exemplary, and there was plenty of room for improvement.
We agree that there is a need for high‐quality research to better understand the broader impact of healthcare design on meaningful outcomes. However, we are not impressed with the quality of much of the existing research tying physical facilities with patient stress or shorter length of stay, as mentioned by Mr. Zilm and colleagues. Evidence supporting investment in expensive facilities should be evaluated with the same high standards and rigor as for other healthcare decisions.
- Changes in patient satisfaction related to hospital renovation: experience with a new clinical building. J Hosp Med. 2015;10(3):165–171. , , , , .
We thank Mr. Zilm and colleagues for their interest in our work.[1] Certainly, we did not intend to imply that well‐designed buildings have little value in the efficient and patient‐centered delivery of healthcare. Our main goal was to highlight (1) that patients can distinguish between facility features and actual care delivery, and poor facilities alone should not be an excuse for poor patient satisfaction; and (2) that global evaluations are more dependent on perceived quality of care than on facility features. Furthermore, we agree with many of the points raised. Certainly, patient satisfaction is but 1 measure of successful facility design, and the delivery of modern healthcare requires updated facilities. However, based on our results, we think that healthcare administrators and designers should consider the return on investment on the costly features that are incorporated purely to improve patient satisfaction rather than for safety and staff effectiveness.
Referral patterns and patient expectations are likely very different for a tertiary care hospital like ours. A different relationship between facility design and patient satisfaction may indeed exist for community hospitals. However, we would caution against making this assumption without supportive evidence. Furthermore, it is difficult to attribute lack of improvement of physician scores in our study because of a ceiling effect. The baseline scores were certainly not exemplary, and there was plenty of room for improvement.
We agree that there is a need for high‐quality research to better understand the broader impact of healthcare design on meaningful outcomes. However, we are not impressed with the quality of much of the existing research tying physical facilities with patient stress or shorter length of stay, as mentioned by Mr. Zilm and colleagues. Evidence supporting investment in expensive facilities should be evaluated with the same high standards and rigor as for other healthcare decisions.
We thank Mr. Zilm and colleagues for their interest in our work.[1] Certainly, we did not intend to imply that well‐designed buildings have little value in the efficient and patient‐centered delivery of healthcare. Our main goal was to highlight (1) that patients can distinguish between facility features and actual care delivery, and poor facilities alone should not be an excuse for poor patient satisfaction; and (2) that global evaluations are more dependent on perceived quality of care than on facility features. Furthermore, we agree with many of the points raised. Certainly, patient satisfaction is but 1 measure of successful facility design, and the delivery of modern healthcare requires updated facilities. However, based on our results, we think that healthcare administrators and designers should consider the return on investment on the costly features that are incorporated purely to improve patient satisfaction rather than for safety and staff effectiveness.
Referral patterns and patient expectations are likely very different for a tertiary care hospital like ours. A different relationship between facility design and patient satisfaction may indeed exist for community hospitals. However, we would caution against making this assumption without supportive evidence. Furthermore, it is difficult to attribute lack of improvement of physician scores in our study because of a ceiling effect. The baseline scores were certainly not exemplary, and there was plenty of room for improvement.
We agree that there is a need for high‐quality research to better understand the broader impact of healthcare design on meaningful outcomes. However, we are not impressed with the quality of much of the existing research tying physical facilities with patient stress or shorter length of stay, as mentioned by Mr. Zilm and colleagues. Evidence supporting investment in expensive facilities should be evaluated with the same high standards and rigor as for other healthcare decisions.
- Changes in patient satisfaction related to hospital renovation: experience with a new clinical building. J Hosp Med. 2015;10(3):165–171. , , , , .
- Changes in patient satisfaction related to hospital renovation: experience with a new clinical building. J Hosp Med. 2015;10(3):165–171. , , , , .
In reply: Resuming anticoagulation after hemorrhage
In Reply: We thank Dr. Jandali for his thoughtful comments on our article. We acknowledge that there may be a small subset of patients in whom low-intensity warfarin may be worth trying—such as patients with a history of idiopathic or recurrent venous thromboembolism in whom problematic (but not life-threatening) bleeding recurs—but only when the international normalized ratio (INR) is at the high end of the therapeutic range or slightly above it. However, when attempting to apply the results from PREVENT1 and ELATE2 to clinical practice and the management of anticoagulation after hemorrhage, it is important to note that in ELATE there was a higher incidence of recurrent thromboembolism in patients on lower-intensity anticoagulation than in those on conventional treatment, and no significant difference in major bleeding was noted between the high- and low-intensity groups.
We acknowledge, though, that the rates of major bleeding were surprisingly low in the high-intensity group in this study relative to historical controls and so may not apply to all patients.
It is also important to recognize that several studies have evaluated low-intensity dosing for stroke prophylaxis in atrial fibrillation with generally disappointing results, and at present, expert opinion continues to support a therapeutic INR goal of 2.0 to 3.0.3
Therefore, we believe that low-intensity warfarin treatment is only appropriate to try in a very small subset of carefully selected patients with a history of venous thromboembolism who have proven that they cannot tolerate full-dose warfarin and in whom a trial of low-dose warfarin treatment carries acceptable risk.
- Ridker PM, Goldhaber SZ, Danielson E, et al; PREVENT Investigators. Long-term, low-intensity warfarin therapy for the prevention of recurrent venous thromboembolism. N Engl J Med 2003; 348:1425–1434.
- Kearon C, Ginsberg JS, Kovacs MJ, et al; Extended Low-Intensity Anticoagulation for Thrombo-Embolism Investigators. Comparison of low-intensity warfarin therapy with conventional-intensity warfarin therapy for long-term prevention of recurrent venous thromboembolism. N Engl J Med 2003; 349:631–639.
- Holbrook A, Schulman S, Witt DM, et al; American College of Chest Physicians. Evidence-based management of anticoagulant therapy: Antithrombotic Therapy and Prevention of Thrombosis, 9th ed: American College of Chest Physicians Evidence-Based Clinical Practice Guidelines. Chest 2012; 141(suppl 2):e152S–e184S.
In Reply: We thank Dr. Jandali for his thoughtful comments on our article. We acknowledge that there may be a small subset of patients in whom low-intensity warfarin may be worth trying—such as patients with a history of idiopathic or recurrent venous thromboembolism in whom problematic (but not life-threatening) bleeding recurs—but only when the international normalized ratio (INR) is at the high end of the therapeutic range or slightly above it. However, when attempting to apply the results from PREVENT1 and ELATE2 to clinical practice and the management of anticoagulation after hemorrhage, it is important to note that in ELATE there was a higher incidence of recurrent thromboembolism in patients on lower-intensity anticoagulation than in those on conventional treatment, and no significant difference in major bleeding was noted between the high- and low-intensity groups.
We acknowledge, though, that the rates of major bleeding were surprisingly low in the high-intensity group in this study relative to historical controls and so may not apply to all patients.
It is also important to recognize that several studies have evaluated low-intensity dosing for stroke prophylaxis in atrial fibrillation with generally disappointing results, and at present, expert opinion continues to support a therapeutic INR goal of 2.0 to 3.0.3
Therefore, we believe that low-intensity warfarin treatment is only appropriate to try in a very small subset of carefully selected patients with a history of venous thromboembolism who have proven that they cannot tolerate full-dose warfarin and in whom a trial of low-dose warfarin treatment carries acceptable risk.
In Reply: We thank Dr. Jandali for his thoughtful comments on our article. We acknowledge that there may be a small subset of patients in whom low-intensity warfarin may be worth trying—such as patients with a history of idiopathic or recurrent venous thromboembolism in whom problematic (but not life-threatening) bleeding recurs—but only when the international normalized ratio (INR) is at the high end of the therapeutic range or slightly above it. However, when attempting to apply the results from PREVENT1 and ELATE2 to clinical practice and the management of anticoagulation after hemorrhage, it is important to note that in ELATE there was a higher incidence of recurrent thromboembolism in patients on lower-intensity anticoagulation than in those on conventional treatment, and no significant difference in major bleeding was noted between the high- and low-intensity groups.
We acknowledge, though, that the rates of major bleeding were surprisingly low in the high-intensity group in this study relative to historical controls and so may not apply to all patients.
It is also important to recognize that several studies have evaluated low-intensity dosing for stroke prophylaxis in atrial fibrillation with generally disappointing results, and at present, expert opinion continues to support a therapeutic INR goal of 2.0 to 3.0.3
Therefore, we believe that low-intensity warfarin treatment is only appropriate to try in a very small subset of carefully selected patients with a history of venous thromboembolism who have proven that they cannot tolerate full-dose warfarin and in whom a trial of low-dose warfarin treatment carries acceptable risk.
- Ridker PM, Goldhaber SZ, Danielson E, et al; PREVENT Investigators. Long-term, low-intensity warfarin therapy for the prevention of recurrent venous thromboembolism. N Engl J Med 2003; 348:1425–1434.
- Kearon C, Ginsberg JS, Kovacs MJ, et al; Extended Low-Intensity Anticoagulation for Thrombo-Embolism Investigators. Comparison of low-intensity warfarin therapy with conventional-intensity warfarin therapy for long-term prevention of recurrent venous thromboembolism. N Engl J Med 2003; 349:631–639.
- Holbrook A, Schulman S, Witt DM, et al; American College of Chest Physicians. Evidence-based management of anticoagulant therapy: Antithrombotic Therapy and Prevention of Thrombosis, 9th ed: American College of Chest Physicians Evidence-Based Clinical Practice Guidelines. Chest 2012; 141(suppl 2):e152S–e184S.
- Ridker PM, Goldhaber SZ, Danielson E, et al; PREVENT Investigators. Long-term, low-intensity warfarin therapy for the prevention of recurrent venous thromboembolism. N Engl J Med 2003; 348:1425–1434.
- Kearon C, Ginsberg JS, Kovacs MJ, et al; Extended Low-Intensity Anticoagulation for Thrombo-Embolism Investigators. Comparison of low-intensity warfarin therapy with conventional-intensity warfarin therapy for long-term prevention of recurrent venous thromboembolism. N Engl J Med 2003; 349:631–639.
- Holbrook A, Schulman S, Witt DM, et al; American College of Chest Physicians. Evidence-based management of anticoagulant therapy: Antithrombotic Therapy and Prevention of Thrombosis, 9th ed: American College of Chest Physicians Evidence-Based Clinical Practice Guidelines. Chest 2012; 141(suppl 2):e152S–e184S.
Resuming anticoagulation after hemorrhage: A practical approach
If a patient receiving anticoagulant therapy suffers a bleeding event, the patient and physician must decide whether and how soon to restart the therapy, and with what agent.
Foremost on our minds tends to be the risk of another hemorrhage. Subtler to appreciate immediately after an event is the continued risk of thrombosis, often from the same medical condition that prompted anticoagulation therapy in the first place (Table 1).
Complicating the decision, there may be a rebound effect: some thrombotic events such as pulmonary embolism and atrial fibrillation-related stroke may be more likely to occur in the first weeks after stopping warfarin than during similar intervals in patients who have not been taking it.1–3 The same thing may happen with the newer, target-specific oral anticoagulants.4–6
Although we have evidence-based guidelines for initiating and managing anticoagulant therapy, ample data on adverse events, and protocols for reversing anticoagulation if bleeding occurs, we do not have clear guidelines on restarting anticoagulation after a hemorrhagic event.
In this article, we outline a practical framework for approaching this clinical dilemma. Used in conjunction with consideration of a patient’s values and preferences as well as input from experts, this framework can help clinicians guide their patients through this challenging clinical decision. It consists of five questions:
- Why is the patient on anticoagulation, and what is the risk of thromboembolism without it?
- What was the clinical impact of the hemorrhage, and what is the risk of rebleeding if anticoagulation is resumed?
- What additional patient factors should be taken into consideration?
- How long should we wait before restarting anticoagulation?
- Would a newer drug be a better choice?
BLEEDING OCCURS IN 2% TO 3% OF PATIENTS PER YEAR
Most of our information on anticoagulation is about vitamin K antagonists—principally warfarin, in use since the 1950s. Among patients taking warfarin outside of clinical trials, the risk of major bleeding is estimated at 2% to 3% per year.7
However, the target-specific oral anticoagulants rivaroxaban (Xarelto), apixaban (Eliquis), dabigatran (Pradaxa) and edoxaban (Savaysa) are being used more and more, and we include them in our discussion insofar as we have information on them. The rates of bleeding with these new drugs in clinical trials have been comparable to or lower than those with warfarin.8 Postmarketing surveillance is under way.
WHY IS THE PATIENT ON ANTICOAGULATION? WHAT IS THE RISK WITHOUT IT?
Common, evidence-based indications for anticoagulation are to prevent complications in patients with venous thromboembolism and to prevent stroke in patients with atrial fibrillation or a mechanical heart valve. Other uses, such as in heart failure and its sequelae, pulmonary hypertension, and splanchnic or hepatic vein thrombosis, have less robust evidence to support them.
When anticoagulation-related bleeding occurs, it is essential to review why the patient is taking the drug and the risk of thromboembolism without it. Some indications pose a higher risk of thromboembolism than others and so argue more strongly for continuing the treatment.
Douketis et al9 developed a risk-stratification scheme for perioperative thromboembolism. We have modified it by adding the CHA2DS2-VASc score (Table 2),9–11 and believe it can be used more widely.
High-risk indications
Conditions that pose a high risk of thrombosis almost always require restarting anticoagulation. Here, the most appropriate question nearly always is not if anticoagulation should be restarted, but when. Examples:
- A mechanical mitral valve
- Antiphospholipid antibody syndrome with recurrent thromboembolic events.
Lower-risk indications
Lower-risk indications allow more leeway in determining if anticoagulation should be resumed. The most straightforward cases fall well within established guidelines. Examples:
- Atrial fibrillation and a CHA2DS2-VASc score of 1. The 2014 guidelines from the American College of Cardiology, American Heart Association, and Heart Rhythm Society10 suggest that patients with nonvalvular atrial fibrillation and a CHA2DS2-VASc score of 1 have three options: an oral anticoagulant, aspirin, and no antithrombotic therapy. If such a patient on anticoagulant therapy subsequently experiences a major gastrointestinal hemorrhage requiring transfusion and intensive care and no definitively treatable source of bleeding is found on endoscopy, one can argue that the risks of continued anticoagulation (recurrent bleeding) now exceed the benefits and that the patient would be better served by aspirin or even no antithrombotic therapy.
- After 6 months of anticoagulation for unprovoked deep vein thrombosis. Several studies showed that aspirin reduced the risk of recurrent venous thromboembolism in patients who completed an initial 6-month course of anticoagulation.12–15 Though these studies did not specifically compare aspirin with warfarin or target-specific oral anticoagulants in preventing recurrent venous thromboembolism after a hemorrhage, it is reasonable to extrapolate their results to this situation.
If the risk of recurrent hemorrhage on anticoagulation is considered to be too great, then aspirin is an alternative to no anticoagulation, as it reduces the risk of recurrent venous thromboembolism.16 However, we advise caution if the bleeding lesion may be specifically exacerbated by aspirin, particularly upper gastrointestinal ulcers.
Moderate-risk indications
- After a partial course of anticoagulation for provoked venous thromboembolism. Suppose a patient in the 10th week of a planned 12-week course of anticoagulation for a surgically provoked, first deep vein thrombosis presents with abdominal pain and is found to have a retroperitoneal hematoma. In light of the risk of recurrent bleeding vs the benefit of resuming anticoagulation for the limited remaining period, her 12-week treatment course can reasonably be shortened to 10 weeks.
The risk of recurrent venous thromboembolism when a patient is off anticoagulation decreases with time from the initial event. The highest risk, estimated at 0.3% to 1.3% per day, is in the first 4 weeks, falling to 0.03% to 0.2% per day in weeks 5 through 12, and 0.05% per day thereafter.17–20
Additionally, a pooled analysis of seven randomized trials suggests that patients with isolated, distal deep vein thrombosis provoked by a temporary risk factor did not have a high risk of recurrence after being treated for 4 to 6 weeks.21 These analyses are based on vitamin K antagonists, though it seems reasonable to extrapolate this information to the target-specific oral anticoagulants.
More challenging are situations in which the evidence supporting the initial or continued need for anticoagulation is less robust, such as in heart failure, pulmonary hypertension, or splanchnic and hepatic vein thrombosis. In these cases, the lack of strong evidence supporting the use of anticoagulation should make us hesitate to resume it after bleeding.
WHAT WAS THE CLINICAL IMPACT? WHAT IS THE RISK OF REBLEEDING?
Different groups have defined major and minor bleeding in different ways.22,23 Several have proposed criteria to standardize how bleeding events (on warfarin and otherwise) are classified,23–25 but the definitions differ.
Specifically, all agree that a “major” bleeding event is one that is fatal, involves bleeding into a major organ, or leads to a substantial decline in hemoglobin level. However, the Thrombolysis in Myocardial Infarction trials use a decline of more than 5 g/dL in their definition,23,25 while the International Society on Thrombosis and Haemostasis uses 2 g/dL.24
Here, we review the clinical impact of the most common sources of anticoagulation-related hemorrhage—gastrointestinal, soft tissue, and urinary tract26—as well as intracerebral hemorrhage, a less common but more uniformly devastating event.27
Clinical impact of gastrointestinal hemorrhage
Each year, about 4.5% of patients taking warfarin have a gastrointestinal hemorrhage, though not all of these events are major.28 Evolving data suggest that the newer agents (particularly dabigatran, rivaroxaban, and edoxaban) pose a higher risk of gastrointestinal bleeding than warfarin.29 Patients may need plasma and blood transfusions and intravenous phytonadione, all of which carry risks, albeit small.
Frequently, endoscopy is needed to find the source of bleeding and to control it. If this does not work, angiographic intervention to infuse vasoconstrictors or embolic coils into the culprit artery may be required, and some patients need surgery. Each intervention carries its own risk.
Clinical impact of soft-tissue hemorrhage
Soft-tissue hemorrhage accounts for more than 20% of warfarin-related bleeding events26; as yet, we know of no data on the rate with the new drugs. Soft-tissue hemorrhage is often localized to the large muscles of the retroperitoneum and legs. Though retroperitoneal hemorrhage accounts for a relatively small portion of soft-tissue hemorrhages, it is associated with high rates of morbidity and death and will therefore be our focus.26
Much of the clinical impact of retroperitoneal hemorrhage is from a mass effect that causes abdominal compartment syndrome, hydroureter, ileus, abscess formation, and acute and chronic pain. At least 20% of cases are associated with femoral neuropathy. It can also lead to deep vein thrombosis from venous compression, coupled with hypercoagulability in response to bleeding. Brisk bleeding can lead to shock and death, and the mortality rate in retroperitoneal hemorrhage is estimated at 20% or higher.30
In many cases, the retroperitoneal hemorrhage will self-tamponade and the blood will be reabsorbed once the bleeding has stopped, but uncontrolled bleeding may require surgical or angiographic intervention.30
Clinical impact of urinary tract hemorrhage
Gross or microscopic hematuria can be found in an estimated 2% to 24% of patients taking warfarin31–33; data are lacking for the target-specific oral anticoagulants. Interventions required to manage urinary tract bleeding include bladder irrigation and, less often, transfusion.31 Since a significant number of cases of hematuria are due to neoplastic disease,32 a diagnostic workup with radiographic imaging of the upper tract and cystoscopy of the lower tract is usually required.31 While life-threatening hemorrhage is uncommon, complications such as transient urinary obstruction from clots may occur.
Clinical impact of intracranial hemorrhage
Intracranial hemorrhage is the most feared and deadly of the bleeding complications of anticoagulation. The incidence in patients on warfarin is estimated at 2% to 3% per year, which is markedly higher than the estimated incidence of 25 per 100,000 person-years in the general population.34 Emerging data indicate that the newer drugs are also associated with a risk of intracranial hemorrhage, though the risk is about half that with vitamin K antagonists.35 Intracranial hemorrhage leads to death or disability in 76% of cases, compared with 3% of cases of bleeding from the gastrointestinal or urinary tract.27
Regardless of the source of bleeding, hospitalization is likely to be required and may be prolonged, with attendant risks of nosocomial harms such as infection.
Risk of rebleeding
Given the scope and severity of anticoagulation-related bleeding, there is strong interest in predicting and preventing it. By some estimates, the incidence of recurrent bleeding after resuming vitamin K antagonists is 8% to 13%.22 Although there are several indices for predicting the risk of major bleeding when starting anticoagulation, there are currently no validated tools to estimate a patient’s risk of rebleeding.36
The patient factor that most consistently predicts major bleeding is a history of bleeding, particularly from the gastrointestinal tract. Finding and controlling the source of bleeding is important.26,37 For example, a patient with gross hematuria who is found on cystoscopy to have a urothelial papilloma is unlikely to have rebleeding if the tumor is successfully resected and serial follow-up shows no regrowth. In contrast, consider a patient with a major gastrointestinal hemorrhage, the source of which remains elusive after upper, lower, and capsule endoscopy or, alternatively, is suspected to be from one of multiple angiodysplastic lesions. Without definitive source management, this patient faces a high risk of rebleeding.
With or without anticoagulation, after a first intracranial hemorrhage the risk of another one is estimated at 2% to 4% per year.34 An observational study found a recurrence rate of 7.5% when vitamin K antagonist therapy was started after an intracranial hemorrhage (though not all patients were on a vitamin K antagonist at the time of the first hemorrhage).38
Patients with lobar hemorrhage and those with suspected cerebral amyloid angiopathy may be at particularly high risk if anticoagulation is resumed. Conversely, initial events attributed to uncontrolled hypertension that subsequently can be well controlled may portend a lower risk of rebleeding.34 For other types of intracranial hemorrhage, recurrence rates can be even higher. Irrespective of anticoagulation, one prospective study estimated the crude annual rebleeding rate with untreated arteriovenous malformations to be 7%.39 In chronic subdural hematoma, the recurrence rate after initial drainage has been estimated at 9.2% to 26.5%, with use of anticoagulants (in this case, vitamin K antagonists) being an independent predictor of recurrence.40
WHAT OTHER PATIENT FACTORS NEED CONSIDERATION?
Target INR on warfarin
An important factor influencing the risk of bleeding with warfarin is the intensity of this therapy.37 A meta-analysis41 found that the risks of major hemorrhage and thromboembolism are minimized if the goal international normalized ratio (INR) is 2.0 to 3.0. When considering resuming anticoagulation after bleeding, make sure the therapeutic target is appropriate.37
Table 3 summarizes recommended therapeutic ranges for frequently encountered indications for warfarin.36,42,43
INR at time of the event and challenges in controlling it
The decision to resume anticoagulation in patients who bled while using warfarin must take into account the actual INR at the time of the event.
For example, consider a patient whose INR values are consistently in the therapeutic range. While on vacation, he receives ciprofloxacin for acute prostatitis from an urgent care team, and no adjustment to INR monitoring or warfarin dose is made. Several days later, he presents with lower gastrointestinal bleeding. His INR is 8, and colonoscopy reveals diverticulosis with a bleeding vessel, responsive to endoscopic therapy. After controlling the source of bleeding and reinforcing the need to always review new medications for potential interactions with anticoagulation, it is reasonable to expect that he once again will be able to keep his INR in the therapeutic range.
A patient on anticoagulation for the same indication but who has a history of repeated supratherapeutic levels, poor adherence, or poor access to INR monitoring poses very different concerns about resuming anticoagulation (as well as which agent to use, as we discuss below).
Of note, a high INR alone does not explain bleeding. It is estimated that a workup for gastrointestinal bleeding and gross hematuria uncovers previously undetected lesions in approximately one-third of cases involving warfarin.26 A similar malignancy-unmasking effect is now recognized in patients using the target-specific oral agents who experience gastrointestinal bleeding.44 Accordingly, we recommend a comprehensive source evaluation for any anticoagulation-related hemorrhage.
Comorbid conditions
Comorbid conditions associated with bleeding include cancer, end-stage renal disease, liver disease, arterial hypertension, prior stroke, and alcohol abuse.37,45 Gait instability, regardless of cause, may also increase the risk of trauma-related hemorrhage, but some have estimated that a patient would need to fall multiple times per week to contraindicate anticoagulation on the basis of falls alone.46
Concurrent medications
Concomitant therapies, including antiplatelet drugs and nonsteroidal anti-inflammatory drugs, increase bleeding risk.47,48 Aspirin and the nonsteroidals, in addition to having antiplatelet effects, also can cause gastric erosion.37 In evaluating whether and when to restart anticoagulation, it is advisable to review the role that concomitant therapies may have had in the index bleeding event and to evaluate the risks and benefits of these other agents.
Additionally, warfarin has many interactions. Although the newer drugs are lauded for having fewer interactions, they are not completely free of them, and the potential for interactions must always be reviewed.49 Further, unlike warfarin therapy, therapy with the newer agents is not routinely monitored with laboratory tests, so toxicity (or underdosing) may not be recognized until an adverse clinical event occurs. Ultimately, it may be safer to resume anticoagulation after a contributing drug can be safely discontinued.
Advanced age
The influence that the patient’s age should have on the decision to restart anticoagulation is unclear. Although the risk of intracranial hemorrhage increases with age, particularly after age 80, limited data exist in this population, particularly with regard to rebleeding. Further, age is a major risk factor for most thrombotic events, including venous thromboembolism and stroke from atrial fibrillation, so although the risks of anticoagulation may be higher, the benefits may also be higher than in younger patients.37,46 We discourage using age alone as a reason to withhold anticoagulation after a hemorrhage.
HOW LONG SHOULD WE WAIT TO RESTART ANTICOAGULATION?
We lack conclusive data on how long to wait to restart anticoagulation after an anticoagulation-associated hemorrhage.
The decision is complicated by evidence suggesting a rebound effect, with an increased risk of pulmonary embolism and atrial fibrillation-related stroke during the first 90 days of interruption of therapy with warfarin as well as with target-specific oral anticoagulants.3–8 In anticoagulation-associated retroperitoneal bleeding, there is increased risk of deep vein thrombosis from compression, even if venous thromboembolism was not the initial indication for anticoagulation.30
In patients with intracranial hemorrhage, evidence suggests that the intracranial hemorrhage itself increases the risk of arterial and venous thromboembolic events. Irrespective of whether a patient was previously on anticoagulation, the risk of arterial and venous thromboembolic events approaches 7% during the initial intracranial hemorrhage-related hospitalization and 9% during the first 90 days.34,50,51
To date, the only information we have about when to resume anticoagulation comes from patients taking vitamin K antagonists.
Timing after gastrointestinal bleeding
Small case series suggest that in the first 2 months after warfarin-associated gastrointestinal bleeding, there is substantial risk of rebleeding when anticoagulation is resumed—and of thrombosis when it is not.52,53 Two retrospective cohort studies may provide some guidance in this dilemma.28,54
Witt et al28 followed 442 patients who presented with gastrointestinal bleeding from any site during warfarin therapy for varied indications for up to 90 days after the index bleeding event. The risk of death was three times lower in patients who restarted warfarin than in those who did not, and their rate of thrombotic events was 10 times lower. The risk of recurrent gastrointestinal bleeding was statistically insignificant, and there were no fatal bleeding events. Anticoagulant therapy was generally resumed within 1 week of the bleeding event, at a median of 4 days.28,55
Qureshi et al54 performed a retrospective cohort study of 1,329 patients with nonvalvular atrial fibrillation who had experienced a gastrointestinal hemorrhage while taking warfarin. They found that resuming warfarin after 7 days was not associated with a higher risk of recurrent gastrointestinal bleeding and that the rates of death and thromboembolism were lower than in patients who resumed warfarin after 30 days. On the other hand, the risk of recurrent gastrointestinal bleeding was significantly greater if therapy was resumed within the first week.
In view of these studies, we believe that most patients should resume anticoagulation after 4 to 7 days of interruption after gastrointestinal bleeding.55
Timing after soft-tissue hemorrhage
The literature on resuming anticoagulation after soft-tissue hemorrhage is sparse. A retrospective study52 looked at this question in patients with spontaneous rectal sheath hematoma who had been receiving antiplatelet drugs, intravenous heparin, vitamin K antagonists, or a combination of these, but not target-specific agents. More than half of the patients were on vitamin K antagonists at the time of hemorrhage. Analysis suggested that when benefits of resuming anticoagulation are believed to outweigh risks, it is reasonable to resume anticoagulation 4 days after the index event.56
Timing after intracranial hemorrhage
Anticoagulation should not be considered within the first 24 hours after intracranial hemorrhage, as over 70% of patients develop some amount of hematoma expansion during this time.34,57 The period thereafter poses a challenge, as the risk of hematoma expansion decreases while the risk of arterial and venous thromboembolism is ongoing and cumulative.50
Perhaps surprisingly, national guidelines suggest starting prophylactic-dosed anticoagulation early in all intracranial hemorrhage patients, including those not previously on warfarin.58,59 In a randomized trial, Boeer et al60 concluded that starting low-dose subcutaneous heparin the day after an intracranial hemorrhage decreased the risk of thromboembolism without increasing the risk of rebleeding.60 Dickmann et al61 similarly concluded that there was no increased risk of rebleeding with early prophylactic-dosed subcutaneous heparin.61 Optimal mechanical thromboprophylaxis, including graduated compression stockings and intermittent pneumatic compression stockings, is also encouraged.34
Expert opinion remains divided on when and if anticoagulants should be resumed.34,62 The American Heart Association suggests that in nonvalvular atrial fibrillation, long-term anticoagulation should be avoided after spontaneous lobar hemorrhage; antiplatelet agents can be considered instead.58 In nonlobar hemorrhage, the American Heart Association suggests that anticoagulation be considered, depending on strength of indication, 7 to 10 days after the onset.58 The European Stroke Initiative suggests patients with strong indications for anticoagulation be restarted on warfarin 10 to 14 days after the event, depending on the risk of thromboembolism and recurrent intracranial hemorrhage.59 Others suggest delaying resumption to 10 to 30 weeks after an index intracranial hemorrhage.63
Overall, in the immediate acute period of intracranial hemorrhage, most patients will likely benefit from acute reversal of anticoagulation, followed by institution of prophylactic-dose anticoagulation after the first 24 hours. Going forward, patients who remain at higher risk of a recurrence of anticoagulant-related intracranial hemorrhage (such as those with lobar hemorrhage, suspected cerebral amyloid angiopathy, and other high-risk factors) than of thromboembolic events may be best managed without anticoagulants. Alternatively, patients with deep hemispheric intracranial hemorrhage, hypertension that can be well controlled, and a high risk of serious thromboembolism may experience net benefit from restarting anticoagulation.34
We recommend considering restarting anticoagulation 7 days after the onset of intracranial hemorrhage in patients at high risk of thromboembolism and after at least 14 days for patients at lower risk (Table 2). Discussions with neurologic and neurosurgical consultants should also inform this timing decision.
WOULD A NEWER DRUG BE A BETTER CHOICE?
The emergence of target-specific oral anticoagulants, including factor Xa inhibitors such as rivaroxaban, apixaban, and edoxaban and the direct thrombin inhibitor dabigatran etexilate, presents further challenges in managing anticoagulation after hemorrhage. Table 4 summarizes the current FDA-approved indications.64–67
These newer agents are attractive because, compared with warfarin, they have wider therapeutic windows, faster onset and offset of action, and fewer drug and food interactions.68 A meta-analysis of data available to date suggests that the new drugs, compared with warfarin, show a favorable risk-benefit profile with reductions in stroke, intracranial hemorrhage, and mortality with similar overall major bleeding rates, except for a possible increase in gastrointestinal bleeding.68
However, when managing anticoagulation after a bleeding event, the newer agents are challenging for two reasons: they may be associated with a higher incidence of gastrointestinal bleeding than warfarin, and they lack the typical reversal agents that can be used to manage an acute bleeding event.68,69
In individual studies comparing warfarin with dabigatran,70 rivaroxaban,71 apixaban,72 or edoxaban73 for stroke prevention in patients with atrial fibrillation, there was no significant difference in the rate of major bleeding between dabigatran in its higher dose (150 mg twice a day) or rivaroxaban compared with warfarin.70,71 The risk of major bleeding was actually lower with apixaban72 and edoxaban.73
In regard to specific types of major bleeding, the rate of intracranial hemorrhage was significantly lower with dabigatran, rivaroxaban, apixaban, and edoxaban than with warfarin.35,68–73 Some have proposed that since the brain is high in tissue factor, inhibition of tissue factor-factor VIIa complexes by vitamin K antagonists leaves the brain vulnerable to hemorrhage. Others suggest that the targeted mechanism of target-specific agents, as opposed to the multiple pathways in both the intrinsic and extrinsic coagulation cascade that vitamin K antagonists affect, may explain this difference.35,74,75
However, some studies suggest that rivaroxaban and the higher doses of dabigatran and edoxaban are associated with higher rates of major gastrointestinal bleeding compared with warfarin.69–71,76 But apixaban demonstrated no significant difference in gastrointestinal bleeding, and instead demonstrated rates of gastrointestinal bleeding comparable to that with aspirin for stroke prevention in atrial fibrillation.72
The new oral anticoagulants lack antidotes or reversal agents such as phytonadione and fresh-frozen plasma that are available to manage warfarin-associated bleeding events. Other proposed reversal options for the new agents include activated charcoal (if the drugs were taken recently enough to remain in the gastrointestinal tract) and concentrated clotting factor product, though research is ongoing in regards to the most appropriate use in clinical practice.37,69 Unlike rivaroxaban and apixaban, dabigatran has low plasma protein binding and is dialyzable, which provides another strategy in managing dabigatran-related bleeding.69
Of note, the above bleeding risk calculations relate to the first anticoagulant-related bleeding event, though presumably the same risk comparison across agents may be applicable to rebleeding events. Given the data above, when anticoagulation is to be resumed after an intracranial hemorrhage, the risk of rebleeding, particularly in the form of recurrent intracranial hemorrhage, may be lower if a target-specific oral anticoagulant is used.75 Similarly, when anticoagulation is to be resumed after a gastrointestinal bleeding event, reinitiation with warfarin or apixaban therapy may present the lowest risk of recurrent gastrointestinal rebleeding. In other sources of bleeding, such as retroperitoneal bleeding, we suggest consideration of transitioning to warfarin, given the availability of reversal agents in the event of recurrent bleeding.
Other important drug-specific factors that must be noted when selecting an agent with which to resume anticoagulation after a hemorrhage include the following:
- In patients with significant renal impairment, the choice of agent will be limited to a vitamin K antagonist.77
- A meta-analysis of randomized clinical trials suggests that in the elderly (age 75 and older) target-specific oral anticoagulants did not cause excess bleeding and were associated with at least equal efficacy compared with vitamin K antagonists.78
- Target-specific oral anticoagulants may be beneficial in patients who have challenges in achieving INR targets, as evidence suggests that switching to them is associated with a reduction in bleeding for patients who struggle to maintain an appropriately therapeutic INR.68 On the other hand, if there is concern that a patient may occasionally miss doses of an anticoagulant, given the rapid onset and offset of action of target-specific agents compared with warfarin, a missed dose of a target-specific agent may result in faster dissolution of anticoagulant effect and increased risk of thrombotic events, and lapses in anticoagulation will not be identified by routine drug monitoring.6–8,75 As such, it is vital to have a frank discussion with any patient who has difficulty maintaining therapeutic INRs on warfarin treatment to make sure that he or she is not missing doses.
- If there is no clear and compelling reason to select a particular agent, cost considerations should be taken into account. We have included estimated 30-day pricing for the various agents in Table 4.
- Jaffer AK, Brotman DJ, Bash LD, Mahmood SK, Lott B, White RH. Variations in perioperative warfarin management: outcomes and practice patterns at nine hospitals. Am J Med 2010; 123:141–150.
- Kaatz S, Douketis JD, Zhou H, Gage BF, White RH. Risk of stroke after surgery in patients with and without chronic atrial fibrillation. J Thromb Haemost 2010; 8:884–890.
- Raunsø J, Selmer C, Olesen JB, et al. Increased short-term risk of thrombo-embolism or death after interruption of warfarin treatment in patients with atrial fibrillation. Eur Heart J 2012; 33:1886–1892.
- Xarelto (rivaroxaban). Highlights of prescribing information. Jansen Pharmaceuticals, Inc. www.xareltohcp.com/sites/default/files/pdf/xarelto_0.pdf#zoom=100. Accessed March 9, 2015.
- Pradaxa (dabigatran etexilate mesylate). Highlights of prescribing information. Boehringer Ingelheim Pharmaceuticals, Inc. http://bidocs.boehringer-ingelheim.com/BIWebAccess/ViewServlet.ser?docBase=renetnt&folderPath=/Prescribing%20Information/PIs/Pradaxa/Pradaxa.pdf. Accessed March 9, 2015.
- Eliquis (apixaban). Highlights of prescribing information. Bristol-Myers Squibb Company. http://packageinserts.bms.com/pi/pi_eliquis.pdf. Accessed March 9, 2015.
- Schulman S, Beyth RJ, Kearon C, Levine MN; American College of Chest Physicians. Hemorrhagic complications of anticoagulant and thrombolytic treatment: American College of Chest Physicians Evidence-Based Clinical Practice Guidelines (8th ed). Chest 2008; 133(suppl 6):257S–298S.
- Siegal DM, Garcia DA, Crowther MA. How I treat target-specific oral anticoagulant-associated bleeding. Blood 2014; 123:1152–1158.
- Douketis JD, Spyropoulos AC, Spencer FA, et al; American College of Chest Physicians. Perioperative management of antithrombotic therapy: Antithrombotic Therapy and Prevention of Thrombosis, 9th ed: American College of Chest Physicians Evidence-Based Clinical Practice Guidelines. Chest 2012; 141(suppl 2):e326S–e350S.
- January CT, Wann LS, Alpert JS, et al. 2014 AHA/ACC/HRS Guideline for the management of patients with atrial fibrillation: a report of the American College of Cardiology/American Heart Association Task Force on Practice Guidelines and the Heart Rhythm Society. J Am Coll Cardiol 2014; 64:e1–e76.
- Cannegieter SC, Rosendaal FR, Briët E. Thromboembolic and bleeding complications in patients with mechanical heart valve prostheses. Circulation 1994; 89:635–641.
- Warkentin TE. Aspirin for dual prevention of venous and arterial thrombosis. N Engl J Med 2012; 367:2039–2041.
- Simes J, Becattini C, Agnelli G, et al; INSPIRE Study Investigators* (International Collaboration of Aspirin Trials for Recurrent Venous Thromboembolism). Aspirin for the Prevention of Recurrent Venous Thromboembolism: The INSPIRE Collaboration. Circulation 2014; 130:1062–1071.
- Becattini C, Agnelli G, Schenone A, et al; WARFASA Investigators. Aspirin for preventing the recurrence of venous thromboembolism. N Engl J Med 2012; 366:1959–1967.
- Brighton TA, Eikelboom JW, Mann K, et al; ASPIRE Investigators. Low-dose aspirin for preventing recurrent venous thromboembolism. N Engl J Med 2012; 367:1979–1987.
- Wakefield TW, Obi AT, Henke PK. An aspirin a day to keep the clots away: can aspirin prevent recurrent thrombosis in extended treatment for venous thromboembolism? Circulation 2014; 130:1031–1033.
- Kearon C, Hirsh J. Management of anticoagulation before and after elective surgery. N Engl J Med 1997; 336:1506–1511.
- Coon WW, Willis PW 3rd. Recurrence of venous thromboembolism. Surgery 1973; 73:823–827.
- Hull R, Delmore T, Genton E, et al. Warfarin sodium versus low-dose heparin in the long-term treatment of venous thrombosis. N Engl J Med 1979; 301:855–858.
- Jaffer AK, Brotman DJ, Chukwumerije N. When patients on warfarin need surgery. Cleve Clin J Med 2003; 70:973–984.
- Boutitie F, Pinede L, Schulman S, et al. Influence of preceding length of anticoagulant treatment and initial presentation of venous thromboembolism on risk of recurrence after stopping treatment: analysis of individual participants’ data from seven trials. BMJ 2011; 342:d3036.
- Guerrouij M, Uppal CS, Alklabi A, Douketis JD. The clinical impact of bleeding during oral anticoagulant therapy: assessment of morbidity, mortality and post-bleed anticoagulant management. J Thromb Thrombolysis 2011; 31:419–423.
- Mehran R, Rao SV, Bhatt DL, et al. Standardized bleeding definitions for cardiovascular clinical trials: a consensus report from the Bleeding Academic Research Consortium. Circulation 2011; 123:2736–2747.
- Schulman S, Kearon C; Subcommittee on Control of Anticoagulation of the Scientific and Standardization Committee of the International Society on Thrombosis and Haemostasis. Definition of major bleeding in clinical investigations of antihemostatic medicinal products in non-surgical patients. J Thromb Haemost 2005; 3:692–694.
- Wiviott SD, Antman EM, Gibson CM, et al; TRITON-TIMI 38 Investigators. Evaluation of prasugrel compared with clopidogrel in patients with acute coronary syndromes: design and rationale for the TRial to assess Improvement in Therapeutic Outcomes by optimizing platelet InhibitioN with prasugrel Thrombolysis In Myocardial Infarction 38 (TRITON-TIMI 38). Am Heart J 2006; 152:627–635.
- Landefeld CS, Beyth RJ. Anticoagulant-related bleeding: clinical epidemiology, prediction, and prevention. Am J Med 1993; 95:315–328.
- Fang MC, Go AS, Chang Y, et al. Death and disability from warfarin-associated intracranial and extracranial hemorrhages. Am J Med 2007; 120:700–705.
- Witt DM, Delate T, Garcia DA, et al. Risk of thromboembolism, recurrent hemorrhage, and death after warfarin therapy interruption for gastrointestinal tract bleeding. Arch Intern Med 2012; 172:1484–1491.
- Holster IL, Valkhoff VE, Kuipers EJ, Tjwa ET. New oral anticoagulants increase risk for gastrointestinal bleeding: a systematic review and meta-analysis. Gastroenterology 2013; 145:105-112.e15.
- Loor G, Bassiouny H, Valentin C, Shao MY, Funaki B, Desai T. Local and systemic consequences of large retroperitoneal clot burdens. World J Surg 2009; 33:1618–1625.
- Satasivam P, Reeves F, Lin M, et al. The effect of oral anticoagulation on the prevalence and management of haematuria in a contemporary Australian patient cohort. BJU Int 2012; 110(suppl 4):80–84.
- Van Savage JG, Fried FA. Anticoagulant associated hematuria: a prospective study. J Urol 1995; 153:1594–1596.
- Mosley DH, Schatz IJ, Breneman GM, Keyes JW. Long-term anticoagulant therapy. Complications and control in a review of 978 cases. JAMA 1963; 186:914–916.
- Goldstein JN, Greenberg SM. Should anticoagulation be resumed after intracerebral hemorrhage? Cleve Clin J Med 2010; 77:791–799.
- Caldeira D, Barra M, Pinto FJ, Ferreira JJ, Costa J. Intracranial hemorrhage risk with the new oral anticoagulants: a systematic review and meta-analysis. J Neurol 2014 Aug 14. [Epub ahead of print]
- Holbrook A, Schulman S, Witt DM, et al; American College of Chest Physicians. Evidence-based management of anticoagulant therapy: Antithrombotic Therapy and Prevention of Thrombosis, 9th ed: American College of Chest Physicians Evidence-Based Clinical Practice Guidelines. Chest 2012; 141(suppl 2):e152S–e184S.
- Ageno W, Gallus AS, Wittkowsky A, Crowther M, Hylek EM, Palareti G; American College of Chest Physicians. Oral anticoagulant therapy: Antithrombotic Therapy and Prevention of Thrombosis, 9th ed: American College of Chest Physicians Evidence-Based Clinical Practice Guidelines. Chest 2012; 141(suppl 2):e44S–e88S.
- Poli D, Antonucci E, Dentali F, et al; Italian Federation of Anticoagulation Clinics (FCSA). Recurrence of ICH after resumption of anticoagulation with VK antagonists: CHIRONE study. Neurology 2014; 82:1020–1026.
- Choi JH, Mast H, Sciacca RR, et al. Clinical outcome after first and recurrent hemorrhage in patients with untreated brain arteriovenous malformation. Stroke 2006; 37:1243–1247.
- Chon KH, Lee JM, Koh EJ, Choi HY. Independent predictors for recurrence of chronic subdural hematoma. Acta Neurochir (Wien) 2012; 154:1541–1548.
- Oake N, Jennings A, Forster AJ, et al. Anticoagulation intensity and outcomes among patients prescribed oral anticoagulant therapy: a systematic review and meta-analysis. CMAJ 2008; 179:235–244.
- Whitlock RP, Sun JC, Fremes SE, Rubens FD, Teoh KH; American College of Chest Physicians. Antithrombotic and thrombolytic therapy for valvular disease: Antithrombotic Therapy and Prevention of Thrombosis, 9th ed: American College of Chest Physicians Evidence-Based Clinical Practice Guidelines. Chest 2012; 141(suppl 2):e576S–e600S.
- Bonow RO, Carabello BA, Chatterjee K, et al; 2006 Writing Committee Members; American College of Cardiology/American Heart Association Task Force. 2008 Focused update incorporated into the ACC/AHA 2006 guidelines for the management of patients with valvular heart disease. Circulation 2008; 118:e523–e661.
- Clemens A, Strack A, Noack H, Konstantinides S, Brueckmann M, Lip GY. Anticoagulant-related gastrointestinal bleeding—could this facilitate early detection of benign or malignant gastrointestinal lesions? Ann Med 2014; 46:672–678.
- Khalid F, Qureshi W, Qureshi S, Alirhayim Z, Garikapati K, Patsias I. Impact of restarting warfarin therapy in renal disease anticoagulated patients with gastrointestinal hemorrhage. Ren Fail 2013; 35:1228–1235.
- Man-Son-Hing M, Nichol G, Lau A, Laupacis A. Choosing antithrombotic therapy for elderly patients with atrial fibrillation who are at risk for falls. Arch Intern Med 1999; 159:677–685.
- Davidson BL, Verheijen S, Lensing AW, et al. Bleeding risk of patients with acute venous thromboembolism taking nonsteroidal anti-inflammatory drugs or aspirin. JAMA Intern Med 2014; 174:947–953.
- Knijff-Dutmer EA, Schut GA, van de Laar MA. Concomitant coumarin-NSAID therapy and risk for bleeding. Ann Pharmacother 2003; 37:12–16.
- Heidbuchel H, Verhamme P, Alings M, et al; European Heart Rhythm Association. European Heart Rhythm Association Practical Guide on the use of new oral anticoagulants in patients with non-valvular atrial fibrillation. Europace 2013; 15:625–651.
- Goldstein JN, Fazen LE, Wendell L, et al. Risk of thromboembolism following acute intracerebral hemorrhage. Neurocrit Care 2009; 10:28–34.
- Christensen MC, Dawson J, Vincent C. Risk of thromboembolic complications after intracerebral hemorrhage according to ethnicity. Adv Ther 2008; 25:831–841.
- Ananthasubramaniam K, Beattie JN, Rosman HS, Jayam V, Borzak S. How safely and for how long can warfarin therapy be withheld in prosthetic heart valve patients hospitalized with a major hemorrhage? Chest 2001; 119:478–484.
- Lee JK, Kang HW, Kim SG, Kim JS, Jung HC. Risks related with withholding and resuming anticoagulation in patients with non-variceal upper gastrointestinal bleeding while on warfarin therapy. Int J Clin Pract 2012; 66:64–68.
- Qureshi W, Mittal C, Patsias I, et al. Restarting anticoagulation and outcomes after major gastrointestinal bleeding in atrial fibrillation. Am J Cardiol 2014; 113:662–668.
- Brotman DJ, Jaffer AK. Resuming anticoagulation in the first week following gastrointestinal tract hemorrhage: should we adopt a 4-day rule? Arch Intern Med 2012; 172:1492–1493.
- Kunkala MR1, Kehl J, Zielinski MD. Spontaneous rectus sheath hematomas: when to restart anticoagulation? World J Surg 2013; 37:2555–2559.
- Davis SM, Broderick J, Hennerici M, et al; Recombinant Activated Factor VII Intracerebral Hemorrhage Trial Investigators. Hematoma growth is a determinant of mortality and poor outcome after intracerebral hemorrhage. Neurology 2006; 66:1175–1181.
- Broderick J, Connolly S, Feldmann E, et al; American Heart Association; American Stroke Association Stroke Council; High Blood Pressure Research Council; Quality of Care and Outcomes in Research Interdisciplinary Working Group. Guidelines for the management of spontaneous intracerebral hemorrhage in adults. Stroke 2007; 38:2001–2023.
- Steiner T, Kaste M, Forsting M, et al. Recommendations for the management of intracranial haemorrhage—part I: spontaneous intracerebral haemorrhage. The European Stroke Initiative Writing Committee and the Writing Committee for the EUSI Executive Committee. Cerebrovasc Dis 2006; 22:294–316. Erratum in: Cerebrovasc Dis 2006; 22:461.
- Boeer A, Voth E, Henze T, Prange HW. Early heparin therapy in patients with spontaneous intracerebral haemorrhage. J Neurol Neurosurg Psychiatry 1991; 54:466–467.
- Dickmann U, Voth E, Schicha H, Henze T, Prange H, Emrich D. Heparin therapy, deep-vein thrombosis and pulmonary embolism after intracerebral hemorrhage. Klin Wochenschr 1988; 66:1182–1183.
- Aguilar MI, Hart RG, Kase CS, et al. Treatment of warfarin-associated intracerebral hemorrhage: literature review and expert opinion. Mayo Clin Proc 2007; 82:82–92. Erratum in: Mayo Clin Proc 2007; 82:387.
- Majeed A, Kim YK, Roberts RS, Holmström M, Schulman S. Optimal timing of resumption of warfarin after intracranial hemorrhage. Stroke 2010; 41:2860–2866.
- US Food and Drug Administration. Drug Information. XARELTO (rivaroxaban) tablets, for oral use. www.accessdata.fda.gov/drugsatfda_docs/label/2013/022406s004lbl.pdf. Accessed March 9, 2015.
- US Food and Drug Administration. Drug Information. ELIQUIS® (apixaban) tablets for oral use. www.accessdata.fda.gov/drugsatfda_docs/label/2014/202155s009lbl.pdf. Accessed March 9, 2015.
- US Food and Drug Administration. Drug Information. PRADAXA® (dabigatran etexilate mesylate) capsules for oral use. www.accessdata.fda.gov/drugsatfda_docs/label/2014/022512s023lbl.pdf. Accessed March 9, 2015.
- New oral anticoagulants for acute venous thromboembolism. Med Lett Drugs Ther 2014; 56:3–4.
- Ruff CT, Giugliano RP, Braunwald E, et al. Comparison of the efficacy and safety of new oral anticoagulants with warfarin in patients with atrial fibrillation: a meta-analysis of randomised trials. Lancet 2014; 383:955–962.
- Nutescu EA, Dager WE, Kalus JS, Lewin JJ 3rd, Cipolle MD. Management of bleeding and reversal strategies for oral anticoagulants: clinical practice considerations. Am J Health Syst Pharm 2013; 70:1914–1929.
- Connolly SJ, Ezekowitz MD, Yusuf S, et al; RE-LY Steering Committee and Investigators. Dabigatran versus warfarin in patients with atrial fibrillation. N Engl J Med 2009; 361:1139–1151. Erratum in: N Engl J Med 2010; 363:1877.
- Patel MR, Mahaffey KW, Garg J, et al; ROCKET AF Investigators. Rivaroxaban versus warfarin in nonvalvular atrial fibrillation. N Engl J Med 2011; 365:883–891.
- Granger CB, Alexander JH, McMurray JJ, et al; ARISTOTLE Committees and Investigators. Apixaban versus warfarin in patients with atrial fibrillation. N Engl J Med 2011; 365:981–992.
- Hokusai-VTE Investigators, Büller HR, Décousus H, Grosso MA, et al. Edoxaban versus warfarin for the treatment of symptomatic venous thromboembolism. N Engl J Med 2013; 369:1406–1415.
- Mackman N. The role of tissue factor and factor VIIa in hemostasis. Anesth Analg 2009; 108:1447–1452.
- Chatterjee S, Sardar P, Biondi-Zoccai G, Kumbhani DJ. New oral anticoagulants and the risk of intracranial hemorrhage: traditional and Bayesian meta-analysis and mixed treatment comparison of randomized trials of new oral anticoagulants in atrial fibrillation. JAMA Neurol 2013; 70:1486–1490.
- Loffredo L, Perri L, Violi F. Impact of new oral anticoagulants on gastrointestinal bleeding in atrial fibrillation: a meta-analysis of interventional trials. Dig Liver Dis 2015 Feb 7. pii: S1590-8658(15)00189-9. doi: 10.1016/j.dld.2015.01.159. [Epub ahead of print]
- Thachil J. The newer direct oral anticoagulants: a practical guide. Clin Med 2014; 14:165–175.
- Sardar P, Chatterjee S, Chaudhari S, Lip GY. New oral anticoagulants in elderly adults: evidence from a meta-analysis of randomized trials. J Am Geriatr Soc 2014; 62:857–864.
If a patient receiving anticoagulant therapy suffers a bleeding event, the patient and physician must decide whether and how soon to restart the therapy, and with what agent.
Foremost on our minds tends to be the risk of another hemorrhage. Subtler to appreciate immediately after an event is the continued risk of thrombosis, often from the same medical condition that prompted anticoagulation therapy in the first place (Table 1).
Complicating the decision, there may be a rebound effect: some thrombotic events such as pulmonary embolism and atrial fibrillation-related stroke may be more likely to occur in the first weeks after stopping warfarin than during similar intervals in patients who have not been taking it.1–3 The same thing may happen with the newer, target-specific oral anticoagulants.4–6
Although we have evidence-based guidelines for initiating and managing anticoagulant therapy, ample data on adverse events, and protocols for reversing anticoagulation if bleeding occurs, we do not have clear guidelines on restarting anticoagulation after a hemorrhagic event.
In this article, we outline a practical framework for approaching this clinical dilemma. Used in conjunction with consideration of a patient’s values and preferences as well as input from experts, this framework can help clinicians guide their patients through this challenging clinical decision. It consists of five questions:
- Why is the patient on anticoagulation, and what is the risk of thromboembolism without it?
- What was the clinical impact of the hemorrhage, and what is the risk of rebleeding if anticoagulation is resumed?
- What additional patient factors should be taken into consideration?
- How long should we wait before restarting anticoagulation?
- Would a newer drug be a better choice?
BLEEDING OCCURS IN 2% TO 3% OF PATIENTS PER YEAR
Most of our information on anticoagulation is about vitamin K antagonists—principally warfarin, in use since the 1950s. Among patients taking warfarin outside of clinical trials, the risk of major bleeding is estimated at 2% to 3% per year.7
However, the target-specific oral anticoagulants rivaroxaban (Xarelto), apixaban (Eliquis), dabigatran (Pradaxa) and edoxaban (Savaysa) are being used more and more, and we include them in our discussion insofar as we have information on them. The rates of bleeding with these new drugs in clinical trials have been comparable to or lower than those with warfarin.8 Postmarketing surveillance is under way.
WHY IS THE PATIENT ON ANTICOAGULATION? WHAT IS THE RISK WITHOUT IT?
Common, evidence-based indications for anticoagulation are to prevent complications in patients with venous thromboembolism and to prevent stroke in patients with atrial fibrillation or a mechanical heart valve. Other uses, such as in heart failure and its sequelae, pulmonary hypertension, and splanchnic or hepatic vein thrombosis, have less robust evidence to support them.
When anticoagulation-related bleeding occurs, it is essential to review why the patient is taking the drug and the risk of thromboembolism without it. Some indications pose a higher risk of thromboembolism than others and so argue more strongly for continuing the treatment.
Douketis et al9 developed a risk-stratification scheme for perioperative thromboembolism. We have modified it by adding the CHA2DS2-VASc score (Table 2),9–11 and believe it can be used more widely.
High-risk indications
Conditions that pose a high risk of thrombosis almost always require restarting anticoagulation. Here, the most appropriate question nearly always is not if anticoagulation should be restarted, but when. Examples:
- A mechanical mitral valve
- Antiphospholipid antibody syndrome with recurrent thromboembolic events.
Lower-risk indications
Lower-risk indications allow more leeway in determining if anticoagulation should be resumed. The most straightforward cases fall well within established guidelines. Examples:
- Atrial fibrillation and a CHA2DS2-VASc score of 1. The 2014 guidelines from the American College of Cardiology, American Heart Association, and Heart Rhythm Society10 suggest that patients with nonvalvular atrial fibrillation and a CHA2DS2-VASc score of 1 have three options: an oral anticoagulant, aspirin, and no antithrombotic therapy. If such a patient on anticoagulant therapy subsequently experiences a major gastrointestinal hemorrhage requiring transfusion and intensive care and no definitively treatable source of bleeding is found on endoscopy, one can argue that the risks of continued anticoagulation (recurrent bleeding) now exceed the benefits and that the patient would be better served by aspirin or even no antithrombotic therapy.
- After 6 months of anticoagulation for unprovoked deep vein thrombosis. Several studies showed that aspirin reduced the risk of recurrent venous thromboembolism in patients who completed an initial 6-month course of anticoagulation.12–15 Though these studies did not specifically compare aspirin with warfarin or target-specific oral anticoagulants in preventing recurrent venous thromboembolism after a hemorrhage, it is reasonable to extrapolate their results to this situation.
If the risk of recurrent hemorrhage on anticoagulation is considered to be too great, then aspirin is an alternative to no anticoagulation, as it reduces the risk of recurrent venous thromboembolism.16 However, we advise caution if the bleeding lesion may be specifically exacerbated by aspirin, particularly upper gastrointestinal ulcers.
Moderate-risk indications
- After a partial course of anticoagulation for provoked venous thromboembolism. Suppose a patient in the 10th week of a planned 12-week course of anticoagulation for a surgically provoked, first deep vein thrombosis presents with abdominal pain and is found to have a retroperitoneal hematoma. In light of the risk of recurrent bleeding vs the benefit of resuming anticoagulation for the limited remaining period, her 12-week treatment course can reasonably be shortened to 10 weeks.
The risk of recurrent venous thromboembolism when a patient is off anticoagulation decreases with time from the initial event. The highest risk, estimated at 0.3% to 1.3% per day, is in the first 4 weeks, falling to 0.03% to 0.2% per day in weeks 5 through 12, and 0.05% per day thereafter.17–20
Additionally, a pooled analysis of seven randomized trials suggests that patients with isolated, distal deep vein thrombosis provoked by a temporary risk factor did not have a high risk of recurrence after being treated for 4 to 6 weeks.21 These analyses are based on vitamin K antagonists, though it seems reasonable to extrapolate this information to the target-specific oral anticoagulants.
More challenging are situations in which the evidence supporting the initial or continued need for anticoagulation is less robust, such as in heart failure, pulmonary hypertension, or splanchnic and hepatic vein thrombosis. In these cases, the lack of strong evidence supporting the use of anticoagulation should make us hesitate to resume it after bleeding.
WHAT WAS THE CLINICAL IMPACT? WHAT IS THE RISK OF REBLEEDING?
Different groups have defined major and minor bleeding in different ways.22,23 Several have proposed criteria to standardize how bleeding events (on warfarin and otherwise) are classified,23–25 but the definitions differ.
Specifically, all agree that a “major” bleeding event is one that is fatal, involves bleeding into a major organ, or leads to a substantial decline in hemoglobin level. However, the Thrombolysis in Myocardial Infarction trials use a decline of more than 5 g/dL in their definition,23,25 while the International Society on Thrombosis and Haemostasis uses 2 g/dL.24
Here, we review the clinical impact of the most common sources of anticoagulation-related hemorrhage—gastrointestinal, soft tissue, and urinary tract26—as well as intracerebral hemorrhage, a less common but more uniformly devastating event.27
Clinical impact of gastrointestinal hemorrhage
Each year, about 4.5% of patients taking warfarin have a gastrointestinal hemorrhage, though not all of these events are major.28 Evolving data suggest that the newer agents (particularly dabigatran, rivaroxaban, and edoxaban) pose a higher risk of gastrointestinal bleeding than warfarin.29 Patients may need plasma and blood transfusions and intravenous phytonadione, all of which carry risks, albeit small.
Frequently, endoscopy is needed to find the source of bleeding and to control it. If this does not work, angiographic intervention to infuse vasoconstrictors or embolic coils into the culprit artery may be required, and some patients need surgery. Each intervention carries its own risk.
Clinical impact of soft-tissue hemorrhage
Soft-tissue hemorrhage accounts for more than 20% of warfarin-related bleeding events26; as yet, we know of no data on the rate with the new drugs. Soft-tissue hemorrhage is often localized to the large muscles of the retroperitoneum and legs. Though retroperitoneal hemorrhage accounts for a relatively small portion of soft-tissue hemorrhages, it is associated with high rates of morbidity and death and will therefore be our focus.26
Much of the clinical impact of retroperitoneal hemorrhage is from a mass effect that causes abdominal compartment syndrome, hydroureter, ileus, abscess formation, and acute and chronic pain. At least 20% of cases are associated with femoral neuropathy. It can also lead to deep vein thrombosis from venous compression, coupled with hypercoagulability in response to bleeding. Brisk bleeding can lead to shock and death, and the mortality rate in retroperitoneal hemorrhage is estimated at 20% or higher.30
In many cases, the retroperitoneal hemorrhage will self-tamponade and the blood will be reabsorbed once the bleeding has stopped, but uncontrolled bleeding may require surgical or angiographic intervention.30
Clinical impact of urinary tract hemorrhage
Gross or microscopic hematuria can be found in an estimated 2% to 24% of patients taking warfarin31–33; data are lacking for the target-specific oral anticoagulants. Interventions required to manage urinary tract bleeding include bladder irrigation and, less often, transfusion.31 Since a significant number of cases of hematuria are due to neoplastic disease,32 a diagnostic workup with radiographic imaging of the upper tract and cystoscopy of the lower tract is usually required.31 While life-threatening hemorrhage is uncommon, complications such as transient urinary obstruction from clots may occur.
Clinical impact of intracranial hemorrhage
Intracranial hemorrhage is the most feared and deadly of the bleeding complications of anticoagulation. The incidence in patients on warfarin is estimated at 2% to 3% per year, which is markedly higher than the estimated incidence of 25 per 100,000 person-years in the general population.34 Emerging data indicate that the newer drugs are also associated with a risk of intracranial hemorrhage, though the risk is about half that with vitamin K antagonists.35 Intracranial hemorrhage leads to death or disability in 76% of cases, compared with 3% of cases of bleeding from the gastrointestinal or urinary tract.27
Regardless of the source of bleeding, hospitalization is likely to be required and may be prolonged, with attendant risks of nosocomial harms such as infection.
Risk of rebleeding
Given the scope and severity of anticoagulation-related bleeding, there is strong interest in predicting and preventing it. By some estimates, the incidence of recurrent bleeding after resuming vitamin K antagonists is 8% to 13%.22 Although there are several indices for predicting the risk of major bleeding when starting anticoagulation, there are currently no validated tools to estimate a patient’s risk of rebleeding.36
The patient factor that most consistently predicts major bleeding is a history of bleeding, particularly from the gastrointestinal tract. Finding and controlling the source of bleeding is important.26,37 For example, a patient with gross hematuria who is found on cystoscopy to have a urothelial papilloma is unlikely to have rebleeding if the tumor is successfully resected and serial follow-up shows no regrowth. In contrast, consider a patient with a major gastrointestinal hemorrhage, the source of which remains elusive after upper, lower, and capsule endoscopy or, alternatively, is suspected to be from one of multiple angiodysplastic lesions. Without definitive source management, this patient faces a high risk of rebleeding.
With or without anticoagulation, after a first intracranial hemorrhage the risk of another one is estimated at 2% to 4% per year.34 An observational study found a recurrence rate of 7.5% when vitamin K antagonist therapy was started after an intracranial hemorrhage (though not all patients were on a vitamin K antagonist at the time of the first hemorrhage).38
Patients with lobar hemorrhage and those with suspected cerebral amyloid angiopathy may be at particularly high risk if anticoagulation is resumed. Conversely, initial events attributed to uncontrolled hypertension that subsequently can be well controlled may portend a lower risk of rebleeding.34 For other types of intracranial hemorrhage, recurrence rates can be even higher. Irrespective of anticoagulation, one prospective study estimated the crude annual rebleeding rate with untreated arteriovenous malformations to be 7%.39 In chronic subdural hematoma, the recurrence rate after initial drainage has been estimated at 9.2% to 26.5%, with use of anticoagulants (in this case, vitamin K antagonists) being an independent predictor of recurrence.40
WHAT OTHER PATIENT FACTORS NEED CONSIDERATION?
Target INR on warfarin
An important factor influencing the risk of bleeding with warfarin is the intensity of this therapy.37 A meta-analysis41 found that the risks of major hemorrhage and thromboembolism are minimized if the goal international normalized ratio (INR) is 2.0 to 3.0. When considering resuming anticoagulation after bleeding, make sure the therapeutic target is appropriate.37
Table 3 summarizes recommended therapeutic ranges for frequently encountered indications for warfarin.36,42,43
INR at time of the event and challenges in controlling it
The decision to resume anticoagulation in patients who bled while using warfarin must take into account the actual INR at the time of the event.
For example, consider a patient whose INR values are consistently in the therapeutic range. While on vacation, he receives ciprofloxacin for acute prostatitis from an urgent care team, and no adjustment to INR monitoring or warfarin dose is made. Several days later, he presents with lower gastrointestinal bleeding. His INR is 8, and colonoscopy reveals diverticulosis with a bleeding vessel, responsive to endoscopic therapy. After controlling the source of bleeding and reinforcing the need to always review new medications for potential interactions with anticoagulation, it is reasonable to expect that he once again will be able to keep his INR in the therapeutic range.
A patient on anticoagulation for the same indication but who has a history of repeated supratherapeutic levels, poor adherence, or poor access to INR monitoring poses very different concerns about resuming anticoagulation (as well as which agent to use, as we discuss below).
Of note, a high INR alone does not explain bleeding. It is estimated that a workup for gastrointestinal bleeding and gross hematuria uncovers previously undetected lesions in approximately one-third of cases involving warfarin.26 A similar malignancy-unmasking effect is now recognized in patients using the target-specific oral agents who experience gastrointestinal bleeding.44 Accordingly, we recommend a comprehensive source evaluation for any anticoagulation-related hemorrhage.
Comorbid conditions
Comorbid conditions associated with bleeding include cancer, end-stage renal disease, liver disease, arterial hypertension, prior stroke, and alcohol abuse.37,45 Gait instability, regardless of cause, may also increase the risk of trauma-related hemorrhage, but some have estimated that a patient would need to fall multiple times per week to contraindicate anticoagulation on the basis of falls alone.46
Concurrent medications
Concomitant therapies, including antiplatelet drugs and nonsteroidal anti-inflammatory drugs, increase bleeding risk.47,48 Aspirin and the nonsteroidals, in addition to having antiplatelet effects, also can cause gastric erosion.37 In evaluating whether and when to restart anticoagulation, it is advisable to review the role that concomitant therapies may have had in the index bleeding event and to evaluate the risks and benefits of these other agents.
Additionally, warfarin has many interactions. Although the newer drugs are lauded for having fewer interactions, they are not completely free of them, and the potential for interactions must always be reviewed.49 Further, unlike warfarin therapy, therapy with the newer agents is not routinely monitored with laboratory tests, so toxicity (or underdosing) may not be recognized until an adverse clinical event occurs. Ultimately, it may be safer to resume anticoagulation after a contributing drug can be safely discontinued.
Advanced age
The influence that the patient’s age should have on the decision to restart anticoagulation is unclear. Although the risk of intracranial hemorrhage increases with age, particularly after age 80, limited data exist in this population, particularly with regard to rebleeding. Further, age is a major risk factor for most thrombotic events, including venous thromboembolism and stroke from atrial fibrillation, so although the risks of anticoagulation may be higher, the benefits may also be higher than in younger patients.37,46 We discourage using age alone as a reason to withhold anticoagulation after a hemorrhage.
HOW LONG SHOULD WE WAIT TO RESTART ANTICOAGULATION?
We lack conclusive data on how long to wait to restart anticoagulation after an anticoagulation-associated hemorrhage.
The decision is complicated by evidence suggesting a rebound effect, with an increased risk of pulmonary embolism and atrial fibrillation-related stroke during the first 90 days of interruption of therapy with warfarin as well as with target-specific oral anticoagulants.3–8 In anticoagulation-associated retroperitoneal bleeding, there is increased risk of deep vein thrombosis from compression, even if venous thromboembolism was not the initial indication for anticoagulation.30
In patients with intracranial hemorrhage, evidence suggests that the intracranial hemorrhage itself increases the risk of arterial and venous thromboembolic events. Irrespective of whether a patient was previously on anticoagulation, the risk of arterial and venous thromboembolic events approaches 7% during the initial intracranial hemorrhage-related hospitalization and 9% during the first 90 days.34,50,51
To date, the only information we have about when to resume anticoagulation comes from patients taking vitamin K antagonists.
Timing after gastrointestinal bleeding
Small case series suggest that in the first 2 months after warfarin-associated gastrointestinal bleeding, there is substantial risk of rebleeding when anticoagulation is resumed—and of thrombosis when it is not.52,53 Two retrospective cohort studies may provide some guidance in this dilemma.28,54
Witt et al28 followed 442 patients who presented with gastrointestinal bleeding from any site during warfarin therapy for varied indications for up to 90 days after the index bleeding event. The risk of death was three times lower in patients who restarted warfarin than in those who did not, and their rate of thrombotic events was 10 times lower. The risk of recurrent gastrointestinal bleeding was statistically insignificant, and there were no fatal bleeding events. Anticoagulant therapy was generally resumed within 1 week of the bleeding event, at a median of 4 days.28,55
Qureshi et al54 performed a retrospective cohort study of 1,329 patients with nonvalvular atrial fibrillation who had experienced a gastrointestinal hemorrhage while taking warfarin. They found that resuming warfarin after 7 days was not associated with a higher risk of recurrent gastrointestinal bleeding and that the rates of death and thromboembolism were lower than in patients who resumed warfarin after 30 days. On the other hand, the risk of recurrent gastrointestinal bleeding was significantly greater if therapy was resumed within the first week.
In view of these studies, we believe that most patients should resume anticoagulation after 4 to 7 days of interruption after gastrointestinal bleeding.55
Timing after soft-tissue hemorrhage
The literature on resuming anticoagulation after soft-tissue hemorrhage is sparse. A retrospective study52 looked at this question in patients with spontaneous rectal sheath hematoma who had been receiving antiplatelet drugs, intravenous heparin, vitamin K antagonists, or a combination of these, but not target-specific agents. More than half of the patients were on vitamin K antagonists at the time of hemorrhage. Analysis suggested that when benefits of resuming anticoagulation are believed to outweigh risks, it is reasonable to resume anticoagulation 4 days after the index event.56
Timing after intracranial hemorrhage
Anticoagulation should not be considered within the first 24 hours after intracranial hemorrhage, as over 70% of patients develop some amount of hematoma expansion during this time.34,57 The period thereafter poses a challenge, as the risk of hematoma expansion decreases while the risk of arterial and venous thromboembolism is ongoing and cumulative.50
Perhaps surprisingly, national guidelines suggest starting prophylactic-dosed anticoagulation early in all intracranial hemorrhage patients, including those not previously on warfarin.58,59 In a randomized trial, Boeer et al60 concluded that starting low-dose subcutaneous heparin the day after an intracranial hemorrhage decreased the risk of thromboembolism without increasing the risk of rebleeding.60 Dickmann et al61 similarly concluded that there was no increased risk of rebleeding with early prophylactic-dosed subcutaneous heparin.61 Optimal mechanical thromboprophylaxis, including graduated compression stockings and intermittent pneumatic compression stockings, is also encouraged.34
Expert opinion remains divided on when and if anticoagulants should be resumed.34,62 The American Heart Association suggests that in nonvalvular atrial fibrillation, long-term anticoagulation should be avoided after spontaneous lobar hemorrhage; antiplatelet agents can be considered instead.58 In nonlobar hemorrhage, the American Heart Association suggests that anticoagulation be considered, depending on strength of indication, 7 to 10 days after the onset.58 The European Stroke Initiative suggests patients with strong indications for anticoagulation be restarted on warfarin 10 to 14 days after the event, depending on the risk of thromboembolism and recurrent intracranial hemorrhage.59 Others suggest delaying resumption to 10 to 30 weeks after an index intracranial hemorrhage.63
Overall, in the immediate acute period of intracranial hemorrhage, most patients will likely benefit from acute reversal of anticoagulation, followed by institution of prophylactic-dose anticoagulation after the first 24 hours. Going forward, patients who remain at higher risk of a recurrence of anticoagulant-related intracranial hemorrhage (such as those with lobar hemorrhage, suspected cerebral amyloid angiopathy, and other high-risk factors) than of thromboembolic events may be best managed without anticoagulants. Alternatively, patients with deep hemispheric intracranial hemorrhage, hypertension that can be well controlled, and a high risk of serious thromboembolism may experience net benefit from restarting anticoagulation.34
We recommend considering restarting anticoagulation 7 days after the onset of intracranial hemorrhage in patients at high risk of thromboembolism and after at least 14 days for patients at lower risk (Table 2). Discussions with neurologic and neurosurgical consultants should also inform this timing decision.
WOULD A NEWER DRUG BE A BETTER CHOICE?
The emergence of target-specific oral anticoagulants, including factor Xa inhibitors such as rivaroxaban, apixaban, and edoxaban and the direct thrombin inhibitor dabigatran etexilate, presents further challenges in managing anticoagulation after hemorrhage. Table 4 summarizes the current FDA-approved indications.64–67
These newer agents are attractive because, compared with warfarin, they have wider therapeutic windows, faster onset and offset of action, and fewer drug and food interactions.68 A meta-analysis of data available to date suggests that the new drugs, compared with warfarin, show a favorable risk-benefit profile with reductions in stroke, intracranial hemorrhage, and mortality with similar overall major bleeding rates, except for a possible increase in gastrointestinal bleeding.68
However, when managing anticoagulation after a bleeding event, the newer agents are challenging for two reasons: they may be associated with a higher incidence of gastrointestinal bleeding than warfarin, and they lack the typical reversal agents that can be used to manage an acute bleeding event.68,69
In individual studies comparing warfarin with dabigatran,70 rivaroxaban,71 apixaban,72 or edoxaban73 for stroke prevention in patients with atrial fibrillation, there was no significant difference in the rate of major bleeding between dabigatran in its higher dose (150 mg twice a day) or rivaroxaban compared with warfarin.70,71 The risk of major bleeding was actually lower with apixaban72 and edoxaban.73
In regard to specific types of major bleeding, the rate of intracranial hemorrhage was significantly lower with dabigatran, rivaroxaban, apixaban, and edoxaban than with warfarin.35,68–73 Some have proposed that since the brain is high in tissue factor, inhibition of tissue factor-factor VIIa complexes by vitamin K antagonists leaves the brain vulnerable to hemorrhage. Others suggest that the targeted mechanism of target-specific agents, as opposed to the multiple pathways in both the intrinsic and extrinsic coagulation cascade that vitamin K antagonists affect, may explain this difference.35,74,75
However, some studies suggest that rivaroxaban and the higher doses of dabigatran and edoxaban are associated with higher rates of major gastrointestinal bleeding compared with warfarin.69–71,76 But apixaban demonstrated no significant difference in gastrointestinal bleeding, and instead demonstrated rates of gastrointestinal bleeding comparable to that with aspirin for stroke prevention in atrial fibrillation.72
The new oral anticoagulants lack antidotes or reversal agents such as phytonadione and fresh-frozen plasma that are available to manage warfarin-associated bleeding events. Other proposed reversal options for the new agents include activated charcoal (if the drugs were taken recently enough to remain in the gastrointestinal tract) and concentrated clotting factor product, though research is ongoing in regards to the most appropriate use in clinical practice.37,69 Unlike rivaroxaban and apixaban, dabigatran has low plasma protein binding and is dialyzable, which provides another strategy in managing dabigatran-related bleeding.69
Of note, the above bleeding risk calculations relate to the first anticoagulant-related bleeding event, though presumably the same risk comparison across agents may be applicable to rebleeding events. Given the data above, when anticoagulation is to be resumed after an intracranial hemorrhage, the risk of rebleeding, particularly in the form of recurrent intracranial hemorrhage, may be lower if a target-specific oral anticoagulant is used.75 Similarly, when anticoagulation is to be resumed after a gastrointestinal bleeding event, reinitiation with warfarin or apixaban therapy may present the lowest risk of recurrent gastrointestinal rebleeding. In other sources of bleeding, such as retroperitoneal bleeding, we suggest consideration of transitioning to warfarin, given the availability of reversal agents in the event of recurrent bleeding.
Other important drug-specific factors that must be noted when selecting an agent with which to resume anticoagulation after a hemorrhage include the following:
- In patients with significant renal impairment, the choice of agent will be limited to a vitamin K antagonist.77
- A meta-analysis of randomized clinical trials suggests that in the elderly (age 75 and older) target-specific oral anticoagulants did not cause excess bleeding and were associated with at least equal efficacy compared with vitamin K antagonists.78
- Target-specific oral anticoagulants may be beneficial in patients who have challenges in achieving INR targets, as evidence suggests that switching to them is associated with a reduction in bleeding for patients who struggle to maintain an appropriately therapeutic INR.68 On the other hand, if there is concern that a patient may occasionally miss doses of an anticoagulant, given the rapid onset and offset of action of target-specific agents compared with warfarin, a missed dose of a target-specific agent may result in faster dissolution of anticoagulant effect and increased risk of thrombotic events, and lapses in anticoagulation will not be identified by routine drug monitoring.6–8,75 As such, it is vital to have a frank discussion with any patient who has difficulty maintaining therapeutic INRs on warfarin treatment to make sure that he or she is not missing doses.
- If there is no clear and compelling reason to select a particular agent, cost considerations should be taken into account. We have included estimated 30-day pricing for the various agents in Table 4.
If a patient receiving anticoagulant therapy suffers a bleeding event, the patient and physician must decide whether and how soon to restart the therapy, and with what agent.
Foremost on our minds tends to be the risk of another hemorrhage. Subtler to appreciate immediately after an event is the continued risk of thrombosis, often from the same medical condition that prompted anticoagulation therapy in the first place (Table 1).
Complicating the decision, there may be a rebound effect: some thrombotic events such as pulmonary embolism and atrial fibrillation-related stroke may be more likely to occur in the first weeks after stopping warfarin than during similar intervals in patients who have not been taking it.1–3 The same thing may happen with the newer, target-specific oral anticoagulants.4–6
Although we have evidence-based guidelines for initiating and managing anticoagulant therapy, ample data on adverse events, and protocols for reversing anticoagulation if bleeding occurs, we do not have clear guidelines on restarting anticoagulation after a hemorrhagic event.
In this article, we outline a practical framework for approaching this clinical dilemma. Used in conjunction with consideration of a patient’s values and preferences as well as input from experts, this framework can help clinicians guide their patients through this challenging clinical decision. It consists of five questions:
- Why is the patient on anticoagulation, and what is the risk of thromboembolism without it?
- What was the clinical impact of the hemorrhage, and what is the risk of rebleeding if anticoagulation is resumed?
- What additional patient factors should be taken into consideration?
- How long should we wait before restarting anticoagulation?
- Would a newer drug be a better choice?
BLEEDING OCCURS IN 2% TO 3% OF PATIENTS PER YEAR
Most of our information on anticoagulation is about vitamin K antagonists—principally warfarin, in use since the 1950s. Among patients taking warfarin outside of clinical trials, the risk of major bleeding is estimated at 2% to 3% per year.7
However, the target-specific oral anticoagulants rivaroxaban (Xarelto), apixaban (Eliquis), dabigatran (Pradaxa) and edoxaban (Savaysa) are being used more and more, and we include them in our discussion insofar as we have information on them. The rates of bleeding with these new drugs in clinical trials have been comparable to or lower than those with warfarin.8 Postmarketing surveillance is under way.
WHY IS THE PATIENT ON ANTICOAGULATION? WHAT IS THE RISK WITHOUT IT?
Common, evidence-based indications for anticoagulation are to prevent complications in patients with venous thromboembolism and to prevent stroke in patients with atrial fibrillation or a mechanical heart valve. Other uses, such as in heart failure and its sequelae, pulmonary hypertension, and splanchnic or hepatic vein thrombosis, have less robust evidence to support them.
When anticoagulation-related bleeding occurs, it is essential to review why the patient is taking the drug and the risk of thromboembolism without it. Some indications pose a higher risk of thromboembolism than others and so argue more strongly for continuing the treatment.
Douketis et al9 developed a risk-stratification scheme for perioperative thromboembolism. We have modified it by adding the CHA2DS2-VASc score (Table 2),9–11 and believe it can be used more widely.
High-risk indications
Conditions that pose a high risk of thrombosis almost always require restarting anticoagulation. Here, the most appropriate question nearly always is not if anticoagulation should be restarted, but when. Examples:
- A mechanical mitral valve
- Antiphospholipid antibody syndrome with recurrent thromboembolic events.
Lower-risk indications
Lower-risk indications allow more leeway in determining if anticoagulation should be resumed. The most straightforward cases fall well within established guidelines. Examples:
- Atrial fibrillation and a CHA2DS2-VASc score of 1. The 2014 guidelines from the American College of Cardiology, American Heart Association, and Heart Rhythm Society10 suggest that patients with nonvalvular atrial fibrillation and a CHA2DS2-VASc score of 1 have three options: an oral anticoagulant, aspirin, and no antithrombotic therapy. If such a patient on anticoagulant therapy subsequently experiences a major gastrointestinal hemorrhage requiring transfusion and intensive care and no definitively treatable source of bleeding is found on endoscopy, one can argue that the risks of continued anticoagulation (recurrent bleeding) now exceed the benefits and that the patient would be better served by aspirin or even no antithrombotic therapy.
- After 6 months of anticoagulation for unprovoked deep vein thrombosis. Several studies showed that aspirin reduced the risk of recurrent venous thromboembolism in patients who completed an initial 6-month course of anticoagulation.12–15 Though these studies did not specifically compare aspirin with warfarin or target-specific oral anticoagulants in preventing recurrent venous thromboembolism after a hemorrhage, it is reasonable to extrapolate their results to this situation.
If the risk of recurrent hemorrhage on anticoagulation is considered to be too great, then aspirin is an alternative to no anticoagulation, as it reduces the risk of recurrent venous thromboembolism.16 However, we advise caution if the bleeding lesion may be specifically exacerbated by aspirin, particularly upper gastrointestinal ulcers.
Moderate-risk indications
- After a partial course of anticoagulation for provoked venous thromboembolism. Suppose a patient in the 10th week of a planned 12-week course of anticoagulation for a surgically provoked, first deep vein thrombosis presents with abdominal pain and is found to have a retroperitoneal hematoma. In light of the risk of recurrent bleeding vs the benefit of resuming anticoagulation for the limited remaining period, her 12-week treatment course can reasonably be shortened to 10 weeks.
The risk of recurrent venous thromboembolism when a patient is off anticoagulation decreases with time from the initial event. The highest risk, estimated at 0.3% to 1.3% per day, is in the first 4 weeks, falling to 0.03% to 0.2% per day in weeks 5 through 12, and 0.05% per day thereafter.17–20
Additionally, a pooled analysis of seven randomized trials suggests that patients with isolated, distal deep vein thrombosis provoked by a temporary risk factor did not have a high risk of recurrence after being treated for 4 to 6 weeks.21 These analyses are based on vitamin K antagonists, though it seems reasonable to extrapolate this information to the target-specific oral anticoagulants.
More challenging are situations in which the evidence supporting the initial or continued need for anticoagulation is less robust, such as in heart failure, pulmonary hypertension, or splanchnic and hepatic vein thrombosis. In these cases, the lack of strong evidence supporting the use of anticoagulation should make us hesitate to resume it after bleeding.
WHAT WAS THE CLINICAL IMPACT? WHAT IS THE RISK OF REBLEEDING?
Different groups have defined major and minor bleeding in different ways.22,23 Several have proposed criteria to standardize how bleeding events (on warfarin and otherwise) are classified,23–25 but the definitions differ.
Specifically, all agree that a “major” bleeding event is one that is fatal, involves bleeding into a major organ, or leads to a substantial decline in hemoglobin level. However, the Thrombolysis in Myocardial Infarction trials use a decline of more than 5 g/dL in their definition,23,25 while the International Society on Thrombosis and Haemostasis uses 2 g/dL.24
Here, we review the clinical impact of the most common sources of anticoagulation-related hemorrhage—gastrointestinal, soft tissue, and urinary tract26—as well as intracerebral hemorrhage, a less common but more uniformly devastating event.27
Clinical impact of gastrointestinal hemorrhage
Each year, about 4.5% of patients taking warfarin have a gastrointestinal hemorrhage, though not all of these events are major.28 Evolving data suggest that the newer agents (particularly dabigatran, rivaroxaban, and edoxaban) pose a higher risk of gastrointestinal bleeding than warfarin.29 Patients may need plasma and blood transfusions and intravenous phytonadione, all of which carry risks, albeit small.
Frequently, endoscopy is needed to find the source of bleeding and to control it. If this does not work, angiographic intervention to infuse vasoconstrictors or embolic coils into the culprit artery may be required, and some patients need surgery. Each intervention carries its own risk.
Clinical impact of soft-tissue hemorrhage
Soft-tissue hemorrhage accounts for more than 20% of warfarin-related bleeding events26; as yet, we know of no data on the rate with the new drugs. Soft-tissue hemorrhage is often localized to the large muscles of the retroperitoneum and legs. Though retroperitoneal hemorrhage accounts for a relatively small portion of soft-tissue hemorrhages, it is associated with high rates of morbidity and death and will therefore be our focus.26
Much of the clinical impact of retroperitoneal hemorrhage is from a mass effect that causes abdominal compartment syndrome, hydroureter, ileus, abscess formation, and acute and chronic pain. At least 20% of cases are associated with femoral neuropathy. It can also lead to deep vein thrombosis from venous compression, coupled with hypercoagulability in response to bleeding. Brisk bleeding can lead to shock and death, and the mortality rate in retroperitoneal hemorrhage is estimated at 20% or higher.30
In many cases, the retroperitoneal hemorrhage will self-tamponade and the blood will be reabsorbed once the bleeding has stopped, but uncontrolled bleeding may require surgical or angiographic intervention.30
Clinical impact of urinary tract hemorrhage
Gross or microscopic hematuria can be found in an estimated 2% to 24% of patients taking warfarin31–33; data are lacking for the target-specific oral anticoagulants. Interventions required to manage urinary tract bleeding include bladder irrigation and, less often, transfusion.31 Since a significant number of cases of hematuria are due to neoplastic disease,32 a diagnostic workup with radiographic imaging of the upper tract and cystoscopy of the lower tract is usually required.31 While life-threatening hemorrhage is uncommon, complications such as transient urinary obstruction from clots may occur.
Clinical impact of intracranial hemorrhage
Intracranial hemorrhage is the most feared and deadly of the bleeding complications of anticoagulation. The incidence in patients on warfarin is estimated at 2% to 3% per year, which is markedly higher than the estimated incidence of 25 per 100,000 person-years in the general population.34 Emerging data indicate that the newer drugs are also associated with a risk of intracranial hemorrhage, though the risk is about half that with vitamin K antagonists.35 Intracranial hemorrhage leads to death or disability in 76% of cases, compared with 3% of cases of bleeding from the gastrointestinal or urinary tract.27
Regardless of the source of bleeding, hospitalization is likely to be required and may be prolonged, with attendant risks of nosocomial harms such as infection.
Risk of rebleeding
Given the scope and severity of anticoagulation-related bleeding, there is strong interest in predicting and preventing it. By some estimates, the incidence of recurrent bleeding after resuming vitamin K antagonists is 8% to 13%.22 Although there are several indices for predicting the risk of major bleeding when starting anticoagulation, there are currently no validated tools to estimate a patient’s risk of rebleeding.36
The patient factor that most consistently predicts major bleeding is a history of bleeding, particularly from the gastrointestinal tract. Finding and controlling the source of bleeding is important.26,37 For example, a patient with gross hematuria who is found on cystoscopy to have a urothelial papilloma is unlikely to have rebleeding if the tumor is successfully resected and serial follow-up shows no regrowth. In contrast, consider a patient with a major gastrointestinal hemorrhage, the source of which remains elusive after upper, lower, and capsule endoscopy or, alternatively, is suspected to be from one of multiple angiodysplastic lesions. Without definitive source management, this patient faces a high risk of rebleeding.
With or without anticoagulation, after a first intracranial hemorrhage the risk of another one is estimated at 2% to 4% per year.34 An observational study found a recurrence rate of 7.5% when vitamin K antagonist therapy was started after an intracranial hemorrhage (though not all patients were on a vitamin K antagonist at the time of the first hemorrhage).38
Patients with lobar hemorrhage and those with suspected cerebral amyloid angiopathy may be at particularly high risk if anticoagulation is resumed. Conversely, initial events attributed to uncontrolled hypertension that subsequently can be well controlled may portend a lower risk of rebleeding.34 For other types of intracranial hemorrhage, recurrence rates can be even higher. Irrespective of anticoagulation, one prospective study estimated the crude annual rebleeding rate with untreated arteriovenous malformations to be 7%.39 In chronic subdural hematoma, the recurrence rate after initial drainage has been estimated at 9.2% to 26.5%, with use of anticoagulants (in this case, vitamin K antagonists) being an independent predictor of recurrence.40
WHAT OTHER PATIENT FACTORS NEED CONSIDERATION?
Target INR on warfarin
An important factor influencing the risk of bleeding with warfarin is the intensity of this therapy.37 A meta-analysis41 found that the risks of major hemorrhage and thromboembolism are minimized if the goal international normalized ratio (INR) is 2.0 to 3.0. When considering resuming anticoagulation after bleeding, make sure the therapeutic target is appropriate.37
Table 3 summarizes recommended therapeutic ranges for frequently encountered indications for warfarin.36,42,43
INR at time of the event and challenges in controlling it
The decision to resume anticoagulation in patients who bled while using warfarin must take into account the actual INR at the time of the event.
For example, consider a patient whose INR values are consistently in the therapeutic range. While on vacation, he receives ciprofloxacin for acute prostatitis from an urgent care team, and no adjustment to INR monitoring or warfarin dose is made. Several days later, he presents with lower gastrointestinal bleeding. His INR is 8, and colonoscopy reveals diverticulosis with a bleeding vessel, responsive to endoscopic therapy. After controlling the source of bleeding and reinforcing the need to always review new medications for potential interactions with anticoagulation, it is reasonable to expect that he once again will be able to keep his INR in the therapeutic range.
A patient on anticoagulation for the same indication but who has a history of repeated supratherapeutic levels, poor adherence, or poor access to INR monitoring poses very different concerns about resuming anticoagulation (as well as which agent to use, as we discuss below).
Of note, a high INR alone does not explain bleeding. It is estimated that a workup for gastrointestinal bleeding and gross hematuria uncovers previously undetected lesions in approximately one-third of cases involving warfarin.26 A similar malignancy-unmasking effect is now recognized in patients using the target-specific oral agents who experience gastrointestinal bleeding.44 Accordingly, we recommend a comprehensive source evaluation for any anticoagulation-related hemorrhage.
Comorbid conditions
Comorbid conditions associated with bleeding include cancer, end-stage renal disease, liver disease, arterial hypertension, prior stroke, and alcohol abuse.37,45 Gait instability, regardless of cause, may also increase the risk of trauma-related hemorrhage, but some have estimated that a patient would need to fall multiple times per week to contraindicate anticoagulation on the basis of falls alone.46
Concurrent medications
Concomitant therapies, including antiplatelet drugs and nonsteroidal anti-inflammatory drugs, increase bleeding risk.47,48 Aspirin and the nonsteroidals, in addition to having antiplatelet effects, also can cause gastric erosion.37 In evaluating whether and when to restart anticoagulation, it is advisable to review the role that concomitant therapies may have had in the index bleeding event and to evaluate the risks and benefits of these other agents.
Additionally, warfarin has many interactions. Although the newer drugs are lauded for having fewer interactions, they are not completely free of them, and the potential for interactions must always be reviewed.49 Further, unlike warfarin therapy, therapy with the newer agents is not routinely monitored with laboratory tests, so toxicity (or underdosing) may not be recognized until an adverse clinical event occurs. Ultimately, it may be safer to resume anticoagulation after a contributing drug can be safely discontinued.
Advanced age
The influence that the patient’s age should have on the decision to restart anticoagulation is unclear. Although the risk of intracranial hemorrhage increases with age, particularly after age 80, limited data exist in this population, particularly with regard to rebleeding. Further, age is a major risk factor for most thrombotic events, including venous thromboembolism and stroke from atrial fibrillation, so although the risks of anticoagulation may be higher, the benefits may also be higher than in younger patients.37,46 We discourage using age alone as a reason to withhold anticoagulation after a hemorrhage.
HOW LONG SHOULD WE WAIT TO RESTART ANTICOAGULATION?
We lack conclusive data on how long to wait to restart anticoagulation after an anticoagulation-associated hemorrhage.
The decision is complicated by evidence suggesting a rebound effect, with an increased risk of pulmonary embolism and atrial fibrillation-related stroke during the first 90 days of interruption of therapy with warfarin as well as with target-specific oral anticoagulants.3–8 In anticoagulation-associated retroperitoneal bleeding, there is increased risk of deep vein thrombosis from compression, even if venous thromboembolism was not the initial indication for anticoagulation.30
In patients with intracranial hemorrhage, evidence suggests that the intracranial hemorrhage itself increases the risk of arterial and venous thromboembolic events. Irrespective of whether a patient was previously on anticoagulation, the risk of arterial and venous thromboembolic events approaches 7% during the initial intracranial hemorrhage-related hospitalization and 9% during the first 90 days.34,50,51
To date, the only information we have about when to resume anticoagulation comes from patients taking vitamin K antagonists.
Timing after gastrointestinal bleeding
Small case series suggest that in the first 2 months after warfarin-associated gastrointestinal bleeding, there is substantial risk of rebleeding when anticoagulation is resumed—and of thrombosis when it is not.52,53 Two retrospective cohort studies may provide some guidance in this dilemma.28,54
Witt et al28 followed 442 patients who presented with gastrointestinal bleeding from any site during warfarin therapy for varied indications for up to 90 days after the index bleeding event. The risk of death was three times lower in patients who restarted warfarin than in those who did not, and their rate of thrombotic events was 10 times lower. The risk of recurrent gastrointestinal bleeding was statistically insignificant, and there were no fatal bleeding events. Anticoagulant therapy was generally resumed within 1 week of the bleeding event, at a median of 4 days.28,55
Qureshi et al54 performed a retrospective cohort study of 1,329 patients with nonvalvular atrial fibrillation who had experienced a gastrointestinal hemorrhage while taking warfarin. They found that resuming warfarin after 7 days was not associated with a higher risk of recurrent gastrointestinal bleeding and that the rates of death and thromboembolism were lower than in patients who resumed warfarin after 30 days. On the other hand, the risk of recurrent gastrointestinal bleeding was significantly greater if therapy was resumed within the first week.
In view of these studies, we believe that most patients should resume anticoagulation after 4 to 7 days of interruption after gastrointestinal bleeding.55
Timing after soft-tissue hemorrhage
The literature on resuming anticoagulation after soft-tissue hemorrhage is sparse. A retrospective study52 looked at this question in patients with spontaneous rectal sheath hematoma who had been receiving antiplatelet drugs, intravenous heparin, vitamin K antagonists, or a combination of these, but not target-specific agents. More than half of the patients were on vitamin K antagonists at the time of hemorrhage. Analysis suggested that when benefits of resuming anticoagulation are believed to outweigh risks, it is reasonable to resume anticoagulation 4 days after the index event.56
Timing after intracranial hemorrhage
Anticoagulation should not be considered within the first 24 hours after intracranial hemorrhage, as over 70% of patients develop some amount of hematoma expansion during this time.34,57 The period thereafter poses a challenge, as the risk of hematoma expansion decreases while the risk of arterial and venous thromboembolism is ongoing and cumulative.50
Perhaps surprisingly, national guidelines suggest starting prophylactic-dosed anticoagulation early in all intracranial hemorrhage patients, including those not previously on warfarin.58,59 In a randomized trial, Boeer et al60 concluded that starting low-dose subcutaneous heparin the day after an intracranial hemorrhage decreased the risk of thromboembolism without increasing the risk of rebleeding.60 Dickmann et al61 similarly concluded that there was no increased risk of rebleeding with early prophylactic-dosed subcutaneous heparin.61 Optimal mechanical thromboprophylaxis, including graduated compression stockings and intermittent pneumatic compression stockings, is also encouraged.34
Expert opinion remains divided on when and if anticoagulants should be resumed.34,62 The American Heart Association suggests that in nonvalvular atrial fibrillation, long-term anticoagulation should be avoided after spontaneous lobar hemorrhage; antiplatelet agents can be considered instead.58 In nonlobar hemorrhage, the American Heart Association suggests that anticoagulation be considered, depending on strength of indication, 7 to 10 days after the onset.58 The European Stroke Initiative suggests patients with strong indications for anticoagulation be restarted on warfarin 10 to 14 days after the event, depending on the risk of thromboembolism and recurrent intracranial hemorrhage.59 Others suggest delaying resumption to 10 to 30 weeks after an index intracranial hemorrhage.63
Overall, in the immediate acute period of intracranial hemorrhage, most patients will likely benefit from acute reversal of anticoagulation, followed by institution of prophylactic-dose anticoagulation after the first 24 hours. Going forward, patients who remain at higher risk of a recurrence of anticoagulant-related intracranial hemorrhage (such as those with lobar hemorrhage, suspected cerebral amyloid angiopathy, and other high-risk factors) than of thromboembolic events may be best managed without anticoagulants. Alternatively, patients with deep hemispheric intracranial hemorrhage, hypertension that can be well controlled, and a high risk of serious thromboembolism may experience net benefit from restarting anticoagulation.34
We recommend considering restarting anticoagulation 7 days after the onset of intracranial hemorrhage in patients at high risk of thromboembolism and after at least 14 days for patients at lower risk (Table 2). Discussions with neurologic and neurosurgical consultants should also inform this timing decision.
WOULD A NEWER DRUG BE A BETTER CHOICE?
The emergence of target-specific oral anticoagulants, including factor Xa inhibitors such as rivaroxaban, apixaban, and edoxaban and the direct thrombin inhibitor dabigatran etexilate, presents further challenges in managing anticoagulation after hemorrhage. Table 4 summarizes the current FDA-approved indications.64–67
These newer agents are attractive because, compared with warfarin, they have wider therapeutic windows, faster onset and offset of action, and fewer drug and food interactions.68 A meta-analysis of data available to date suggests that the new drugs, compared with warfarin, show a favorable risk-benefit profile with reductions in stroke, intracranial hemorrhage, and mortality with similar overall major bleeding rates, except for a possible increase in gastrointestinal bleeding.68
However, when managing anticoagulation after a bleeding event, the newer agents are challenging for two reasons: they may be associated with a higher incidence of gastrointestinal bleeding than warfarin, and they lack the typical reversal agents that can be used to manage an acute bleeding event.68,69
In individual studies comparing warfarin with dabigatran,70 rivaroxaban,71 apixaban,72 or edoxaban73 for stroke prevention in patients with atrial fibrillation, there was no significant difference in the rate of major bleeding between dabigatran in its higher dose (150 mg twice a day) or rivaroxaban compared with warfarin.70,71 The risk of major bleeding was actually lower with apixaban72 and edoxaban.73
In regard to specific types of major bleeding, the rate of intracranial hemorrhage was significantly lower with dabigatran, rivaroxaban, apixaban, and edoxaban than with warfarin.35,68–73 Some have proposed that since the brain is high in tissue factor, inhibition of tissue factor-factor VIIa complexes by vitamin K antagonists leaves the brain vulnerable to hemorrhage. Others suggest that the targeted mechanism of target-specific agents, as opposed to the multiple pathways in both the intrinsic and extrinsic coagulation cascade that vitamin K antagonists affect, may explain this difference.35,74,75
However, some studies suggest that rivaroxaban and the higher doses of dabigatran and edoxaban are associated with higher rates of major gastrointestinal bleeding compared with warfarin.69–71,76 But apixaban demonstrated no significant difference in gastrointestinal bleeding, and instead demonstrated rates of gastrointestinal bleeding comparable to that with aspirin for stroke prevention in atrial fibrillation.72
The new oral anticoagulants lack antidotes or reversal agents such as phytonadione and fresh-frozen plasma that are available to manage warfarin-associated bleeding events. Other proposed reversal options for the new agents include activated charcoal (if the drugs were taken recently enough to remain in the gastrointestinal tract) and concentrated clotting factor product, though research is ongoing in regards to the most appropriate use in clinical practice.37,69 Unlike rivaroxaban and apixaban, dabigatran has low plasma protein binding and is dialyzable, which provides another strategy in managing dabigatran-related bleeding.69
Of note, the above bleeding risk calculations relate to the first anticoagulant-related bleeding event, though presumably the same risk comparison across agents may be applicable to rebleeding events. Given the data above, when anticoagulation is to be resumed after an intracranial hemorrhage, the risk of rebleeding, particularly in the form of recurrent intracranial hemorrhage, may be lower if a target-specific oral anticoagulant is used.75 Similarly, when anticoagulation is to be resumed after a gastrointestinal bleeding event, reinitiation with warfarin or apixaban therapy may present the lowest risk of recurrent gastrointestinal rebleeding. In other sources of bleeding, such as retroperitoneal bleeding, we suggest consideration of transitioning to warfarin, given the availability of reversal agents in the event of recurrent bleeding.
Other important drug-specific factors that must be noted when selecting an agent with which to resume anticoagulation after a hemorrhage include the following:
- In patients with significant renal impairment, the choice of agent will be limited to a vitamin K antagonist.77
- A meta-analysis of randomized clinical trials suggests that in the elderly (age 75 and older) target-specific oral anticoagulants did not cause excess bleeding and were associated with at least equal efficacy compared with vitamin K antagonists.78
- Target-specific oral anticoagulants may be beneficial in patients who have challenges in achieving INR targets, as evidence suggests that switching to them is associated with a reduction in bleeding for patients who struggle to maintain an appropriately therapeutic INR.68 On the other hand, if there is concern that a patient may occasionally miss doses of an anticoagulant, given the rapid onset and offset of action of target-specific agents compared with warfarin, a missed dose of a target-specific agent may result in faster dissolution of anticoagulant effect and increased risk of thrombotic events, and lapses in anticoagulation will not be identified by routine drug monitoring.6–8,75 As such, it is vital to have a frank discussion with any patient who has difficulty maintaining therapeutic INRs on warfarin treatment to make sure that he or she is not missing doses.
- If there is no clear and compelling reason to select a particular agent, cost considerations should be taken into account. We have included estimated 30-day pricing for the various agents in Table 4.
- Jaffer AK, Brotman DJ, Bash LD, Mahmood SK, Lott B, White RH. Variations in perioperative warfarin management: outcomes and practice patterns at nine hospitals. Am J Med 2010; 123:141–150.
- Kaatz S, Douketis JD, Zhou H, Gage BF, White RH. Risk of stroke after surgery in patients with and without chronic atrial fibrillation. J Thromb Haemost 2010; 8:884–890.
- Raunsø J, Selmer C, Olesen JB, et al. Increased short-term risk of thrombo-embolism or death after interruption of warfarin treatment in patients with atrial fibrillation. Eur Heart J 2012; 33:1886–1892.
- Xarelto (rivaroxaban). Highlights of prescribing information. Jansen Pharmaceuticals, Inc. www.xareltohcp.com/sites/default/files/pdf/xarelto_0.pdf#zoom=100. Accessed March 9, 2015.
- Pradaxa (dabigatran etexilate mesylate). Highlights of prescribing information. Boehringer Ingelheim Pharmaceuticals, Inc. http://bidocs.boehringer-ingelheim.com/BIWebAccess/ViewServlet.ser?docBase=renetnt&folderPath=/Prescribing%20Information/PIs/Pradaxa/Pradaxa.pdf. Accessed March 9, 2015.
- Eliquis (apixaban). Highlights of prescribing information. Bristol-Myers Squibb Company. http://packageinserts.bms.com/pi/pi_eliquis.pdf. Accessed March 9, 2015.
- Schulman S, Beyth RJ, Kearon C, Levine MN; American College of Chest Physicians. Hemorrhagic complications of anticoagulant and thrombolytic treatment: American College of Chest Physicians Evidence-Based Clinical Practice Guidelines (8th ed). Chest 2008; 133(suppl 6):257S–298S.
- Siegal DM, Garcia DA, Crowther MA. How I treat target-specific oral anticoagulant-associated bleeding. Blood 2014; 123:1152–1158.
- Douketis JD, Spyropoulos AC, Spencer FA, et al; American College of Chest Physicians. Perioperative management of antithrombotic therapy: Antithrombotic Therapy and Prevention of Thrombosis, 9th ed: American College of Chest Physicians Evidence-Based Clinical Practice Guidelines. Chest 2012; 141(suppl 2):e326S–e350S.
- January CT, Wann LS, Alpert JS, et al. 2014 AHA/ACC/HRS Guideline for the management of patients with atrial fibrillation: a report of the American College of Cardiology/American Heart Association Task Force on Practice Guidelines and the Heart Rhythm Society. J Am Coll Cardiol 2014; 64:e1–e76.
- Cannegieter SC, Rosendaal FR, Briët E. Thromboembolic and bleeding complications in patients with mechanical heart valve prostheses. Circulation 1994; 89:635–641.
- Warkentin TE. Aspirin for dual prevention of venous and arterial thrombosis. N Engl J Med 2012; 367:2039–2041.
- Simes J, Becattini C, Agnelli G, et al; INSPIRE Study Investigators* (International Collaboration of Aspirin Trials for Recurrent Venous Thromboembolism). Aspirin for the Prevention of Recurrent Venous Thromboembolism: The INSPIRE Collaboration. Circulation 2014; 130:1062–1071.
- Becattini C, Agnelli G, Schenone A, et al; WARFASA Investigators. Aspirin for preventing the recurrence of venous thromboembolism. N Engl J Med 2012; 366:1959–1967.
- Brighton TA, Eikelboom JW, Mann K, et al; ASPIRE Investigators. Low-dose aspirin for preventing recurrent venous thromboembolism. N Engl J Med 2012; 367:1979–1987.
- Wakefield TW, Obi AT, Henke PK. An aspirin a day to keep the clots away: can aspirin prevent recurrent thrombosis in extended treatment for venous thromboembolism? Circulation 2014; 130:1031–1033.
- Kearon C, Hirsh J. Management of anticoagulation before and after elective surgery. N Engl J Med 1997; 336:1506–1511.
- Coon WW, Willis PW 3rd. Recurrence of venous thromboembolism. Surgery 1973; 73:823–827.
- Hull R, Delmore T, Genton E, et al. Warfarin sodium versus low-dose heparin in the long-term treatment of venous thrombosis. N Engl J Med 1979; 301:855–858.
- Jaffer AK, Brotman DJ, Chukwumerije N. When patients on warfarin need surgery. Cleve Clin J Med 2003; 70:973–984.
- Boutitie F, Pinede L, Schulman S, et al. Influence of preceding length of anticoagulant treatment and initial presentation of venous thromboembolism on risk of recurrence after stopping treatment: analysis of individual participants’ data from seven trials. BMJ 2011; 342:d3036.
- Guerrouij M, Uppal CS, Alklabi A, Douketis JD. The clinical impact of bleeding during oral anticoagulant therapy: assessment of morbidity, mortality and post-bleed anticoagulant management. J Thromb Thrombolysis 2011; 31:419–423.
- Mehran R, Rao SV, Bhatt DL, et al. Standardized bleeding definitions for cardiovascular clinical trials: a consensus report from the Bleeding Academic Research Consortium. Circulation 2011; 123:2736–2747.
- Schulman S, Kearon C; Subcommittee on Control of Anticoagulation of the Scientific and Standardization Committee of the International Society on Thrombosis and Haemostasis. Definition of major bleeding in clinical investigations of antihemostatic medicinal products in non-surgical patients. J Thromb Haemost 2005; 3:692–694.
- Wiviott SD, Antman EM, Gibson CM, et al; TRITON-TIMI 38 Investigators. Evaluation of prasugrel compared with clopidogrel in patients with acute coronary syndromes: design and rationale for the TRial to assess Improvement in Therapeutic Outcomes by optimizing platelet InhibitioN with prasugrel Thrombolysis In Myocardial Infarction 38 (TRITON-TIMI 38). Am Heart J 2006; 152:627–635.
- Landefeld CS, Beyth RJ. Anticoagulant-related bleeding: clinical epidemiology, prediction, and prevention. Am J Med 1993; 95:315–328.
- Fang MC, Go AS, Chang Y, et al. Death and disability from warfarin-associated intracranial and extracranial hemorrhages. Am J Med 2007; 120:700–705.
- Witt DM, Delate T, Garcia DA, et al. Risk of thromboembolism, recurrent hemorrhage, and death after warfarin therapy interruption for gastrointestinal tract bleeding. Arch Intern Med 2012; 172:1484–1491.
- Holster IL, Valkhoff VE, Kuipers EJ, Tjwa ET. New oral anticoagulants increase risk for gastrointestinal bleeding: a systematic review and meta-analysis. Gastroenterology 2013; 145:105-112.e15.
- Loor G, Bassiouny H, Valentin C, Shao MY, Funaki B, Desai T. Local and systemic consequences of large retroperitoneal clot burdens. World J Surg 2009; 33:1618–1625.
- Satasivam P, Reeves F, Lin M, et al. The effect of oral anticoagulation on the prevalence and management of haematuria in a contemporary Australian patient cohort. BJU Int 2012; 110(suppl 4):80–84.
- Van Savage JG, Fried FA. Anticoagulant associated hematuria: a prospective study. J Urol 1995; 153:1594–1596.
- Mosley DH, Schatz IJ, Breneman GM, Keyes JW. Long-term anticoagulant therapy. Complications and control in a review of 978 cases. JAMA 1963; 186:914–916.
- Goldstein JN, Greenberg SM. Should anticoagulation be resumed after intracerebral hemorrhage? Cleve Clin J Med 2010; 77:791–799.
- Caldeira D, Barra M, Pinto FJ, Ferreira JJ, Costa J. Intracranial hemorrhage risk with the new oral anticoagulants: a systematic review and meta-analysis. J Neurol 2014 Aug 14. [Epub ahead of print]
- Holbrook A, Schulman S, Witt DM, et al; American College of Chest Physicians. Evidence-based management of anticoagulant therapy: Antithrombotic Therapy and Prevention of Thrombosis, 9th ed: American College of Chest Physicians Evidence-Based Clinical Practice Guidelines. Chest 2012; 141(suppl 2):e152S–e184S.
- Ageno W, Gallus AS, Wittkowsky A, Crowther M, Hylek EM, Palareti G; American College of Chest Physicians. Oral anticoagulant therapy: Antithrombotic Therapy and Prevention of Thrombosis, 9th ed: American College of Chest Physicians Evidence-Based Clinical Practice Guidelines. Chest 2012; 141(suppl 2):e44S–e88S.
- Poli D, Antonucci E, Dentali F, et al; Italian Federation of Anticoagulation Clinics (FCSA). Recurrence of ICH after resumption of anticoagulation with VK antagonists: CHIRONE study. Neurology 2014; 82:1020–1026.
- Choi JH, Mast H, Sciacca RR, et al. Clinical outcome after first and recurrent hemorrhage in patients with untreated brain arteriovenous malformation. Stroke 2006; 37:1243–1247.
- Chon KH, Lee JM, Koh EJ, Choi HY. Independent predictors for recurrence of chronic subdural hematoma. Acta Neurochir (Wien) 2012; 154:1541–1548.
- Oake N, Jennings A, Forster AJ, et al. Anticoagulation intensity and outcomes among patients prescribed oral anticoagulant therapy: a systematic review and meta-analysis. CMAJ 2008; 179:235–244.
- Whitlock RP, Sun JC, Fremes SE, Rubens FD, Teoh KH; American College of Chest Physicians. Antithrombotic and thrombolytic therapy for valvular disease: Antithrombotic Therapy and Prevention of Thrombosis, 9th ed: American College of Chest Physicians Evidence-Based Clinical Practice Guidelines. Chest 2012; 141(suppl 2):e576S–e600S.
- Bonow RO, Carabello BA, Chatterjee K, et al; 2006 Writing Committee Members; American College of Cardiology/American Heart Association Task Force. 2008 Focused update incorporated into the ACC/AHA 2006 guidelines for the management of patients with valvular heart disease. Circulation 2008; 118:e523–e661.
- Clemens A, Strack A, Noack H, Konstantinides S, Brueckmann M, Lip GY. Anticoagulant-related gastrointestinal bleeding—could this facilitate early detection of benign or malignant gastrointestinal lesions? Ann Med 2014; 46:672–678.
- Khalid F, Qureshi W, Qureshi S, Alirhayim Z, Garikapati K, Patsias I. Impact of restarting warfarin therapy in renal disease anticoagulated patients with gastrointestinal hemorrhage. Ren Fail 2013; 35:1228–1235.
- Man-Son-Hing M, Nichol G, Lau A, Laupacis A. Choosing antithrombotic therapy for elderly patients with atrial fibrillation who are at risk for falls. Arch Intern Med 1999; 159:677–685.
- Davidson BL, Verheijen S, Lensing AW, et al. Bleeding risk of patients with acute venous thromboembolism taking nonsteroidal anti-inflammatory drugs or aspirin. JAMA Intern Med 2014; 174:947–953.
- Knijff-Dutmer EA, Schut GA, van de Laar MA. Concomitant coumarin-NSAID therapy and risk for bleeding. Ann Pharmacother 2003; 37:12–16.
- Heidbuchel H, Verhamme P, Alings M, et al; European Heart Rhythm Association. European Heart Rhythm Association Practical Guide on the use of new oral anticoagulants in patients with non-valvular atrial fibrillation. Europace 2013; 15:625–651.
- Goldstein JN, Fazen LE, Wendell L, et al. Risk of thromboembolism following acute intracerebral hemorrhage. Neurocrit Care 2009; 10:28–34.
- Christensen MC, Dawson J, Vincent C. Risk of thromboembolic complications after intracerebral hemorrhage according to ethnicity. Adv Ther 2008; 25:831–841.
- Ananthasubramaniam K, Beattie JN, Rosman HS, Jayam V, Borzak S. How safely and for how long can warfarin therapy be withheld in prosthetic heart valve patients hospitalized with a major hemorrhage? Chest 2001; 119:478–484.
- Lee JK, Kang HW, Kim SG, Kim JS, Jung HC. Risks related with withholding and resuming anticoagulation in patients with non-variceal upper gastrointestinal bleeding while on warfarin therapy. Int J Clin Pract 2012; 66:64–68.
- Qureshi W, Mittal C, Patsias I, et al. Restarting anticoagulation and outcomes after major gastrointestinal bleeding in atrial fibrillation. Am J Cardiol 2014; 113:662–668.
- Brotman DJ, Jaffer AK. Resuming anticoagulation in the first week following gastrointestinal tract hemorrhage: should we adopt a 4-day rule? Arch Intern Med 2012; 172:1492–1493.
- Kunkala MR1, Kehl J, Zielinski MD. Spontaneous rectus sheath hematomas: when to restart anticoagulation? World J Surg 2013; 37:2555–2559.
- Davis SM, Broderick J, Hennerici M, et al; Recombinant Activated Factor VII Intracerebral Hemorrhage Trial Investigators. Hematoma growth is a determinant of mortality and poor outcome after intracerebral hemorrhage. Neurology 2006; 66:1175–1181.
- Broderick J, Connolly S, Feldmann E, et al; American Heart Association; American Stroke Association Stroke Council; High Blood Pressure Research Council; Quality of Care and Outcomes in Research Interdisciplinary Working Group. Guidelines for the management of spontaneous intracerebral hemorrhage in adults. Stroke 2007; 38:2001–2023.
- Steiner T, Kaste M, Forsting M, et al. Recommendations for the management of intracranial haemorrhage—part I: spontaneous intracerebral haemorrhage. The European Stroke Initiative Writing Committee and the Writing Committee for the EUSI Executive Committee. Cerebrovasc Dis 2006; 22:294–316. Erratum in: Cerebrovasc Dis 2006; 22:461.
- Boeer A, Voth E, Henze T, Prange HW. Early heparin therapy in patients with spontaneous intracerebral haemorrhage. J Neurol Neurosurg Psychiatry 1991; 54:466–467.
- Dickmann U, Voth E, Schicha H, Henze T, Prange H, Emrich D. Heparin therapy, deep-vein thrombosis and pulmonary embolism after intracerebral hemorrhage. Klin Wochenschr 1988; 66:1182–1183.
- Aguilar MI, Hart RG, Kase CS, et al. Treatment of warfarin-associated intracerebral hemorrhage: literature review and expert opinion. Mayo Clin Proc 2007; 82:82–92. Erratum in: Mayo Clin Proc 2007; 82:387.
- Majeed A, Kim YK, Roberts RS, Holmström M, Schulman S. Optimal timing of resumption of warfarin after intracranial hemorrhage. Stroke 2010; 41:2860–2866.
- US Food and Drug Administration. Drug Information. XARELTO (rivaroxaban) tablets, for oral use. www.accessdata.fda.gov/drugsatfda_docs/label/2013/022406s004lbl.pdf. Accessed March 9, 2015.
- US Food and Drug Administration. Drug Information. ELIQUIS® (apixaban) tablets for oral use. www.accessdata.fda.gov/drugsatfda_docs/label/2014/202155s009lbl.pdf. Accessed March 9, 2015.
- US Food and Drug Administration. Drug Information. PRADAXA® (dabigatran etexilate mesylate) capsules for oral use. www.accessdata.fda.gov/drugsatfda_docs/label/2014/022512s023lbl.pdf. Accessed March 9, 2015.
- New oral anticoagulants for acute venous thromboembolism. Med Lett Drugs Ther 2014; 56:3–4.
- Ruff CT, Giugliano RP, Braunwald E, et al. Comparison of the efficacy and safety of new oral anticoagulants with warfarin in patients with atrial fibrillation: a meta-analysis of randomised trials. Lancet 2014; 383:955–962.
- Nutescu EA, Dager WE, Kalus JS, Lewin JJ 3rd, Cipolle MD. Management of bleeding and reversal strategies for oral anticoagulants: clinical practice considerations. Am J Health Syst Pharm 2013; 70:1914–1929.
- Connolly SJ, Ezekowitz MD, Yusuf S, et al; RE-LY Steering Committee and Investigators. Dabigatran versus warfarin in patients with atrial fibrillation. N Engl J Med 2009; 361:1139–1151. Erratum in: N Engl J Med 2010; 363:1877.
- Patel MR, Mahaffey KW, Garg J, et al; ROCKET AF Investigators. Rivaroxaban versus warfarin in nonvalvular atrial fibrillation. N Engl J Med 2011; 365:883–891.
- Granger CB, Alexander JH, McMurray JJ, et al; ARISTOTLE Committees and Investigators. Apixaban versus warfarin in patients with atrial fibrillation. N Engl J Med 2011; 365:981–992.
- Hokusai-VTE Investigators, Büller HR, Décousus H, Grosso MA, et al. Edoxaban versus warfarin for the treatment of symptomatic venous thromboembolism. N Engl J Med 2013; 369:1406–1415.
- Mackman N. The role of tissue factor and factor VIIa in hemostasis. Anesth Analg 2009; 108:1447–1452.
- Chatterjee S, Sardar P, Biondi-Zoccai G, Kumbhani DJ. New oral anticoagulants and the risk of intracranial hemorrhage: traditional and Bayesian meta-analysis and mixed treatment comparison of randomized trials of new oral anticoagulants in atrial fibrillation. JAMA Neurol 2013; 70:1486–1490.
- Loffredo L, Perri L, Violi F. Impact of new oral anticoagulants on gastrointestinal bleeding in atrial fibrillation: a meta-analysis of interventional trials. Dig Liver Dis 2015 Feb 7. pii: S1590-8658(15)00189-9. doi: 10.1016/j.dld.2015.01.159. [Epub ahead of print]
- Thachil J. The newer direct oral anticoagulants: a practical guide. Clin Med 2014; 14:165–175.
- Sardar P, Chatterjee S, Chaudhari S, Lip GY. New oral anticoagulants in elderly adults: evidence from a meta-analysis of randomized trials. J Am Geriatr Soc 2014; 62:857–864.
- Jaffer AK, Brotman DJ, Bash LD, Mahmood SK, Lott B, White RH. Variations in perioperative warfarin management: outcomes and practice patterns at nine hospitals. Am J Med 2010; 123:141–150.
- Kaatz S, Douketis JD, Zhou H, Gage BF, White RH. Risk of stroke after surgery in patients with and without chronic atrial fibrillation. J Thromb Haemost 2010; 8:884–890.
- Raunsø J, Selmer C, Olesen JB, et al. Increased short-term risk of thrombo-embolism or death after interruption of warfarin treatment in patients with atrial fibrillation. Eur Heart J 2012; 33:1886–1892.
- Xarelto (rivaroxaban). Highlights of prescribing information. Jansen Pharmaceuticals, Inc. www.xareltohcp.com/sites/default/files/pdf/xarelto_0.pdf#zoom=100. Accessed March 9, 2015.
- Pradaxa (dabigatran etexilate mesylate). Highlights of prescribing information. Boehringer Ingelheim Pharmaceuticals, Inc. http://bidocs.boehringer-ingelheim.com/BIWebAccess/ViewServlet.ser?docBase=renetnt&folderPath=/Prescribing%20Information/PIs/Pradaxa/Pradaxa.pdf. Accessed March 9, 2015.
- Eliquis (apixaban). Highlights of prescribing information. Bristol-Myers Squibb Company. http://packageinserts.bms.com/pi/pi_eliquis.pdf. Accessed March 9, 2015.
- Schulman S, Beyth RJ, Kearon C, Levine MN; American College of Chest Physicians. Hemorrhagic complications of anticoagulant and thrombolytic treatment: American College of Chest Physicians Evidence-Based Clinical Practice Guidelines (8th ed). Chest 2008; 133(suppl 6):257S–298S.
- Siegal DM, Garcia DA, Crowther MA. How I treat target-specific oral anticoagulant-associated bleeding. Blood 2014; 123:1152–1158.
- Douketis JD, Spyropoulos AC, Spencer FA, et al; American College of Chest Physicians. Perioperative management of antithrombotic therapy: Antithrombotic Therapy and Prevention of Thrombosis, 9th ed: American College of Chest Physicians Evidence-Based Clinical Practice Guidelines. Chest 2012; 141(suppl 2):e326S–e350S.
- January CT, Wann LS, Alpert JS, et al. 2014 AHA/ACC/HRS Guideline for the management of patients with atrial fibrillation: a report of the American College of Cardiology/American Heart Association Task Force on Practice Guidelines and the Heart Rhythm Society. J Am Coll Cardiol 2014; 64:e1–e76.
- Cannegieter SC, Rosendaal FR, Briët E. Thromboembolic and bleeding complications in patients with mechanical heart valve prostheses. Circulation 1994; 89:635–641.
- Warkentin TE. Aspirin for dual prevention of venous and arterial thrombosis. N Engl J Med 2012; 367:2039–2041.
- Simes J, Becattini C, Agnelli G, et al; INSPIRE Study Investigators* (International Collaboration of Aspirin Trials for Recurrent Venous Thromboembolism). Aspirin for the Prevention of Recurrent Venous Thromboembolism: The INSPIRE Collaboration. Circulation 2014; 130:1062–1071.
- Becattini C, Agnelli G, Schenone A, et al; WARFASA Investigators. Aspirin for preventing the recurrence of venous thromboembolism. N Engl J Med 2012; 366:1959–1967.
- Brighton TA, Eikelboom JW, Mann K, et al; ASPIRE Investigators. Low-dose aspirin for preventing recurrent venous thromboembolism. N Engl J Med 2012; 367:1979–1987.
- Wakefield TW, Obi AT, Henke PK. An aspirin a day to keep the clots away: can aspirin prevent recurrent thrombosis in extended treatment for venous thromboembolism? Circulation 2014; 130:1031–1033.
- Kearon C, Hirsh J. Management of anticoagulation before and after elective surgery. N Engl J Med 1997; 336:1506–1511.
- Coon WW, Willis PW 3rd. Recurrence of venous thromboembolism. Surgery 1973; 73:823–827.
- Hull R, Delmore T, Genton E, et al. Warfarin sodium versus low-dose heparin in the long-term treatment of venous thrombosis. N Engl J Med 1979; 301:855–858.
- Jaffer AK, Brotman DJ, Chukwumerije N. When patients on warfarin need surgery. Cleve Clin J Med 2003; 70:973–984.
- Boutitie F, Pinede L, Schulman S, et al. Influence of preceding length of anticoagulant treatment and initial presentation of venous thromboembolism on risk of recurrence after stopping treatment: analysis of individual participants’ data from seven trials. BMJ 2011; 342:d3036.
- Guerrouij M, Uppal CS, Alklabi A, Douketis JD. The clinical impact of bleeding during oral anticoagulant therapy: assessment of morbidity, mortality and post-bleed anticoagulant management. J Thromb Thrombolysis 2011; 31:419–423.
- Mehran R, Rao SV, Bhatt DL, et al. Standardized bleeding definitions for cardiovascular clinical trials: a consensus report from the Bleeding Academic Research Consortium. Circulation 2011; 123:2736–2747.
- Schulman S, Kearon C; Subcommittee on Control of Anticoagulation of the Scientific and Standardization Committee of the International Society on Thrombosis and Haemostasis. Definition of major bleeding in clinical investigations of antihemostatic medicinal products in non-surgical patients. J Thromb Haemost 2005; 3:692–694.
- Wiviott SD, Antman EM, Gibson CM, et al; TRITON-TIMI 38 Investigators. Evaluation of prasugrel compared with clopidogrel in patients with acute coronary syndromes: design and rationale for the TRial to assess Improvement in Therapeutic Outcomes by optimizing platelet InhibitioN with prasugrel Thrombolysis In Myocardial Infarction 38 (TRITON-TIMI 38). Am Heart J 2006; 152:627–635.
- Landefeld CS, Beyth RJ. Anticoagulant-related bleeding: clinical epidemiology, prediction, and prevention. Am J Med 1993; 95:315–328.
- Fang MC, Go AS, Chang Y, et al. Death and disability from warfarin-associated intracranial and extracranial hemorrhages. Am J Med 2007; 120:700–705.
- Witt DM, Delate T, Garcia DA, et al. Risk of thromboembolism, recurrent hemorrhage, and death after warfarin therapy interruption for gastrointestinal tract bleeding. Arch Intern Med 2012; 172:1484–1491.
- Holster IL, Valkhoff VE, Kuipers EJ, Tjwa ET. New oral anticoagulants increase risk for gastrointestinal bleeding: a systematic review and meta-analysis. Gastroenterology 2013; 145:105-112.e15.
- Loor G, Bassiouny H, Valentin C, Shao MY, Funaki B, Desai T. Local and systemic consequences of large retroperitoneal clot burdens. World J Surg 2009; 33:1618–1625.
- Satasivam P, Reeves F, Lin M, et al. The effect of oral anticoagulation on the prevalence and management of haematuria in a contemporary Australian patient cohort. BJU Int 2012; 110(suppl 4):80–84.
- Van Savage JG, Fried FA. Anticoagulant associated hematuria: a prospective study. J Urol 1995; 153:1594–1596.
- Mosley DH, Schatz IJ, Breneman GM, Keyes JW. Long-term anticoagulant therapy. Complications and control in a review of 978 cases. JAMA 1963; 186:914–916.
- Goldstein JN, Greenberg SM. Should anticoagulation be resumed after intracerebral hemorrhage? Cleve Clin J Med 2010; 77:791–799.
- Caldeira D, Barra M, Pinto FJ, Ferreira JJ, Costa J. Intracranial hemorrhage risk with the new oral anticoagulants: a systematic review and meta-analysis. J Neurol 2014 Aug 14. [Epub ahead of print]
- Holbrook A, Schulman S, Witt DM, et al; American College of Chest Physicians. Evidence-based management of anticoagulant therapy: Antithrombotic Therapy and Prevention of Thrombosis, 9th ed: American College of Chest Physicians Evidence-Based Clinical Practice Guidelines. Chest 2012; 141(suppl 2):e152S–e184S.
- Ageno W, Gallus AS, Wittkowsky A, Crowther M, Hylek EM, Palareti G; American College of Chest Physicians. Oral anticoagulant therapy: Antithrombotic Therapy and Prevention of Thrombosis, 9th ed: American College of Chest Physicians Evidence-Based Clinical Practice Guidelines. Chest 2012; 141(suppl 2):e44S–e88S.
- Poli D, Antonucci E, Dentali F, et al; Italian Federation of Anticoagulation Clinics (FCSA). Recurrence of ICH after resumption of anticoagulation with VK antagonists: CHIRONE study. Neurology 2014; 82:1020–1026.
- Choi JH, Mast H, Sciacca RR, et al. Clinical outcome after first and recurrent hemorrhage in patients with untreated brain arteriovenous malformation. Stroke 2006; 37:1243–1247.
- Chon KH, Lee JM, Koh EJ, Choi HY. Independent predictors for recurrence of chronic subdural hematoma. Acta Neurochir (Wien) 2012; 154:1541–1548.
- Oake N, Jennings A, Forster AJ, et al. Anticoagulation intensity and outcomes among patients prescribed oral anticoagulant therapy: a systematic review and meta-analysis. CMAJ 2008; 179:235–244.
- Whitlock RP, Sun JC, Fremes SE, Rubens FD, Teoh KH; American College of Chest Physicians. Antithrombotic and thrombolytic therapy for valvular disease: Antithrombotic Therapy and Prevention of Thrombosis, 9th ed: American College of Chest Physicians Evidence-Based Clinical Practice Guidelines. Chest 2012; 141(suppl 2):e576S–e600S.
- Bonow RO, Carabello BA, Chatterjee K, et al; 2006 Writing Committee Members; American College of Cardiology/American Heart Association Task Force. 2008 Focused update incorporated into the ACC/AHA 2006 guidelines for the management of patients with valvular heart disease. Circulation 2008; 118:e523–e661.
- Clemens A, Strack A, Noack H, Konstantinides S, Brueckmann M, Lip GY. Anticoagulant-related gastrointestinal bleeding—could this facilitate early detection of benign or malignant gastrointestinal lesions? Ann Med 2014; 46:672–678.
- Khalid F, Qureshi W, Qureshi S, Alirhayim Z, Garikapati K, Patsias I. Impact of restarting warfarin therapy in renal disease anticoagulated patients with gastrointestinal hemorrhage. Ren Fail 2013; 35:1228–1235.
- Man-Son-Hing M, Nichol G, Lau A, Laupacis A. Choosing antithrombotic therapy for elderly patients with atrial fibrillation who are at risk for falls. Arch Intern Med 1999; 159:677–685.
- Davidson BL, Verheijen S, Lensing AW, et al. Bleeding risk of patients with acute venous thromboembolism taking nonsteroidal anti-inflammatory drugs or aspirin. JAMA Intern Med 2014; 174:947–953.
- Knijff-Dutmer EA, Schut GA, van de Laar MA. Concomitant coumarin-NSAID therapy and risk for bleeding. Ann Pharmacother 2003; 37:12–16.
- Heidbuchel H, Verhamme P, Alings M, et al; European Heart Rhythm Association. European Heart Rhythm Association Practical Guide on the use of new oral anticoagulants in patients with non-valvular atrial fibrillation. Europace 2013; 15:625–651.
- Goldstein JN, Fazen LE, Wendell L, et al. Risk of thromboembolism following acute intracerebral hemorrhage. Neurocrit Care 2009; 10:28–34.
- Christensen MC, Dawson J, Vincent C. Risk of thromboembolic complications after intracerebral hemorrhage according to ethnicity. Adv Ther 2008; 25:831–841.
- Ananthasubramaniam K, Beattie JN, Rosman HS, Jayam V, Borzak S. How safely and for how long can warfarin therapy be withheld in prosthetic heart valve patients hospitalized with a major hemorrhage? Chest 2001; 119:478–484.
- Lee JK, Kang HW, Kim SG, Kim JS, Jung HC. Risks related with withholding and resuming anticoagulation in patients with non-variceal upper gastrointestinal bleeding while on warfarin therapy. Int J Clin Pract 2012; 66:64–68.
- Qureshi W, Mittal C, Patsias I, et al. Restarting anticoagulation and outcomes after major gastrointestinal bleeding in atrial fibrillation. Am J Cardiol 2014; 113:662–668.
- Brotman DJ, Jaffer AK. Resuming anticoagulation in the first week following gastrointestinal tract hemorrhage: should we adopt a 4-day rule? Arch Intern Med 2012; 172:1492–1493.
- Kunkala MR1, Kehl J, Zielinski MD. Spontaneous rectus sheath hematomas: when to restart anticoagulation? World J Surg 2013; 37:2555–2559.
- Davis SM, Broderick J, Hennerici M, et al; Recombinant Activated Factor VII Intracerebral Hemorrhage Trial Investigators. Hematoma growth is a determinant of mortality and poor outcome after intracerebral hemorrhage. Neurology 2006; 66:1175–1181.
- Broderick J, Connolly S, Feldmann E, et al; American Heart Association; American Stroke Association Stroke Council; High Blood Pressure Research Council; Quality of Care and Outcomes in Research Interdisciplinary Working Group. Guidelines for the management of spontaneous intracerebral hemorrhage in adults. Stroke 2007; 38:2001–2023.
- Steiner T, Kaste M, Forsting M, et al. Recommendations for the management of intracranial haemorrhage—part I: spontaneous intracerebral haemorrhage. The European Stroke Initiative Writing Committee and the Writing Committee for the EUSI Executive Committee. Cerebrovasc Dis 2006; 22:294–316. Erratum in: Cerebrovasc Dis 2006; 22:461.
- Boeer A, Voth E, Henze T, Prange HW. Early heparin therapy in patients with spontaneous intracerebral haemorrhage. J Neurol Neurosurg Psychiatry 1991; 54:466–467.
- Dickmann U, Voth E, Schicha H, Henze T, Prange H, Emrich D. Heparin therapy, deep-vein thrombosis and pulmonary embolism after intracerebral hemorrhage. Klin Wochenschr 1988; 66:1182–1183.
- Aguilar MI, Hart RG, Kase CS, et al. Treatment of warfarin-associated intracerebral hemorrhage: literature review and expert opinion. Mayo Clin Proc 2007; 82:82–92. Erratum in: Mayo Clin Proc 2007; 82:387.
- Majeed A, Kim YK, Roberts RS, Holmström M, Schulman S. Optimal timing of resumption of warfarin after intracranial hemorrhage. Stroke 2010; 41:2860–2866.
- US Food and Drug Administration. Drug Information. XARELTO (rivaroxaban) tablets, for oral use. www.accessdata.fda.gov/drugsatfda_docs/label/2013/022406s004lbl.pdf. Accessed March 9, 2015.
- US Food and Drug Administration. Drug Information. ELIQUIS® (apixaban) tablets for oral use. www.accessdata.fda.gov/drugsatfda_docs/label/2014/202155s009lbl.pdf. Accessed March 9, 2015.
- US Food and Drug Administration. Drug Information. PRADAXA® (dabigatran etexilate mesylate) capsules for oral use. www.accessdata.fda.gov/drugsatfda_docs/label/2014/022512s023lbl.pdf. Accessed March 9, 2015.
- New oral anticoagulants for acute venous thromboembolism. Med Lett Drugs Ther 2014; 56:3–4.
- Ruff CT, Giugliano RP, Braunwald E, et al. Comparison of the efficacy and safety of new oral anticoagulants with warfarin in patients with atrial fibrillation: a meta-analysis of randomised trials. Lancet 2014; 383:955–962.
- Nutescu EA, Dager WE, Kalus JS, Lewin JJ 3rd, Cipolle MD. Management of bleeding and reversal strategies for oral anticoagulants: clinical practice considerations. Am J Health Syst Pharm 2013; 70:1914–1929.
- Connolly SJ, Ezekowitz MD, Yusuf S, et al; RE-LY Steering Committee and Investigators. Dabigatran versus warfarin in patients with atrial fibrillation. N Engl J Med 2009; 361:1139–1151. Erratum in: N Engl J Med 2010; 363:1877.
- Patel MR, Mahaffey KW, Garg J, et al; ROCKET AF Investigators. Rivaroxaban versus warfarin in nonvalvular atrial fibrillation. N Engl J Med 2011; 365:883–891.
- Granger CB, Alexander JH, McMurray JJ, et al; ARISTOTLE Committees and Investigators. Apixaban versus warfarin in patients with atrial fibrillation. N Engl J Med 2011; 365:981–992.
- Hokusai-VTE Investigators, Büller HR, Décousus H, Grosso MA, et al. Edoxaban versus warfarin for the treatment of symptomatic venous thromboembolism. N Engl J Med 2013; 369:1406–1415.
- Mackman N. The role of tissue factor and factor VIIa in hemostasis. Anesth Analg 2009; 108:1447–1452.
- Chatterjee S, Sardar P, Biondi-Zoccai G, Kumbhani DJ. New oral anticoagulants and the risk of intracranial hemorrhage: traditional and Bayesian meta-analysis and mixed treatment comparison of randomized trials of new oral anticoagulants in atrial fibrillation. JAMA Neurol 2013; 70:1486–1490.
- Loffredo L, Perri L, Violi F. Impact of new oral anticoagulants on gastrointestinal bleeding in atrial fibrillation: a meta-analysis of interventional trials. Dig Liver Dis 2015 Feb 7. pii: S1590-8658(15)00189-9. doi: 10.1016/j.dld.2015.01.159. [Epub ahead of print]
- Thachil J. The newer direct oral anticoagulants: a practical guide. Clin Med 2014; 14:165–175.
- Sardar P, Chatterjee S, Chaudhari S, Lip GY. New oral anticoagulants in elderly adults: evidence from a meta-analysis of randomized trials. J Am Geriatr Soc 2014; 62:857–864.
KEY POINTS
- Not all patients on anticoagulation at the time of a bleeding event have a strong indication to continue anticoagulation afterward.
- Important considerations when deciding whether to resume anticoagulation after hemorrhage are whether the source of bleeding has been found and controlled and, if the patient is receiving warfarin, whether he or she can be expected to maintain the target international normalized ratio.
- The newer oral anticoagulants, including factor Xa inhibitors and direct thrombin inhibitors, lack antidotes or reversal agents, and their risk of causing bleeding compared with warfarin varies by site of bleeding.
Hospital Renovation Patient Satisfaction
Hospitals are expensive and complex facilities to build and renovate. It is estimated $200 billion is being spent in the United States during this decade on hospital construction and renovation, and further expenditures in this area are expected.[1] Aging hospital infrastructure, competition, and health system expansion have motivated institutions to invest in renovation and new hospital building construction.[2, 3, 4, 5, 6, 7] There is a trend toward patient‐centered design in new hospital construction. Features of this trend include same‐handed design (ie, rooms on a unit have all beds oriented in the same direction and do not share headwalls); use of sound absorbent materials to reduced ambient noise[7, 8, 9]; rooms with improved view and increased natural lighting to reduce anxiety, decrease delirium, and increase sense of wellbeing[10, 11, 12]; incorporation of natural elements like gardens, water features, and art[12, 13, 14, 15, 16, 17, 18]; single‐patient rooms to reduce transmission of infection and enhance privacy and visitor comfort[7, 19, 20]; presence of comfortable waiting rooms and visitor accommodations to enhance comfort and family participation[21, 22, 23]; and hotel‐like amenities such as on‐demand entertainment and room service menus.[24, 25]
There is a belief among some hospital leaders that patients are generally unable to distinguish their positive experience with a pleasing healthcare environment from their positive experience with care, and thus improving facilities will lead to improved satisfaction across the board.[26, 27] In a controlled study of hospitalized patients, appealing rooms were associated with increased satisfaction with services including housekeeping and food service staff, meals, as well as physicians and overall satisfaction.[26] A 2012 survey of hospital leadership found that expanding and renovating facilities was considered a top priority in improving patient satisfaction, with 82% of the respondents stating that this was important.[27]
Despite these attitudes, the impact of patient‐centered design on patient satisfaction is not well understood. Studies have shown that renovations and hospital construction that incorporates noise reduction strategies, positive distraction, patient and caregiver control, attractive waiting rooms, improved patient room appearance, private rooms, and large windows result in improved satisfaction with nursing, noise level, unit environment and cleanliness, perceived wait time, discharge preparedness, and overall care. [7, 19, 20, 23, 28] However, these studies were limited by small sample size, inclusion of a narrow group of patients (eg, ambulatory, obstetric, geriatric rehabilitation, intensive care unit), and concurrent use of interventions other than design improvement (eg, nurse and patient education). Many of these studies did not use the ubiquitous Hospital Consumer Assessment of Healthcare Providers and Systems (HCAHPS) and Press Ganey patient satisfaction surveys.
We sought to determine the changes in patient satisfaction that occurred during a natural experiment, in which clinical units (comprising stable nursing, physician, and unit teams) were relocated from an historic clinical building to a new clinical building that featured patient‐centered design, using HCAHPS and Press Ganey surveys and a large study population. We hypothesized that new building features would positively impact both facility related (eg, noise level), nonfacility related (eg, physician and housekeeping service related), and overall satisfaction.
METHODS
This was a retrospective analysis of prospectively collected Press Ganey and HCAPHS patient satisfaction survey data for a single academic tertiary care hospital.[29] The research project was reviewed and approved by the institutional review board.
Participants
All patients discharged from 12 clinical units that relocated to the new clinical building and returned patient satisfaction surveys served as study patients. The moved units included the coronary care unit, cardiac step down unit, medical intensive care unit, neuro critical care unit, surgical intensive care unit, orthopedic unit, neurology unit, neurosurgery unit, obstetrics units, gynecology unit, urology unit, cardiothoracic surgery unit, and the transplant surgery and renal transplant unit. Patients on clinical units that did not move served as concurrent controls.
Exposure
Patients admitted to the new clinical building experienced several patient‐centered design features. These features included easy access to healing gardens with a water feature, soaring lobbies, a collection of more than 500 works of art, well‐decorated and light‐filled patient rooms with sleeping accommodations for family members, sound‐absorbing features in patient care corridors ranging from acoustical ceiling tiles to a quiet nurse‐call system, and an interactive television network with Internet, movies, and games. All patients during the baseline period and control patients during the study period were located in typical patient rooms with standard hospital amenities. No other major patient satisfaction interventions were initiated during the pre‐ or postperiod in either arm of the study; ongoing patient satisfaction efforts (such as unit‐based customer care representatives) were deployed broadly and not restricted to the new clinical building. Clinical teams comprised of physicians, nurses, and ancillary staff did not change significantly after the move.
Time Periods
The move to new clinical building occurred on May 1, 2012. After allowing for a 15‐day washout period, the postmove period included Press Ganey and HCAHPS surveys returned for discharges that occurred during a 7.5‐month period between May 15, 2102 and December 31, 2012. Baseline data included Press Ganey and HCAHPS surveys returned for discharges in the preceding 12 months (May 1, 2011 to April 30, 2012). Sensitivity analysis using only 7.5 months of baseline data did not reveal any significant difference when compared with 12‐month baseline data, and we report only data from the 12‐month baseline period.
Instruments
Press Ganey and HCAHPS patient satisfaction surveys were sent via mail in the same envelope. Fifty percent of the discharged patients were randomized to receive the surveys. The Press Ganey survey contained 33 items covering across several subdomains including room, meal, nursing, physician, ancillary staff, visitor, discharge, and overall satisfaction. The HCAHPS survey contained 29 Centers for Medicare and Medicaid Services (CMS)‐mandated items, of which 21 are related to patient satisfaction. The development and testing and methods for administration and reporting of the HCAHPS survey have been previously described.[30, 31] Press Ganey patient satisfaction survey results have been reported in the literature.[32, 33]
Outcome Variables
Press Ganey and HCAHPS patient satisfaction survey responses were the primary outcome variables of the study. The survey items were categorized as facility related (eg, noise level), nonfacility related (eg, physician and nursing staff satisfaction), and overall satisfaction related.
Covariates
Age, sex, length of stay (LOS), insurance type, and all‐payer refined diagnosis‐related groupassociated illness complexity were included as covariates.
Statistical Analysis
Percent top‐box scores were calculated for each survey item as the percent of patients who responded very good for a given item on Press Ganey survey items and always or definitely yes or 9 or 10 on HCAHPS survey items. CMS utilizes percent top‐box scores to calculate payments under the Value Based Purchasing (VBP) program and to report the results publicly. Numerous studies have also reported percent top‐box scores for HCAHPS survey results.[31, 32, 33, 34]
Odds ratios of premove versus postmove percentage of top‐box scores, adjusted for age, sex, LOS, complexity of illness, and insurance type were determined using logistic regression for the units that moved. Similar scores were calculated for unmoved units to detect secular trends. To determine whether the differences between the moved and unmoved units were significant, we introduced the interaction term (moved vs unmoved unit status) (pre‐ vs postmove time period) into the logistic regression models and examined the adjusted P value for this term. All statistical analysis was performed using SAS Institute Inc.'s (Cary, NC) JMP Pro 10.0.0.
RESULTS
The study included 1648 respondents in the moved units in the baseline period (ie, units designated to move to a new clinical building) and 1373 respondents in the postmove period. There were 1593 respondents in the control group during the baseline period and 1049 respondents in the postmove period. For the units that moved, survey response rates were 28.5% prior to the move and 28.3% after the move. For the units that did not move, survey response rates were 20.9% prior to the move and 22.7% after the move. A majority of survey respondents on the nursing units that moved were white, male, and had private insurance (Table 1). There were no significant differences between respondents across these characteristics between the pre‐ and postmove periods. Mean age and LOS were also similar. For these units, there were 70.5% private rooms prior to the move and 100% after the move. For the unmoved units, 58.9% of the rooms were private in the baseline period and 72.7% were private in the study period. Similar to the units that moved, characteristics of the respondents on the unmoved units also did not differ significantly in the postmove period.
Patient demographics | Moved Units (N=3,021) | Unmoved Units (N=2,642) | ||||
---|---|---|---|---|---|---|
Pre | Post | P Value | Pre | Post | P Value | |
| ||||||
White | 75.3% | 78.2% | 0.07 | 66.7% | 68.5% | 0.31 |
Mean age, y | 57.3 | 57.4 | 0.84 | 57.3 | 57.1 | 0.81 |
Male | 54.3% | 53.0% | 0.48 | 40.5% | 42.3% | 0.23 |
Self‐reported health | ||||||
Excellent or very good | 54.7% | 51.2% | 0.04 | 38.7% | 39.5% | 0.11 |
Good | 27.8% | 32.0% | 29.3% | 32.2% | ||
Fair or poor | 17.5% | 16.9% | 32.0% | 28.3% | ||
Self‐reported language | ||||||
English | 96.0% | 97.2% | 0.06 | 96.8% | 97.1% | 0.63 |
Other | 4.0% | 2.8% | 3.2% | 2.9% | ||
Self‐reported education | ||||||
Less than high school | 5.8% | 5.0% | 0.24 | 10.8% | 10.4% | 0.24 |
High school grad | 46.4% | 44.2% | 48.6% | 45.5% | ||
College grad or more | 47.7% | 50.7% | 40.7% | 44.7% | ||
Insurance type | ||||||
Medicaid | 6.7% | 5.5% | 0.11 | 10.8% | 9.0% | 0.32 |
Medicare | 32.0% | 35.5% | 36.0% | 36.1% | ||
Private insurance | 55.6% | 52.8% | 48.0% | 50.3% | ||
Mean APRDRG complexity* | 2.1 | 2.1 | 0.09 | 2.3 | 2.3 | 0.14 |
Mean LOS | 4.7 | 5.0 | 0.12 | 4.9 | 5.0 | 0.77 |
Service | ||||||
Medicine | 15.4% | 16.2% | 0.51 | 40.0% | 34.5% | 0.10 |
Surgery | 50.7% | 45.7% | 40.1% | 44.1% | ||
Neurosciences | 20.3% | 24.1% | 6.0% | 6.0% | ||
Obstetrics/gynecology | 7.5% | 8.2% | 5.7% | 5.6% |
The move was associated with significant improvements in facility‐related satisfaction (Tables 2 and 3). The most prominent increases in satisfaction were with pleasantness of dcor (33.6% vs 66.2%), noise level (39.9% vs 59.3%), and visitor accommodation and comfort (50.0% vs 70.3 %). There was improvement in satisfaction related to cleanliness of the room (49.0% vs 68.6 %), but no significant increase in satisfaction with courtesy of the person cleaning the room (59.8% vs 67.7%) when compared with units that did move.
Satisfaction Domain | Moved Units | Unmoved Units | P Value of the Difference in Odds Ratio Between Moved and Unmoved Units | |||||
---|---|---|---|---|---|---|---|---|
% Top Box | Adjusted Odds Ratio* (95% CI) | % Top Box | Adjusted Odds Ratio* (95% CI) | |||||
Pre | Post | Pre | Post | |||||
| ||||||||
FACILITY RELATED | ||||||||
Hospital environment | ||||||||
Cleanliness of the room and bathroom | 61.0 | 70.8 | 1.62 (1.40‐1.90) | 64.0 | 69.2 | 1.24 (1.03‐1.48) | 0.03 | |
Quietness of the room | 51.3 | 65.4 | 1.89 (1.63‐2.19) | 58.6 | 60.3 | 1.08 (0.90‐1.28) | <0.0001 | |
NONFACILITY RELATED | ||||||||
Nursing communication | ||||||||
Nurses treated with courtesy/respect | 84.0 | 86.7 | 1.28 (1.05‐1.57) | 83.6 | 87.1 | 1.29 (1.02‐1.64) | 0.92 | |
Nurses listened | 73.1 | 76.4 | 1.21 (1.03‐1.43) | 74.2 | 75.5 | 1.05 (0.86‐1.27) | 0.26 | |
Nurses explained | 75.0 | 76.6 | 1.10 (0.94‐1.30) | 76.0 | 76.2 | 1.00 (0.82‐1.21) | 0.43 | |
Physician communication | ||||||||
Doctors treated with courtesy/respect | 89.5 | 90.5 | 1.13 (0.89‐1.42) | 84.9 | 87.3 | 1.20 (0.94‐1.53) | 0.77 | |
Doctors listened | 81.4 | 81.0 | 0.93 (0.83‐1.19) | 77.7 | 77.1 | 0.94 (0.77‐1.15) | 0.68 | |
Doctors explained | 79.2 | 79.0 | 1.00(0.84‐1.19) | 75.7 | 74.4 | 0.92 (0.76‐1.12) | 0.49 | |
Other | ||||||||
Help toileting as soon as you wanted | 61.8 | 63.7 | 1.08 (0.89‐1.32) | 62.3 | 60.6 | 0.92 (0.71‐1.18) | 0.31 | |
Pain well controlled | 63.2 | 63.8 | 1.06 (0.90‐1.25) | 62.0 | 62.6 | 0.99 (0.81‐1.20) | 060 | |
Staff do everything to help with pain | 77.7 | 80.1 | 1.19 (0.99‐1.44) | 76.8 | 75.7 | 0.90 (0.75‐1.13) | 0.07 | |
Staff describe medicine side effects | 47.0 | 47.6 | 1.05 (0.89‐1.24) | 49.2 | 47.1 | 0.91 (0.74‐1.11) | 0.32 | |
Tell you what new medicine was for | 76.4 | 76.4 | 1.02 (0.84‐1.25) | 77.1 | 78.8 | 1.09(0.85‐1.39) | 0.65 | |
Overall | ||||||||
Rate hospital (010) | 75.0 | 83.3 | 1.71 (1.44‐2.05) | 75.7 | 77.6 | 1.06 (0.87‐1.29) | 0.006 | |
Recommend hospital | 82.5 | 87.1 | 1.43 (1.18‐1.76) | 81.4 | 82.0 | 0.98 (0.79‐1.22) | 0.03 |
Satisfaction Domain | Moved Unit | Unmoved Unit | P Value of the Difference in Odds Ratio Between Moved and Unmoved Units | ||||
---|---|---|---|---|---|---|---|
% Top Box | Adjusted Odds Ratio* (95% CI) | % Top Box | Adjusted Odds Ratio* (95% CI) | ||||
Pre | Post | Pre | Post | ||||
| |||||||
FACILITY RELATED | |||||||
Room | |||||||
Pleasantness of room dcor | 33.6 | 64.8 | 3.77 (3.24‐4.38) | 41.6 | 47.0 | 1.21 (1.02‐1.44) | <0.0001 |
Room cleanliness | 49.0 | 68.6 | 2.35 (2.02‐2.73) | 51.6 | 59.1 | 1.32 (1.12‐1.58) | <0.0001 |
Room temperature | 43.1 | 54.9 | 1.64 (1.43‐1.90) | 45.0 | 48.8 | 1.14 (0.96‐1.36) | 0.002 |
Noise level in and around the room | 40.2 | 59.2 | 2.23 (1.92‐2.58) | 45.5 | 47.6 | 1.07 (0.90‐1.22) | <0.0001 |
Visitor related | |||||||
Accommodations and comfort of visitors | 50.0 | 70.3 | 2.44 (2.10‐2.83) | 55.3 | 59.1 | 1.14 (0.96‐1.35) | <0.0001 |
NONFACILITY RELATED | |||||||
Food | |||||||
Temperature of the food | 31.1 | 33.6 | 1.15 (0.99‐1.34) | 34.0 | 38.9 | 1.23 (1.02‐1.47) | 0.51 |
Quality of the food | 25.8 | 27.1 | 1.10 (0.93‐1.30) | 30.2 | 36.2 | 1.32 (1.10‐1.59) | 0.12 |
Courtesy of the person who served food | 63.9 | 62.3 | 0.93 (0.80‐1.10) | 66.0 | 61.4 | 0.82 (0.69‐0.98) | 0.26 |
Nursing | |||||||
Friendliness/courtesy of the nurses | 76.3 | 82.8 | 1.49 (1.26‐1.79) | 77.7 | 80.1 | 1.10 (0.90‐1.37) | 0.04 |
Promptness of response to call | 60.1 | 62.6 | 1.14 (0.98‐1.33) | 59.2 | 62.0 | 1.10 (0.91‐1.31) | 0.80 |
Nurses' attitude toward requests | 71.0 | 75.8 | 1.30 (1.11‐1.54) | 70.5 | 72.4 | 1.06 (0.88‐1.28) | 0.13 |
Attention to special/personal needs | 66.7 | 72.2 | 1.32 (1.13‐1.54) | 67.8 | 70.3 | 1.09 (0.91‐1.31) | 0.16 |
Nurses kept you informed | 64.3 | 72.2 | 1.46 (1.25‐1.70) | 65.8 | 69.8 | 1.17 (0.98‐1.41) | 0.88 |
Skill of the nurses | 75.3 | 79.5 | 1.28 (1.08‐1.52) | 74.3 | 78.6 | 1.23 (1.01‐1.51) | 0.89 |
Ancillary staff | |||||||
Courtesy of the person cleaning the room | 59.8 | 67.7 | 1.41 (1.21‐1.65) | 61.2 | 66.5 | 1.24 (1.03‐1.49) | 0.28 |
Courtesy of the person who took blood | 66.5 | 68.1 | 1.10 (0.94‐1.28) | 63.2 | 63.1 | 0.96 (0.76‐1.08) | 0.34 |
Courtesy of the person who started the IV | 70.0 | 71.7 | 1.09 (0.93‐1.28) | 66.6 | 69.3 | 1.11 (0.92‐1.33) | 0.88 |
Visitor related | |||||||
Staff attitude toward visitors | 68.1 | 79.4 | 1.84 (1.56‐2.18) | 70.3 | 72.2 | 1.06 (0.87‐1.28) | <0.0001 |
Physician | |||||||
Time physician spent with you | 55.0 | 58.9 | 1.20 (1.04‐1.39) | 53.2 | 55.9 | 1.10 (0.92‐1.30) | 0.46 |
Physician concern questions/worries | 67.2 | 70.7 | 1.20 (1.03‐1.40) | 64.3 | 66.1 | 1.05 (0.88‐1.26) | 0.31 |
Physician kept you informed | 65.3 | 67.5 | 1.12 (0.96‐1.30) | 61.6 | 63.2 | 1.05 (0.88‐1.25) | 0.58 |
Friendliness/courtesy of physician | 76.3 | 78.1 | 1.11 (0.93‐1.31) | 71.0 | 73.3 | 1.08 (0.90‐1.31) | 0.89 |
Skill of physician | 85.4 | 88.5 | 1.35 (1.09‐1.68) | 78.0 | 81.0 | 1.15 (0.93‐1.43) | 0.34 |
Discharge | |||||||
Extent felt ready for discharge | 62.0 | 66.7 | 1.23 (1.07‐1.44) | 59.2 | 62.3 | 1.10 (0.92‐1.30) | 0.35 |
Speed of discharge process | 50.7 | 54.2 | 1.16 (1.01‐1.33) | 47.8 | 50.0 | 1.07 (0.90‐1.27) | 0.49 |
Instructions for care at home | 66.4 | 71.1 | 1.25 (1.06‐1.46) | 64.0 | 67.7 | 1.16 (0.97‐1.39) | 0.54 |
Staff concern for your privacy | 65.3 | 71.8 | 1.37 (1.17‐0.85) | 63.6 | 66.2 | 1.10 (0.91‐1.31) | 0.07 |
Miscellaneous | |||||||
How well your pain was controlled | 64.2 | 66.5 | 1.14 (0.97‐1.32) | 60.2 | 62.6 | 1.07 (0.89‐1.28) | 0.66 |
Staff addressed emotional needs | 60.0 | 63.4 | 1.19 (1.02‐1.38) | 55.1 | 60.2 | 1.20 (1.01‐1.42) | 0.90 |
Response to concerns/complaints | 61.1 | 64.5 | 1.19 (1.02‐1.38) | 57.2 | 60.1 | 1.10 (0.92‐1.31) | 0.57 |
Overall | |||||||
Staff worked together to care for you | 72.6 | 77.2 | 1.29 (1.10‐1.52) | 70.3 | 73.2 | 1.13 (0.93‐1.37) | 0.30 |
Likelihood of recommending hospital | 79.1 | 84.3 | 1.44 (1.20‐1.74) | 76.3 | 79.2 | 1.14 (0.93‐1.39) | 0.10 |
Overall rating of care given | 76.8 | 83.0 | 1.50 (1.25‐1.80) | 74.7 | 77.2 | 1.10 (0.90‐1.34) | 0.03 |
With regard to nonfacility‐related satisfaction, there were statistically higher scores in several nursing, physician, and discharge‐related satisfaction domains after the move. However, these changes were not associated with the move to the new clinical building as they were not significantly different from improvements on the unmoved units. Among nonfacility‐related items, only staff attitude toward visitors showed significant improvement (68.1% vs 79.4%). There was a significant improvement in hospital rating (75.0% vs 83.3% in the moved units and 75.7% vs 77.6% in the unmoved units). However, the other 3 measures of overall satisfaction did not show significant improvement associated with the move to the new clinical building when compared to the concurrent controls.
DISCUSSION
Contrary to our hypothesis and a belief held by many, we found that patients appeared able to distinguish their experience with hospital environment from their experience with providers and other services. Improvement in hospital facilities with incorporation of patient‐centered features was associated with improvements that were largely limited to increases in satisfaction with quietness, cleanliness, temperature, and dcor of the room along with visitor‐related satisfaction. Notably, there was no significant improvement in satisfaction related to physicians, nurses, housekeeping, and other service staff. There was improvement in satisfaction with staff attitude toward visitors, but this can be attributed to availability of visitor‐friendly facilities. There was a significant improvement in 1 of the 4 measures of overall satisfaction. Our findings also support the construct validity of HCAHPS and Press Ganey patient satisfaction surveys.
Ours is one of the largest studies on patient satisfaction related to patient‐centered design features in the inpatient acute care setting. Swan et al. also studied patients in an acute inpatient setting and compared satisfaction related to appealing versus typical hospital rooms. Patients were matched for case mix, insurance, gender, types of medical services received and LOS, and were served by the same set of physicians and similar food service and housekeeping staff.[26] Unlike our study, they found improved satisfaction related to physicians, housekeeping staff, food service staff, meals, and overall satisfaction. However, the study had some limitations. In particular, the study sample was self‐selected because the patients in this group were required to pay an extra daily fee to utilize the appealing room. Additionally, there were only 177 patients across the 2 groups, and the actual differences in satisfaction scores were small. Our sample was larger and patients in the study group were admitted to units in the new clinical buildings by the same criteria as they were admitted to the historic building prior to the move, and there were no significant differences in baseline characteristics between the comparison groups.
Jansen et al. also found broad improvements in patient satisfaction in a study of over 309 maternity unit patients in a new construction, all private‐room maternity unit with more appealing design elements and comfort features for visitors.[7] Improved satisfaction was noted with the physical environment, nursing care, assistance with feeding, respect for privacy, and discharge planning. However, it is difficult to extrapolate the results of this study to other settings, as maternity unit patients constitute a unique patient demographic with unique care needs. Additionally, when compared with patients in the control group, the patients in the study group were cared for by nurses who had a lower workload and who were not assigned other patients with more complex needs. Because nursing availability may be expected to impact satisfaction with clinical domains, the impact of private and appealing room may very well have been limited to improved satisfaction with the physical environment.
Despite the widespread belief among healthcare leadership that facility renovation or expansion is a vital strategy for improving patient satisfaction, our study shows that this may not be a dominant factor.[27] In fact, the Planetree model showed that improvement in satisfaction related to physical environment and nursing care was associated with implementation of both patient‐centered design features as well as with utilization of nurses that were trained to provide personalized care, educate patients, and involve patients and family.[28] It is more likely that provider‐level interventions will have a greater impact on provider level and overall satisfaction. This idea is supported by a recent JD Powers study suggesting that facilities represent only 19% of overall satisfaction in the inpatient setting.[35]
Although our study focused on patient‐centered design features, several renovation and construction projects have also focused on design features that improve patient safety and provider satisfaction, workflow, efficiency, productivity, stress, and time spent in direct care.[9] Interventions in these areas may lead to improvement in patient outcomes and perhaps lead to improvement in patient satisfaction; however, this relationship has not been well established at present.
In an era of cost containment, healthcare administrators are faced with high‐priced interventions, competing needs, limited resources, low profit margins, and often unclear evidence on cost‐effectiveness and return on investment of healthcare design features. Benefits are related to competitive advantage, higher reputation, patient retention, decreased malpractice costs, and increased Medicare payments through VBP programs that incentivize improved performance on quality metrics and patient satisfaction surveys. Our study supports the idea that a significant improvement in patient satisfaction related to creature comforts can be achieved with investment in patient‐centered design features. However, our findings also suggest that institutions should perform an individualized cost‐benefit analysis related to improvements in this narrow area of patient satisfaction. In our study, incorporation of patient‐centered design features resulted in improvement on 2 VBP HCAHPS measures, and its contribution toward total performance score under the VBP program would be limited.
Strengths of our study include the use of concurrent controls and our ability to capitalize on a natural experiment in which care teams remained constant before and after a move to a new clinical building. However, our study has some limitations. It was conducted at a single tertiary care academic center that predominantly serves an inner city population and referral patients seeking specialized care. Drivers of patient satisfaction may be different in community hospitals, and a different relationship may be observed between patient‐centered design and domains of patient satisfaction in this setting. Further studies in different hospital settings are needed to confirm our findings. Additionally, we were limited by the low response rate of the surveys. However, this is a widespread problem with all patient satisfaction research utilizing voluntary surveys, and our response rates are consistent with those previously reported.[34, 36, 37, 38] Furthermore, low response rates have not impeded the implementation of pay‐for‐performance programs on a national scale using HCHAPS.
In conclusion, our study suggests that hospitals should not use outdated facilities as an excuse for achievement of suboptimal satisfaction scores. Patients respond positively to creature comforts, pleasing surroundings, and visitor‐friendly facilities but can distinguish these positive experiences from experiences in other patient satisfaction domains. In our study, the move to a higher‐amenity building had only a modest impact on overall patient satisfaction, perhaps because clinical care is the primary driver of this outcome. Contrary to belief held by some hospital leaders, major strides in overall satisfaction across the board and other subdomains of satisfaction likely require intervention in areas other than facility renovation and expansion.
Disclosures
Zishan Siddiqui, MD, was supported by the Osler Center of Clinical Excellence Faculty Scholarship Grant. Funds from Johns Hopkins Hospitalist Scholars Program supported the research project. The authors have no conflict of interests to disclose.
- Create a blueprint for successful hospital construction. Nurs Manage. 2006;37(6):39–44. , .
- Walter Reed National Military Medical Center website. Facts at a glance. Available at: http://www.wrnmmc.capmed.mil/About%20Us/SitePages/Facts.aspx. Accessed June 19, 2013.
- http://www.healthcaredesignmagazine.com/building‐ideas/keys‐collaboration. Accessed June 19, 2013. . Keys to collaboration. Healthcare Design website. Available at:
- http://www.healthcaredesignmagazine.com/building‐ideas/tale‐4‐hospitals. Accessed June 19, 2013. . A tale of 4 hospitals. Healthcare Design website. Available at:
- http://www.healthcaredesignmagazine.com/building‐ideas/gateway‐east. Accessed June 19, 2013. . Gateway to the east. Healthcare Design website. Available at:
- http://www.healthcaredesignmagazine.com/building‐ideas/lessons‐learned. Accessed June 19, 2013. . Lessons learned. Healthcare Design website. Available at:
- Single room maternity care and client satisfaction. Birth. 2000;27(4):235–243. , , , , .
- Same‐handed and mirrored unit configurations: is there a difference in patient and nurse outcomes? J Nurs Adm. 2011;41(6):273–279. , , , .
- The Pebble Projects: coordinated evidence‐based case studies. Build Res Inform. 2008;36(2):129–145. , .
- Effects of exposure to nature and abstract pictures on patients recovering from open heart surgery. J Soc Psychophysiol Res. 1993;30:7. , , .
- Postoperative delirium. Curr Drug Targets. 2005;6(7):807–814. , , , .
- Stimulus deprivation in windowless rooms. Anaesthesia. 1977;32(7):598–602. .
- Post‐occupancy evaluation of healing gardens in a pediatric cancer center. Landsc Urban Plan. 2005;73(2):167–183. , , , .
- Healing gardens in hospitals. Interdiscip Des Res J. 2007;1(1):1–27. .
- Restorative gardens. BMJ. 1993;306(6885):1080–1081. , .
- Effects of interior design on wellness: theory and recent scientific research. J Health Care Inter Des. 1991;3:97–109. .
- Sunny hospital rooms expedite recovery from severe and refractory depressions. J Affect Disord. 1996;40(1‐2):49–51. , .
- Art in hospital spaces: the role of hospitals in an aestheticised society. Int J Cult Policy. 2007;13(1):85–101. .
- Renovation of a semiprivate patient room. Bowman Center Geriatric Rehabilitation Unit. Nurs Clin North Am 1995;30(1):97–115. , , .
- (2013). Effect of intensive care environment on family and patient satisfaction: a before‐after study. Intensive Care Med. 2013;39(9):1626–1634. , , , et al.
- Outcomes of environmental appraisal of different hospital waiting areas. Environ Behav. 2003;35(6):842–869. , , , , .
- Redesigning the neurocritical care unit to enhance family participation and improve outcomes. Cleve Clin J Med. 2009;76(suppl 2):S70–S74. .
- The ecology of the patient visit: physical attractiveness, waiting times, and perceived quality of care. J Ambul Care Manage. 2008;31(2):128–141. , .
- Patient satisfaction and the new consumer. Hosp Health Netw. 2006;80(57):59–62. .
- Patient satisfaction. Hospitals embrace hotel‐like amenities. Hosp Health Netw. 2007;81(11):24–26. .
- Do appealing hospital rooms increase patient evaluations of physicians, nurses, and hospital services? Health Care Manage Rev. 2003;28(3):254–264. , , .
- http://www.healthleadersmedia.com/intelligence/detail.cfm?content_id=28289334(2):125–133. . Patient experience and HCAHPS: little consensus on a top priority. Health Leaders Media website. Available at
- Centers for Medicare 67:27–37.
- Hospital Consumer Assessment of Healthcare Providers and Systems. Summary analysis. http://www.hcahpsonline.org/SummaryAnalyses.aspx. Accessed October 1, 2014.
- Centers for Medicare 44(2 pt 1):501–518.
- J.D. Power and Associates. Patient satisfaction influenced more by hospital staff than by the hospital facilities. Available at: http://www.jdpower.com/press‐releases/2012‐national‐patient‐experience‐study#sthash.gSv6wAdc.dpuf. Accessed December 10, 2013.
- Racial and ethnic differences in a patient survey: patients' values, ratings, and reports regarding physician primary care performance in a large health maintenance organization. Med Care. 2000;38(3): 300–310. , , , , .
- Patient experience in safety‐net hospitals implications for improving care and Value‐Based Purchasing patient experience in safety‐net hospitals. Arch Intern Med. 2012;172(16):1204–1210. , , , .
- Comparison of Hospital Consumer Assessment of Healthcare Providers and Systems patient satisfaction scores for specialty hospitals and general medical hospitals: confounding effect of survey response rate. J Hosp Med. 2014;9(9):590–593. , , , .
Hospitals are expensive and complex facilities to build and renovate. It is estimated $200 billion is being spent in the United States during this decade on hospital construction and renovation, and further expenditures in this area are expected.[1] Aging hospital infrastructure, competition, and health system expansion have motivated institutions to invest in renovation and new hospital building construction.[2, 3, 4, 5, 6, 7] There is a trend toward patient‐centered design in new hospital construction. Features of this trend include same‐handed design (ie, rooms on a unit have all beds oriented in the same direction and do not share headwalls); use of sound absorbent materials to reduced ambient noise[7, 8, 9]; rooms with improved view and increased natural lighting to reduce anxiety, decrease delirium, and increase sense of wellbeing[10, 11, 12]; incorporation of natural elements like gardens, water features, and art[12, 13, 14, 15, 16, 17, 18]; single‐patient rooms to reduce transmission of infection and enhance privacy and visitor comfort[7, 19, 20]; presence of comfortable waiting rooms and visitor accommodations to enhance comfort and family participation[21, 22, 23]; and hotel‐like amenities such as on‐demand entertainment and room service menus.[24, 25]
There is a belief among some hospital leaders that patients are generally unable to distinguish their positive experience with a pleasing healthcare environment from their positive experience with care, and thus improving facilities will lead to improved satisfaction across the board.[26, 27] In a controlled study of hospitalized patients, appealing rooms were associated with increased satisfaction with services including housekeeping and food service staff, meals, as well as physicians and overall satisfaction.[26] A 2012 survey of hospital leadership found that expanding and renovating facilities was considered a top priority in improving patient satisfaction, with 82% of the respondents stating that this was important.[27]
Despite these attitudes, the impact of patient‐centered design on patient satisfaction is not well understood. Studies have shown that renovations and hospital construction that incorporates noise reduction strategies, positive distraction, patient and caregiver control, attractive waiting rooms, improved patient room appearance, private rooms, and large windows result in improved satisfaction with nursing, noise level, unit environment and cleanliness, perceived wait time, discharge preparedness, and overall care. [7, 19, 20, 23, 28] However, these studies were limited by small sample size, inclusion of a narrow group of patients (eg, ambulatory, obstetric, geriatric rehabilitation, intensive care unit), and concurrent use of interventions other than design improvement (eg, nurse and patient education). Many of these studies did not use the ubiquitous Hospital Consumer Assessment of Healthcare Providers and Systems (HCAHPS) and Press Ganey patient satisfaction surveys.
We sought to determine the changes in patient satisfaction that occurred during a natural experiment, in which clinical units (comprising stable nursing, physician, and unit teams) were relocated from an historic clinical building to a new clinical building that featured patient‐centered design, using HCAHPS and Press Ganey surveys and a large study population. We hypothesized that new building features would positively impact both facility related (eg, noise level), nonfacility related (eg, physician and housekeeping service related), and overall satisfaction.
METHODS
This was a retrospective analysis of prospectively collected Press Ganey and HCAPHS patient satisfaction survey data for a single academic tertiary care hospital.[29] The research project was reviewed and approved by the institutional review board.
Participants
All patients discharged from 12 clinical units that relocated to the new clinical building and returned patient satisfaction surveys served as study patients. The moved units included the coronary care unit, cardiac step down unit, medical intensive care unit, neuro critical care unit, surgical intensive care unit, orthopedic unit, neurology unit, neurosurgery unit, obstetrics units, gynecology unit, urology unit, cardiothoracic surgery unit, and the transplant surgery and renal transplant unit. Patients on clinical units that did not move served as concurrent controls.
Exposure
Patients admitted to the new clinical building experienced several patient‐centered design features. These features included easy access to healing gardens with a water feature, soaring lobbies, a collection of more than 500 works of art, well‐decorated and light‐filled patient rooms with sleeping accommodations for family members, sound‐absorbing features in patient care corridors ranging from acoustical ceiling tiles to a quiet nurse‐call system, and an interactive television network with Internet, movies, and games. All patients during the baseline period and control patients during the study period were located in typical patient rooms with standard hospital amenities. No other major patient satisfaction interventions were initiated during the pre‐ or postperiod in either arm of the study; ongoing patient satisfaction efforts (such as unit‐based customer care representatives) were deployed broadly and not restricted to the new clinical building. Clinical teams comprised of physicians, nurses, and ancillary staff did not change significantly after the move.
Time Periods
The move to new clinical building occurred on May 1, 2012. After allowing for a 15‐day washout period, the postmove period included Press Ganey and HCAHPS surveys returned for discharges that occurred during a 7.5‐month period between May 15, 2102 and December 31, 2012. Baseline data included Press Ganey and HCAHPS surveys returned for discharges in the preceding 12 months (May 1, 2011 to April 30, 2012). Sensitivity analysis using only 7.5 months of baseline data did not reveal any significant difference when compared with 12‐month baseline data, and we report only data from the 12‐month baseline period.
Instruments
Press Ganey and HCAHPS patient satisfaction surveys were sent via mail in the same envelope. Fifty percent of the discharged patients were randomized to receive the surveys. The Press Ganey survey contained 33 items covering across several subdomains including room, meal, nursing, physician, ancillary staff, visitor, discharge, and overall satisfaction. The HCAHPS survey contained 29 Centers for Medicare and Medicaid Services (CMS)‐mandated items, of which 21 are related to patient satisfaction. The development and testing and methods for administration and reporting of the HCAHPS survey have been previously described.[30, 31] Press Ganey patient satisfaction survey results have been reported in the literature.[32, 33]
Outcome Variables
Press Ganey and HCAHPS patient satisfaction survey responses were the primary outcome variables of the study. The survey items were categorized as facility related (eg, noise level), nonfacility related (eg, physician and nursing staff satisfaction), and overall satisfaction related.
Covariates
Age, sex, length of stay (LOS), insurance type, and all‐payer refined diagnosis‐related groupassociated illness complexity were included as covariates.
Statistical Analysis
Percent top‐box scores were calculated for each survey item as the percent of patients who responded very good for a given item on Press Ganey survey items and always or definitely yes or 9 or 10 on HCAHPS survey items. CMS utilizes percent top‐box scores to calculate payments under the Value Based Purchasing (VBP) program and to report the results publicly. Numerous studies have also reported percent top‐box scores for HCAHPS survey results.[31, 32, 33, 34]
Odds ratios of premove versus postmove percentage of top‐box scores, adjusted for age, sex, LOS, complexity of illness, and insurance type were determined using logistic regression for the units that moved. Similar scores were calculated for unmoved units to detect secular trends. To determine whether the differences between the moved and unmoved units were significant, we introduced the interaction term (moved vs unmoved unit status) (pre‐ vs postmove time period) into the logistic regression models and examined the adjusted P value for this term. All statistical analysis was performed using SAS Institute Inc.'s (Cary, NC) JMP Pro 10.0.0.
RESULTS
The study included 1648 respondents in the moved units in the baseline period (ie, units designated to move to a new clinical building) and 1373 respondents in the postmove period. There were 1593 respondents in the control group during the baseline period and 1049 respondents in the postmove period. For the units that moved, survey response rates were 28.5% prior to the move and 28.3% after the move. For the units that did not move, survey response rates were 20.9% prior to the move and 22.7% after the move. A majority of survey respondents on the nursing units that moved were white, male, and had private insurance (Table 1). There were no significant differences between respondents across these characteristics between the pre‐ and postmove periods. Mean age and LOS were also similar. For these units, there were 70.5% private rooms prior to the move and 100% after the move. For the unmoved units, 58.9% of the rooms were private in the baseline period and 72.7% were private in the study period. Similar to the units that moved, characteristics of the respondents on the unmoved units also did not differ significantly in the postmove period.
Patient demographics | Moved Units (N=3,021) | Unmoved Units (N=2,642) | ||||
---|---|---|---|---|---|---|
Pre | Post | P Value | Pre | Post | P Value | |
| ||||||
White | 75.3% | 78.2% | 0.07 | 66.7% | 68.5% | 0.31 |
Mean age, y | 57.3 | 57.4 | 0.84 | 57.3 | 57.1 | 0.81 |
Male | 54.3% | 53.0% | 0.48 | 40.5% | 42.3% | 0.23 |
Self‐reported health | ||||||
Excellent or very good | 54.7% | 51.2% | 0.04 | 38.7% | 39.5% | 0.11 |
Good | 27.8% | 32.0% | 29.3% | 32.2% | ||
Fair or poor | 17.5% | 16.9% | 32.0% | 28.3% | ||
Self‐reported language | ||||||
English | 96.0% | 97.2% | 0.06 | 96.8% | 97.1% | 0.63 |
Other | 4.0% | 2.8% | 3.2% | 2.9% | ||
Self‐reported education | ||||||
Less than high school | 5.8% | 5.0% | 0.24 | 10.8% | 10.4% | 0.24 |
High school grad | 46.4% | 44.2% | 48.6% | 45.5% | ||
College grad or more | 47.7% | 50.7% | 40.7% | 44.7% | ||
Insurance type | ||||||
Medicaid | 6.7% | 5.5% | 0.11 | 10.8% | 9.0% | 0.32 |
Medicare | 32.0% | 35.5% | 36.0% | 36.1% | ||
Private insurance | 55.6% | 52.8% | 48.0% | 50.3% | ||
Mean APRDRG complexity* | 2.1 | 2.1 | 0.09 | 2.3 | 2.3 | 0.14 |
Mean LOS | 4.7 | 5.0 | 0.12 | 4.9 | 5.0 | 0.77 |
Service | ||||||
Medicine | 15.4% | 16.2% | 0.51 | 40.0% | 34.5% | 0.10 |
Surgery | 50.7% | 45.7% | 40.1% | 44.1% | ||
Neurosciences | 20.3% | 24.1% | 6.0% | 6.0% | ||
Obstetrics/gynecology | 7.5% | 8.2% | 5.7% | 5.6% |
The move was associated with significant improvements in facility‐related satisfaction (Tables 2 and 3). The most prominent increases in satisfaction were with pleasantness of dcor (33.6% vs 66.2%), noise level (39.9% vs 59.3%), and visitor accommodation and comfort (50.0% vs 70.3 %). There was improvement in satisfaction related to cleanliness of the room (49.0% vs 68.6 %), but no significant increase in satisfaction with courtesy of the person cleaning the room (59.8% vs 67.7%) when compared with units that did move.
Satisfaction Domain | Moved Units | Unmoved Units | P Value of the Difference in Odds Ratio Between Moved and Unmoved Units | |||||
---|---|---|---|---|---|---|---|---|
% Top Box | Adjusted Odds Ratio* (95% CI) | % Top Box | Adjusted Odds Ratio* (95% CI) | |||||
Pre | Post | Pre | Post | |||||
| ||||||||
FACILITY RELATED | ||||||||
Hospital environment | ||||||||
Cleanliness of the room and bathroom | 61.0 | 70.8 | 1.62 (1.40‐1.90) | 64.0 | 69.2 | 1.24 (1.03‐1.48) | 0.03 | |
Quietness of the room | 51.3 | 65.4 | 1.89 (1.63‐2.19) | 58.6 | 60.3 | 1.08 (0.90‐1.28) | <0.0001 | |
NONFACILITY RELATED | ||||||||
Nursing communication | ||||||||
Nurses treated with courtesy/respect | 84.0 | 86.7 | 1.28 (1.05‐1.57) | 83.6 | 87.1 | 1.29 (1.02‐1.64) | 0.92 | |
Nurses listened | 73.1 | 76.4 | 1.21 (1.03‐1.43) | 74.2 | 75.5 | 1.05 (0.86‐1.27) | 0.26 | |
Nurses explained | 75.0 | 76.6 | 1.10 (0.94‐1.30) | 76.0 | 76.2 | 1.00 (0.82‐1.21) | 0.43 | |
Physician communication | ||||||||
Doctors treated with courtesy/respect | 89.5 | 90.5 | 1.13 (0.89‐1.42) | 84.9 | 87.3 | 1.20 (0.94‐1.53) | 0.77 | |
Doctors listened | 81.4 | 81.0 | 0.93 (0.83‐1.19) | 77.7 | 77.1 | 0.94 (0.77‐1.15) | 0.68 | |
Doctors explained | 79.2 | 79.0 | 1.00(0.84‐1.19) | 75.7 | 74.4 | 0.92 (0.76‐1.12) | 0.49 | |
Other | ||||||||
Help toileting as soon as you wanted | 61.8 | 63.7 | 1.08 (0.89‐1.32) | 62.3 | 60.6 | 0.92 (0.71‐1.18) | 0.31 | |
Pain well controlled | 63.2 | 63.8 | 1.06 (0.90‐1.25) | 62.0 | 62.6 | 0.99 (0.81‐1.20) | 060 | |
Staff do everything to help with pain | 77.7 | 80.1 | 1.19 (0.99‐1.44) | 76.8 | 75.7 | 0.90 (0.75‐1.13) | 0.07 | |
Staff describe medicine side effects | 47.0 | 47.6 | 1.05 (0.89‐1.24) | 49.2 | 47.1 | 0.91 (0.74‐1.11) | 0.32 | |
Tell you what new medicine was for | 76.4 | 76.4 | 1.02 (0.84‐1.25) | 77.1 | 78.8 | 1.09(0.85‐1.39) | 0.65 | |
Overall | ||||||||
Rate hospital (010) | 75.0 | 83.3 | 1.71 (1.44‐2.05) | 75.7 | 77.6 | 1.06 (0.87‐1.29) | 0.006 | |
Recommend hospital | 82.5 | 87.1 | 1.43 (1.18‐1.76) | 81.4 | 82.0 | 0.98 (0.79‐1.22) | 0.03 |
Satisfaction Domain | Moved Unit | Unmoved Unit | P Value of the Difference in Odds Ratio Between Moved and Unmoved Units | ||||
---|---|---|---|---|---|---|---|
% Top Box | Adjusted Odds Ratio* (95% CI) | % Top Box | Adjusted Odds Ratio* (95% CI) | ||||
Pre | Post | Pre | Post | ||||
| |||||||
FACILITY RELATED | |||||||
Room | |||||||
Pleasantness of room dcor | 33.6 | 64.8 | 3.77 (3.24‐4.38) | 41.6 | 47.0 | 1.21 (1.02‐1.44) | <0.0001 |
Room cleanliness | 49.0 | 68.6 | 2.35 (2.02‐2.73) | 51.6 | 59.1 | 1.32 (1.12‐1.58) | <0.0001 |
Room temperature | 43.1 | 54.9 | 1.64 (1.43‐1.90) | 45.0 | 48.8 | 1.14 (0.96‐1.36) | 0.002 |
Noise level in and around the room | 40.2 | 59.2 | 2.23 (1.92‐2.58) | 45.5 | 47.6 | 1.07 (0.90‐1.22) | <0.0001 |
Visitor related | |||||||
Accommodations and comfort of visitors | 50.0 | 70.3 | 2.44 (2.10‐2.83) | 55.3 | 59.1 | 1.14 (0.96‐1.35) | <0.0001 |
NONFACILITY RELATED | |||||||
Food | |||||||
Temperature of the food | 31.1 | 33.6 | 1.15 (0.99‐1.34) | 34.0 | 38.9 | 1.23 (1.02‐1.47) | 0.51 |
Quality of the food | 25.8 | 27.1 | 1.10 (0.93‐1.30) | 30.2 | 36.2 | 1.32 (1.10‐1.59) | 0.12 |
Courtesy of the person who served food | 63.9 | 62.3 | 0.93 (0.80‐1.10) | 66.0 | 61.4 | 0.82 (0.69‐0.98) | 0.26 |
Nursing | |||||||
Friendliness/courtesy of the nurses | 76.3 | 82.8 | 1.49 (1.26‐1.79) | 77.7 | 80.1 | 1.10 (0.90‐1.37) | 0.04 |
Promptness of response to call | 60.1 | 62.6 | 1.14 (0.98‐1.33) | 59.2 | 62.0 | 1.10 (0.91‐1.31) | 0.80 |
Nurses' attitude toward requests | 71.0 | 75.8 | 1.30 (1.11‐1.54) | 70.5 | 72.4 | 1.06 (0.88‐1.28) | 0.13 |
Attention to special/personal needs | 66.7 | 72.2 | 1.32 (1.13‐1.54) | 67.8 | 70.3 | 1.09 (0.91‐1.31) | 0.16 |
Nurses kept you informed | 64.3 | 72.2 | 1.46 (1.25‐1.70) | 65.8 | 69.8 | 1.17 (0.98‐1.41) | 0.88 |
Skill of the nurses | 75.3 | 79.5 | 1.28 (1.08‐1.52) | 74.3 | 78.6 | 1.23 (1.01‐1.51) | 0.89 |
Ancillary staff | |||||||
Courtesy of the person cleaning the room | 59.8 | 67.7 | 1.41 (1.21‐1.65) | 61.2 | 66.5 | 1.24 (1.03‐1.49) | 0.28 |
Courtesy of the person who took blood | 66.5 | 68.1 | 1.10 (0.94‐1.28) | 63.2 | 63.1 | 0.96 (0.76‐1.08) | 0.34 |
Courtesy of the person who started the IV | 70.0 | 71.7 | 1.09 (0.93‐1.28) | 66.6 | 69.3 | 1.11 (0.92‐1.33) | 0.88 |
Visitor related | |||||||
Staff attitude toward visitors | 68.1 | 79.4 | 1.84 (1.56‐2.18) | 70.3 | 72.2 | 1.06 (0.87‐1.28) | <0.0001 |
Physician | |||||||
Time physician spent with you | 55.0 | 58.9 | 1.20 (1.04‐1.39) | 53.2 | 55.9 | 1.10 (0.92‐1.30) | 0.46 |
Physician concern questions/worries | 67.2 | 70.7 | 1.20 (1.03‐1.40) | 64.3 | 66.1 | 1.05 (0.88‐1.26) | 0.31 |
Physician kept you informed | 65.3 | 67.5 | 1.12 (0.96‐1.30) | 61.6 | 63.2 | 1.05 (0.88‐1.25) | 0.58 |
Friendliness/courtesy of physician | 76.3 | 78.1 | 1.11 (0.93‐1.31) | 71.0 | 73.3 | 1.08 (0.90‐1.31) | 0.89 |
Skill of physician | 85.4 | 88.5 | 1.35 (1.09‐1.68) | 78.0 | 81.0 | 1.15 (0.93‐1.43) | 0.34 |
Discharge | |||||||
Extent felt ready for discharge | 62.0 | 66.7 | 1.23 (1.07‐1.44) | 59.2 | 62.3 | 1.10 (0.92‐1.30) | 0.35 |
Speed of discharge process | 50.7 | 54.2 | 1.16 (1.01‐1.33) | 47.8 | 50.0 | 1.07 (0.90‐1.27) | 0.49 |
Instructions for care at home | 66.4 | 71.1 | 1.25 (1.06‐1.46) | 64.0 | 67.7 | 1.16 (0.97‐1.39) | 0.54 |
Staff concern for your privacy | 65.3 | 71.8 | 1.37 (1.17‐0.85) | 63.6 | 66.2 | 1.10 (0.91‐1.31) | 0.07 |
Miscellaneous | |||||||
How well your pain was controlled | 64.2 | 66.5 | 1.14 (0.97‐1.32) | 60.2 | 62.6 | 1.07 (0.89‐1.28) | 0.66 |
Staff addressed emotional needs | 60.0 | 63.4 | 1.19 (1.02‐1.38) | 55.1 | 60.2 | 1.20 (1.01‐1.42) | 0.90 |
Response to concerns/complaints | 61.1 | 64.5 | 1.19 (1.02‐1.38) | 57.2 | 60.1 | 1.10 (0.92‐1.31) | 0.57 |
Overall | |||||||
Staff worked together to care for you | 72.6 | 77.2 | 1.29 (1.10‐1.52) | 70.3 | 73.2 | 1.13 (0.93‐1.37) | 0.30 |
Likelihood of recommending hospital | 79.1 | 84.3 | 1.44 (1.20‐1.74) | 76.3 | 79.2 | 1.14 (0.93‐1.39) | 0.10 |
Overall rating of care given | 76.8 | 83.0 | 1.50 (1.25‐1.80) | 74.7 | 77.2 | 1.10 (0.90‐1.34) | 0.03 |
With regard to nonfacility‐related satisfaction, there were statistically higher scores in several nursing, physician, and discharge‐related satisfaction domains after the move. However, these changes were not associated with the move to the new clinical building as they were not significantly different from improvements on the unmoved units. Among nonfacility‐related items, only staff attitude toward visitors showed significant improvement (68.1% vs 79.4%). There was a significant improvement in hospital rating (75.0% vs 83.3% in the moved units and 75.7% vs 77.6% in the unmoved units). However, the other 3 measures of overall satisfaction did not show significant improvement associated with the move to the new clinical building when compared to the concurrent controls.
DISCUSSION
Contrary to our hypothesis and a belief held by many, we found that patients appeared able to distinguish their experience with hospital environment from their experience with providers and other services. Improvement in hospital facilities with incorporation of patient‐centered features was associated with improvements that were largely limited to increases in satisfaction with quietness, cleanliness, temperature, and dcor of the room along with visitor‐related satisfaction. Notably, there was no significant improvement in satisfaction related to physicians, nurses, housekeeping, and other service staff. There was improvement in satisfaction with staff attitude toward visitors, but this can be attributed to availability of visitor‐friendly facilities. There was a significant improvement in 1 of the 4 measures of overall satisfaction. Our findings also support the construct validity of HCAHPS and Press Ganey patient satisfaction surveys.
Ours is one of the largest studies on patient satisfaction related to patient‐centered design features in the inpatient acute care setting. Swan et al. also studied patients in an acute inpatient setting and compared satisfaction related to appealing versus typical hospital rooms. Patients were matched for case mix, insurance, gender, types of medical services received and LOS, and were served by the same set of physicians and similar food service and housekeeping staff.[26] Unlike our study, they found improved satisfaction related to physicians, housekeeping staff, food service staff, meals, and overall satisfaction. However, the study had some limitations. In particular, the study sample was self‐selected because the patients in this group were required to pay an extra daily fee to utilize the appealing room. Additionally, there were only 177 patients across the 2 groups, and the actual differences in satisfaction scores were small. Our sample was larger and patients in the study group were admitted to units in the new clinical buildings by the same criteria as they were admitted to the historic building prior to the move, and there were no significant differences in baseline characteristics between the comparison groups.
Jansen et al. also found broad improvements in patient satisfaction in a study of over 309 maternity unit patients in a new construction, all private‐room maternity unit with more appealing design elements and comfort features for visitors.[7] Improved satisfaction was noted with the physical environment, nursing care, assistance with feeding, respect for privacy, and discharge planning. However, it is difficult to extrapolate the results of this study to other settings, as maternity unit patients constitute a unique patient demographic with unique care needs. Additionally, when compared with patients in the control group, the patients in the study group were cared for by nurses who had a lower workload and who were not assigned other patients with more complex needs. Because nursing availability may be expected to impact satisfaction with clinical domains, the impact of private and appealing room may very well have been limited to improved satisfaction with the physical environment.
Despite the widespread belief among healthcare leadership that facility renovation or expansion is a vital strategy for improving patient satisfaction, our study shows that this may not be a dominant factor.[27] In fact, the Planetree model showed that improvement in satisfaction related to physical environment and nursing care was associated with implementation of both patient‐centered design features as well as with utilization of nurses that were trained to provide personalized care, educate patients, and involve patients and family.[28] It is more likely that provider‐level interventions will have a greater impact on provider level and overall satisfaction. This idea is supported by a recent JD Powers study suggesting that facilities represent only 19% of overall satisfaction in the inpatient setting.[35]
Although our study focused on patient‐centered design features, several renovation and construction projects have also focused on design features that improve patient safety and provider satisfaction, workflow, efficiency, productivity, stress, and time spent in direct care.[9] Interventions in these areas may lead to improvement in patient outcomes and perhaps lead to improvement in patient satisfaction; however, this relationship has not been well established at present.
In an era of cost containment, healthcare administrators are faced with high‐priced interventions, competing needs, limited resources, low profit margins, and often unclear evidence on cost‐effectiveness and return on investment of healthcare design features. Benefits are related to competitive advantage, higher reputation, patient retention, decreased malpractice costs, and increased Medicare payments through VBP programs that incentivize improved performance on quality metrics and patient satisfaction surveys. Our study supports the idea that a significant improvement in patient satisfaction related to creature comforts can be achieved with investment in patient‐centered design features. However, our findings also suggest that institutions should perform an individualized cost‐benefit analysis related to improvements in this narrow area of patient satisfaction. In our study, incorporation of patient‐centered design features resulted in improvement on 2 VBP HCAHPS measures, and its contribution toward total performance score under the VBP program would be limited.
Strengths of our study include the use of concurrent controls and our ability to capitalize on a natural experiment in which care teams remained constant before and after a move to a new clinical building. However, our study has some limitations. It was conducted at a single tertiary care academic center that predominantly serves an inner city population and referral patients seeking specialized care. Drivers of patient satisfaction may be different in community hospitals, and a different relationship may be observed between patient‐centered design and domains of patient satisfaction in this setting. Further studies in different hospital settings are needed to confirm our findings. Additionally, we were limited by the low response rate of the surveys. However, this is a widespread problem with all patient satisfaction research utilizing voluntary surveys, and our response rates are consistent with those previously reported.[34, 36, 37, 38] Furthermore, low response rates have not impeded the implementation of pay‐for‐performance programs on a national scale using HCHAPS.
In conclusion, our study suggests that hospitals should not use outdated facilities as an excuse for achievement of suboptimal satisfaction scores. Patients respond positively to creature comforts, pleasing surroundings, and visitor‐friendly facilities but can distinguish these positive experiences from experiences in other patient satisfaction domains. In our study, the move to a higher‐amenity building had only a modest impact on overall patient satisfaction, perhaps because clinical care is the primary driver of this outcome. Contrary to belief held by some hospital leaders, major strides in overall satisfaction across the board and other subdomains of satisfaction likely require intervention in areas other than facility renovation and expansion.
Disclosures
Zishan Siddiqui, MD, was supported by the Osler Center of Clinical Excellence Faculty Scholarship Grant. Funds from Johns Hopkins Hospitalist Scholars Program supported the research project. The authors have no conflict of interests to disclose.
Hospitals are expensive and complex facilities to build and renovate. It is estimated $200 billion is being spent in the United States during this decade on hospital construction and renovation, and further expenditures in this area are expected.[1] Aging hospital infrastructure, competition, and health system expansion have motivated institutions to invest in renovation and new hospital building construction.[2, 3, 4, 5, 6, 7] There is a trend toward patient‐centered design in new hospital construction. Features of this trend include same‐handed design (ie, rooms on a unit have all beds oriented in the same direction and do not share headwalls); use of sound absorbent materials to reduced ambient noise[7, 8, 9]; rooms with improved view and increased natural lighting to reduce anxiety, decrease delirium, and increase sense of wellbeing[10, 11, 12]; incorporation of natural elements like gardens, water features, and art[12, 13, 14, 15, 16, 17, 18]; single‐patient rooms to reduce transmission of infection and enhance privacy and visitor comfort[7, 19, 20]; presence of comfortable waiting rooms and visitor accommodations to enhance comfort and family participation[21, 22, 23]; and hotel‐like amenities such as on‐demand entertainment and room service menus.[24, 25]
There is a belief among some hospital leaders that patients are generally unable to distinguish their positive experience with a pleasing healthcare environment from their positive experience with care, and thus improving facilities will lead to improved satisfaction across the board.[26, 27] In a controlled study of hospitalized patients, appealing rooms were associated with increased satisfaction with services including housekeeping and food service staff, meals, as well as physicians and overall satisfaction.[26] A 2012 survey of hospital leadership found that expanding and renovating facilities was considered a top priority in improving patient satisfaction, with 82% of the respondents stating that this was important.[27]
Despite these attitudes, the impact of patient‐centered design on patient satisfaction is not well understood. Studies have shown that renovations and hospital construction that incorporates noise reduction strategies, positive distraction, patient and caregiver control, attractive waiting rooms, improved patient room appearance, private rooms, and large windows result in improved satisfaction with nursing, noise level, unit environment and cleanliness, perceived wait time, discharge preparedness, and overall care. [7, 19, 20, 23, 28] However, these studies were limited by small sample size, inclusion of a narrow group of patients (eg, ambulatory, obstetric, geriatric rehabilitation, intensive care unit), and concurrent use of interventions other than design improvement (eg, nurse and patient education). Many of these studies did not use the ubiquitous Hospital Consumer Assessment of Healthcare Providers and Systems (HCAHPS) and Press Ganey patient satisfaction surveys.
We sought to determine the changes in patient satisfaction that occurred during a natural experiment, in which clinical units (comprising stable nursing, physician, and unit teams) were relocated from an historic clinical building to a new clinical building that featured patient‐centered design, using HCAHPS and Press Ganey surveys and a large study population. We hypothesized that new building features would positively impact both facility related (eg, noise level), nonfacility related (eg, physician and housekeeping service related), and overall satisfaction.
METHODS
This was a retrospective analysis of prospectively collected Press Ganey and HCAPHS patient satisfaction survey data for a single academic tertiary care hospital.[29] The research project was reviewed and approved by the institutional review board.
Participants
All patients discharged from 12 clinical units that relocated to the new clinical building and returned patient satisfaction surveys served as study patients. The moved units included the coronary care unit, cardiac step down unit, medical intensive care unit, neuro critical care unit, surgical intensive care unit, orthopedic unit, neurology unit, neurosurgery unit, obstetrics units, gynecology unit, urology unit, cardiothoracic surgery unit, and the transplant surgery and renal transplant unit. Patients on clinical units that did not move served as concurrent controls.
Exposure
Patients admitted to the new clinical building experienced several patient‐centered design features. These features included easy access to healing gardens with a water feature, soaring lobbies, a collection of more than 500 works of art, well‐decorated and light‐filled patient rooms with sleeping accommodations for family members, sound‐absorbing features in patient care corridors ranging from acoustical ceiling tiles to a quiet nurse‐call system, and an interactive television network with Internet, movies, and games. All patients during the baseline period and control patients during the study period were located in typical patient rooms with standard hospital amenities. No other major patient satisfaction interventions were initiated during the pre‐ or postperiod in either arm of the study; ongoing patient satisfaction efforts (such as unit‐based customer care representatives) were deployed broadly and not restricted to the new clinical building. Clinical teams comprised of physicians, nurses, and ancillary staff did not change significantly after the move.
Time Periods
The move to new clinical building occurred on May 1, 2012. After allowing for a 15‐day washout period, the postmove period included Press Ganey and HCAHPS surveys returned for discharges that occurred during a 7.5‐month period between May 15, 2102 and December 31, 2012. Baseline data included Press Ganey and HCAHPS surveys returned for discharges in the preceding 12 months (May 1, 2011 to April 30, 2012). Sensitivity analysis using only 7.5 months of baseline data did not reveal any significant difference when compared with 12‐month baseline data, and we report only data from the 12‐month baseline period.
Instruments
Press Ganey and HCAHPS patient satisfaction surveys were sent via mail in the same envelope. Fifty percent of the discharged patients were randomized to receive the surveys. The Press Ganey survey contained 33 items covering across several subdomains including room, meal, nursing, physician, ancillary staff, visitor, discharge, and overall satisfaction. The HCAHPS survey contained 29 Centers for Medicare and Medicaid Services (CMS)‐mandated items, of which 21 are related to patient satisfaction. The development and testing and methods for administration and reporting of the HCAHPS survey have been previously described.[30, 31] Press Ganey patient satisfaction survey results have been reported in the literature.[32, 33]
Outcome Variables
Press Ganey and HCAHPS patient satisfaction survey responses were the primary outcome variables of the study. The survey items were categorized as facility related (eg, noise level), nonfacility related (eg, physician and nursing staff satisfaction), and overall satisfaction related.
Covariates
Age, sex, length of stay (LOS), insurance type, and all‐payer refined diagnosis‐related groupassociated illness complexity were included as covariates.
Statistical Analysis
Percent top‐box scores were calculated for each survey item as the percent of patients who responded very good for a given item on Press Ganey survey items and always or definitely yes or 9 or 10 on HCAHPS survey items. CMS utilizes percent top‐box scores to calculate payments under the Value Based Purchasing (VBP) program and to report the results publicly. Numerous studies have also reported percent top‐box scores for HCAHPS survey results.[31, 32, 33, 34]
Odds ratios of premove versus postmove percentage of top‐box scores, adjusted for age, sex, LOS, complexity of illness, and insurance type were determined using logistic regression for the units that moved. Similar scores were calculated for unmoved units to detect secular trends. To determine whether the differences between the moved and unmoved units were significant, we introduced the interaction term (moved vs unmoved unit status) (pre‐ vs postmove time period) into the logistic regression models and examined the adjusted P value for this term. All statistical analysis was performed using SAS Institute Inc.'s (Cary, NC) JMP Pro 10.0.0.
RESULTS
The study included 1648 respondents in the moved units in the baseline period (ie, units designated to move to a new clinical building) and 1373 respondents in the postmove period. There were 1593 respondents in the control group during the baseline period and 1049 respondents in the postmove period. For the units that moved, survey response rates were 28.5% prior to the move and 28.3% after the move. For the units that did not move, survey response rates were 20.9% prior to the move and 22.7% after the move. A majority of survey respondents on the nursing units that moved were white, male, and had private insurance (Table 1). There were no significant differences between respondents across these characteristics between the pre‐ and postmove periods. Mean age and LOS were also similar. For these units, there were 70.5% private rooms prior to the move and 100% after the move. For the unmoved units, 58.9% of the rooms were private in the baseline period and 72.7% were private in the study period. Similar to the units that moved, characteristics of the respondents on the unmoved units also did not differ significantly in the postmove period.
Patient demographics | Moved Units (N=3,021) | Unmoved Units (N=2,642) | ||||
---|---|---|---|---|---|---|
Pre | Post | P Value | Pre | Post | P Value | |
| ||||||
White | 75.3% | 78.2% | 0.07 | 66.7% | 68.5% | 0.31 |
Mean age, y | 57.3 | 57.4 | 0.84 | 57.3 | 57.1 | 0.81 |
Male | 54.3% | 53.0% | 0.48 | 40.5% | 42.3% | 0.23 |
Self‐reported health | ||||||
Excellent or very good | 54.7% | 51.2% | 0.04 | 38.7% | 39.5% | 0.11 |
Good | 27.8% | 32.0% | 29.3% | 32.2% | ||
Fair or poor | 17.5% | 16.9% | 32.0% | 28.3% | ||
Self‐reported language | ||||||
English | 96.0% | 97.2% | 0.06 | 96.8% | 97.1% | 0.63 |
Other | 4.0% | 2.8% | 3.2% | 2.9% | ||
Self‐reported education | ||||||
Less than high school | 5.8% | 5.0% | 0.24 | 10.8% | 10.4% | 0.24 |
High school grad | 46.4% | 44.2% | 48.6% | 45.5% | ||
College grad or more | 47.7% | 50.7% | 40.7% | 44.7% | ||
Insurance type | ||||||
Medicaid | 6.7% | 5.5% | 0.11 | 10.8% | 9.0% | 0.32 |
Medicare | 32.0% | 35.5% | 36.0% | 36.1% | ||
Private insurance | 55.6% | 52.8% | 48.0% | 50.3% | ||
Mean APRDRG complexity* | 2.1 | 2.1 | 0.09 | 2.3 | 2.3 | 0.14 |
Mean LOS | 4.7 | 5.0 | 0.12 | 4.9 | 5.0 | 0.77 |
Service | ||||||
Medicine | 15.4% | 16.2% | 0.51 | 40.0% | 34.5% | 0.10 |
Surgery | 50.7% | 45.7% | 40.1% | 44.1% | ||
Neurosciences | 20.3% | 24.1% | 6.0% | 6.0% | ||
Obstetrics/gynecology | 7.5% | 8.2% | 5.7% | 5.6% |
The move was associated with significant improvements in facility‐related satisfaction (Tables 2 and 3). The most prominent increases in satisfaction were with pleasantness of dcor (33.6% vs 66.2%), noise level (39.9% vs 59.3%), and visitor accommodation and comfort (50.0% vs 70.3 %). There was improvement in satisfaction related to cleanliness of the room (49.0% vs 68.6 %), but no significant increase in satisfaction with courtesy of the person cleaning the room (59.8% vs 67.7%) when compared with units that did move.
Satisfaction Domain | Moved Units | Unmoved Units | P Value of the Difference in Odds Ratio Between Moved and Unmoved Units | |||||
---|---|---|---|---|---|---|---|---|
% Top Box | Adjusted Odds Ratio* (95% CI) | % Top Box | Adjusted Odds Ratio* (95% CI) | |||||
Pre | Post | Pre | Post | |||||
| ||||||||
FACILITY RELATED | ||||||||
Hospital environment | ||||||||
Cleanliness of the room and bathroom | 61.0 | 70.8 | 1.62 (1.40‐1.90) | 64.0 | 69.2 | 1.24 (1.03‐1.48) | 0.03 | |
Quietness of the room | 51.3 | 65.4 | 1.89 (1.63‐2.19) | 58.6 | 60.3 | 1.08 (0.90‐1.28) | <0.0001 | |
NONFACILITY RELATED | ||||||||
Nursing communication | ||||||||
Nurses treated with courtesy/respect | 84.0 | 86.7 | 1.28 (1.05‐1.57) | 83.6 | 87.1 | 1.29 (1.02‐1.64) | 0.92 | |
Nurses listened | 73.1 | 76.4 | 1.21 (1.03‐1.43) | 74.2 | 75.5 | 1.05 (0.86‐1.27) | 0.26 | |
Nurses explained | 75.0 | 76.6 | 1.10 (0.94‐1.30) | 76.0 | 76.2 | 1.00 (0.82‐1.21) | 0.43 | |
Physician communication | ||||||||
Doctors treated with courtesy/respect | 89.5 | 90.5 | 1.13 (0.89‐1.42) | 84.9 | 87.3 | 1.20 (0.94‐1.53) | 0.77 | |
Doctors listened | 81.4 | 81.0 | 0.93 (0.83‐1.19) | 77.7 | 77.1 | 0.94 (0.77‐1.15) | 0.68 | |
Doctors explained | 79.2 | 79.0 | 1.00(0.84‐1.19) | 75.7 | 74.4 | 0.92 (0.76‐1.12) | 0.49 | |
Other | ||||||||
Help toileting as soon as you wanted | 61.8 | 63.7 | 1.08 (0.89‐1.32) | 62.3 | 60.6 | 0.92 (0.71‐1.18) | 0.31 | |
Pain well controlled | 63.2 | 63.8 | 1.06 (0.90‐1.25) | 62.0 | 62.6 | 0.99 (0.81‐1.20) | 060 | |
Staff do everything to help with pain | 77.7 | 80.1 | 1.19 (0.99‐1.44) | 76.8 | 75.7 | 0.90 (0.75‐1.13) | 0.07 | |
Staff describe medicine side effects | 47.0 | 47.6 | 1.05 (0.89‐1.24) | 49.2 | 47.1 | 0.91 (0.74‐1.11) | 0.32 | |
Tell you what new medicine was for | 76.4 | 76.4 | 1.02 (0.84‐1.25) | 77.1 | 78.8 | 1.09(0.85‐1.39) | 0.65 | |
Overall | ||||||||
Rate hospital (010) | 75.0 | 83.3 | 1.71 (1.44‐2.05) | 75.7 | 77.6 | 1.06 (0.87‐1.29) | 0.006 | |
Recommend hospital | 82.5 | 87.1 | 1.43 (1.18‐1.76) | 81.4 | 82.0 | 0.98 (0.79‐1.22) | 0.03 |
Satisfaction Domain | Moved Unit | Unmoved Unit | P Value of the Difference in Odds Ratio Between Moved and Unmoved Units | ||||
---|---|---|---|---|---|---|---|
% Top Box | Adjusted Odds Ratio* (95% CI) | % Top Box | Adjusted Odds Ratio* (95% CI) | ||||
Pre | Post | Pre | Post | ||||
| |||||||
FACILITY RELATED | |||||||
Room | |||||||
Pleasantness of room dcor | 33.6 | 64.8 | 3.77 (3.24‐4.38) | 41.6 | 47.0 | 1.21 (1.02‐1.44) | <0.0001 |
Room cleanliness | 49.0 | 68.6 | 2.35 (2.02‐2.73) | 51.6 | 59.1 | 1.32 (1.12‐1.58) | <0.0001 |
Room temperature | 43.1 | 54.9 | 1.64 (1.43‐1.90) | 45.0 | 48.8 | 1.14 (0.96‐1.36) | 0.002 |
Noise level in and around the room | 40.2 | 59.2 | 2.23 (1.92‐2.58) | 45.5 | 47.6 | 1.07 (0.90‐1.22) | <0.0001 |
Visitor related | |||||||
Accommodations and comfort of visitors | 50.0 | 70.3 | 2.44 (2.10‐2.83) | 55.3 | 59.1 | 1.14 (0.96‐1.35) | <0.0001 |
NONFACILITY RELATED | |||||||
Food | |||||||
Temperature of the food | 31.1 | 33.6 | 1.15 (0.99‐1.34) | 34.0 | 38.9 | 1.23 (1.02‐1.47) | 0.51 |
Quality of the food | 25.8 | 27.1 | 1.10 (0.93‐1.30) | 30.2 | 36.2 | 1.32 (1.10‐1.59) | 0.12 |
Courtesy of the person who served food | 63.9 | 62.3 | 0.93 (0.80‐1.10) | 66.0 | 61.4 | 0.82 (0.69‐0.98) | 0.26 |
Nursing | |||||||
Friendliness/courtesy of the nurses | 76.3 | 82.8 | 1.49 (1.26‐1.79) | 77.7 | 80.1 | 1.10 (0.90‐1.37) | 0.04 |
Promptness of response to call | 60.1 | 62.6 | 1.14 (0.98‐1.33) | 59.2 | 62.0 | 1.10 (0.91‐1.31) | 0.80 |
Nurses' attitude toward requests | 71.0 | 75.8 | 1.30 (1.11‐1.54) | 70.5 | 72.4 | 1.06 (0.88‐1.28) | 0.13 |
Attention to special/personal needs | 66.7 | 72.2 | 1.32 (1.13‐1.54) | 67.8 | 70.3 | 1.09 (0.91‐1.31) | 0.16 |
Nurses kept you informed | 64.3 | 72.2 | 1.46 (1.25‐1.70) | 65.8 | 69.8 | 1.17 (0.98‐1.41) | 0.88 |
Skill of the nurses | 75.3 | 79.5 | 1.28 (1.08‐1.52) | 74.3 | 78.6 | 1.23 (1.01‐1.51) | 0.89 |
Ancillary staff | |||||||
Courtesy of the person cleaning the room | 59.8 | 67.7 | 1.41 (1.21‐1.65) | 61.2 | 66.5 | 1.24 (1.03‐1.49) | 0.28 |
Courtesy of the person who took blood | 66.5 | 68.1 | 1.10 (0.94‐1.28) | 63.2 | 63.1 | 0.96 (0.76‐1.08) | 0.34 |
Courtesy of the person who started the IV | 70.0 | 71.7 | 1.09 (0.93‐1.28) | 66.6 | 69.3 | 1.11 (0.92‐1.33) | 0.88 |
Visitor related | |||||||
Staff attitude toward visitors | 68.1 | 79.4 | 1.84 (1.56‐2.18) | 70.3 | 72.2 | 1.06 (0.87‐1.28) | <0.0001 |
Physician | |||||||
Time physician spent with you | 55.0 | 58.9 | 1.20 (1.04‐1.39) | 53.2 | 55.9 | 1.10 (0.92‐1.30) | 0.46 |
Physician concern questions/worries | 67.2 | 70.7 | 1.20 (1.03‐1.40) | 64.3 | 66.1 | 1.05 (0.88‐1.26) | 0.31 |
Physician kept you informed | 65.3 | 67.5 | 1.12 (0.96‐1.30) | 61.6 | 63.2 | 1.05 (0.88‐1.25) | 0.58 |
Friendliness/courtesy of physician | 76.3 | 78.1 | 1.11 (0.93‐1.31) | 71.0 | 73.3 | 1.08 (0.90‐1.31) | 0.89 |
Skill of physician | 85.4 | 88.5 | 1.35 (1.09‐1.68) | 78.0 | 81.0 | 1.15 (0.93‐1.43) | 0.34 |
Discharge | |||||||
Extent felt ready for discharge | 62.0 | 66.7 | 1.23 (1.07‐1.44) | 59.2 | 62.3 | 1.10 (0.92‐1.30) | 0.35 |
Speed of discharge process | 50.7 | 54.2 | 1.16 (1.01‐1.33) | 47.8 | 50.0 | 1.07 (0.90‐1.27) | 0.49 |
Instructions for care at home | 66.4 | 71.1 | 1.25 (1.06‐1.46) | 64.0 | 67.7 | 1.16 (0.97‐1.39) | 0.54 |
Staff concern for your privacy | 65.3 | 71.8 | 1.37 (1.17‐0.85) | 63.6 | 66.2 | 1.10 (0.91‐1.31) | 0.07 |
Miscellaneous | |||||||
How well your pain was controlled | 64.2 | 66.5 | 1.14 (0.97‐1.32) | 60.2 | 62.6 | 1.07 (0.89‐1.28) | 0.66 |
Staff addressed emotional needs | 60.0 | 63.4 | 1.19 (1.02‐1.38) | 55.1 | 60.2 | 1.20 (1.01‐1.42) | 0.90 |
Response to concerns/complaints | 61.1 | 64.5 | 1.19 (1.02‐1.38) | 57.2 | 60.1 | 1.10 (0.92‐1.31) | 0.57 |
Overall | |||||||
Staff worked together to care for you | 72.6 | 77.2 | 1.29 (1.10‐1.52) | 70.3 | 73.2 | 1.13 (0.93‐1.37) | 0.30 |
Likelihood of recommending hospital | 79.1 | 84.3 | 1.44 (1.20‐1.74) | 76.3 | 79.2 | 1.14 (0.93‐1.39) | 0.10 |
Overall rating of care given | 76.8 | 83.0 | 1.50 (1.25‐1.80) | 74.7 | 77.2 | 1.10 (0.90‐1.34) | 0.03 |
With regard to nonfacility‐related satisfaction, there were statistically higher scores in several nursing, physician, and discharge‐related satisfaction domains after the move. However, these changes were not associated with the move to the new clinical building as they were not significantly different from improvements on the unmoved units. Among nonfacility‐related items, only staff attitude toward visitors showed significant improvement (68.1% vs 79.4%). There was a significant improvement in hospital rating (75.0% vs 83.3% in the moved units and 75.7% vs 77.6% in the unmoved units). However, the other 3 measures of overall satisfaction did not show significant improvement associated with the move to the new clinical building when compared to the concurrent controls.
DISCUSSION
Contrary to our hypothesis and a belief held by many, we found that patients appeared able to distinguish their experience with hospital environment from their experience with providers and other services. Improvement in hospital facilities with incorporation of patient‐centered features was associated with improvements that were largely limited to increases in satisfaction with quietness, cleanliness, temperature, and dcor of the room along with visitor‐related satisfaction. Notably, there was no significant improvement in satisfaction related to physicians, nurses, housekeeping, and other service staff. There was improvement in satisfaction with staff attitude toward visitors, but this can be attributed to availability of visitor‐friendly facilities. There was a significant improvement in 1 of the 4 measures of overall satisfaction. Our findings also support the construct validity of HCAHPS and Press Ganey patient satisfaction surveys.
Ours is one of the largest studies on patient satisfaction related to patient‐centered design features in the inpatient acute care setting. Swan et al. also studied patients in an acute inpatient setting and compared satisfaction related to appealing versus typical hospital rooms. Patients were matched for case mix, insurance, gender, types of medical services received and LOS, and were served by the same set of physicians and similar food service and housekeeping staff.[26] Unlike our study, they found improved satisfaction related to physicians, housekeeping staff, food service staff, meals, and overall satisfaction. However, the study had some limitations. In particular, the study sample was self‐selected because the patients in this group were required to pay an extra daily fee to utilize the appealing room. Additionally, there were only 177 patients across the 2 groups, and the actual differences in satisfaction scores were small. Our sample was larger and patients in the study group were admitted to units in the new clinical buildings by the same criteria as they were admitted to the historic building prior to the move, and there were no significant differences in baseline characteristics between the comparison groups.
Jansen et al. also found broad improvements in patient satisfaction in a study of over 309 maternity unit patients in a new construction, all private‐room maternity unit with more appealing design elements and comfort features for visitors.[7] Improved satisfaction was noted with the physical environment, nursing care, assistance with feeding, respect for privacy, and discharge planning. However, it is difficult to extrapolate the results of this study to other settings, as maternity unit patients constitute a unique patient demographic with unique care needs. Additionally, when compared with patients in the control group, the patients in the study group were cared for by nurses who had a lower workload and who were not assigned other patients with more complex needs. Because nursing availability may be expected to impact satisfaction with clinical domains, the impact of private and appealing room may very well have been limited to improved satisfaction with the physical environment.
Despite the widespread belief among healthcare leadership that facility renovation or expansion is a vital strategy for improving patient satisfaction, our study shows that this may not be a dominant factor.[27] In fact, the Planetree model showed that improvement in satisfaction related to physical environment and nursing care was associated with implementation of both patient‐centered design features as well as with utilization of nurses that were trained to provide personalized care, educate patients, and involve patients and family.[28] It is more likely that provider‐level interventions will have a greater impact on provider level and overall satisfaction. This idea is supported by a recent JD Powers study suggesting that facilities represent only 19% of overall satisfaction in the inpatient setting.[35]
Although our study focused on patient‐centered design features, several renovation and construction projects have also focused on design features that improve patient safety and provider satisfaction, workflow, efficiency, productivity, stress, and time spent in direct care.[9] Interventions in these areas may lead to improvement in patient outcomes and perhaps lead to improvement in patient satisfaction; however, this relationship has not been well established at present.
In an era of cost containment, healthcare administrators are faced with high‐priced interventions, competing needs, limited resources, low profit margins, and often unclear evidence on cost‐effectiveness and return on investment of healthcare design features. Benefits are related to competitive advantage, higher reputation, patient retention, decreased malpractice costs, and increased Medicare payments through VBP programs that incentivize improved performance on quality metrics and patient satisfaction surveys. Our study supports the idea that a significant improvement in patient satisfaction related to creature comforts can be achieved with investment in patient‐centered design features. However, our findings also suggest that institutions should perform an individualized cost‐benefit analysis related to improvements in this narrow area of patient satisfaction. In our study, incorporation of patient‐centered design features resulted in improvement on 2 VBP HCAHPS measures, and its contribution toward total performance score under the VBP program would be limited.
Strengths of our study include the use of concurrent controls and our ability to capitalize on a natural experiment in which care teams remained constant before and after a move to a new clinical building. However, our study has some limitations. It was conducted at a single tertiary care academic center that predominantly serves an inner city population and referral patients seeking specialized care. Drivers of patient satisfaction may be different in community hospitals, and a different relationship may be observed between patient‐centered design and domains of patient satisfaction in this setting. Further studies in different hospital settings are needed to confirm our findings. Additionally, we were limited by the low response rate of the surveys. However, this is a widespread problem with all patient satisfaction research utilizing voluntary surveys, and our response rates are consistent with those previously reported.[34, 36, 37, 38] Furthermore, low response rates have not impeded the implementation of pay‐for‐performance programs on a national scale using HCHAPS.
In conclusion, our study suggests that hospitals should not use outdated facilities as an excuse for achievement of suboptimal satisfaction scores. Patients respond positively to creature comforts, pleasing surroundings, and visitor‐friendly facilities but can distinguish these positive experiences from experiences in other patient satisfaction domains. In our study, the move to a higher‐amenity building had only a modest impact on overall patient satisfaction, perhaps because clinical care is the primary driver of this outcome. Contrary to belief held by some hospital leaders, major strides in overall satisfaction across the board and other subdomains of satisfaction likely require intervention in areas other than facility renovation and expansion.
Disclosures
Zishan Siddiqui, MD, was supported by the Osler Center of Clinical Excellence Faculty Scholarship Grant. Funds from Johns Hopkins Hospitalist Scholars Program supported the research project. The authors have no conflict of interests to disclose.
- Create a blueprint for successful hospital construction. Nurs Manage. 2006;37(6):39–44. , .
- Walter Reed National Military Medical Center website. Facts at a glance. Available at: http://www.wrnmmc.capmed.mil/About%20Us/SitePages/Facts.aspx. Accessed June 19, 2013.
- http://www.healthcaredesignmagazine.com/building‐ideas/keys‐collaboration. Accessed June 19, 2013. . Keys to collaboration. Healthcare Design website. Available at:
- http://www.healthcaredesignmagazine.com/building‐ideas/tale‐4‐hospitals. Accessed June 19, 2013. . A tale of 4 hospitals. Healthcare Design website. Available at:
- http://www.healthcaredesignmagazine.com/building‐ideas/gateway‐east. Accessed June 19, 2013. . Gateway to the east. Healthcare Design website. Available at:
- http://www.healthcaredesignmagazine.com/building‐ideas/lessons‐learned. Accessed June 19, 2013. . Lessons learned. Healthcare Design website. Available at:
- Single room maternity care and client satisfaction. Birth. 2000;27(4):235–243. , , , , .
- Same‐handed and mirrored unit configurations: is there a difference in patient and nurse outcomes? J Nurs Adm. 2011;41(6):273–279. , , , .
- The Pebble Projects: coordinated evidence‐based case studies. Build Res Inform. 2008;36(2):129–145. , .
- Effects of exposure to nature and abstract pictures on patients recovering from open heart surgery. J Soc Psychophysiol Res. 1993;30:7. , , .
- Postoperative delirium. Curr Drug Targets. 2005;6(7):807–814. , , , .
- Stimulus deprivation in windowless rooms. Anaesthesia. 1977;32(7):598–602. .
- Post‐occupancy evaluation of healing gardens in a pediatric cancer center. Landsc Urban Plan. 2005;73(2):167–183. , , , .
- Healing gardens in hospitals. Interdiscip Des Res J. 2007;1(1):1–27. .
- Restorative gardens. BMJ. 1993;306(6885):1080–1081. , .
- Effects of interior design on wellness: theory and recent scientific research. J Health Care Inter Des. 1991;3:97–109. .
- Sunny hospital rooms expedite recovery from severe and refractory depressions. J Affect Disord. 1996;40(1‐2):49–51. , .
- Art in hospital spaces: the role of hospitals in an aestheticised society. Int J Cult Policy. 2007;13(1):85–101. .
- Renovation of a semiprivate patient room. Bowman Center Geriatric Rehabilitation Unit. Nurs Clin North Am 1995;30(1):97–115. , , .
- (2013). Effect of intensive care environment on family and patient satisfaction: a before‐after study. Intensive Care Med. 2013;39(9):1626–1634. , , , et al.
- Outcomes of environmental appraisal of different hospital waiting areas. Environ Behav. 2003;35(6):842–869. , , , , .
- Redesigning the neurocritical care unit to enhance family participation and improve outcomes. Cleve Clin J Med. 2009;76(suppl 2):S70–S74. .
- The ecology of the patient visit: physical attractiveness, waiting times, and perceived quality of care. J Ambul Care Manage. 2008;31(2):128–141. , .
- Patient satisfaction and the new consumer. Hosp Health Netw. 2006;80(57):59–62. .
- Patient satisfaction. Hospitals embrace hotel‐like amenities. Hosp Health Netw. 2007;81(11):24–26. .
- Do appealing hospital rooms increase patient evaluations of physicians, nurses, and hospital services? Health Care Manage Rev. 2003;28(3):254–264. , , .
- http://www.healthleadersmedia.com/intelligence/detail.cfm?content_id=28289334(2):125–133. . Patient experience and HCAHPS: little consensus on a top priority. Health Leaders Media website. Available at
- Centers for Medicare 67:27–37.
- Hospital Consumer Assessment of Healthcare Providers and Systems. Summary analysis. http://www.hcahpsonline.org/SummaryAnalyses.aspx. Accessed October 1, 2014.
- Centers for Medicare 44(2 pt 1):501–518.
- J.D. Power and Associates. Patient satisfaction influenced more by hospital staff than by the hospital facilities. Available at: http://www.jdpower.com/press‐releases/2012‐national‐patient‐experience‐study#sthash.gSv6wAdc.dpuf. Accessed December 10, 2013.
- Racial and ethnic differences in a patient survey: patients' values, ratings, and reports regarding physician primary care performance in a large health maintenance organization. Med Care. 2000;38(3): 300–310. , , , , .
- Patient experience in safety‐net hospitals implications for improving care and Value‐Based Purchasing patient experience in safety‐net hospitals. Arch Intern Med. 2012;172(16):1204–1210. , , , .
- Comparison of Hospital Consumer Assessment of Healthcare Providers and Systems patient satisfaction scores for specialty hospitals and general medical hospitals: confounding effect of survey response rate. J Hosp Med. 2014;9(9):590–593. , , , .
- Create a blueprint for successful hospital construction. Nurs Manage. 2006;37(6):39–44. , .
- Walter Reed National Military Medical Center website. Facts at a glance. Available at: http://www.wrnmmc.capmed.mil/About%20Us/SitePages/Facts.aspx. Accessed June 19, 2013.
- http://www.healthcaredesignmagazine.com/building‐ideas/keys‐collaboration. Accessed June 19, 2013. . Keys to collaboration. Healthcare Design website. Available at:
- http://www.healthcaredesignmagazine.com/building‐ideas/tale‐4‐hospitals. Accessed June 19, 2013. . A tale of 4 hospitals. Healthcare Design website. Available at:
- http://www.healthcaredesignmagazine.com/building‐ideas/gateway‐east. Accessed June 19, 2013. . Gateway to the east. Healthcare Design website. Available at:
- http://www.healthcaredesignmagazine.com/building‐ideas/lessons‐learned. Accessed June 19, 2013. . Lessons learned. Healthcare Design website. Available at:
- Single room maternity care and client satisfaction. Birth. 2000;27(4):235–243. , , , , .
- Same‐handed and mirrored unit configurations: is there a difference in patient and nurse outcomes? J Nurs Adm. 2011;41(6):273–279. , , , .
- The Pebble Projects: coordinated evidence‐based case studies. Build Res Inform. 2008;36(2):129–145. , .
- Effects of exposure to nature and abstract pictures on patients recovering from open heart surgery. J Soc Psychophysiol Res. 1993;30:7. , , .
- Postoperative delirium. Curr Drug Targets. 2005;6(7):807–814. , , , .
- Stimulus deprivation in windowless rooms. Anaesthesia. 1977;32(7):598–602. .
- Post‐occupancy evaluation of healing gardens in a pediatric cancer center. Landsc Urban Plan. 2005;73(2):167–183. , , , .
- Healing gardens in hospitals. Interdiscip Des Res J. 2007;1(1):1–27. .
- Restorative gardens. BMJ. 1993;306(6885):1080–1081. , .
- Effects of interior design on wellness: theory and recent scientific research. J Health Care Inter Des. 1991;3:97–109. .
- Sunny hospital rooms expedite recovery from severe and refractory depressions. J Affect Disord. 1996;40(1‐2):49–51. , .
- Art in hospital spaces: the role of hospitals in an aestheticised society. Int J Cult Policy. 2007;13(1):85–101. .
- Renovation of a semiprivate patient room. Bowman Center Geriatric Rehabilitation Unit. Nurs Clin North Am 1995;30(1):97–115. , , .
- (2013). Effect of intensive care environment on family and patient satisfaction: a before‐after study. Intensive Care Med. 2013;39(9):1626–1634. , , , et al.
- Outcomes of environmental appraisal of different hospital waiting areas. Environ Behav. 2003;35(6):842–869. , , , , .
- Redesigning the neurocritical care unit to enhance family participation and improve outcomes. Cleve Clin J Med. 2009;76(suppl 2):S70–S74. .
- The ecology of the patient visit: physical attractiveness, waiting times, and perceived quality of care. J Ambul Care Manage. 2008;31(2):128–141. , .
- Patient satisfaction and the new consumer. Hosp Health Netw. 2006;80(57):59–62. .
- Patient satisfaction. Hospitals embrace hotel‐like amenities. Hosp Health Netw. 2007;81(11):24–26. .
- Do appealing hospital rooms increase patient evaluations of physicians, nurses, and hospital services? Health Care Manage Rev. 2003;28(3):254–264. , , .
- http://www.healthleadersmedia.com/intelligence/detail.cfm?content_id=28289334(2):125–133. . Patient experience and HCAHPS: little consensus on a top priority. Health Leaders Media website. Available at
- Centers for Medicare 67:27–37.
- Hospital Consumer Assessment of Healthcare Providers and Systems. Summary analysis. http://www.hcahpsonline.org/SummaryAnalyses.aspx. Accessed October 1, 2014.
- Centers for Medicare 44(2 pt 1):501–518.
- J.D. Power and Associates. Patient satisfaction influenced more by hospital staff than by the hospital facilities. Available at: http://www.jdpower.com/press‐releases/2012‐national‐patient‐experience‐study#sthash.gSv6wAdc.dpuf. Accessed December 10, 2013.
- Racial and ethnic differences in a patient survey: patients' values, ratings, and reports regarding physician primary care performance in a large health maintenance organization. Med Care. 2000;38(3): 300–310. , , , , .
- Patient experience in safety‐net hospitals implications for improving care and Value‐Based Purchasing patient experience in safety‐net hospitals. Arch Intern Med. 2012;172(16):1204–1210. , , , .
- Comparison of Hospital Consumer Assessment of Healthcare Providers and Systems patient satisfaction scores for specialty hospitals and general medical hospitals: confounding effect of survey response rate. J Hosp Med. 2014;9(9):590–593. , , , .
© 2014 Society of Hospital Medicine
PCPs Who Adopted the Hospitalist Model
Although primary care physicians (PCPs) have traditionally treated patients in both ambulatory and hospital settings, many relinquished inpatient duties to hospitalists in recent decades.[1] Little is known about the PCPs who relinquished inpatient care duties or how the transition to the hospitalist model occurred. For example, what are the characteristics of PCPs who change? Do PCPs adopt the hospitalist model enthusiastically or cautiously? Characterizing PCPs who adopted the hospitalist model can help hospitalists understand their specialty's history and also inform health services research.
Much of the interest in the hospitalist model has been generated by studies reporting improved outcomes and lower hospital lengths of stay associated with hospitalist care.[2, 3, 4, 5] Conversely, detractors of the model point to reports of higher postacute care utilization among hospitalist patients.[6] Although these studies usually adjusted for differences among patients and hospitals, they did not account for PCP characteristics. As patients' access to PCPs and their PCP's capabilities are both plausible factors that could influence hospital length of stay (eg, decisions to complete more or less of a workup in the hospital), quality of care transitions, and postdischarge utilization, it is important to determine if PCPs who use hospitalists differ systematically from those who do not to correctly interpret health system utilization patterns that currently are attributed only to hospitalists.[7, 8]
We conducted this study to determine if observable PCP factors are associated with patients' use of hospitalists and to describe the trajectory by which PCPs referred their patients to hospitalists over time.
METHODS
Source of Data
We used claims data from 100% of Texas Medicare beneficiaries from 2000 to 2009, including Medicare beneficiary summary files, Medicare Provider Analysis and Review (MedPAR) files, Outpatient Standard Analytical Files (OutSAF), and Medicare Carrier files. Diagnosis related group (DRG)‐associated information, including weights, and Major Diagnostic Categories, were obtained from Centers for Medicare & Medicaid Services (
Establishment of the Study Cohort
Using the MedPAR file, we first selected hospital admissions from acute care hospitals in Texas for each year of the study period. We excluded beneficiaries younger than 66 years old, with incomplete Medicare Parts A and B enrollment, or with any health maintenance organization enrollment in the 12 months prior to the admission of interest. For patients with more than 1 admission in a given year, we randomly selected 1 admission. We then attempted to assign each patient to a PCP. We defined a PCP as a generalist (general practitioner, family physician, internist, or geriatrician) who saw a given beneficiary on 3 or more occasions in an outpatient setting in the year prior to the admission of interest.[9] We identified outpatient visits using Current Procedural Terminology (CPT) codes 99201 to 99205 (new patient encounters), and 99211 to 99215 (established patient encounters) from Carrier files. If more than 1 generalist physician saw the beneficiary on 3 or more occasions in a given year, the physician with more than 75% of the total outpatient evaluation and management (E&M) billings was classified as the beneficiary's PCP. Using these criteria, approximately 66% of patients were assigned to a PCP.
For cross‐sectional analyses, we restricted our cohort to beneficiaries whose PCPs were associated with at least 20 inpatients in a given year. To study trends in PCP practice patterns over time, we further restricted the cohort to beneficiaries whose PCPs were associated with at least 20 inpatients in every year of the study period, resulting in 1172 PCPs for the trajectory analyses. The reliability of PCPs' practice profiles increases as the number of patients in their panel increases. We chose 20 inpatients as the minimum because PCPs with 20 hospitalized patients per study year would achieve a reliability of 0.9 for estimating the proportion of their patients that received care from hospitalists.[10]
Identification of Hospitalists
We defined hospitalists as generalists who had at least 100 E&M billings in a given year and generated at least 90% of their total E&M billings in the year from inpatient services.[1] Inpatient E&M billings were identified by CPT codes 99221 to 99223 (new or established patient encounters), 99231 to 99233 (subsequent hospital care), and 99251 to 99255 (inpatient consultations).[1]
Patient Measures
Patient demographic information including, age at admission, gender, race/ethnicity, and Medicaid eligibility were obtained from Medicare beneficiary summary files. We used the Medicaid indicator as a proxy for low socioeconomic status. Information on weekday versus weekend admission, emergent admission, and DRG were obtained from MedPAR files. The DRG category (circulatory system, digestive system, infectious disease, nervous system, respiratory system, or other) was determined based on its Major Diagnostic Category. We determined residence in a nursing facility in the 3 months before the admission of interest from the MedPAR files and by E&M codes 99304 to 99318 (nursing facility services) from Carrier files.[11] Comorbidities were identified using the claims from MedPAR, Carrier, and OutSAF files in the year prior to the admission of interest.[12] Total hospitalizations and outpatient visits in the prior year were identified from MedPAR files and Carrier files, respectively.
PCP Measures
We categorized PCPs by specialty (general practice, gamily practice, geriatric medicine, or internal medicine), years in practice, gender, US‐ versus foreign‐trained, metropolitan statistical area (MSA) of their practice location, and board certification status. The specialty was identified from Carrier files and the other information from AMA data. For each PCP, the total number of outpatient visits and total number of patients seen as outpatients in each year was calculated based on E&M codes (9920199205, 9921199215) from Carrier files. For each year, we computed the average outpatient age, gender, race, and outpatient comorbidity for each PCP's patient panel. We computed hospital volumes using the number of hospitalized patients associated with each PCP in the study cohort.
Study Outcome
To determine whether hospitalized patients received care from hospitalists during a given hospitalization, we identified all inpatient E&M bills from generalist physicians during the admission of interest by linking MedPAR and Carrier files. If more than 50% of the generalist inpatient E&M billings from generalist physicians were from 1 or more hospitalists, the patient was considered to have received care from hospitalists.
Statistical Analyses
Multilevel analyses were used to account for the clustering of patients within PCPs. All multilevel models were adjusted for patient characteristics including age, race/ethnicity, gender, Medicaid eligibility, emergency admission, weekend admission, DRG weight, DRG category, any nursing home stay in the prior 3 months, number of comorbidities, number of hospitalizations, and number of physician visits in the year prior to the admission of interest. To analyze trends in practice patterns, we first used multilevel models to calculate the proportions of inpatients cared for by hospitalists each year for each of the 1172 PCPs with at least 20 patients. Then we employed an SAS procedure (PROC TRAJ) developed by Jones et al. to classify these PCPs into groups based on their trajectories.[13] This group‐based trajectory modeling allowed us to identify relatively homogeneous clusters within a heterogeneous sample population.[14] We chose a model that classified the PCPs into 4 groups.[15] With 4 groups, the average of the posterior probabilities of group membership for the PCPs assigned to each group exceeded 0.93, indicating a low rate of misclassification among these 4 distinct groups. For the 1172 PCPs, we tested interactions between year of hospitalization and PCP characteristics while adjusting for patient characteristics in order to investigate whether or not the impacts of PCP characteristics on how likely their patients being cared for by hospitalists differed with time. All analyses were performed with SAS version 9.2 (SAS Institute Inc., Cary, NC).
RESULTS
During the 2001 through 2009 study period, between 2252 and 2848 PCPs were associated with at least 20 hospitalized beneficiaries in any single year. Among these, 1172 PCPs were associated with at least 20 hospitalized beneficiaries in every year of the study period. These 1172 PCPs were associated with 608,686 hospitalizations over the 9 years.
Table 1 presents the characteristics of the PCPs who contributed to the cross‐sectional analyses in 2001 (N=2252) and 2009 (N=2387), as well as the 1172 PCPs for whom we had data for all 9 years for the longitudinal analyses. Most PCPs were male, trained in the United States, and were board certified. The average number of Medicare patients seen by these PCPs and number of outpatient Medicare visits went up about 7% between 2001 and 2009.
PCP Characteristics | Cross‐Sectional Analysis | Trajectory Analysis, 20012009 | |
---|---|---|---|
2001 | 2009 | ||
| |||
Overall, no. (%) | 2,252 (100%) | 2,387 (100%) | 1,172 (100%) |
Specialty, no. (%) | |||
General practice | 39 (1.7%) | 34 (1.4%) | 15 (1.3%) |
Family practice | 948 (42.1%) | 1,089 (45.6%) | 466 (39.8%) |
Internal medicine | 1,255 (55.7%) | 1,249 (52.3%) | 688 (58.7%) |
Geriatrics | 10 (0.4%) | 15 (0.6%) | 3 (0.3%) |
Gender, no. (%) | |||
Male | 1,990 (88.4%) | 2,015 (84.4%) | 1,072 (91.5%) |
Female | 262 (11.6%) | 372 (15.6%) | 100 (8.5%) |
Trained in the United States, no. (%) | |||
Yes | 1,669 (74.1%) | 1,738 (72.8%) | 844 (72.0%) |
No | 583 (25.9%) | 649 (27.2%) | 328 (28.0%) |
Metropolitan statistical area, no. (%) | |||
99,999 or less | 417 (17.5) | 237 (20.2) | |
100,000249,000 | 438 (18.3) | 234 (20.0) | |
250,000999,999 | 381 (16.0) | 216 (18.4) | |
1,000,000 or more | 1,151 (48.2) | 485 (41.4) | |
Board certification, no. (%) | |||
Yes | 1,657 (69.4%) | 800 (68.3%) | |
No | 730 (30.6%) | 372 (31.7%) | |
Years in practice, 2001, meanSD (Q1Q3) | 22.310.6 (15.028.0) | 21.28.9 (15.027.0) | |
Years in practice, 2009, meanSD (Q1Q3) | 25.010.2 (17.032.0) | 29.28.9 (23.035.0) | |
Total no. of Medicare outpatient visits, 2001, meanSD (Q1Q3) | 1,624.8879.2 (1,057.51,970.0) | 1,883.39,48.5 (1,236.52,240.5) | |
Total no. of Medicare outpatient visits, 2009, meanSD (Q1Q3) | 1,733.81,053.3 (1,080.02,048.0) | 2,020.51,200.9 (1,334.52,373.0) | |
Total no. of Medicare outpatients, 2001, meanSD (Q1Q3) | 418.6186.9 (284.0522.0) | 473.4189.5 (338.0580.5) | |
Total no. of Medicare outpatients, 2009, meanSD (Q1Q3) | 448.7217.8 (300.0548.0) | 508.7238.2 (350.5615.0) | |
No. of hospitalized patients, 2001, meanSD (Q1Q3) | 46.025.0 (27.057.0) | 53.028.0 (32.066.0) | |
No. of hospitalized patients, 2009, meanSD (Q1Q3) | 44.024.0 (26.052.0) | 52.027.0 (33.065.0) | |
Average outpatient age, 2001, meanSD (Q1Q3) | 72.82.3 (71.574.2) | 72.82.1 (71.774.1) | |
Average outpatient age, 2009, meanSD (Q1Q3) | 72.12.8 (70.673.9) | 72.82.7 (71.474.5) | |
Average outpatient gender (% male), 2001, meanSD (Q1Q3) | 38.17.0 (35.542.3) | 38.56.4 (36.242.3) | |
Average outpatient gender (% male), 2009, meanSD (Q1Q3) | 40.27.6 (37.644.8) | 41.06.5 (38.644.8) | |
Average outpatient race (% white), 2001, meanSD (Q1Q3) | 84.316.4 (79.295.5) | 85.414.3 (79.995.7) | |
Average outpatient race (% white), 2009, meanSD (Q1Q3) | 85.214.4 (79.895.2) | 86.312.9 (80.895.6) | |
Average outpatient comorbidity, 2001, meanSD (Q1Q3)a | 1.60.5 (1.21.8) | 1.60.4 (1.21.8) | |
Average outpatient comorbidity, 2009, meanSD (Q1Q3)a | 2.20.6 (1.82.5) | 2.20.6 (1.72.5) |
Figure 1 graphs the percentage of PCPs as a function of what percent of their hospitalized patients received care from hospitalists, and how that changed from 2001 to 2009. For 70.9% of PCPs, fewer than 5% of their hospitalized patients received hospitalist care in 2001. By 2009, the percent of PCPs in this category had decreased to 15.2%. In contrast, in 2001, more than half of the patients for 2.1% of PCPs received hospitalist care, and the percent of PCPs in this category increased to 26.3% by 2009.
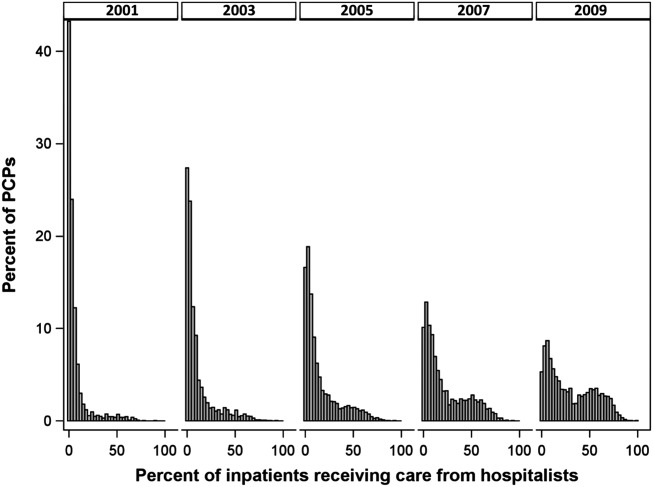
The pattern in Figure 1 shows that PCPs' use of hospitalists changed continuously and gradually over time. However, this pattern describes the PCPs as a group. When examined at the individual PCP level, different patterns emerge. Figure 2, which presents selected individual PCP's use of hospitalists over time, shows several distinct subpatterns of PCP practice behaviors. First, there are PCPs whose use of hospitalists was high in 2001 and stayed high or increased over time (eg, PCP A). There also were PCPs whose use of hospitalists stayed low over the entire study period (eg, PCP B). Finally, there were PCPs whose use of hospitalists was low in 2001 but high in 2009 (eg, PCP C). For this last group, the pattern of change in hospitalist utilization over time was discontinuous; that is, most of the increase occurred over a 1‐ or 2‐year period, instead of increasing gradually over time.
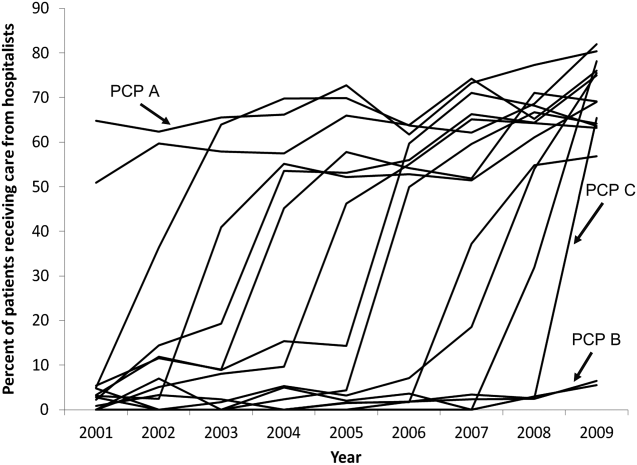
Among the 1172 PCPs associated with 20 hospitalized beneficiaries each year in all 9 years of the study period, group‐based trajectory modeling classified their practice patterns into 4 distinct trajectories (Figure 3). Among PCPs in group 1, more than one‐third of their hospitalized patients were cared for by hospitalists in 2001, and this increased to 60% by 2009. PCPs in groups 2 and 3 rarely used hospitalist care in 2001 but increased their use over time. The increase started early in the period for PCPs in group 2 and later for those in group 3. PCPs in group 4 were associated with little hospitalist use throughout the study period.
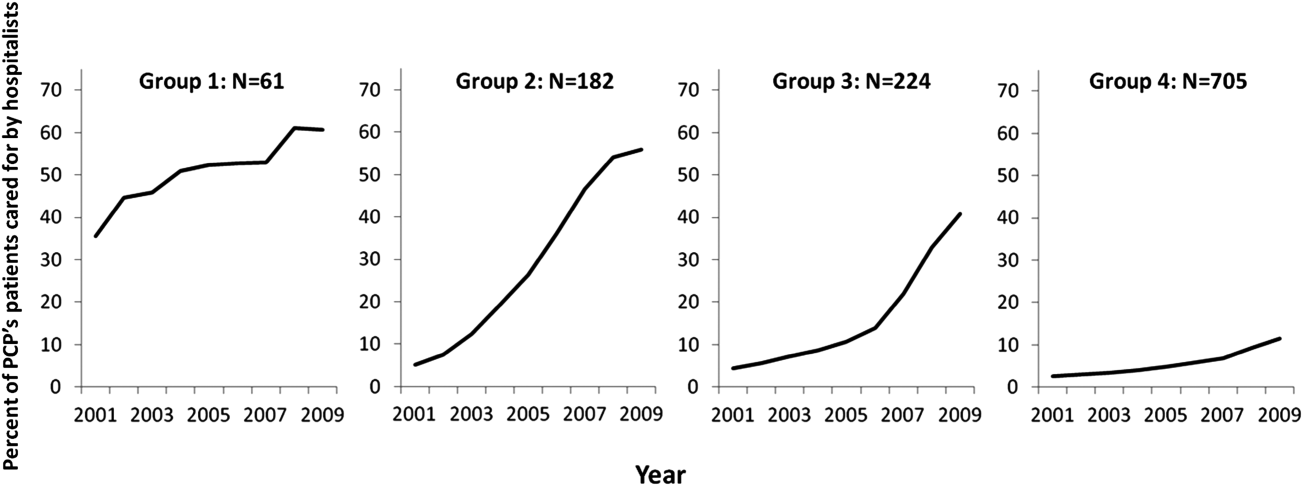
We constructed a model to describe the odds of a patient receiving care from hospitalists during the study period using patients associated with these 1172 PCPs. After adjusting for patient characteristics, the residual intraclass correlation coefficient for PCP level was 0.334, which indicates that 33.4% of the variance in whether a hospitalized patient received care from a hospitalist is explained by which PCP the patient saw. When adjusting for both patient and PCP characteristics, the overall odds of a patient receiving hospitalist care increased by 30% (95% confidence interval [CI]: 1.29‐1.30) per year from 2001 through 2009.
There were also significant interactions between year of hospitalization and several PCP characteristics. These interactions are illustrated in Table 2, which stratifies each of those PCP characteristics by 3 time periods: 2001 to 2003, 2004 to 2006, and 2007 to 2009. In all time periods, patients were more likely to receive hospitalist care if their PCP was US trained (US vs international medical graduate: odds ratio [OR]: 1.42, 95% CI: 1.19‐1.69 in 20012003; OR: 1.46, 95% CI: 1.23‐1.73 in 20072009), or specialized in family medicine (family medicine vs internal medicine: OR: 1.46, 95% CI: 1.25‐1.72 in 20012003; OR: 1.46, 95% CI: 1.25‐1.70 in 20072009). Over time, the relative odds of a patient receiving care from hospitalists decreased if their PCP was female (female vs male: OR: 1.91, 95% CI: 1.46‐2.50 in 20012003 vs OR: 1.50, 95% CI: 1.15‐1.95 in 20072009) or practiced in an urban area (largest vs smallest MSA: OR: 3.34, 95% CI: 2.72‐4.09 in 20012003; OR: 2.22, 95% CI: 1.82‐2.71 in 20072009). Although the longest‐practicing PCPs were most likely to use hospitalists in the early 2000s, this effect disappeared by 2007 to 2009 (most vs least years in practice: OR: 1.35, 95% CI: 1.06‐1.72 in 20012003 vs OR: 0.92, 95% CI: 0.73‐1.17 in 20072009).
PCP Characteristics | 20012003, OR (95% CI) | 20042006, OR (95% CI) | 20072009, OR (95% CI) |
---|---|---|---|
| |||
Family practicea vs. internal medicineb | 1.46 (1.251.72) | 1.50 (1.281.76) | 1.46 (1.251.70) |
Female vs male | 1.91 (1.462.50) | 1.43 (1.091.86) | 1.50 (1.151.95) |
United States trained (yes vs no) | 1.42 (1.191.69) | 1.53 (1.281.81) | 1.46 (1.231.73) |
Metropolitan statistical area | |||
99,999 or less | 1.00 | 1.00 | 1.00 |
100,000249,000 | 0.83 (0.651.05) | 1.00 (0.791.25) | 1.13 (0.901.41) |
250,000999,999 | 0.92 (0.721.17) | 1.03 (0.821.31) | 0.98 (0.771.23) |
1,000,000 or more | 3.34 (2.724.09) | 2.90 (2.373.54) | 2.22 (1.822.71) |
Years in practice, 2001 | |||
Q1 (lowest) | 1.00 | 1.00 | 1.00 |
Q2 | 0.89 (0.711.12) | 0.83 (0.671.04) | 0.92 (0.741.14) |
Q3 | 1.06 (0.841.34) | 0.99 (0.791.24) | 1.03 (0.821.29) |
Q4 | 1.25 (0.991.59) | 1.13 (0.891.42) | 1.15 (0.921.45) |
Q5 (highest) | 1.35 (1.061.72) | 1.05 (0.831.33) | 0.92 (0.731.17) |
Total no. of outpatient visitsc | |||
Q1 (lowest) | 1.00 | 1.00 | 1.00 |
Q2 | 1.21 (1.121.30) | 1.07 (1.001.14) | 1.13 (1.071.19) |
Q3 | 1.42 (1.301.54) | 1.18 (1.091.27) | 1.14 (1.071.22) |
Q4 | 1.34 (1.211.47) | 1.34 (1.231.46) | 1.25 (1.161.35) |
Q5 (highest) | 1.46 (1.301.63) | 1.33 (1.211.47) | 1.32 (1.201.44) |
No. of hospitalized patientsc | |||
Q1 (lowest) | 1.00 | 1.00 | 1.00 |
Q2 | 1.07 (1.001.15) | 0.91 (0.860.96) | 0.85 (0.810.89) |
Q3 | 1.00 (0.921.08) | 0.87 (0.820.93) | 0.74 (0.700.79) |
Q4 | 0.89 (0.810.97) | 0.76 (0.710.82) | 0.62 (0.580.67) |
Q5 (highest) | 1.05 (0.951.18) | 0.67 (0.610.73) | 0.55 (0.510.60) |
Average outpatient agec | |||
Q1 (lowest) | 1.00 | 1.00 | 1.00 |
Q2 | 0.94 (0.871.01) | 1.15 (1.081.23) | 1.18 (1.111.25) |
Q3 | 0.82 (0.760.90) | 1.05 (0.971.13) | 1.17 (1.091.25) |
Q4 | 0.71 (0.650.79) | 1.03 (0.951.12) | 1.10 (1.021.19) |
Q5 (highest) | 0.72 (0.640.81) | 1.12 (1.011.23) | 1.15 (1.051.26) |
Average outpatient gender (% male)c | |||
Q1 (lowest) | 1.00 | 1.00 | 1.00 |
Q2 | 1.10 (1.021.18) | 1.19 (1.101.27) | 1.27 (1.181.37) |
Q3 | 1.12 (1.031.22) | 1.27 (1.171.37) | 1.43 (1.321.54) |
Q4 | 1.36 (1.251.48) | 1.49 (1.371.61) | 1.52 (1.401.65) |
Q5 (highest) | 1.47 (1.341.61) | 1.84 (1.682.00) | 1.68 (1.541.83) |
Average outpatient race (% white)c | |||
Q1 (lowest) | 1.00 | 1.00 | 1.00 |
Q2 | 1.08 (0.981.20) | 1.01 (0.921.10) | 1.23 (1.131.34) |
Q3 | 1.27 (1.131.43) | 1.06 (0.951.18) | 1.21 (1.091.34) |
Q4 | 1.47 (1.291.67) | 0.97 (0.861.09) | 1.33 (1.181.48) |
Q5 (highest) | 1.39 (1.211.59) | 1.18 (1.041.34) | 1.25 (1.101.42) |
Average outpatient comorbidityc | |||
Q1 (lowest) | 1.00 | 1.00 | 1.00 |
Q2 | 1.26 (1.191.35) | 1.23 (1.161.31) | 1.22 (1.141.30) |
Q3 | 1.62 (1.491.75) | 1.61 (1.501.72) | 1.43 (1.341.54) |
Q4 | 1.96 (1.792.15) | 1.86 (1.722.02) | 1.59 (1.471.72) |
Q5 (highest) | 1.79 (1.592.01) | 2.20 (2.002.41) | 2.03 (1.852.22) |
In terms of PCP workload, patients of PCPs with high outpatient activity were more likely to receive hospitalists care throughout the study period, although the association had decreased by 2007 to 2009 (highest vs lowest outpatient volume: OR: 1.46, 95% CI: 1.30‐1.63 in 20012003 vs OR: 1.32, 95% CI: 1.20‐1.44 in 20072009). In contrast, PCPs with the lowest inpatient volumes became more likely to use hospitalists by the end of the study period (highest vs lowest inpatient volume: OR: 1.05, 95% CI: 0.95‐1.18 in 20012003 vs OR: 0.55, 95% CI: 0.51‐0.60 in 20072009).
The characteristics of PCPs' practice panels also were associated with patients' likelihood of receiving care from hospitalists. PCPs whose practice panels consisted of patients who were predominantly male, white, or with more outpatient comorbidities were consistently more likely to use hospitalists throughout the study period. PCPs with older patient panels were less likely to use hospitalists in 2001 to 2003, but by 2007 to 2009, they were slightly more likely to do so (oldest vs youngest average outpatient panel age: OR: 0.72, 95% CI: 0.64‐0.81 in 20012003 vs OR: 1.15, 95% CI: 1.05‐1.26 in 20072009).
CONCLUSIONS
Prior studies of the hospitalist model have shown that the likelihood of a patient receiving inpatient care from hospitalists is associated with patient characteristics, hospital characteristics, geographic region, and type of admission.[1, 16, 17] We found that PCP characteristics also predict whether patients receive care from hospitalists and that their use of hospitalists developed dynamically between 2001 to 2009. Although many factors (such as whether patients were admitted to a hospital where their PCP had admitting privileges) can influence the decision to use hospitalists, we found that over one‐third of the variance in whether a hospitalized patient received care from a hospitalist is explained by which PCP the patient saw. In showing that systemic differences exist among PCPs who use hospitalists and those who do not, our study suggests that future research on the hospitalist model should, if possible, adjust for PCP characteristics in addition to hospital and patient factors.
Although this study identifies the existence and magnitude of differences in whether or not PCPs use hospitalists, it cannot explain why the differences exist. We only can offer hypotheses. For example, our finding that PCPs with the most years of practice experience were more likely to use hospitalists in the early 2000s but not in more recent years suggests that in hospital medicine's early years, long‐practicing generalist physicians were choosing between practicing traditionalist medicine and adopting the hospitalists model, but by 2009, experienced generalist physicians had already specialized to either inpatient or outpatient settings earlier in their careers. On the other hand, the decreasing odds of urban PCPs using hospitalists may reflect a relative growth in hospitalist use in less populated areas rather than a change in urban PCPs' practice patterns.
PCPs trained in family medicine have reported less inpatient training and less comfort with providing hospital care,[18, 19] thus it is unsurprising that family physicians were more likely to refer patients to hospitalists. Although a recent study reported that family physicians' inpatient volumes remained constant, whereas those of outpatient internists declined between 2003 and 2012, the analysis used University Health Consortium data and thus reflects practice patterns in academic medical centers.[20] Our data suggest that outside of academia, family physicians have embraced the hospitalists as clinical partners.
Meltzer and Chung had previously proposed an economic model to describe the growing use of hospitalists in the United States. They posited that decisions to adopt the hospitalist model are governed by trade‐offs between coordination costs (eg, time and effort spent coordinating multiple providers across different settings) and switching costs (eg, time spent traveling between the office and the hospital or the effort of adjusting to different work settings).[16] The authors hypothesized that empirical testing of this model would show PCPs are more likely to use hospitalists if they have less available professional time (ie, work fewer hours per week), are female (due to competing demands from domestic responsibilities), have relatively few hospitalized patients, or live in areas with high traffic congestion. Our findings provide empirical evidence to support their division‐of‐labor model in showing that patients were more likely to receive hospitalist care if their PCP was female, practiced in an urban location, had higher outpatient practice volumes, or had lower inpatient volumes.
At first glance, some of our findings appear to contradict our earlier study, which showed that younger, black, male patients are more likely to receive inpatient care from hospitalists.[1] However, that study included patients regardless of whether they had a PCP. This study shows that when patients have a PCP, their PCPs are more likely to refer them to hospitalists if they are older, white, male, and have more comorbid conditions. A potential explanation for this finding is that PCPs may preferentially use hospitalists when caring for older and sicker hospitalized patients. For example, commentators often cite hospitalists' constant availability in the hospital as a valuable resource when caring for acutely ill patients.[21, 22]
Another potential explanation is that despite their preferences, PCPs who care for younger, minority patients lack access to hospitalist services. One large study of Medicare beneficiaries reported that physicians who care for black patients are less well‐trained clinically and often lack access to important clinical resources such as diagnostic imaging and nonemergency hospital admissions.[23] Similarly, international medical graduates are more likely than their US‐trained counterparts to care for underserved patients and to practice in small, independent offices.[24, 25, 26] As hospitalist groups often rely on cross‐subsidization from sources within a large healthcare organization, independent PCPs may have less access to their services when compared with PCPs in managed care organizations or large integrated groups. Viewed in this context, our findings imply that although hospitalists often care for socioeconomically vulnerable patients (eg, younger, uninsured, black men) who lack access to primary care services,[1] they also appear to share care responsibilities for more complex hospitalized patients with PCPs in more affluent communities. Further research may determine if the availability of hospitalists influences racial disparities in hospital care.
Our study has limitations. It is an observational study and thus subject to bias and confounding. As our cohort was formed using fee‐for‐service Medicare data in a single, large state, it may not be generalizable to PCPs who practice in other states, who care for a younger population, or who do not accept Medicare. Our findings also may not reflect the practice patterns of physicians‐in‐training, PCP populations with high board‐certification rates, those employed in temporary positions, or those who interrupt their practices for personal reasons, as we restricted our study to established PCPs who had been in practice long and consistently enough to be associated with 20 hospitalized patients during every year of the study. For example, the lower proportion of female PCPs in our cohort (15.6% in our study in 2009 vs 27.5% reported in a nationally representative 2008 survey[27]) may be explained by our exclusion of women who take prolonged time off for childcare duties. We also did not establish whether patient outcomes or healthcare costs differ between PCPs who adopted the hospitalist model and traditionalists. Finally, we could not examine the effect of a number of PCP factors that could plausibly influence whether or not PCPs relinquish inpatient care to hospitalists, such as their comfort with providing inpatient care, having hospital admitting privileges, having office‐based access to hospitals' electronic medical records, or the distance between their office and the hospital. However, this study lays the groundwork for future studies to explore these factors.
In summary, this study is the first, to our knowledge, to characterize PCPs who relinquished inpatient responsibilities to hospitalists. Our findings suggest that some groups of PCPs are more likely to refer patient to hospitalists, that the relationship between hospitalists and PCPs has evolved over time, and that the hospitalist model still has ample room to grow.
ACKNOWLEDGMENTS
Disclosures: This study was supported by grants from the National Institute on Aging (1RO1‐AG033134 and P30‐AG024832) and the National Cancer Institute (K05‐CA124923). The authors have no financial conflicts of interest to disclose. An oral abstract of this article was presented on May 18, 2013 at the Society of Hospital Medicine Annual Meeting in National Harbor, Maryland.
- Growth in the care of older patients by hospitalists in the United States. N Engl J Med. 2009;360(11):1102–1112. , , , .
- Effect of hospitalists on length of stay in the medicare population: variation according to hospital and patient characteristics. J Am Geriatr Soc. 2010;58(9):1649–1657. , .
- Outcomes of care by hospitalists, general internists, and family physicians. N Engl J Med. 2007;357(25):2589–2600. , , , , , .
- Hospitalist care and length of stay in patients requiring complex discharge planning and close clinical monitoring. Arch Intern Med. 2007;167(17):1869–1874. , , , , .
- The impact of hospitalists on the cost and quality of inpatient care in the United States: a research synthesis. Med Care Res Rev. 2005;62(4):379–406. , .
- Association of hospitalist care with medical utilization after discharge: evidence of cost shift from a cohort study. Ann Intern Med. 2011;155(3):152–159. , .
- Hospital care and medical utilization after discharge. Ann Intern Med. 2011;155(10):719–720; author reply 722. , .
- Hospital care and medical utilization after discharge. Ann Intern Med. 2011;155(10):721; author reply 722. .
- Administrative data algorithms can describe ambulatory physician utilization. Health Serv Res. 2007;42:1783–1796. , , , , , .
- Estimating the reliability of continuous measures with Cronbach's alpha or the intraclass correlation coefficient: toward the integration of two traditions. J Clin Epidemiol. 1991;44(4–5):381–390. , .
- Ability of Medicare claims data to identify nursing home patients: a validation study. Med Care. 2008;46(11):1184–1187. , , .
- Comorbidity measures for use with administrative data. Med Care. 1998;36(1):8–27. , , , .
- A SAS procedure based on mixture models for estimating developmental trajectories. Sociol Methods Res. 2001;29(3):374–393. , , .
- Group‐Based Modeling of Development. Cambridge, MA: Harvard University Press; 2005. .
- Group‐based trajectory modeling in clinical research. Annu Rev Clin Psychol. 2010;6:109–138. , .
- , . Coordination, switching costs and the division of labor in general medicine: an economic explanation for the emergence of hospitalists in the United States. National Bureau of Economic Research Working Paper Series No. 16040. Cambridge, MA: National Bureau of Economic Research; 2010.
- Continuity of outpatient and inpatient care by primary care physicians for hospitalized older adults. JAMA. 2009;301(16):1671–1680. , , , , , .
- Hospitalists and family physicians: understanding opportunities and risks. J Fam Pract. 2004;53(6):473–481. .
- Preparedness of internal medicine and family practice residents for treating common conditions. JAMA. 2002;288(20):2609–2614. , , , , , .
- The status of adult inpatient care by family physicians at US academic medical centers and affiliated teaching hospitals 2003 to 2012: the impact of the hospitalist movement. Fam Med. 2014;46(2):94–99. , , , .
- Hospitalists and the hospital medicine system of care are good for patient care. Arch Intern Med. 2008;168(12):1254–1256; discussion 1259–1260. .
- Hospitalists in the United States—mission accomplished or work in progress? N Engl J Med. 2004;350(19):1935–1936. .
- Primary care physicians who treat blacks and whites. N Engl J Med. 2004;351(6):575–584. , , , , .
- International medical graduates and the primary care workforce for rural underserved areas. Health Aff (Millwood). 2003;22(2):255–262. , , , .
- Medical migration and the physician workforce. International medical graduates and American medicine. JAMA. 1995;273(19):1521–1527. , , .
- International medical graduates in family medicine in the United States of America: an exploration of professional characteristics and attitudes. Hum Resour Health. 2006;4:17. , , , , .
- A snapshot of U.S. physicians: key findings from the 2008 Health Tracking Physician Survey. Data Bull (Cent Stud Health Syst Change). 2009(35):1–11. , , .
Although primary care physicians (PCPs) have traditionally treated patients in both ambulatory and hospital settings, many relinquished inpatient duties to hospitalists in recent decades.[1] Little is known about the PCPs who relinquished inpatient care duties or how the transition to the hospitalist model occurred. For example, what are the characteristics of PCPs who change? Do PCPs adopt the hospitalist model enthusiastically or cautiously? Characterizing PCPs who adopted the hospitalist model can help hospitalists understand their specialty's history and also inform health services research.
Much of the interest in the hospitalist model has been generated by studies reporting improved outcomes and lower hospital lengths of stay associated with hospitalist care.[2, 3, 4, 5] Conversely, detractors of the model point to reports of higher postacute care utilization among hospitalist patients.[6] Although these studies usually adjusted for differences among patients and hospitals, they did not account for PCP characteristics. As patients' access to PCPs and their PCP's capabilities are both plausible factors that could influence hospital length of stay (eg, decisions to complete more or less of a workup in the hospital), quality of care transitions, and postdischarge utilization, it is important to determine if PCPs who use hospitalists differ systematically from those who do not to correctly interpret health system utilization patterns that currently are attributed only to hospitalists.[7, 8]
We conducted this study to determine if observable PCP factors are associated with patients' use of hospitalists and to describe the trajectory by which PCPs referred their patients to hospitalists over time.
METHODS
Source of Data
We used claims data from 100% of Texas Medicare beneficiaries from 2000 to 2009, including Medicare beneficiary summary files, Medicare Provider Analysis and Review (MedPAR) files, Outpatient Standard Analytical Files (OutSAF), and Medicare Carrier files. Diagnosis related group (DRG)‐associated information, including weights, and Major Diagnostic Categories, were obtained from Centers for Medicare & Medicaid Services (
Establishment of the Study Cohort
Using the MedPAR file, we first selected hospital admissions from acute care hospitals in Texas for each year of the study period. We excluded beneficiaries younger than 66 years old, with incomplete Medicare Parts A and B enrollment, or with any health maintenance organization enrollment in the 12 months prior to the admission of interest. For patients with more than 1 admission in a given year, we randomly selected 1 admission. We then attempted to assign each patient to a PCP. We defined a PCP as a generalist (general practitioner, family physician, internist, or geriatrician) who saw a given beneficiary on 3 or more occasions in an outpatient setting in the year prior to the admission of interest.[9] We identified outpatient visits using Current Procedural Terminology (CPT) codes 99201 to 99205 (new patient encounters), and 99211 to 99215 (established patient encounters) from Carrier files. If more than 1 generalist physician saw the beneficiary on 3 or more occasions in a given year, the physician with more than 75% of the total outpatient evaluation and management (E&M) billings was classified as the beneficiary's PCP. Using these criteria, approximately 66% of patients were assigned to a PCP.
For cross‐sectional analyses, we restricted our cohort to beneficiaries whose PCPs were associated with at least 20 inpatients in a given year. To study trends in PCP practice patterns over time, we further restricted the cohort to beneficiaries whose PCPs were associated with at least 20 inpatients in every year of the study period, resulting in 1172 PCPs for the trajectory analyses. The reliability of PCPs' practice profiles increases as the number of patients in their panel increases. We chose 20 inpatients as the minimum because PCPs with 20 hospitalized patients per study year would achieve a reliability of 0.9 for estimating the proportion of their patients that received care from hospitalists.[10]
Identification of Hospitalists
We defined hospitalists as generalists who had at least 100 E&M billings in a given year and generated at least 90% of their total E&M billings in the year from inpatient services.[1] Inpatient E&M billings were identified by CPT codes 99221 to 99223 (new or established patient encounters), 99231 to 99233 (subsequent hospital care), and 99251 to 99255 (inpatient consultations).[1]
Patient Measures
Patient demographic information including, age at admission, gender, race/ethnicity, and Medicaid eligibility were obtained from Medicare beneficiary summary files. We used the Medicaid indicator as a proxy for low socioeconomic status. Information on weekday versus weekend admission, emergent admission, and DRG were obtained from MedPAR files. The DRG category (circulatory system, digestive system, infectious disease, nervous system, respiratory system, or other) was determined based on its Major Diagnostic Category. We determined residence in a nursing facility in the 3 months before the admission of interest from the MedPAR files and by E&M codes 99304 to 99318 (nursing facility services) from Carrier files.[11] Comorbidities were identified using the claims from MedPAR, Carrier, and OutSAF files in the year prior to the admission of interest.[12] Total hospitalizations and outpatient visits in the prior year were identified from MedPAR files and Carrier files, respectively.
PCP Measures
We categorized PCPs by specialty (general practice, gamily practice, geriatric medicine, or internal medicine), years in practice, gender, US‐ versus foreign‐trained, metropolitan statistical area (MSA) of their practice location, and board certification status. The specialty was identified from Carrier files and the other information from AMA data. For each PCP, the total number of outpatient visits and total number of patients seen as outpatients in each year was calculated based on E&M codes (9920199205, 9921199215) from Carrier files. For each year, we computed the average outpatient age, gender, race, and outpatient comorbidity for each PCP's patient panel. We computed hospital volumes using the number of hospitalized patients associated with each PCP in the study cohort.
Study Outcome
To determine whether hospitalized patients received care from hospitalists during a given hospitalization, we identified all inpatient E&M bills from generalist physicians during the admission of interest by linking MedPAR and Carrier files. If more than 50% of the generalist inpatient E&M billings from generalist physicians were from 1 or more hospitalists, the patient was considered to have received care from hospitalists.
Statistical Analyses
Multilevel analyses were used to account for the clustering of patients within PCPs. All multilevel models were adjusted for patient characteristics including age, race/ethnicity, gender, Medicaid eligibility, emergency admission, weekend admission, DRG weight, DRG category, any nursing home stay in the prior 3 months, number of comorbidities, number of hospitalizations, and number of physician visits in the year prior to the admission of interest. To analyze trends in practice patterns, we first used multilevel models to calculate the proportions of inpatients cared for by hospitalists each year for each of the 1172 PCPs with at least 20 patients. Then we employed an SAS procedure (PROC TRAJ) developed by Jones et al. to classify these PCPs into groups based on their trajectories.[13] This group‐based trajectory modeling allowed us to identify relatively homogeneous clusters within a heterogeneous sample population.[14] We chose a model that classified the PCPs into 4 groups.[15] With 4 groups, the average of the posterior probabilities of group membership for the PCPs assigned to each group exceeded 0.93, indicating a low rate of misclassification among these 4 distinct groups. For the 1172 PCPs, we tested interactions between year of hospitalization and PCP characteristics while adjusting for patient characteristics in order to investigate whether or not the impacts of PCP characteristics on how likely their patients being cared for by hospitalists differed with time. All analyses were performed with SAS version 9.2 (SAS Institute Inc., Cary, NC).
RESULTS
During the 2001 through 2009 study period, between 2252 and 2848 PCPs were associated with at least 20 hospitalized beneficiaries in any single year. Among these, 1172 PCPs were associated with at least 20 hospitalized beneficiaries in every year of the study period. These 1172 PCPs were associated with 608,686 hospitalizations over the 9 years.
Table 1 presents the characteristics of the PCPs who contributed to the cross‐sectional analyses in 2001 (N=2252) and 2009 (N=2387), as well as the 1172 PCPs for whom we had data for all 9 years for the longitudinal analyses. Most PCPs were male, trained in the United States, and were board certified. The average number of Medicare patients seen by these PCPs and number of outpatient Medicare visits went up about 7% between 2001 and 2009.
PCP Characteristics | Cross‐Sectional Analysis | Trajectory Analysis, 20012009 | |
---|---|---|---|
2001 | 2009 | ||
| |||
Overall, no. (%) | 2,252 (100%) | 2,387 (100%) | 1,172 (100%) |
Specialty, no. (%) | |||
General practice | 39 (1.7%) | 34 (1.4%) | 15 (1.3%) |
Family practice | 948 (42.1%) | 1,089 (45.6%) | 466 (39.8%) |
Internal medicine | 1,255 (55.7%) | 1,249 (52.3%) | 688 (58.7%) |
Geriatrics | 10 (0.4%) | 15 (0.6%) | 3 (0.3%) |
Gender, no. (%) | |||
Male | 1,990 (88.4%) | 2,015 (84.4%) | 1,072 (91.5%) |
Female | 262 (11.6%) | 372 (15.6%) | 100 (8.5%) |
Trained in the United States, no. (%) | |||
Yes | 1,669 (74.1%) | 1,738 (72.8%) | 844 (72.0%) |
No | 583 (25.9%) | 649 (27.2%) | 328 (28.0%) |
Metropolitan statistical area, no. (%) | |||
99,999 or less | 417 (17.5) | 237 (20.2) | |
100,000249,000 | 438 (18.3) | 234 (20.0) | |
250,000999,999 | 381 (16.0) | 216 (18.4) | |
1,000,000 or more | 1,151 (48.2) | 485 (41.4) | |
Board certification, no. (%) | |||
Yes | 1,657 (69.4%) | 800 (68.3%) | |
No | 730 (30.6%) | 372 (31.7%) | |
Years in practice, 2001, meanSD (Q1Q3) | 22.310.6 (15.028.0) | 21.28.9 (15.027.0) | |
Years in practice, 2009, meanSD (Q1Q3) | 25.010.2 (17.032.0) | 29.28.9 (23.035.0) | |
Total no. of Medicare outpatient visits, 2001, meanSD (Q1Q3) | 1,624.8879.2 (1,057.51,970.0) | 1,883.39,48.5 (1,236.52,240.5) | |
Total no. of Medicare outpatient visits, 2009, meanSD (Q1Q3) | 1,733.81,053.3 (1,080.02,048.0) | 2,020.51,200.9 (1,334.52,373.0) | |
Total no. of Medicare outpatients, 2001, meanSD (Q1Q3) | 418.6186.9 (284.0522.0) | 473.4189.5 (338.0580.5) | |
Total no. of Medicare outpatients, 2009, meanSD (Q1Q3) | 448.7217.8 (300.0548.0) | 508.7238.2 (350.5615.0) | |
No. of hospitalized patients, 2001, meanSD (Q1Q3) | 46.025.0 (27.057.0) | 53.028.0 (32.066.0) | |
No. of hospitalized patients, 2009, meanSD (Q1Q3) | 44.024.0 (26.052.0) | 52.027.0 (33.065.0) | |
Average outpatient age, 2001, meanSD (Q1Q3) | 72.82.3 (71.574.2) | 72.82.1 (71.774.1) | |
Average outpatient age, 2009, meanSD (Q1Q3) | 72.12.8 (70.673.9) | 72.82.7 (71.474.5) | |
Average outpatient gender (% male), 2001, meanSD (Q1Q3) | 38.17.0 (35.542.3) | 38.56.4 (36.242.3) | |
Average outpatient gender (% male), 2009, meanSD (Q1Q3) | 40.27.6 (37.644.8) | 41.06.5 (38.644.8) | |
Average outpatient race (% white), 2001, meanSD (Q1Q3) | 84.316.4 (79.295.5) | 85.414.3 (79.995.7) | |
Average outpatient race (% white), 2009, meanSD (Q1Q3) | 85.214.4 (79.895.2) | 86.312.9 (80.895.6) | |
Average outpatient comorbidity, 2001, meanSD (Q1Q3)a | 1.60.5 (1.21.8) | 1.60.4 (1.21.8) | |
Average outpatient comorbidity, 2009, meanSD (Q1Q3)a | 2.20.6 (1.82.5) | 2.20.6 (1.72.5) |
Figure 1 graphs the percentage of PCPs as a function of what percent of their hospitalized patients received care from hospitalists, and how that changed from 2001 to 2009. For 70.9% of PCPs, fewer than 5% of their hospitalized patients received hospitalist care in 2001. By 2009, the percent of PCPs in this category had decreased to 15.2%. In contrast, in 2001, more than half of the patients for 2.1% of PCPs received hospitalist care, and the percent of PCPs in this category increased to 26.3% by 2009.
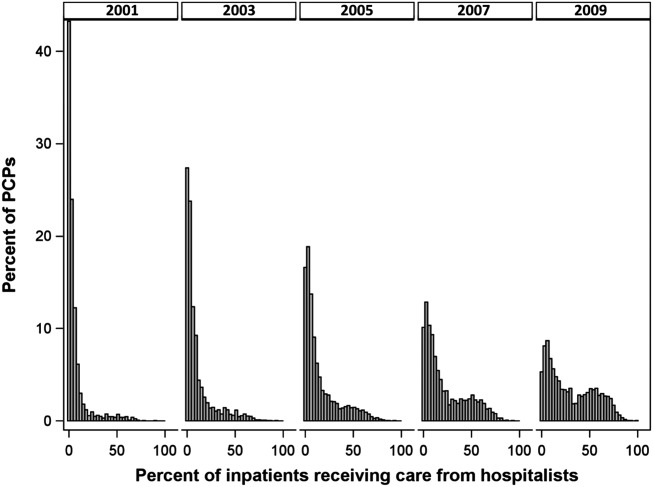
The pattern in Figure 1 shows that PCPs' use of hospitalists changed continuously and gradually over time. However, this pattern describes the PCPs as a group. When examined at the individual PCP level, different patterns emerge. Figure 2, which presents selected individual PCP's use of hospitalists over time, shows several distinct subpatterns of PCP practice behaviors. First, there are PCPs whose use of hospitalists was high in 2001 and stayed high or increased over time (eg, PCP A). There also were PCPs whose use of hospitalists stayed low over the entire study period (eg, PCP B). Finally, there were PCPs whose use of hospitalists was low in 2001 but high in 2009 (eg, PCP C). For this last group, the pattern of change in hospitalist utilization over time was discontinuous; that is, most of the increase occurred over a 1‐ or 2‐year period, instead of increasing gradually over time.
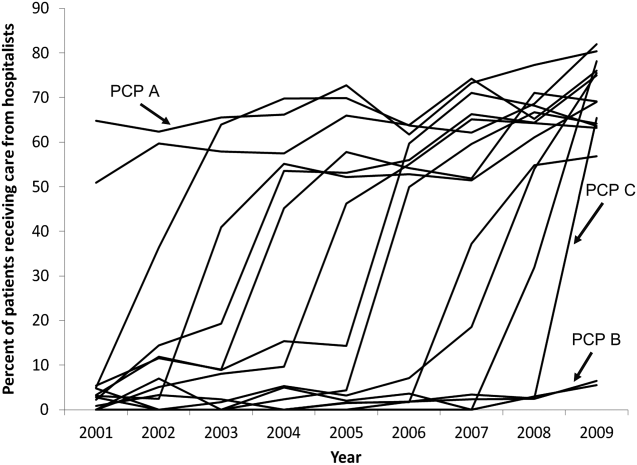
Among the 1172 PCPs associated with 20 hospitalized beneficiaries each year in all 9 years of the study period, group‐based trajectory modeling classified their practice patterns into 4 distinct trajectories (Figure 3). Among PCPs in group 1, more than one‐third of their hospitalized patients were cared for by hospitalists in 2001, and this increased to 60% by 2009. PCPs in groups 2 and 3 rarely used hospitalist care in 2001 but increased their use over time. The increase started early in the period for PCPs in group 2 and later for those in group 3. PCPs in group 4 were associated with little hospitalist use throughout the study period.
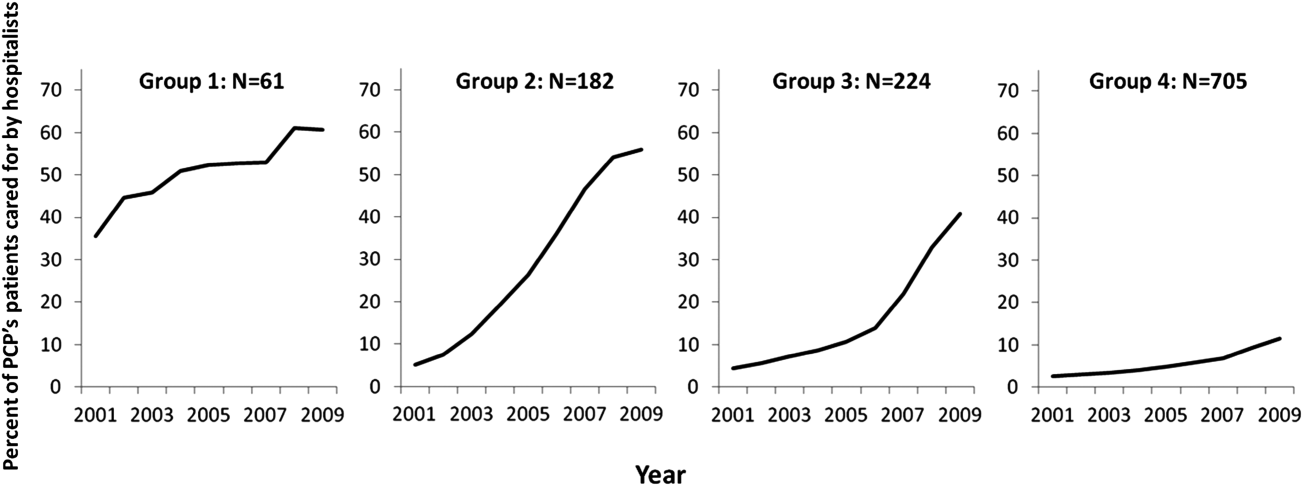
We constructed a model to describe the odds of a patient receiving care from hospitalists during the study period using patients associated with these 1172 PCPs. After adjusting for patient characteristics, the residual intraclass correlation coefficient for PCP level was 0.334, which indicates that 33.4% of the variance in whether a hospitalized patient received care from a hospitalist is explained by which PCP the patient saw. When adjusting for both patient and PCP characteristics, the overall odds of a patient receiving hospitalist care increased by 30% (95% confidence interval [CI]: 1.29‐1.30) per year from 2001 through 2009.
There were also significant interactions between year of hospitalization and several PCP characteristics. These interactions are illustrated in Table 2, which stratifies each of those PCP characteristics by 3 time periods: 2001 to 2003, 2004 to 2006, and 2007 to 2009. In all time periods, patients were more likely to receive hospitalist care if their PCP was US trained (US vs international medical graduate: odds ratio [OR]: 1.42, 95% CI: 1.19‐1.69 in 20012003; OR: 1.46, 95% CI: 1.23‐1.73 in 20072009), or specialized in family medicine (family medicine vs internal medicine: OR: 1.46, 95% CI: 1.25‐1.72 in 20012003; OR: 1.46, 95% CI: 1.25‐1.70 in 20072009). Over time, the relative odds of a patient receiving care from hospitalists decreased if their PCP was female (female vs male: OR: 1.91, 95% CI: 1.46‐2.50 in 20012003 vs OR: 1.50, 95% CI: 1.15‐1.95 in 20072009) or practiced in an urban area (largest vs smallest MSA: OR: 3.34, 95% CI: 2.72‐4.09 in 20012003; OR: 2.22, 95% CI: 1.82‐2.71 in 20072009). Although the longest‐practicing PCPs were most likely to use hospitalists in the early 2000s, this effect disappeared by 2007 to 2009 (most vs least years in practice: OR: 1.35, 95% CI: 1.06‐1.72 in 20012003 vs OR: 0.92, 95% CI: 0.73‐1.17 in 20072009).
PCP Characteristics | 20012003, OR (95% CI) | 20042006, OR (95% CI) | 20072009, OR (95% CI) |
---|---|---|---|
| |||
Family practicea vs. internal medicineb | 1.46 (1.251.72) | 1.50 (1.281.76) | 1.46 (1.251.70) |
Female vs male | 1.91 (1.462.50) | 1.43 (1.091.86) | 1.50 (1.151.95) |
United States trained (yes vs no) | 1.42 (1.191.69) | 1.53 (1.281.81) | 1.46 (1.231.73) |
Metropolitan statistical area | |||
99,999 or less | 1.00 | 1.00 | 1.00 |
100,000249,000 | 0.83 (0.651.05) | 1.00 (0.791.25) | 1.13 (0.901.41) |
250,000999,999 | 0.92 (0.721.17) | 1.03 (0.821.31) | 0.98 (0.771.23) |
1,000,000 or more | 3.34 (2.724.09) | 2.90 (2.373.54) | 2.22 (1.822.71) |
Years in practice, 2001 | |||
Q1 (lowest) | 1.00 | 1.00 | 1.00 |
Q2 | 0.89 (0.711.12) | 0.83 (0.671.04) | 0.92 (0.741.14) |
Q3 | 1.06 (0.841.34) | 0.99 (0.791.24) | 1.03 (0.821.29) |
Q4 | 1.25 (0.991.59) | 1.13 (0.891.42) | 1.15 (0.921.45) |
Q5 (highest) | 1.35 (1.061.72) | 1.05 (0.831.33) | 0.92 (0.731.17) |
Total no. of outpatient visitsc | |||
Q1 (lowest) | 1.00 | 1.00 | 1.00 |
Q2 | 1.21 (1.121.30) | 1.07 (1.001.14) | 1.13 (1.071.19) |
Q3 | 1.42 (1.301.54) | 1.18 (1.091.27) | 1.14 (1.071.22) |
Q4 | 1.34 (1.211.47) | 1.34 (1.231.46) | 1.25 (1.161.35) |
Q5 (highest) | 1.46 (1.301.63) | 1.33 (1.211.47) | 1.32 (1.201.44) |
No. of hospitalized patientsc | |||
Q1 (lowest) | 1.00 | 1.00 | 1.00 |
Q2 | 1.07 (1.001.15) | 0.91 (0.860.96) | 0.85 (0.810.89) |
Q3 | 1.00 (0.921.08) | 0.87 (0.820.93) | 0.74 (0.700.79) |
Q4 | 0.89 (0.810.97) | 0.76 (0.710.82) | 0.62 (0.580.67) |
Q5 (highest) | 1.05 (0.951.18) | 0.67 (0.610.73) | 0.55 (0.510.60) |
Average outpatient agec | |||
Q1 (lowest) | 1.00 | 1.00 | 1.00 |
Q2 | 0.94 (0.871.01) | 1.15 (1.081.23) | 1.18 (1.111.25) |
Q3 | 0.82 (0.760.90) | 1.05 (0.971.13) | 1.17 (1.091.25) |
Q4 | 0.71 (0.650.79) | 1.03 (0.951.12) | 1.10 (1.021.19) |
Q5 (highest) | 0.72 (0.640.81) | 1.12 (1.011.23) | 1.15 (1.051.26) |
Average outpatient gender (% male)c | |||
Q1 (lowest) | 1.00 | 1.00 | 1.00 |
Q2 | 1.10 (1.021.18) | 1.19 (1.101.27) | 1.27 (1.181.37) |
Q3 | 1.12 (1.031.22) | 1.27 (1.171.37) | 1.43 (1.321.54) |
Q4 | 1.36 (1.251.48) | 1.49 (1.371.61) | 1.52 (1.401.65) |
Q5 (highest) | 1.47 (1.341.61) | 1.84 (1.682.00) | 1.68 (1.541.83) |
Average outpatient race (% white)c | |||
Q1 (lowest) | 1.00 | 1.00 | 1.00 |
Q2 | 1.08 (0.981.20) | 1.01 (0.921.10) | 1.23 (1.131.34) |
Q3 | 1.27 (1.131.43) | 1.06 (0.951.18) | 1.21 (1.091.34) |
Q4 | 1.47 (1.291.67) | 0.97 (0.861.09) | 1.33 (1.181.48) |
Q5 (highest) | 1.39 (1.211.59) | 1.18 (1.041.34) | 1.25 (1.101.42) |
Average outpatient comorbidityc | |||
Q1 (lowest) | 1.00 | 1.00 | 1.00 |
Q2 | 1.26 (1.191.35) | 1.23 (1.161.31) | 1.22 (1.141.30) |
Q3 | 1.62 (1.491.75) | 1.61 (1.501.72) | 1.43 (1.341.54) |
Q4 | 1.96 (1.792.15) | 1.86 (1.722.02) | 1.59 (1.471.72) |
Q5 (highest) | 1.79 (1.592.01) | 2.20 (2.002.41) | 2.03 (1.852.22) |
In terms of PCP workload, patients of PCPs with high outpatient activity were more likely to receive hospitalists care throughout the study period, although the association had decreased by 2007 to 2009 (highest vs lowest outpatient volume: OR: 1.46, 95% CI: 1.30‐1.63 in 20012003 vs OR: 1.32, 95% CI: 1.20‐1.44 in 20072009). In contrast, PCPs with the lowest inpatient volumes became more likely to use hospitalists by the end of the study period (highest vs lowest inpatient volume: OR: 1.05, 95% CI: 0.95‐1.18 in 20012003 vs OR: 0.55, 95% CI: 0.51‐0.60 in 20072009).
The characteristics of PCPs' practice panels also were associated with patients' likelihood of receiving care from hospitalists. PCPs whose practice panels consisted of patients who were predominantly male, white, or with more outpatient comorbidities were consistently more likely to use hospitalists throughout the study period. PCPs with older patient panels were less likely to use hospitalists in 2001 to 2003, but by 2007 to 2009, they were slightly more likely to do so (oldest vs youngest average outpatient panel age: OR: 0.72, 95% CI: 0.64‐0.81 in 20012003 vs OR: 1.15, 95% CI: 1.05‐1.26 in 20072009).
CONCLUSIONS
Prior studies of the hospitalist model have shown that the likelihood of a patient receiving inpatient care from hospitalists is associated with patient characteristics, hospital characteristics, geographic region, and type of admission.[1, 16, 17] We found that PCP characteristics also predict whether patients receive care from hospitalists and that their use of hospitalists developed dynamically between 2001 to 2009. Although many factors (such as whether patients were admitted to a hospital where their PCP had admitting privileges) can influence the decision to use hospitalists, we found that over one‐third of the variance in whether a hospitalized patient received care from a hospitalist is explained by which PCP the patient saw. In showing that systemic differences exist among PCPs who use hospitalists and those who do not, our study suggests that future research on the hospitalist model should, if possible, adjust for PCP characteristics in addition to hospital and patient factors.
Although this study identifies the existence and magnitude of differences in whether or not PCPs use hospitalists, it cannot explain why the differences exist. We only can offer hypotheses. For example, our finding that PCPs with the most years of practice experience were more likely to use hospitalists in the early 2000s but not in more recent years suggests that in hospital medicine's early years, long‐practicing generalist physicians were choosing between practicing traditionalist medicine and adopting the hospitalists model, but by 2009, experienced generalist physicians had already specialized to either inpatient or outpatient settings earlier in their careers. On the other hand, the decreasing odds of urban PCPs using hospitalists may reflect a relative growth in hospitalist use in less populated areas rather than a change in urban PCPs' practice patterns.
PCPs trained in family medicine have reported less inpatient training and less comfort with providing hospital care,[18, 19] thus it is unsurprising that family physicians were more likely to refer patients to hospitalists. Although a recent study reported that family physicians' inpatient volumes remained constant, whereas those of outpatient internists declined between 2003 and 2012, the analysis used University Health Consortium data and thus reflects practice patterns in academic medical centers.[20] Our data suggest that outside of academia, family physicians have embraced the hospitalists as clinical partners.
Meltzer and Chung had previously proposed an economic model to describe the growing use of hospitalists in the United States. They posited that decisions to adopt the hospitalist model are governed by trade‐offs between coordination costs (eg, time and effort spent coordinating multiple providers across different settings) and switching costs (eg, time spent traveling between the office and the hospital or the effort of adjusting to different work settings).[16] The authors hypothesized that empirical testing of this model would show PCPs are more likely to use hospitalists if they have less available professional time (ie, work fewer hours per week), are female (due to competing demands from domestic responsibilities), have relatively few hospitalized patients, or live in areas with high traffic congestion. Our findings provide empirical evidence to support their division‐of‐labor model in showing that patients were more likely to receive hospitalist care if their PCP was female, practiced in an urban location, had higher outpatient practice volumes, or had lower inpatient volumes.
At first glance, some of our findings appear to contradict our earlier study, which showed that younger, black, male patients are more likely to receive inpatient care from hospitalists.[1] However, that study included patients regardless of whether they had a PCP. This study shows that when patients have a PCP, their PCPs are more likely to refer them to hospitalists if they are older, white, male, and have more comorbid conditions. A potential explanation for this finding is that PCPs may preferentially use hospitalists when caring for older and sicker hospitalized patients. For example, commentators often cite hospitalists' constant availability in the hospital as a valuable resource when caring for acutely ill patients.[21, 22]
Another potential explanation is that despite their preferences, PCPs who care for younger, minority patients lack access to hospitalist services. One large study of Medicare beneficiaries reported that physicians who care for black patients are less well‐trained clinically and often lack access to important clinical resources such as diagnostic imaging and nonemergency hospital admissions.[23] Similarly, international medical graduates are more likely than their US‐trained counterparts to care for underserved patients and to practice in small, independent offices.[24, 25, 26] As hospitalist groups often rely on cross‐subsidization from sources within a large healthcare organization, independent PCPs may have less access to their services when compared with PCPs in managed care organizations or large integrated groups. Viewed in this context, our findings imply that although hospitalists often care for socioeconomically vulnerable patients (eg, younger, uninsured, black men) who lack access to primary care services,[1] they also appear to share care responsibilities for more complex hospitalized patients with PCPs in more affluent communities. Further research may determine if the availability of hospitalists influences racial disparities in hospital care.
Our study has limitations. It is an observational study and thus subject to bias and confounding. As our cohort was formed using fee‐for‐service Medicare data in a single, large state, it may not be generalizable to PCPs who practice in other states, who care for a younger population, or who do not accept Medicare. Our findings also may not reflect the practice patterns of physicians‐in‐training, PCP populations with high board‐certification rates, those employed in temporary positions, or those who interrupt their practices for personal reasons, as we restricted our study to established PCPs who had been in practice long and consistently enough to be associated with 20 hospitalized patients during every year of the study. For example, the lower proportion of female PCPs in our cohort (15.6% in our study in 2009 vs 27.5% reported in a nationally representative 2008 survey[27]) may be explained by our exclusion of women who take prolonged time off for childcare duties. We also did not establish whether patient outcomes or healthcare costs differ between PCPs who adopted the hospitalist model and traditionalists. Finally, we could not examine the effect of a number of PCP factors that could plausibly influence whether or not PCPs relinquish inpatient care to hospitalists, such as their comfort with providing inpatient care, having hospital admitting privileges, having office‐based access to hospitals' electronic medical records, or the distance between their office and the hospital. However, this study lays the groundwork for future studies to explore these factors.
In summary, this study is the first, to our knowledge, to characterize PCPs who relinquished inpatient responsibilities to hospitalists. Our findings suggest that some groups of PCPs are more likely to refer patient to hospitalists, that the relationship between hospitalists and PCPs has evolved over time, and that the hospitalist model still has ample room to grow.
ACKNOWLEDGMENTS
Disclosures: This study was supported by grants from the National Institute on Aging (1RO1‐AG033134 and P30‐AG024832) and the National Cancer Institute (K05‐CA124923). The authors have no financial conflicts of interest to disclose. An oral abstract of this article was presented on May 18, 2013 at the Society of Hospital Medicine Annual Meeting in National Harbor, Maryland.
Although primary care physicians (PCPs) have traditionally treated patients in both ambulatory and hospital settings, many relinquished inpatient duties to hospitalists in recent decades.[1] Little is known about the PCPs who relinquished inpatient care duties or how the transition to the hospitalist model occurred. For example, what are the characteristics of PCPs who change? Do PCPs adopt the hospitalist model enthusiastically or cautiously? Characterizing PCPs who adopted the hospitalist model can help hospitalists understand their specialty's history and also inform health services research.
Much of the interest in the hospitalist model has been generated by studies reporting improved outcomes and lower hospital lengths of stay associated with hospitalist care.[2, 3, 4, 5] Conversely, detractors of the model point to reports of higher postacute care utilization among hospitalist patients.[6] Although these studies usually adjusted for differences among patients and hospitals, they did not account for PCP characteristics. As patients' access to PCPs and their PCP's capabilities are both plausible factors that could influence hospital length of stay (eg, decisions to complete more or less of a workup in the hospital), quality of care transitions, and postdischarge utilization, it is important to determine if PCPs who use hospitalists differ systematically from those who do not to correctly interpret health system utilization patterns that currently are attributed only to hospitalists.[7, 8]
We conducted this study to determine if observable PCP factors are associated with patients' use of hospitalists and to describe the trajectory by which PCPs referred their patients to hospitalists over time.
METHODS
Source of Data
We used claims data from 100% of Texas Medicare beneficiaries from 2000 to 2009, including Medicare beneficiary summary files, Medicare Provider Analysis and Review (MedPAR) files, Outpatient Standard Analytical Files (OutSAF), and Medicare Carrier files. Diagnosis related group (DRG)‐associated information, including weights, and Major Diagnostic Categories, were obtained from Centers for Medicare & Medicaid Services (
Establishment of the Study Cohort
Using the MedPAR file, we first selected hospital admissions from acute care hospitals in Texas for each year of the study period. We excluded beneficiaries younger than 66 years old, with incomplete Medicare Parts A and B enrollment, or with any health maintenance organization enrollment in the 12 months prior to the admission of interest. For patients with more than 1 admission in a given year, we randomly selected 1 admission. We then attempted to assign each patient to a PCP. We defined a PCP as a generalist (general practitioner, family physician, internist, or geriatrician) who saw a given beneficiary on 3 or more occasions in an outpatient setting in the year prior to the admission of interest.[9] We identified outpatient visits using Current Procedural Terminology (CPT) codes 99201 to 99205 (new patient encounters), and 99211 to 99215 (established patient encounters) from Carrier files. If more than 1 generalist physician saw the beneficiary on 3 or more occasions in a given year, the physician with more than 75% of the total outpatient evaluation and management (E&M) billings was classified as the beneficiary's PCP. Using these criteria, approximately 66% of patients were assigned to a PCP.
For cross‐sectional analyses, we restricted our cohort to beneficiaries whose PCPs were associated with at least 20 inpatients in a given year. To study trends in PCP practice patterns over time, we further restricted the cohort to beneficiaries whose PCPs were associated with at least 20 inpatients in every year of the study period, resulting in 1172 PCPs for the trajectory analyses. The reliability of PCPs' practice profiles increases as the number of patients in their panel increases. We chose 20 inpatients as the minimum because PCPs with 20 hospitalized patients per study year would achieve a reliability of 0.9 for estimating the proportion of their patients that received care from hospitalists.[10]
Identification of Hospitalists
We defined hospitalists as generalists who had at least 100 E&M billings in a given year and generated at least 90% of their total E&M billings in the year from inpatient services.[1] Inpatient E&M billings were identified by CPT codes 99221 to 99223 (new or established patient encounters), 99231 to 99233 (subsequent hospital care), and 99251 to 99255 (inpatient consultations).[1]
Patient Measures
Patient demographic information including, age at admission, gender, race/ethnicity, and Medicaid eligibility were obtained from Medicare beneficiary summary files. We used the Medicaid indicator as a proxy for low socioeconomic status. Information on weekday versus weekend admission, emergent admission, and DRG were obtained from MedPAR files. The DRG category (circulatory system, digestive system, infectious disease, nervous system, respiratory system, or other) was determined based on its Major Diagnostic Category. We determined residence in a nursing facility in the 3 months before the admission of interest from the MedPAR files and by E&M codes 99304 to 99318 (nursing facility services) from Carrier files.[11] Comorbidities were identified using the claims from MedPAR, Carrier, and OutSAF files in the year prior to the admission of interest.[12] Total hospitalizations and outpatient visits in the prior year were identified from MedPAR files and Carrier files, respectively.
PCP Measures
We categorized PCPs by specialty (general practice, gamily practice, geriatric medicine, or internal medicine), years in practice, gender, US‐ versus foreign‐trained, metropolitan statistical area (MSA) of their practice location, and board certification status. The specialty was identified from Carrier files and the other information from AMA data. For each PCP, the total number of outpatient visits and total number of patients seen as outpatients in each year was calculated based on E&M codes (9920199205, 9921199215) from Carrier files. For each year, we computed the average outpatient age, gender, race, and outpatient comorbidity for each PCP's patient panel. We computed hospital volumes using the number of hospitalized patients associated with each PCP in the study cohort.
Study Outcome
To determine whether hospitalized patients received care from hospitalists during a given hospitalization, we identified all inpatient E&M bills from generalist physicians during the admission of interest by linking MedPAR and Carrier files. If more than 50% of the generalist inpatient E&M billings from generalist physicians were from 1 or more hospitalists, the patient was considered to have received care from hospitalists.
Statistical Analyses
Multilevel analyses were used to account for the clustering of patients within PCPs. All multilevel models were adjusted for patient characteristics including age, race/ethnicity, gender, Medicaid eligibility, emergency admission, weekend admission, DRG weight, DRG category, any nursing home stay in the prior 3 months, number of comorbidities, number of hospitalizations, and number of physician visits in the year prior to the admission of interest. To analyze trends in practice patterns, we first used multilevel models to calculate the proportions of inpatients cared for by hospitalists each year for each of the 1172 PCPs with at least 20 patients. Then we employed an SAS procedure (PROC TRAJ) developed by Jones et al. to classify these PCPs into groups based on their trajectories.[13] This group‐based trajectory modeling allowed us to identify relatively homogeneous clusters within a heterogeneous sample population.[14] We chose a model that classified the PCPs into 4 groups.[15] With 4 groups, the average of the posterior probabilities of group membership for the PCPs assigned to each group exceeded 0.93, indicating a low rate of misclassification among these 4 distinct groups. For the 1172 PCPs, we tested interactions between year of hospitalization and PCP characteristics while adjusting for patient characteristics in order to investigate whether or not the impacts of PCP characteristics on how likely their patients being cared for by hospitalists differed with time. All analyses were performed with SAS version 9.2 (SAS Institute Inc., Cary, NC).
RESULTS
During the 2001 through 2009 study period, between 2252 and 2848 PCPs were associated with at least 20 hospitalized beneficiaries in any single year. Among these, 1172 PCPs were associated with at least 20 hospitalized beneficiaries in every year of the study period. These 1172 PCPs were associated with 608,686 hospitalizations over the 9 years.
Table 1 presents the characteristics of the PCPs who contributed to the cross‐sectional analyses in 2001 (N=2252) and 2009 (N=2387), as well as the 1172 PCPs for whom we had data for all 9 years for the longitudinal analyses. Most PCPs were male, trained in the United States, and were board certified. The average number of Medicare patients seen by these PCPs and number of outpatient Medicare visits went up about 7% between 2001 and 2009.
PCP Characteristics | Cross‐Sectional Analysis | Trajectory Analysis, 20012009 | |
---|---|---|---|
2001 | 2009 | ||
| |||
Overall, no. (%) | 2,252 (100%) | 2,387 (100%) | 1,172 (100%) |
Specialty, no. (%) | |||
General practice | 39 (1.7%) | 34 (1.4%) | 15 (1.3%) |
Family practice | 948 (42.1%) | 1,089 (45.6%) | 466 (39.8%) |
Internal medicine | 1,255 (55.7%) | 1,249 (52.3%) | 688 (58.7%) |
Geriatrics | 10 (0.4%) | 15 (0.6%) | 3 (0.3%) |
Gender, no. (%) | |||
Male | 1,990 (88.4%) | 2,015 (84.4%) | 1,072 (91.5%) |
Female | 262 (11.6%) | 372 (15.6%) | 100 (8.5%) |
Trained in the United States, no. (%) | |||
Yes | 1,669 (74.1%) | 1,738 (72.8%) | 844 (72.0%) |
No | 583 (25.9%) | 649 (27.2%) | 328 (28.0%) |
Metropolitan statistical area, no. (%) | |||
99,999 or less | 417 (17.5) | 237 (20.2) | |
100,000249,000 | 438 (18.3) | 234 (20.0) | |
250,000999,999 | 381 (16.0) | 216 (18.4) | |
1,000,000 or more | 1,151 (48.2) | 485 (41.4) | |
Board certification, no. (%) | |||
Yes | 1,657 (69.4%) | 800 (68.3%) | |
No | 730 (30.6%) | 372 (31.7%) | |
Years in practice, 2001, meanSD (Q1Q3) | 22.310.6 (15.028.0) | 21.28.9 (15.027.0) | |
Years in practice, 2009, meanSD (Q1Q3) | 25.010.2 (17.032.0) | 29.28.9 (23.035.0) | |
Total no. of Medicare outpatient visits, 2001, meanSD (Q1Q3) | 1,624.8879.2 (1,057.51,970.0) | 1,883.39,48.5 (1,236.52,240.5) | |
Total no. of Medicare outpatient visits, 2009, meanSD (Q1Q3) | 1,733.81,053.3 (1,080.02,048.0) | 2,020.51,200.9 (1,334.52,373.0) | |
Total no. of Medicare outpatients, 2001, meanSD (Q1Q3) | 418.6186.9 (284.0522.0) | 473.4189.5 (338.0580.5) | |
Total no. of Medicare outpatients, 2009, meanSD (Q1Q3) | 448.7217.8 (300.0548.0) | 508.7238.2 (350.5615.0) | |
No. of hospitalized patients, 2001, meanSD (Q1Q3) | 46.025.0 (27.057.0) | 53.028.0 (32.066.0) | |
No. of hospitalized patients, 2009, meanSD (Q1Q3) | 44.024.0 (26.052.0) | 52.027.0 (33.065.0) | |
Average outpatient age, 2001, meanSD (Q1Q3) | 72.82.3 (71.574.2) | 72.82.1 (71.774.1) | |
Average outpatient age, 2009, meanSD (Q1Q3) | 72.12.8 (70.673.9) | 72.82.7 (71.474.5) | |
Average outpatient gender (% male), 2001, meanSD (Q1Q3) | 38.17.0 (35.542.3) | 38.56.4 (36.242.3) | |
Average outpatient gender (% male), 2009, meanSD (Q1Q3) | 40.27.6 (37.644.8) | 41.06.5 (38.644.8) | |
Average outpatient race (% white), 2001, meanSD (Q1Q3) | 84.316.4 (79.295.5) | 85.414.3 (79.995.7) | |
Average outpatient race (% white), 2009, meanSD (Q1Q3) | 85.214.4 (79.895.2) | 86.312.9 (80.895.6) | |
Average outpatient comorbidity, 2001, meanSD (Q1Q3)a | 1.60.5 (1.21.8) | 1.60.4 (1.21.8) | |
Average outpatient comorbidity, 2009, meanSD (Q1Q3)a | 2.20.6 (1.82.5) | 2.20.6 (1.72.5) |
Figure 1 graphs the percentage of PCPs as a function of what percent of their hospitalized patients received care from hospitalists, and how that changed from 2001 to 2009. For 70.9% of PCPs, fewer than 5% of their hospitalized patients received hospitalist care in 2001. By 2009, the percent of PCPs in this category had decreased to 15.2%. In contrast, in 2001, more than half of the patients for 2.1% of PCPs received hospitalist care, and the percent of PCPs in this category increased to 26.3% by 2009.
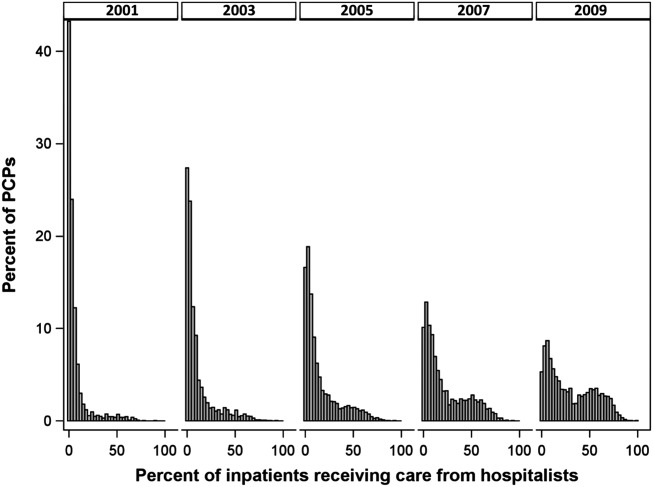
The pattern in Figure 1 shows that PCPs' use of hospitalists changed continuously and gradually over time. However, this pattern describes the PCPs as a group. When examined at the individual PCP level, different patterns emerge. Figure 2, which presents selected individual PCP's use of hospitalists over time, shows several distinct subpatterns of PCP practice behaviors. First, there are PCPs whose use of hospitalists was high in 2001 and stayed high or increased over time (eg, PCP A). There also were PCPs whose use of hospitalists stayed low over the entire study period (eg, PCP B). Finally, there were PCPs whose use of hospitalists was low in 2001 but high in 2009 (eg, PCP C). For this last group, the pattern of change in hospitalist utilization over time was discontinuous; that is, most of the increase occurred over a 1‐ or 2‐year period, instead of increasing gradually over time.
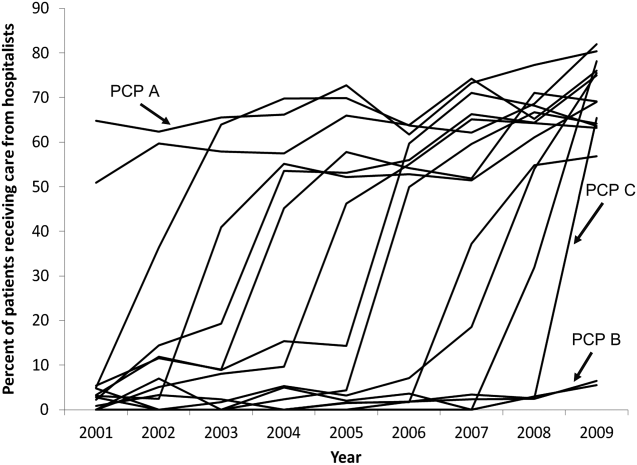
Among the 1172 PCPs associated with 20 hospitalized beneficiaries each year in all 9 years of the study period, group‐based trajectory modeling classified their practice patterns into 4 distinct trajectories (Figure 3). Among PCPs in group 1, more than one‐third of their hospitalized patients were cared for by hospitalists in 2001, and this increased to 60% by 2009. PCPs in groups 2 and 3 rarely used hospitalist care in 2001 but increased their use over time. The increase started early in the period for PCPs in group 2 and later for those in group 3. PCPs in group 4 were associated with little hospitalist use throughout the study period.
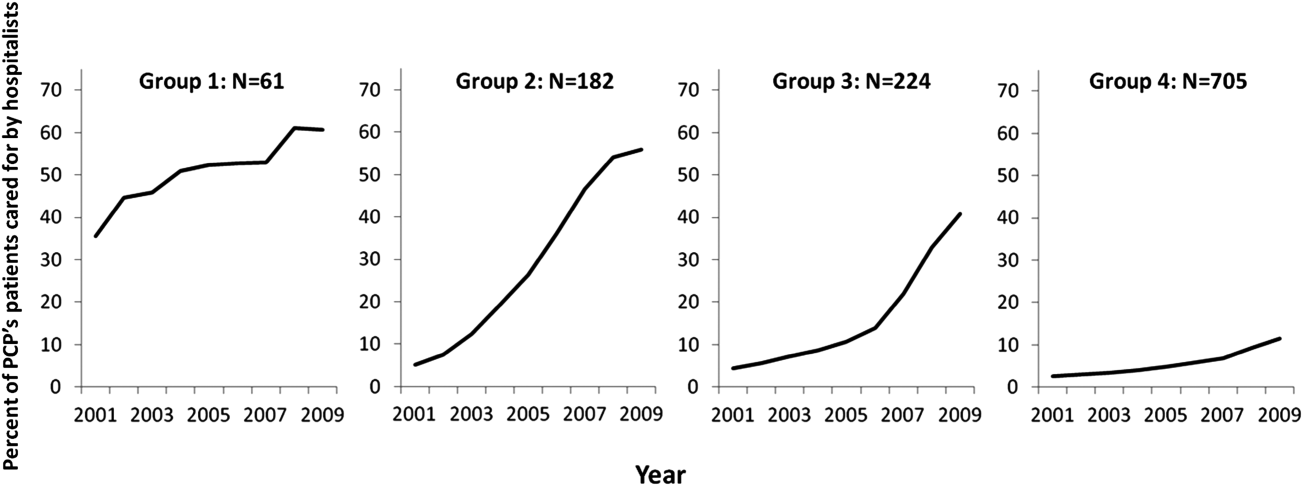
We constructed a model to describe the odds of a patient receiving care from hospitalists during the study period using patients associated with these 1172 PCPs. After adjusting for patient characteristics, the residual intraclass correlation coefficient for PCP level was 0.334, which indicates that 33.4% of the variance in whether a hospitalized patient received care from a hospitalist is explained by which PCP the patient saw. When adjusting for both patient and PCP characteristics, the overall odds of a patient receiving hospitalist care increased by 30% (95% confidence interval [CI]: 1.29‐1.30) per year from 2001 through 2009.
There were also significant interactions between year of hospitalization and several PCP characteristics. These interactions are illustrated in Table 2, which stratifies each of those PCP characteristics by 3 time periods: 2001 to 2003, 2004 to 2006, and 2007 to 2009. In all time periods, patients were more likely to receive hospitalist care if their PCP was US trained (US vs international medical graduate: odds ratio [OR]: 1.42, 95% CI: 1.19‐1.69 in 20012003; OR: 1.46, 95% CI: 1.23‐1.73 in 20072009), or specialized in family medicine (family medicine vs internal medicine: OR: 1.46, 95% CI: 1.25‐1.72 in 20012003; OR: 1.46, 95% CI: 1.25‐1.70 in 20072009). Over time, the relative odds of a patient receiving care from hospitalists decreased if their PCP was female (female vs male: OR: 1.91, 95% CI: 1.46‐2.50 in 20012003 vs OR: 1.50, 95% CI: 1.15‐1.95 in 20072009) or practiced in an urban area (largest vs smallest MSA: OR: 3.34, 95% CI: 2.72‐4.09 in 20012003; OR: 2.22, 95% CI: 1.82‐2.71 in 20072009). Although the longest‐practicing PCPs were most likely to use hospitalists in the early 2000s, this effect disappeared by 2007 to 2009 (most vs least years in practice: OR: 1.35, 95% CI: 1.06‐1.72 in 20012003 vs OR: 0.92, 95% CI: 0.73‐1.17 in 20072009).
PCP Characteristics | 20012003, OR (95% CI) | 20042006, OR (95% CI) | 20072009, OR (95% CI) |
---|---|---|---|
| |||
Family practicea vs. internal medicineb | 1.46 (1.251.72) | 1.50 (1.281.76) | 1.46 (1.251.70) |
Female vs male | 1.91 (1.462.50) | 1.43 (1.091.86) | 1.50 (1.151.95) |
United States trained (yes vs no) | 1.42 (1.191.69) | 1.53 (1.281.81) | 1.46 (1.231.73) |
Metropolitan statistical area | |||
99,999 or less | 1.00 | 1.00 | 1.00 |
100,000249,000 | 0.83 (0.651.05) | 1.00 (0.791.25) | 1.13 (0.901.41) |
250,000999,999 | 0.92 (0.721.17) | 1.03 (0.821.31) | 0.98 (0.771.23) |
1,000,000 or more | 3.34 (2.724.09) | 2.90 (2.373.54) | 2.22 (1.822.71) |
Years in practice, 2001 | |||
Q1 (lowest) | 1.00 | 1.00 | 1.00 |
Q2 | 0.89 (0.711.12) | 0.83 (0.671.04) | 0.92 (0.741.14) |
Q3 | 1.06 (0.841.34) | 0.99 (0.791.24) | 1.03 (0.821.29) |
Q4 | 1.25 (0.991.59) | 1.13 (0.891.42) | 1.15 (0.921.45) |
Q5 (highest) | 1.35 (1.061.72) | 1.05 (0.831.33) | 0.92 (0.731.17) |
Total no. of outpatient visitsc | |||
Q1 (lowest) | 1.00 | 1.00 | 1.00 |
Q2 | 1.21 (1.121.30) | 1.07 (1.001.14) | 1.13 (1.071.19) |
Q3 | 1.42 (1.301.54) | 1.18 (1.091.27) | 1.14 (1.071.22) |
Q4 | 1.34 (1.211.47) | 1.34 (1.231.46) | 1.25 (1.161.35) |
Q5 (highest) | 1.46 (1.301.63) | 1.33 (1.211.47) | 1.32 (1.201.44) |
No. of hospitalized patientsc | |||
Q1 (lowest) | 1.00 | 1.00 | 1.00 |
Q2 | 1.07 (1.001.15) | 0.91 (0.860.96) | 0.85 (0.810.89) |
Q3 | 1.00 (0.921.08) | 0.87 (0.820.93) | 0.74 (0.700.79) |
Q4 | 0.89 (0.810.97) | 0.76 (0.710.82) | 0.62 (0.580.67) |
Q5 (highest) | 1.05 (0.951.18) | 0.67 (0.610.73) | 0.55 (0.510.60) |
Average outpatient agec | |||
Q1 (lowest) | 1.00 | 1.00 | 1.00 |
Q2 | 0.94 (0.871.01) | 1.15 (1.081.23) | 1.18 (1.111.25) |
Q3 | 0.82 (0.760.90) | 1.05 (0.971.13) | 1.17 (1.091.25) |
Q4 | 0.71 (0.650.79) | 1.03 (0.951.12) | 1.10 (1.021.19) |
Q5 (highest) | 0.72 (0.640.81) | 1.12 (1.011.23) | 1.15 (1.051.26) |
Average outpatient gender (% male)c | |||
Q1 (lowest) | 1.00 | 1.00 | 1.00 |
Q2 | 1.10 (1.021.18) | 1.19 (1.101.27) | 1.27 (1.181.37) |
Q3 | 1.12 (1.031.22) | 1.27 (1.171.37) | 1.43 (1.321.54) |
Q4 | 1.36 (1.251.48) | 1.49 (1.371.61) | 1.52 (1.401.65) |
Q5 (highest) | 1.47 (1.341.61) | 1.84 (1.682.00) | 1.68 (1.541.83) |
Average outpatient race (% white)c | |||
Q1 (lowest) | 1.00 | 1.00 | 1.00 |
Q2 | 1.08 (0.981.20) | 1.01 (0.921.10) | 1.23 (1.131.34) |
Q3 | 1.27 (1.131.43) | 1.06 (0.951.18) | 1.21 (1.091.34) |
Q4 | 1.47 (1.291.67) | 0.97 (0.861.09) | 1.33 (1.181.48) |
Q5 (highest) | 1.39 (1.211.59) | 1.18 (1.041.34) | 1.25 (1.101.42) |
Average outpatient comorbidityc | |||
Q1 (lowest) | 1.00 | 1.00 | 1.00 |
Q2 | 1.26 (1.191.35) | 1.23 (1.161.31) | 1.22 (1.141.30) |
Q3 | 1.62 (1.491.75) | 1.61 (1.501.72) | 1.43 (1.341.54) |
Q4 | 1.96 (1.792.15) | 1.86 (1.722.02) | 1.59 (1.471.72) |
Q5 (highest) | 1.79 (1.592.01) | 2.20 (2.002.41) | 2.03 (1.852.22) |
In terms of PCP workload, patients of PCPs with high outpatient activity were more likely to receive hospitalists care throughout the study period, although the association had decreased by 2007 to 2009 (highest vs lowest outpatient volume: OR: 1.46, 95% CI: 1.30‐1.63 in 20012003 vs OR: 1.32, 95% CI: 1.20‐1.44 in 20072009). In contrast, PCPs with the lowest inpatient volumes became more likely to use hospitalists by the end of the study period (highest vs lowest inpatient volume: OR: 1.05, 95% CI: 0.95‐1.18 in 20012003 vs OR: 0.55, 95% CI: 0.51‐0.60 in 20072009).
The characteristics of PCPs' practice panels also were associated with patients' likelihood of receiving care from hospitalists. PCPs whose practice panels consisted of patients who were predominantly male, white, or with more outpatient comorbidities were consistently more likely to use hospitalists throughout the study period. PCPs with older patient panels were less likely to use hospitalists in 2001 to 2003, but by 2007 to 2009, they were slightly more likely to do so (oldest vs youngest average outpatient panel age: OR: 0.72, 95% CI: 0.64‐0.81 in 20012003 vs OR: 1.15, 95% CI: 1.05‐1.26 in 20072009).
CONCLUSIONS
Prior studies of the hospitalist model have shown that the likelihood of a patient receiving inpatient care from hospitalists is associated with patient characteristics, hospital characteristics, geographic region, and type of admission.[1, 16, 17] We found that PCP characteristics also predict whether patients receive care from hospitalists and that their use of hospitalists developed dynamically between 2001 to 2009. Although many factors (such as whether patients were admitted to a hospital where their PCP had admitting privileges) can influence the decision to use hospitalists, we found that over one‐third of the variance in whether a hospitalized patient received care from a hospitalist is explained by which PCP the patient saw. In showing that systemic differences exist among PCPs who use hospitalists and those who do not, our study suggests that future research on the hospitalist model should, if possible, adjust for PCP characteristics in addition to hospital and patient factors.
Although this study identifies the existence and magnitude of differences in whether or not PCPs use hospitalists, it cannot explain why the differences exist. We only can offer hypotheses. For example, our finding that PCPs with the most years of practice experience were more likely to use hospitalists in the early 2000s but not in more recent years suggests that in hospital medicine's early years, long‐practicing generalist physicians were choosing between practicing traditionalist medicine and adopting the hospitalists model, but by 2009, experienced generalist physicians had already specialized to either inpatient or outpatient settings earlier in their careers. On the other hand, the decreasing odds of urban PCPs using hospitalists may reflect a relative growth in hospitalist use in less populated areas rather than a change in urban PCPs' practice patterns.
PCPs trained in family medicine have reported less inpatient training and less comfort with providing hospital care,[18, 19] thus it is unsurprising that family physicians were more likely to refer patients to hospitalists. Although a recent study reported that family physicians' inpatient volumes remained constant, whereas those of outpatient internists declined between 2003 and 2012, the analysis used University Health Consortium data and thus reflects practice patterns in academic medical centers.[20] Our data suggest that outside of academia, family physicians have embraced the hospitalists as clinical partners.
Meltzer and Chung had previously proposed an economic model to describe the growing use of hospitalists in the United States. They posited that decisions to adopt the hospitalist model are governed by trade‐offs between coordination costs (eg, time and effort spent coordinating multiple providers across different settings) and switching costs (eg, time spent traveling between the office and the hospital or the effort of adjusting to different work settings).[16] The authors hypothesized that empirical testing of this model would show PCPs are more likely to use hospitalists if they have less available professional time (ie, work fewer hours per week), are female (due to competing demands from domestic responsibilities), have relatively few hospitalized patients, or live in areas with high traffic congestion. Our findings provide empirical evidence to support their division‐of‐labor model in showing that patients were more likely to receive hospitalist care if their PCP was female, practiced in an urban location, had higher outpatient practice volumes, or had lower inpatient volumes.
At first glance, some of our findings appear to contradict our earlier study, which showed that younger, black, male patients are more likely to receive inpatient care from hospitalists.[1] However, that study included patients regardless of whether they had a PCP. This study shows that when patients have a PCP, their PCPs are more likely to refer them to hospitalists if they are older, white, male, and have more comorbid conditions. A potential explanation for this finding is that PCPs may preferentially use hospitalists when caring for older and sicker hospitalized patients. For example, commentators often cite hospitalists' constant availability in the hospital as a valuable resource when caring for acutely ill patients.[21, 22]
Another potential explanation is that despite their preferences, PCPs who care for younger, minority patients lack access to hospitalist services. One large study of Medicare beneficiaries reported that physicians who care for black patients are less well‐trained clinically and often lack access to important clinical resources such as diagnostic imaging and nonemergency hospital admissions.[23] Similarly, international medical graduates are more likely than their US‐trained counterparts to care for underserved patients and to practice in small, independent offices.[24, 25, 26] As hospitalist groups often rely on cross‐subsidization from sources within a large healthcare organization, independent PCPs may have less access to their services when compared with PCPs in managed care organizations or large integrated groups. Viewed in this context, our findings imply that although hospitalists often care for socioeconomically vulnerable patients (eg, younger, uninsured, black men) who lack access to primary care services,[1] they also appear to share care responsibilities for more complex hospitalized patients with PCPs in more affluent communities. Further research may determine if the availability of hospitalists influences racial disparities in hospital care.
Our study has limitations. It is an observational study and thus subject to bias and confounding. As our cohort was formed using fee‐for‐service Medicare data in a single, large state, it may not be generalizable to PCPs who practice in other states, who care for a younger population, or who do not accept Medicare. Our findings also may not reflect the practice patterns of physicians‐in‐training, PCP populations with high board‐certification rates, those employed in temporary positions, or those who interrupt their practices for personal reasons, as we restricted our study to established PCPs who had been in practice long and consistently enough to be associated with 20 hospitalized patients during every year of the study. For example, the lower proportion of female PCPs in our cohort (15.6% in our study in 2009 vs 27.5% reported in a nationally representative 2008 survey[27]) may be explained by our exclusion of women who take prolonged time off for childcare duties. We also did not establish whether patient outcomes or healthcare costs differ between PCPs who adopted the hospitalist model and traditionalists. Finally, we could not examine the effect of a number of PCP factors that could plausibly influence whether or not PCPs relinquish inpatient care to hospitalists, such as their comfort with providing inpatient care, having hospital admitting privileges, having office‐based access to hospitals' electronic medical records, or the distance between their office and the hospital. However, this study lays the groundwork for future studies to explore these factors.
In summary, this study is the first, to our knowledge, to characterize PCPs who relinquished inpatient responsibilities to hospitalists. Our findings suggest that some groups of PCPs are more likely to refer patient to hospitalists, that the relationship between hospitalists and PCPs has evolved over time, and that the hospitalist model still has ample room to grow.
ACKNOWLEDGMENTS
Disclosures: This study was supported by grants from the National Institute on Aging (1RO1‐AG033134 and P30‐AG024832) and the National Cancer Institute (K05‐CA124923). The authors have no financial conflicts of interest to disclose. An oral abstract of this article was presented on May 18, 2013 at the Society of Hospital Medicine Annual Meeting in National Harbor, Maryland.
- Growth in the care of older patients by hospitalists in the United States. N Engl J Med. 2009;360(11):1102–1112. , , , .
- Effect of hospitalists on length of stay in the medicare population: variation according to hospital and patient characteristics. J Am Geriatr Soc. 2010;58(9):1649–1657. , .
- Outcomes of care by hospitalists, general internists, and family physicians. N Engl J Med. 2007;357(25):2589–2600. , , , , , .
- Hospitalist care and length of stay in patients requiring complex discharge planning and close clinical monitoring. Arch Intern Med. 2007;167(17):1869–1874. , , , , .
- The impact of hospitalists on the cost and quality of inpatient care in the United States: a research synthesis. Med Care Res Rev. 2005;62(4):379–406. , .
- Association of hospitalist care with medical utilization after discharge: evidence of cost shift from a cohort study. Ann Intern Med. 2011;155(3):152–159. , .
- Hospital care and medical utilization after discharge. Ann Intern Med. 2011;155(10):719–720; author reply 722. , .
- Hospital care and medical utilization after discharge. Ann Intern Med. 2011;155(10):721; author reply 722. .
- Administrative data algorithms can describe ambulatory physician utilization. Health Serv Res. 2007;42:1783–1796. , , , , , .
- Estimating the reliability of continuous measures with Cronbach's alpha or the intraclass correlation coefficient: toward the integration of two traditions. J Clin Epidemiol. 1991;44(4–5):381–390. , .
- Ability of Medicare claims data to identify nursing home patients: a validation study. Med Care. 2008;46(11):1184–1187. , , .
- Comorbidity measures for use with administrative data. Med Care. 1998;36(1):8–27. , , , .
- A SAS procedure based on mixture models for estimating developmental trajectories. Sociol Methods Res. 2001;29(3):374–393. , , .
- Group‐Based Modeling of Development. Cambridge, MA: Harvard University Press; 2005. .
- Group‐based trajectory modeling in clinical research. Annu Rev Clin Psychol. 2010;6:109–138. , .
- , . Coordination, switching costs and the division of labor in general medicine: an economic explanation for the emergence of hospitalists in the United States. National Bureau of Economic Research Working Paper Series No. 16040. Cambridge, MA: National Bureau of Economic Research; 2010.
- Continuity of outpatient and inpatient care by primary care physicians for hospitalized older adults. JAMA. 2009;301(16):1671–1680. , , , , , .
- Hospitalists and family physicians: understanding opportunities and risks. J Fam Pract. 2004;53(6):473–481. .
- Preparedness of internal medicine and family practice residents for treating common conditions. JAMA. 2002;288(20):2609–2614. , , , , , .
- The status of adult inpatient care by family physicians at US academic medical centers and affiliated teaching hospitals 2003 to 2012: the impact of the hospitalist movement. Fam Med. 2014;46(2):94–99. , , , .
- Hospitalists and the hospital medicine system of care are good for patient care. Arch Intern Med. 2008;168(12):1254–1256; discussion 1259–1260. .
- Hospitalists in the United States—mission accomplished or work in progress? N Engl J Med. 2004;350(19):1935–1936. .
- Primary care physicians who treat blacks and whites. N Engl J Med. 2004;351(6):575–584. , , , , .
- International medical graduates and the primary care workforce for rural underserved areas. Health Aff (Millwood). 2003;22(2):255–262. , , , .
- Medical migration and the physician workforce. International medical graduates and American medicine. JAMA. 1995;273(19):1521–1527. , , .
- International medical graduates in family medicine in the United States of America: an exploration of professional characteristics and attitudes. Hum Resour Health. 2006;4:17. , , , , .
- A snapshot of U.S. physicians: key findings from the 2008 Health Tracking Physician Survey. Data Bull (Cent Stud Health Syst Change). 2009(35):1–11. , , .
- Growth in the care of older patients by hospitalists in the United States. N Engl J Med. 2009;360(11):1102–1112. , , , .
- Effect of hospitalists on length of stay in the medicare population: variation according to hospital and patient characteristics. J Am Geriatr Soc. 2010;58(9):1649–1657. , .
- Outcomes of care by hospitalists, general internists, and family physicians. N Engl J Med. 2007;357(25):2589–2600. , , , , , .
- Hospitalist care and length of stay in patients requiring complex discharge planning and close clinical monitoring. Arch Intern Med. 2007;167(17):1869–1874. , , , , .
- The impact of hospitalists on the cost and quality of inpatient care in the United States: a research synthesis. Med Care Res Rev. 2005;62(4):379–406. , .
- Association of hospitalist care with medical utilization after discharge: evidence of cost shift from a cohort study. Ann Intern Med. 2011;155(3):152–159. , .
- Hospital care and medical utilization after discharge. Ann Intern Med. 2011;155(10):719–720; author reply 722. , .
- Hospital care and medical utilization after discharge. Ann Intern Med. 2011;155(10):721; author reply 722. .
- Administrative data algorithms can describe ambulatory physician utilization. Health Serv Res. 2007;42:1783–1796. , , , , , .
- Estimating the reliability of continuous measures with Cronbach's alpha or the intraclass correlation coefficient: toward the integration of two traditions. J Clin Epidemiol. 1991;44(4–5):381–390. , .
- Ability of Medicare claims data to identify nursing home patients: a validation study. Med Care. 2008;46(11):1184–1187. , , .
- Comorbidity measures for use with administrative data. Med Care. 1998;36(1):8–27. , , , .
- A SAS procedure based on mixture models for estimating developmental trajectories. Sociol Methods Res. 2001;29(3):374–393. , , .
- Group‐Based Modeling of Development. Cambridge, MA: Harvard University Press; 2005. .
- Group‐based trajectory modeling in clinical research. Annu Rev Clin Psychol. 2010;6:109–138. , .
- , . Coordination, switching costs and the division of labor in general medicine: an economic explanation for the emergence of hospitalists in the United States. National Bureau of Economic Research Working Paper Series No. 16040. Cambridge, MA: National Bureau of Economic Research; 2010.
- Continuity of outpatient and inpatient care by primary care physicians for hospitalized older adults. JAMA. 2009;301(16):1671–1680. , , , , , .
- Hospitalists and family physicians: understanding opportunities and risks. J Fam Pract. 2004;53(6):473–481. .
- Preparedness of internal medicine and family practice residents for treating common conditions. JAMA. 2002;288(20):2609–2614. , , , , , .
- The status of adult inpatient care by family physicians at US academic medical centers and affiliated teaching hospitals 2003 to 2012: the impact of the hospitalist movement. Fam Med. 2014;46(2):94–99. , , , .
- Hospitalists and the hospital medicine system of care are good for patient care. Arch Intern Med. 2008;168(12):1254–1256; discussion 1259–1260. .
- Hospitalists in the United States—mission accomplished or work in progress? N Engl J Med. 2004;350(19):1935–1936. .
- Primary care physicians who treat blacks and whites. N Engl J Med. 2004;351(6):575–584. , , , , .
- International medical graduates and the primary care workforce for rural underserved areas. Health Aff (Millwood). 2003;22(2):255–262. , , , .
- Medical migration and the physician workforce. International medical graduates and American medicine. JAMA. 1995;273(19):1521–1527. , , .
- International medical graduates in family medicine in the United States of America: an exploration of professional characteristics and attitudes. Hum Resour Health. 2006;4:17. , , , , .
- A snapshot of U.S. physicians: key findings from the 2008 Health Tracking Physician Survey. Data Bull (Cent Stud Health Syst Change). 2009(35):1–11. , , .
© 2015 Society of Hospital Medicine
Dashboards and P4P in VTE Prophylaxis
The Affordable Care Act explicitly outlines improving the value of healthcare by increasing quality and decreasing costs. It emphasizes value‐based purchasing, the transparency of performance metrics, and the use of payment incentives to reward quality.[1, 2] Venous thromboembolism (VTE) prophylaxis is one of these publicly reported performance measures. The National Quality Forum recommends that each patient be evaluated on hospital admission and during their hospitalization for VTE risk level and for appropriate thromboprophylaxis to be used, if required.[3] Similarly, the Joint Commission includes appropriate VTE prophylaxis in its Core Measures.[4] Patient experience and performance metrics, including VTE prophylaxis, constitute the hospital value‐based purchasing (VBP) component of healthcare reform.[5] For a hypothetical 327‐bed hospital, an estimated $1.7 million of a hospital's inpatient payments from Medicare will be at risk from VBP alone.[2]
VTE prophylaxis is a common target of quality improvement projects. Effective, safe, and cost‐effective measures to prevent VTE exist, including pharmacologic and mechanical prophylaxis.[6, 7] Despite these measures, compliance rates are often below 50%.[8] Different interventions have been pursued to ensure appropriate VTE prophylaxis, including computerized provider order entry (CPOE), electronic alerts, mandatory VTE risk assessment and prophylaxis, and provider education campaigns.[9] Recent studies show that CPOE systems with mandatory fields can increase VTE prophylaxis rates to above 80%, yet the goal of a high reliability health system is for 100% of patients to receive recommended therapy.[10, 11, 12, 13, 14, 15] Interventions to improve prophylaxis rates that have included multiple strategies, such as computerized order sets, feedback, and education, have been the most effective, increasing compliance to above 90%.[9, 11, 16] These systems can be enhanced with additional interventions such as providing individualized provider education and feedback, understanding of work flow, and ensuring patients receive the prescribed therapies.[12] For example, a physician dashboard could be employed to provide a snapshot and historical trend of key performance indicators using graphical displays and indicators.[17]
Dashboards and pay‐for‐performance programs have been increasingly used to increase the visibility of these metrics, provide feedback, visually display benchmarks and goals, and proactively monitor for achievements and setbacks.[18] Although these strategies are often addressed at departmental (or greater) levels, applying them at the level of the individual provider may assist hospitals in reducing preventable harm and achieving safety and quality goals, especially at higher benchmarks. With their expanding role, hospitalists provide a key opportunity to lead improvement efforts and to study the impact of dashboards and pay‐for performance at the provider level to achieve VTE prophylaxis performance targets. Hospitalists are often the front‐line provider for inpatients and deliver up to 70% of inpatient general medical services.[19] The objective of our study was to evaluate the impact of providing individual provider feedback and employing a pay‐for‐performance program on baseline performance of VTE prophylaxis among hospitalists. We hypothesized that performance feedback through the use of a dashboard would increase appropriate VTE prophylaxis, and this effect would be further augmented by incorporation of a pay‐for‐performance program.
METHODS
Hospitalist Dashboard
In 2010, hospitalist program leaders met with hospital administrators to create a hospitalist dashboard that would provide regularly updated summaries of performance measures for individual hospitalists. The final set of metrics identified included appropriate VTE prophylaxis, length of stay, patients discharged per day, discharges before 3 pm, depth of coding, patient satisfaction, readmissions, communication with the primary care provider, and time to signature for discharge summaries (Figure 1A). The dashboard was introduced at a general hospitalist meeting during which its purpose, methodology, and accessibility were described; it was subsequently implemented in January 2011.
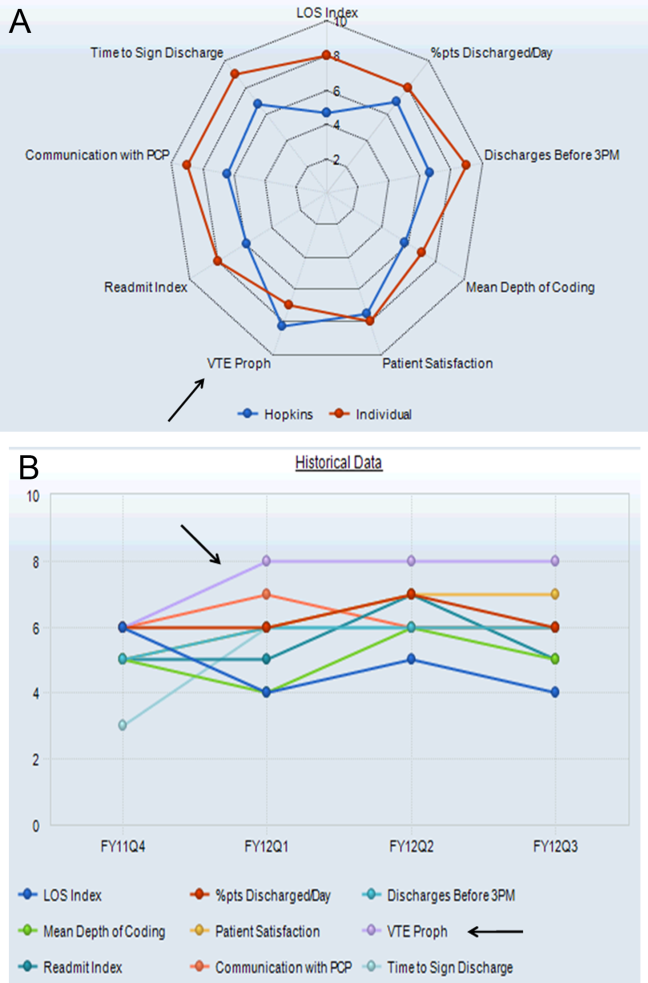
Benchmarks were established for each metric, standardized to establish a scale ranging from 1 through 9, and incorporated into the dashboard (Figure 1A). Higher scores (creating a larger geometric shape) were desirable. For the VTE prophylaxis measure, scores of 1 through 9 corresponded to <60%, 60% to 64.9%, 65% to 69.9%, 70% to 74.9%, 75% to 79.9%, 80% to 84.9%, 85% to 89.9%, 90% to 94.9%, and 95% American College of Chest Physicians (ACCP)‐compliant VTE prophylaxis, respectively.[12, 20] Each provider was able to access the aggregated dashboard (showing the group mean) and his/her individualized dashboard using an individualized login and password for the institutional portal. This portal is used during the provider's workflow, including medical record review and order entry. Both a polygonal summary graphic (Figure 1A) and trend (Figure 1B) view of the dashboard were available to the provider. A comparison of the individual provider to the hospitalist group average was displayed (Figure 1A). At monthly program meetings, the dashboard, group results, and trends were discussed.
Venous Thromboembolism Prophylaxis Compliance
Our study was performed in a tertiary academic medical center with an approximately 20‐member hospitalist group (the precise membership varied over time), whose responsibilities include, among other clinical duties, staffing a 17‐bed general medicine unit with telemetry. The scope of diagnoses and acuity of patients admitted to the hospitalist service is similar to the housestaff services. Some hospitalist faculty serve both as hospitalist and nonhospitalist general medicine service team attendings, but the comparison groups were staffed by hospitalists for <20% of the time. For admissions, all hospitalists use a standardized general medicine admission order set that is integrated into the CPOE system (Sunrise Clinical Manager; Allscripts, Chicago, IL) and completed for all admitted patients. A mandatory VTE risk screen, which includes an assessment of VTE risk factors and pharmacological prophylaxis contraindications, must be completed by the ordering physician as part of this order set (Figure 2A). The system then prompts the provider with a risk‐appropriate VTE prophylaxis recommendation that the provider may subsequently order, including mechanical prophylaxis (Figure 2B). Based on ACCP VTE prevention guidelines, risk‐appropriate prophylaxis was determined using an electronic algorithm that categorized patients into risk categories based on the presence of major VTE risk factors (Figure 2A).[12, 15, 20] If none of these were present, the provider selected No major risk factors known. Both an assessment of current use of anticoagulation and a clinically high risk of bleeding were also included (Figure 2A). If none of these were present, the provider selected No contraindications known. This algorithm is published in detail elsewhere and has been shown to not increase major bleeding episodes.[12, 15] The VTE risk assessment, but not the VTE order itself, was a mandatory field. This allowed the physician discretion to choose among various pharmacological agents and mechanical mechanisms based on patient and physician preferences.
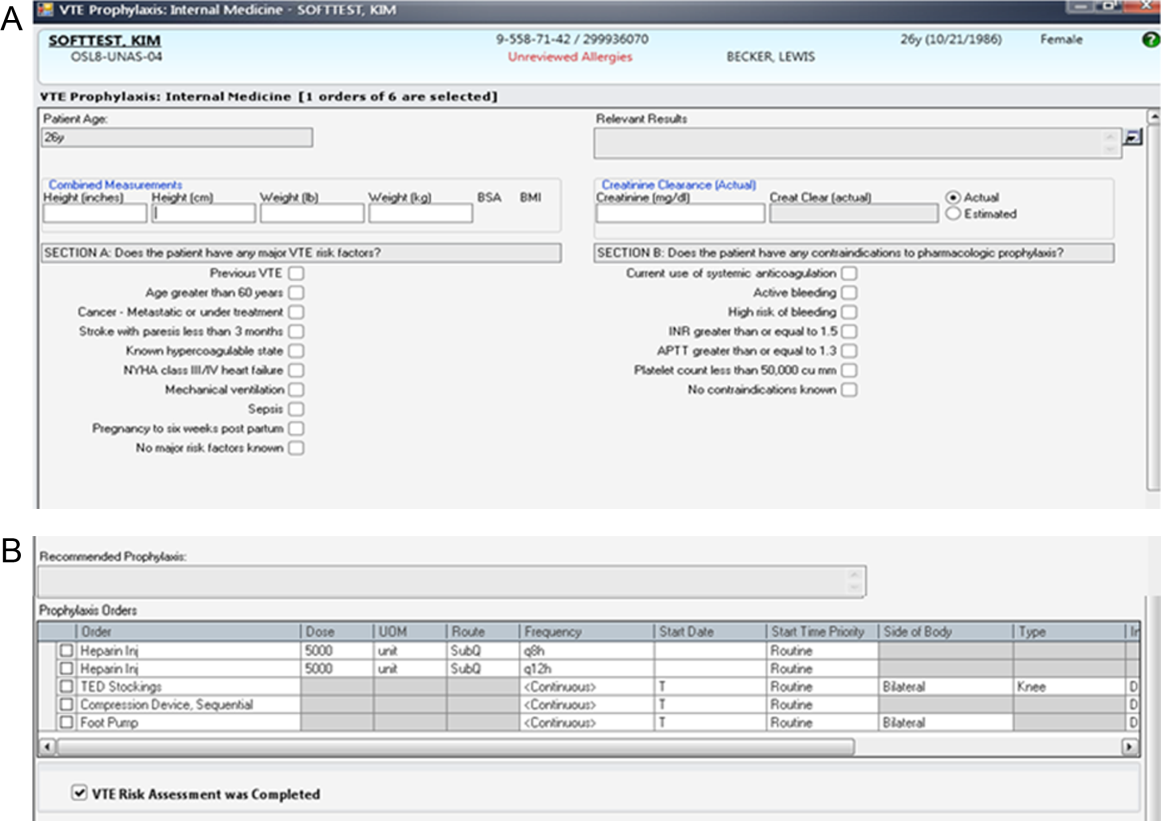
Compliance of risk‐appropriate VTE prophylaxis was determined 24 hours after the admission order set was completed using an automated electronic query of the CPOE system. Low molecular‐weight heparin prescription was included in the compliance algorithm as acceptable prophylaxis. Prescription of pharmacological VTE prophylaxis when a contraindication was present was considered noncompliant. The metric was assigned to the attending physician who billed for the first inpatient encounter.
Pay‐for‐Performance Program
In July 2011, a pay‐for‐performance program was added to the dashboard. All full‐time and part‐time hospitalists were eligible. The financial incentive was determined according to hospital priority and funds available. The VTE prophylaxis metric was prorated by clinical effort, with a maximum of $0.50 per work relative value unit (RVU). To optimize performance, a threshold of 80% compliance had to be surpassed before any payment was made. Progressively increasing percentages of the incentive were earned as compliance increased from 80% to 100%, corresponding to dashboard scores of 6, 7, 8, and 9: <80% (scores 1 to 5)=no payment; 80% to 84.9% (score 6)=$0.125 per RVU; 85% to 89.9% (score 7)=$0.25 per RVU; 90% to 94.9% (score 8)=$0.375 per RVU; and 95% (score 9)=$0.50 per RVU (maximum incentive). Payments were accrued quarterly and paid at the end of the fiscal year as a cumulative, separate performance supplement.
Individualized physician feedback through the dashboard was continued during the pay‐for‐performance period. Average hospitalist group compliance continued to be displayed on the electronic dashboard and was explicitly reviewed at monthly hospitalist meetings.
The VTE prophylaxis order set and data collection and analyses were approved by the Johns Hopkins Medicine Institutional Review Board. The dashboard and pay‐for‐performance program were initiated by the institution as part of a proof of concept quality improvement project.
Analysis
We examined all inpatient admissions to the hospitalist unit from 2008 to 2012. We included patients admitted to and discharged from the hospitalist unit and excluded patients transferred into/out of the unit and encounters with a length of stay <24 hours. VTE prophylaxis orders were queried from the CPOE system 24 hours after the patient was admitted to determine compliance.
After allowing for a run‐in period (2008), we analyzed the change in percent compliance for 3 periods: (1) CPOE‐based VTE order set alone (baseline [BASE], January 2009 to December 2010); (2) group and individual physician feedback using the dashboard (dashboard only [DASH], January to June 2011); and (3) dashboard tied to the pay‐for‐performance program (dashboard with pay‐for‐performance [P4P], July 2011 to December 2012). The CPOE‐based VTE order set was used during all 3 periods. We used the other medical services as a control to ensure that there were no temporal trends toward improved prophylaxis on a service without the intervention. VTE prophylaxis compliance was examined by calculating percent compliance using the same algorithm for the 4 resident‐staffed general medicine service teams at our institution, which utilized the same CPOE system but did not receive the dashboard or pay‐for‐performance interventions. We used locally weighted scatterplot smoothing, a locally weighted regression of percent compliance over time, to graphically display changes in group compliance over time.[21, 22]
We also performed linear regression to assess the rate of change in group compliance and included spline terms that allowed slope to vary for each of the 3 time periods.[23, 24] Clustered analysis accounted for potentially correlated serial measurements of compliance for an individual provider. A separate analysis examined the effect of provider turnover and individual provider improvement during each of the 3 periods. Tests of significance were 2‐sided, with an level of 0.05. Statistical analysis was performed using Stata 12.1 (StataCorp LP, College Station, TX).
RESULTS
Venous Thromboembolism Prophylaxis Compliance
We analyzed 3144 inpatient admissions by 38 hospitalists from 2009 to 2012. The 5 most frequent coded diagnoses were heart failure, acute kidney failure, syncope, pneumonia, and chest pain. Patients had a median length of stay of 3 days [interquartile range: 26]. During the dashboard‐only period, on average, providers improved in compliance by 4% (95% confidence interval [CI]: 35; P<0.001). With the addition of the pay‐for‐performance program, providers improved by an additional 4% (95% CI: 35; P<0.001). Group compliance significantly improved from 86% (95% CI: 8588) during the BASE period of the CPOE‐based VTE order set to 90% (95% CI: 8893) during the DASH period (P=0.01) and 94% (95% CI: 9396) during the subsequent P4P program (P=0.01) (Figure 3). Both inappropriate prophylaxis and lack of prophylaxis, when indicated, resulted in a non‐compliance rating. During the 3 periods, inappropriate prophylaxis decreased from 7.9% to 6.2% to 2.6% during the BASE, DASH, and subsequent P4P periods, respectively. Similarly, lack of prophylaxis when indicated decreased from 6.1% to 3.2% to 3.1% during the BASE, DASH, and subsequent P4P periods, respectively.
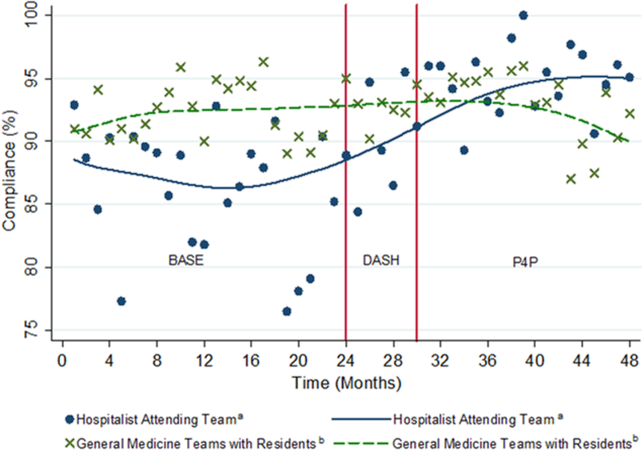
The average compliance of the 4 non‐hospitalist general medicine service teams was initially higher than that of the hospitalist service during the CPOE‐based VTE order set (90%) and DASH (92%) periods, but subsequently plateaued and was exceeded by the hospitalist service during the combined P4P (92%) period (Figure 3). However, there was no statistically significant difference between the general medicine service teams and hospitalist service during the DASH (P=0.15) and subsequent P4P (P=0.76) periods.
We also analyzed the rate of VTE prophylaxis compliance improvement (slope) with cut points at each time period transition (Figure 3). Risk‐appropriate VTE prophylaxis during the BASE period did not exhibit significant improvement as indicated by the slope (P=0.23) (Figure 3). In contrast, during the DASH period, VTE prophylaxis compliance significantly increased by 1.58% per month (95% CI: 0.41‐2.76; P=0.01). The addition of the P4P program, however, did not further significantly increase the rate of compliance (P=0.78).
A subgroup analysis restricted to the 19 providers present during all 3 periods was performed to assess for potential confounding from physician turnover. The percent compliance increased in a similar fashion: BASE period of CPOE‐based VTE order set, 85% (95% CI: 8386); DASH, 90% (95% CI: 8893); and P4P, 94% (95% CI: 9296).
Pay‐for‐Performance Program
Nineteen providers met the threshold for pay‐for‐performance (80% appropriate VTE prophylaxis), with 9 providers in the intermediate categories (80%94.9%) and 10 in the full incentive category (95%). The mean individual payout for the incentive was $633 (standard deviation 350), with a total disbursement of $12,029. The majority of payments (17 of 19) were under $1000.
DISCUSSION
A key component of healthcare reform has been value‐based purchasing, which emphasizes extrinsic motivation through the transparency of performance metrics and use of payment incentives to reward quality. Our study evaluates the impact of both extrinsic (payments) and intrinsic (professionalism and peer norms) motivation. It specifically attributed an individual performance metric, VTE prophylaxis, to an attending physician, provided both individualized and group feedback using an electronic dashboard, and incorporated a pay‐for‐performance program. Prescription of risk‐appropriate VTE prophylaxis significantly increased with the implementation of the dashboard and subsequent pay‐for performance program. The fastest rate of improvement occurred after the addition of the dashboard. Sensitivity analyses for provider turnover and comparisons to the general medicine services showed our results to be independent of a general trend of improvement, both at the provider and institutional levels.
Our prior studies demonstrated that order sets significantly improve performance, from a baseline compliance of risk‐appropriate VTE prophylaxis of 66% to 84%.[13, 15, 25] In the current study, compliance was relatively flat during the BASE period, which included these order sets. The greatest rate of continued improvement in compliance occurred during the DASH period, emphasizing both the importance of provider feedback and receptivity and adaptability in the prescribing behavior of hospitalists. Because the goal of a high‐reliability health system is for 100% of patients to receive recommended therapy, multiple approaches are necessary for success.
Nationally, benchmarks for performance measures continue to be raised, with the highest performers achieving above 95%.[26] Additional interventions, such as dashboards and pay‐for‐performance programs, supplement CPOE systems to achieve high reliability. In our study, the compliance rate during the baseline period, which included a CPOE‐based, clinical support‐enabled VTE order set, was 86%. Initially the compliance of the general medicine teams with residents exceeded that of the hospitalist attending teams, which may reflect a greater willingness of resident teams to comply with order sets and automated recommendations. This emphasizes the importance of continuous individual feedback and provider education at the attending physician level to enhance both guideline compliance and decrease provider care variation. Ultimately, with the addition of the dashboard and subsequent pay‐for‐performance program, compliance was increased to 90% and 94%, respectively. Although the major mechanism used by policymakers to improve quality of care is extrinsic motivation, this study demonstrates that intrinsic motivation through peer norms can enhance extrinsic efforts and may be more influential. Both of these programs, dashboards and pay‐for‐performance, may ultimately assist institutions in changing provider behavior and achieving these harder‐to‐achieve higher benchmarks.
We recognize that there are several limitations to our study. First, this is a single‐site program limited to an attending‐physician‐only service. There was strong data support and a defined CPOE algorithm for this initiative. Multi‐site studies will need to overcome the additional challenges of varying service structures and electronic medical record and provider order entry systems. Second, it is difficult to show actual changes in VTE events over time with appropriate prophylaxis. Although VTE prophylaxis is recommended for patients with VTE risk factors, there are conflicting findings about whether prophylaxis prevents VTE events in lower‐risk patients, and current studies suggest that most patients with VTE events are severely ill and develop VTE despite receiving prophylaxis.[27, 28, 29] Our study was underpowered to detect these potential differences in VTE rates, and although the algorithm has been shown to not increase bleeding rates, we did not measure bleeding rates during this study.[12, 15] Our institutional experience suggests that the majority of VTE events occur despite appropriate prophylaxis.[30] Also, VTE prophylaxis may be ordered, but intervening events, such as procedures and changes in risk status or patient refusal, may prevent patients from receiving appropriate prophylaxis.[31, 32] Similarly, hospitals with higher quality scores have higher VTE prophylaxis rates but worse risk‐adjusted VTE rates, which may result from increased surveillance for VTE, suggesting surveillance bias limits the usefulness of the VTE quality measure.[33, 34] Nevertheless, VTE prophylaxis remains a publicly reported Core Measure tied to financial incentives.[4, 5] Third, there may be an unmeasured factor specific to the hospitalist program, which could potentially account for an overall improvement in quality of care. Although the rate of increase in appropriate prophylaxis was not statistically significant during the baseline period, there did appear to be some improvement in prophylaxis toward the end of the period. However, there were no other VTE‐related provider feedback programs being simultaneously pursued during this study. VTE prophylaxis for the non‐hospitalist services showed a relatively stable, non‐increasing compliance rate for the general medical services. Although it was possible for successful residents to age into the hospitalist service, thereby improving rates of prophylaxis based on changes in group makeup, our subgroup analysis of the providers present throughout all phases of the study showed our results to be robust. Similarly, there may have been a cross‐contamination effect of hospitalist faculty who attended on both hospitalist and non‐hospitalist general medicine service teams. This, however, would attenuate any impact of the programs, and thus the effects may in fact be greater than reported. Fourth, establishment of both the dashboard and pay‐for‐performance program required significant institutional and program leadership and resources. To be successful, the dashboard must be in the provider's workflow, transparent, minimize reporter burden, use existing systems, and be actively fed back to providers, ideally those directly entering orders. Our greatest rate of improvement occurred during the feedback‐only phase of this study, emphasizing the importance of physician feedback, provider‐level accountability, and engagement. We suspect that the relatively modest pay‐for‐performance incentive served mainly as a means of engaging providers in self‐monitoring, rather than as a means to change behavior through true incentivization. Although we did not track individual physician views of the dashboard, we reinforced trends, deviations, and expectations at regularly scheduled meetings and provided feedback and patient‐level data to individual providers. Fifth, the design of the pay‐for‐performance program may have also influenced its effectiveness. These types of programs may be more effective when they provide frequent visible, small payments rather than one large payment, and when the payment is framed as a loss rather than a gain.[35] Finally, physician champions and consistent feedback through departmental meetings or visual displays may be required for program success. The initial resources to create the dashboard, continued maintenance and monitoring of performance, and payment of financial incentives all require institutional commitment. A partnership of physicians, program leaders, and institutional administrators is necessary for both initial and continued success.
To achieve performance goals and benchmarks, multiple strategies that combine extrinsic and intrinsic motivation are necessary. As shown by our study, the use of a dashboard and pay‐for‐performance can be tailored to an institution's goals, in line with national standards. The specific goal (risk‐appropriate VTE prophylaxis) and benchmarks (80%, 85%, 90%, 95%) can be individualized to a particular institution. For example, if readmission rates are above target, readmissions could be added as a dashboard metric. The specific benchmark would be determined by historical trends and administrative targets. Similarly, the overall financial incentives could be adjusted based on the financial resources available. Other process measures, such as influenza vaccination screening and administration, could also be targeted. For all of these objectives, continued provider feedback and engagement are critical for progressive success, especially to decrease variability in care at the attending physician level. Incorporating the value‐based purchasing philosophy from the Affordable Care Act, our study suggests that the combination of standardized order sets, real‐time dashboards, and physician‐level incentives may assist hospitals in achieving quality and safety benchmarks, especially at higher targets.
Acknowledgements
The authors thank Meir Gottlieb, BS, from Salar Inc. for data support; Murali Padmanaban, BS, from Johns Hopkins University for his assistance in linking the administrative billing data with real‐time physician orders; and Hsin‐Chieh Yeh, PhD, from the Bloomberg School of Public Health for her statistical advice and additional review. We also thank Mr. Ronald R. Peterson, President, Johns Hopkins Health System and Johns Hopkins Hospital, for providing funding support for the physician incentive payments.
Disclosures: Drs. Michtalik and Brotman had full access to all of the data in the study and take responsibility for the integrity of the data and accuracy of the data analysis. Study concept and design: Drs. Michtalik, Streiff, Finkelstein, Pronovost, and Brotman. Acquisition of data: Drs. Michtalik, Streiff, Brotman and Mr. Carolan, Mr. Lau, Mrs. Durkin. Analysis and interpretation of data: Drs. Michtalik, Haut, Streiff, Brotman and Mr. Carolan, Mr. Lau. Drafting of the manuscript: Drs. Michtalik and Brotman. Critical revision of the manuscript for important intellectual content: Drs. Michtalik, Haut, Streiff, Finkelstein, Pronovost, Brotman and Mr. Carolan, Mr. Lau, Mrs. Durkin. Statistical analysis and supervision: Drs. Michtalik and Brotman. Obtaining funding: Drs. Streiff and Brotman. Technical support: Dr. Streiff and Mr. Carolan, Mr. Lau, Mrs. Durkin
This study was supported by a National Institutes of Health grant T32 HP10025‐17‐00 (Dr. Michtalik), the National Institutes of Health/Johns Hopkins Institute for Clinical and Translational Research KL2 Award 5KL2RR025006 (Dr. Michtalik), the Agency for Healthcare Research and Quality Mentored Clinical Scientist Development K08 Awards 1K08HS017952‐01 (Dr. Haut) and 1K08HS022331‐01A1 (Dr. Michtalik), and the Johns Hopkins Hospitalist Scholars Fund. The funders had no role in the design and conduct of the study; collection, management, analysis, and interpretation of the data; preparation, review, or approval of the manuscript; and decision to submit the manuscript for publication.
Dr. Haut receives royalties from Lippincott, Williams & Wilkins. Dr. Streiff has received research funding from Portola and Bristol Myers Squibb, honoraria for CME lectures from Sanofi‐Aventis and Ortho‐McNeil, consulted for Eisai, Daiichi‐Sankyo, Boerhinger‐Ingelheim, Janssen Healthcare, and Pfizer. Mr. Lau, Drs. Haut, Streiff, and Pronovost are supported by a contract from the Patient‐Centered Outcomes Research Institute (PCORI) titled Preventing Venous Thromboembolism: Empowering Patients and Enabling Patient‐Centered Care via Health Information Technology (CE‐12‐11‐4489). Dr. Brotman has received research support from Siemens Healthcare Diagnostics, Bristol‐Myers Squibb, the Agency for Healthcare Research and Quality, Centers for Medicare & Medicaid Services, the Amerigroup Corporation, and the Guerrieri Family Foundation. He has received honoraria from the Gerson Lehrman Group, the Dunn Group, and from Quantia Communications, and received royalties from McGraw‐Hill.
- Medicare Program, Centers for Medicare 76(88):26490–26547.
- Quality meets finance: payments at risk with value‐based purchasing, readmission, and hospital‐acquired conditions force hospitalists to focus. Hospitalist. 2013;17(1):31. .
- National Quality Forum. March 2009. Safe practices for better healthcare—2009 update. Available at: http://www.qualityforum.org/Publications/2009/03/Safe_Practices_for_Better_Healthcare%E2%80%932009_Update.aspx. Accessed November 1, 2014.
- Joint Commission on Accreditation of Healthcare Organizations. Approved: more options for hospital core measures. Jt Comm Perspect. 2009;29(4):1–6.
- Centers for Medicare 208(2):227–240.
- Thromboprophylaxis in nonsurgical patients. Hematology Am Soc Hematol Educ Program. 2012;2012:631–637. , .
- Venous thromboembolism risk and prophylaxis in the acute hospital care setting (ENDORSE study): a multinational cross‐sectional study. Lancet. 2008;371(9610):387–394. , , , et al.
- Practices to prevent venous thromboembolism: a brief review. BMJ Qual Saf. 2014;23(3):187–195. , .
- Improving hospital venous thromboembolism prophylaxis with electronic decision support. J Hosp Med. 2013;8(3):115–120. , , , et al.
- Innovative approaches to increase deep vein thrombosis prophylaxis rate resulting in a decrease in hospital‐acquired deep vein thrombosis at a tertiary‐care teaching hospital. J Hosp Med. 2008;3(2):148–155. , , .
- Lessons from the Johns Hopkins Multi‐Disciplinary Venous Thromboembolism (VTE) Prevention Collaborative. BMJ. 2012;344:e3935. , , , et al.
- Improved prophylaxis and decreased rates of preventable harm with the use of a mandatory computerized clinical decision support tool for prophylaxis for venous thromboembolism in trauma. Arch Surg. 2012;147(10):901–907. , , , et al.
- Designing and implementing effective venous thromboembolism prevention protocols: lessons from collaborative efforts. J Thromb Thrombolysis. 2010;29(2):159–166. , .
- Impact of a venous thromboembolism prophylaxis "smart order set": improved compliance, fewer events. Am J Hematol. 2013;88(7):545–549. , , , et al.
- Improving adherence to venous thromoembolism prophylaxis using multiple interventions. BMJ. 2012;344:e3935. , .
- Health Resources and Services Administration of the U.S. Department of Health and Human Services. Managing data for performance improvement. Available at: http://www.hrsa.gov/quality/toolbox/methodology/performanceimprovement/part2.html. Accessed December 18, 2014.
- Improving patient safety by taking systems seriously. JAMA. 2008;299(4):445–447. , .
- Growth in the care of older patients by hospitalists in the United States. N Engl J Med. 2009;360(11):1102–1112. , , , .
- Prevention of venous thromboembolism: American College of Chest Physicians evidence‐based clinical practice guidelines (8th edition). Chest. 2008;133(6 suppl):381S–453S. , , , et al.
- Robust locally weighted regression and smoothing scatterplots. J Am Stat Assoc. 1979;74(368):829–836. .
- Locally weighted regression: An approach to regression analysis by local fitting. J Am Stat Assoc. 1988;83(403):596–610. , .
- Regression Methods in Biostatistics: Linear, Logistic, Survival, and Repeated Measures Models. 2nd ed. New York, NY: Springer; 2012. , , , .
- Regression Modeling Strategies: With Applications to Linear Models, Logistic Regression, and Survival Analysis. New York, NY: Springer‐Verlag; 2001. .
- Med Care. doi: 10.1097/MLR.0000000000000251. , , , et al. Eliminating healthcare disparities via mandatory clinical decision support: the venous thromboembolism (VTE) example [published online ahead of print November 4, 2014].
- Joint Commission. Improving America's hospitals: the Joint Commission's annual report on quality and safety. 2012. Available at: http://www.jointcommission.org/assets/1/18/TJC_Annual_Report_2012.pdf. Accessed September 8, 2013.
- Hospital performance for pharmacologic venous thromboembolism prophylaxis and rate of venous thromboembolism: a cohort study. JAMA Intern Med. 2014;174(10):1577–1584. , , , et al.
- Incidence of hospital‐acquired venous thromboembolic codes in medical patients hospitalized in academic medical centers. J Hosp Med. 2014;9(4):221–225. , , , et al.
- No association between hospital‐reported perioperative venous thromboembolism prophylaxis and outcome rates in publicly reported data. JAMA Surg. 2014;149(4):400–401. , , , , .
- Linking processes and outcomes: a key strategy to prevent and report harm from venous thromboembolism in surgical patients. JAMA Surg. 2013;148(3):299–300. , , , , .
- Patterns of non‐administration of ordered doses of venous thromboembolism prophylaxis: implications for novel intervention strategies. PLoS One. 2013;8(6):e66311. , , , et al.
- Nonadministration of thromboprophylaxis in hospitalized patients with HIV: a missed opportunity for prevention? J Hosp Med. 2014;9(4):215–220. , , , et al.
- Evaluation of surveillance bias and the validity of the venous thromboembolism quality measure. JAMA. 2013;310(14):1482–1489. , , , et al.
- Surveillance bias in outcomes reporting. JAMA. 2011;305(23):2462–2463. , .
- Pay for performance in health care: an international overview of initiatives. Med Care Res Rev. 2012;69(3):251–276. .
The Affordable Care Act explicitly outlines improving the value of healthcare by increasing quality and decreasing costs. It emphasizes value‐based purchasing, the transparency of performance metrics, and the use of payment incentives to reward quality.[1, 2] Venous thromboembolism (VTE) prophylaxis is one of these publicly reported performance measures. The National Quality Forum recommends that each patient be evaluated on hospital admission and during their hospitalization for VTE risk level and for appropriate thromboprophylaxis to be used, if required.[3] Similarly, the Joint Commission includes appropriate VTE prophylaxis in its Core Measures.[4] Patient experience and performance metrics, including VTE prophylaxis, constitute the hospital value‐based purchasing (VBP) component of healthcare reform.[5] For a hypothetical 327‐bed hospital, an estimated $1.7 million of a hospital's inpatient payments from Medicare will be at risk from VBP alone.[2]
VTE prophylaxis is a common target of quality improvement projects. Effective, safe, and cost‐effective measures to prevent VTE exist, including pharmacologic and mechanical prophylaxis.[6, 7] Despite these measures, compliance rates are often below 50%.[8] Different interventions have been pursued to ensure appropriate VTE prophylaxis, including computerized provider order entry (CPOE), electronic alerts, mandatory VTE risk assessment and prophylaxis, and provider education campaigns.[9] Recent studies show that CPOE systems with mandatory fields can increase VTE prophylaxis rates to above 80%, yet the goal of a high reliability health system is for 100% of patients to receive recommended therapy.[10, 11, 12, 13, 14, 15] Interventions to improve prophylaxis rates that have included multiple strategies, such as computerized order sets, feedback, and education, have been the most effective, increasing compliance to above 90%.[9, 11, 16] These systems can be enhanced with additional interventions such as providing individualized provider education and feedback, understanding of work flow, and ensuring patients receive the prescribed therapies.[12] For example, a physician dashboard could be employed to provide a snapshot and historical trend of key performance indicators using graphical displays and indicators.[17]
Dashboards and pay‐for‐performance programs have been increasingly used to increase the visibility of these metrics, provide feedback, visually display benchmarks and goals, and proactively monitor for achievements and setbacks.[18] Although these strategies are often addressed at departmental (or greater) levels, applying them at the level of the individual provider may assist hospitals in reducing preventable harm and achieving safety and quality goals, especially at higher benchmarks. With their expanding role, hospitalists provide a key opportunity to lead improvement efforts and to study the impact of dashboards and pay‐for performance at the provider level to achieve VTE prophylaxis performance targets. Hospitalists are often the front‐line provider for inpatients and deliver up to 70% of inpatient general medical services.[19] The objective of our study was to evaluate the impact of providing individual provider feedback and employing a pay‐for‐performance program on baseline performance of VTE prophylaxis among hospitalists. We hypothesized that performance feedback through the use of a dashboard would increase appropriate VTE prophylaxis, and this effect would be further augmented by incorporation of a pay‐for‐performance program.
METHODS
Hospitalist Dashboard
In 2010, hospitalist program leaders met with hospital administrators to create a hospitalist dashboard that would provide regularly updated summaries of performance measures for individual hospitalists. The final set of metrics identified included appropriate VTE prophylaxis, length of stay, patients discharged per day, discharges before 3 pm, depth of coding, patient satisfaction, readmissions, communication with the primary care provider, and time to signature for discharge summaries (Figure 1A). The dashboard was introduced at a general hospitalist meeting during which its purpose, methodology, and accessibility were described; it was subsequently implemented in January 2011.
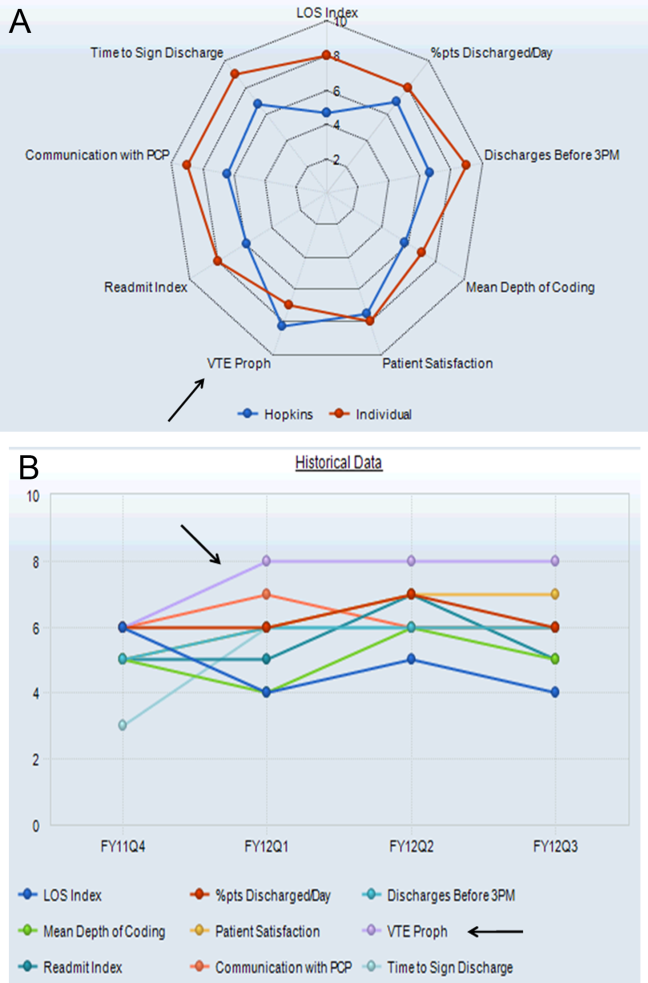
Benchmarks were established for each metric, standardized to establish a scale ranging from 1 through 9, and incorporated into the dashboard (Figure 1A). Higher scores (creating a larger geometric shape) were desirable. For the VTE prophylaxis measure, scores of 1 through 9 corresponded to <60%, 60% to 64.9%, 65% to 69.9%, 70% to 74.9%, 75% to 79.9%, 80% to 84.9%, 85% to 89.9%, 90% to 94.9%, and 95% American College of Chest Physicians (ACCP)‐compliant VTE prophylaxis, respectively.[12, 20] Each provider was able to access the aggregated dashboard (showing the group mean) and his/her individualized dashboard using an individualized login and password for the institutional portal. This portal is used during the provider's workflow, including medical record review and order entry. Both a polygonal summary graphic (Figure 1A) and trend (Figure 1B) view of the dashboard were available to the provider. A comparison of the individual provider to the hospitalist group average was displayed (Figure 1A). At monthly program meetings, the dashboard, group results, and trends were discussed.
Venous Thromboembolism Prophylaxis Compliance
Our study was performed in a tertiary academic medical center with an approximately 20‐member hospitalist group (the precise membership varied over time), whose responsibilities include, among other clinical duties, staffing a 17‐bed general medicine unit with telemetry. The scope of diagnoses and acuity of patients admitted to the hospitalist service is similar to the housestaff services. Some hospitalist faculty serve both as hospitalist and nonhospitalist general medicine service team attendings, but the comparison groups were staffed by hospitalists for <20% of the time. For admissions, all hospitalists use a standardized general medicine admission order set that is integrated into the CPOE system (Sunrise Clinical Manager; Allscripts, Chicago, IL) and completed for all admitted patients. A mandatory VTE risk screen, which includes an assessment of VTE risk factors and pharmacological prophylaxis contraindications, must be completed by the ordering physician as part of this order set (Figure 2A). The system then prompts the provider with a risk‐appropriate VTE prophylaxis recommendation that the provider may subsequently order, including mechanical prophylaxis (Figure 2B). Based on ACCP VTE prevention guidelines, risk‐appropriate prophylaxis was determined using an electronic algorithm that categorized patients into risk categories based on the presence of major VTE risk factors (Figure 2A).[12, 15, 20] If none of these were present, the provider selected No major risk factors known. Both an assessment of current use of anticoagulation and a clinically high risk of bleeding were also included (Figure 2A). If none of these were present, the provider selected No contraindications known. This algorithm is published in detail elsewhere and has been shown to not increase major bleeding episodes.[12, 15] The VTE risk assessment, but not the VTE order itself, was a mandatory field. This allowed the physician discretion to choose among various pharmacological agents and mechanical mechanisms based on patient and physician preferences.
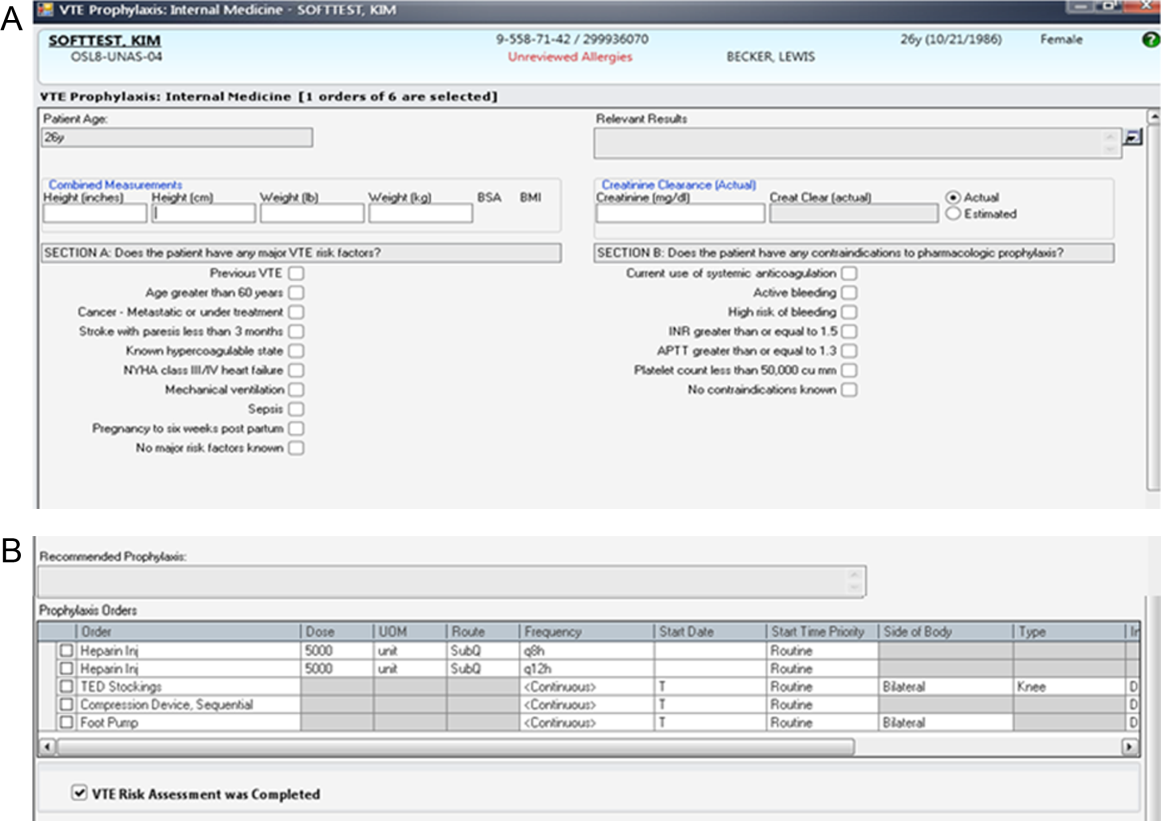
Compliance of risk‐appropriate VTE prophylaxis was determined 24 hours after the admission order set was completed using an automated electronic query of the CPOE system. Low molecular‐weight heparin prescription was included in the compliance algorithm as acceptable prophylaxis. Prescription of pharmacological VTE prophylaxis when a contraindication was present was considered noncompliant. The metric was assigned to the attending physician who billed for the first inpatient encounter.
Pay‐for‐Performance Program
In July 2011, a pay‐for‐performance program was added to the dashboard. All full‐time and part‐time hospitalists were eligible. The financial incentive was determined according to hospital priority and funds available. The VTE prophylaxis metric was prorated by clinical effort, with a maximum of $0.50 per work relative value unit (RVU). To optimize performance, a threshold of 80% compliance had to be surpassed before any payment was made. Progressively increasing percentages of the incentive were earned as compliance increased from 80% to 100%, corresponding to dashboard scores of 6, 7, 8, and 9: <80% (scores 1 to 5)=no payment; 80% to 84.9% (score 6)=$0.125 per RVU; 85% to 89.9% (score 7)=$0.25 per RVU; 90% to 94.9% (score 8)=$0.375 per RVU; and 95% (score 9)=$0.50 per RVU (maximum incentive). Payments were accrued quarterly and paid at the end of the fiscal year as a cumulative, separate performance supplement.
Individualized physician feedback through the dashboard was continued during the pay‐for‐performance period. Average hospitalist group compliance continued to be displayed on the electronic dashboard and was explicitly reviewed at monthly hospitalist meetings.
The VTE prophylaxis order set and data collection and analyses were approved by the Johns Hopkins Medicine Institutional Review Board. The dashboard and pay‐for‐performance program were initiated by the institution as part of a proof of concept quality improvement project.
Analysis
We examined all inpatient admissions to the hospitalist unit from 2008 to 2012. We included patients admitted to and discharged from the hospitalist unit and excluded patients transferred into/out of the unit and encounters with a length of stay <24 hours. VTE prophylaxis orders were queried from the CPOE system 24 hours after the patient was admitted to determine compliance.
After allowing for a run‐in period (2008), we analyzed the change in percent compliance for 3 periods: (1) CPOE‐based VTE order set alone (baseline [BASE], January 2009 to December 2010); (2) group and individual physician feedback using the dashboard (dashboard only [DASH], January to June 2011); and (3) dashboard tied to the pay‐for‐performance program (dashboard with pay‐for‐performance [P4P], July 2011 to December 2012). The CPOE‐based VTE order set was used during all 3 periods. We used the other medical services as a control to ensure that there were no temporal trends toward improved prophylaxis on a service without the intervention. VTE prophylaxis compliance was examined by calculating percent compliance using the same algorithm for the 4 resident‐staffed general medicine service teams at our institution, which utilized the same CPOE system but did not receive the dashboard or pay‐for‐performance interventions. We used locally weighted scatterplot smoothing, a locally weighted regression of percent compliance over time, to graphically display changes in group compliance over time.[21, 22]
We also performed linear regression to assess the rate of change in group compliance and included spline terms that allowed slope to vary for each of the 3 time periods.[23, 24] Clustered analysis accounted for potentially correlated serial measurements of compliance for an individual provider. A separate analysis examined the effect of provider turnover and individual provider improvement during each of the 3 periods. Tests of significance were 2‐sided, with an level of 0.05. Statistical analysis was performed using Stata 12.1 (StataCorp LP, College Station, TX).
RESULTS
Venous Thromboembolism Prophylaxis Compliance
We analyzed 3144 inpatient admissions by 38 hospitalists from 2009 to 2012. The 5 most frequent coded diagnoses were heart failure, acute kidney failure, syncope, pneumonia, and chest pain. Patients had a median length of stay of 3 days [interquartile range: 26]. During the dashboard‐only period, on average, providers improved in compliance by 4% (95% confidence interval [CI]: 35; P<0.001). With the addition of the pay‐for‐performance program, providers improved by an additional 4% (95% CI: 35; P<0.001). Group compliance significantly improved from 86% (95% CI: 8588) during the BASE period of the CPOE‐based VTE order set to 90% (95% CI: 8893) during the DASH period (P=0.01) and 94% (95% CI: 9396) during the subsequent P4P program (P=0.01) (Figure 3). Both inappropriate prophylaxis and lack of prophylaxis, when indicated, resulted in a non‐compliance rating. During the 3 periods, inappropriate prophylaxis decreased from 7.9% to 6.2% to 2.6% during the BASE, DASH, and subsequent P4P periods, respectively. Similarly, lack of prophylaxis when indicated decreased from 6.1% to 3.2% to 3.1% during the BASE, DASH, and subsequent P4P periods, respectively.
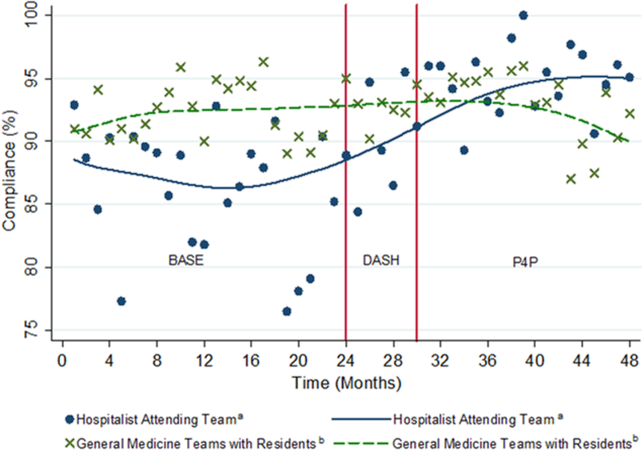
The average compliance of the 4 non‐hospitalist general medicine service teams was initially higher than that of the hospitalist service during the CPOE‐based VTE order set (90%) and DASH (92%) periods, but subsequently plateaued and was exceeded by the hospitalist service during the combined P4P (92%) period (Figure 3). However, there was no statistically significant difference between the general medicine service teams and hospitalist service during the DASH (P=0.15) and subsequent P4P (P=0.76) periods.
We also analyzed the rate of VTE prophylaxis compliance improvement (slope) with cut points at each time period transition (Figure 3). Risk‐appropriate VTE prophylaxis during the BASE period did not exhibit significant improvement as indicated by the slope (P=0.23) (Figure 3). In contrast, during the DASH period, VTE prophylaxis compliance significantly increased by 1.58% per month (95% CI: 0.41‐2.76; P=0.01). The addition of the P4P program, however, did not further significantly increase the rate of compliance (P=0.78).
A subgroup analysis restricted to the 19 providers present during all 3 periods was performed to assess for potential confounding from physician turnover. The percent compliance increased in a similar fashion: BASE period of CPOE‐based VTE order set, 85% (95% CI: 8386); DASH, 90% (95% CI: 8893); and P4P, 94% (95% CI: 9296).
Pay‐for‐Performance Program
Nineteen providers met the threshold for pay‐for‐performance (80% appropriate VTE prophylaxis), with 9 providers in the intermediate categories (80%94.9%) and 10 in the full incentive category (95%). The mean individual payout for the incentive was $633 (standard deviation 350), with a total disbursement of $12,029. The majority of payments (17 of 19) were under $1000.
DISCUSSION
A key component of healthcare reform has been value‐based purchasing, which emphasizes extrinsic motivation through the transparency of performance metrics and use of payment incentives to reward quality. Our study evaluates the impact of both extrinsic (payments) and intrinsic (professionalism and peer norms) motivation. It specifically attributed an individual performance metric, VTE prophylaxis, to an attending physician, provided both individualized and group feedback using an electronic dashboard, and incorporated a pay‐for‐performance program. Prescription of risk‐appropriate VTE prophylaxis significantly increased with the implementation of the dashboard and subsequent pay‐for performance program. The fastest rate of improvement occurred after the addition of the dashboard. Sensitivity analyses for provider turnover and comparisons to the general medicine services showed our results to be independent of a general trend of improvement, both at the provider and institutional levels.
Our prior studies demonstrated that order sets significantly improve performance, from a baseline compliance of risk‐appropriate VTE prophylaxis of 66% to 84%.[13, 15, 25] In the current study, compliance was relatively flat during the BASE period, which included these order sets. The greatest rate of continued improvement in compliance occurred during the DASH period, emphasizing both the importance of provider feedback and receptivity and adaptability in the prescribing behavior of hospitalists. Because the goal of a high‐reliability health system is for 100% of patients to receive recommended therapy, multiple approaches are necessary for success.
Nationally, benchmarks for performance measures continue to be raised, with the highest performers achieving above 95%.[26] Additional interventions, such as dashboards and pay‐for‐performance programs, supplement CPOE systems to achieve high reliability. In our study, the compliance rate during the baseline period, which included a CPOE‐based, clinical support‐enabled VTE order set, was 86%. Initially the compliance of the general medicine teams with residents exceeded that of the hospitalist attending teams, which may reflect a greater willingness of resident teams to comply with order sets and automated recommendations. This emphasizes the importance of continuous individual feedback and provider education at the attending physician level to enhance both guideline compliance and decrease provider care variation. Ultimately, with the addition of the dashboard and subsequent pay‐for‐performance program, compliance was increased to 90% and 94%, respectively. Although the major mechanism used by policymakers to improve quality of care is extrinsic motivation, this study demonstrates that intrinsic motivation through peer norms can enhance extrinsic efforts and may be more influential. Both of these programs, dashboards and pay‐for‐performance, may ultimately assist institutions in changing provider behavior and achieving these harder‐to‐achieve higher benchmarks.
We recognize that there are several limitations to our study. First, this is a single‐site program limited to an attending‐physician‐only service. There was strong data support and a defined CPOE algorithm for this initiative. Multi‐site studies will need to overcome the additional challenges of varying service structures and electronic medical record and provider order entry systems. Second, it is difficult to show actual changes in VTE events over time with appropriate prophylaxis. Although VTE prophylaxis is recommended for patients with VTE risk factors, there are conflicting findings about whether prophylaxis prevents VTE events in lower‐risk patients, and current studies suggest that most patients with VTE events are severely ill and develop VTE despite receiving prophylaxis.[27, 28, 29] Our study was underpowered to detect these potential differences in VTE rates, and although the algorithm has been shown to not increase bleeding rates, we did not measure bleeding rates during this study.[12, 15] Our institutional experience suggests that the majority of VTE events occur despite appropriate prophylaxis.[30] Also, VTE prophylaxis may be ordered, but intervening events, such as procedures and changes in risk status or patient refusal, may prevent patients from receiving appropriate prophylaxis.[31, 32] Similarly, hospitals with higher quality scores have higher VTE prophylaxis rates but worse risk‐adjusted VTE rates, which may result from increased surveillance for VTE, suggesting surveillance bias limits the usefulness of the VTE quality measure.[33, 34] Nevertheless, VTE prophylaxis remains a publicly reported Core Measure tied to financial incentives.[4, 5] Third, there may be an unmeasured factor specific to the hospitalist program, which could potentially account for an overall improvement in quality of care. Although the rate of increase in appropriate prophylaxis was not statistically significant during the baseline period, there did appear to be some improvement in prophylaxis toward the end of the period. However, there were no other VTE‐related provider feedback programs being simultaneously pursued during this study. VTE prophylaxis for the non‐hospitalist services showed a relatively stable, non‐increasing compliance rate for the general medical services. Although it was possible for successful residents to age into the hospitalist service, thereby improving rates of prophylaxis based on changes in group makeup, our subgroup analysis of the providers present throughout all phases of the study showed our results to be robust. Similarly, there may have been a cross‐contamination effect of hospitalist faculty who attended on both hospitalist and non‐hospitalist general medicine service teams. This, however, would attenuate any impact of the programs, and thus the effects may in fact be greater than reported. Fourth, establishment of both the dashboard and pay‐for‐performance program required significant institutional and program leadership and resources. To be successful, the dashboard must be in the provider's workflow, transparent, minimize reporter burden, use existing systems, and be actively fed back to providers, ideally those directly entering orders. Our greatest rate of improvement occurred during the feedback‐only phase of this study, emphasizing the importance of physician feedback, provider‐level accountability, and engagement. We suspect that the relatively modest pay‐for‐performance incentive served mainly as a means of engaging providers in self‐monitoring, rather than as a means to change behavior through true incentivization. Although we did not track individual physician views of the dashboard, we reinforced trends, deviations, and expectations at regularly scheduled meetings and provided feedback and patient‐level data to individual providers. Fifth, the design of the pay‐for‐performance program may have also influenced its effectiveness. These types of programs may be more effective when they provide frequent visible, small payments rather than one large payment, and when the payment is framed as a loss rather than a gain.[35] Finally, physician champions and consistent feedback through departmental meetings or visual displays may be required for program success. The initial resources to create the dashboard, continued maintenance and monitoring of performance, and payment of financial incentives all require institutional commitment. A partnership of physicians, program leaders, and institutional administrators is necessary for both initial and continued success.
To achieve performance goals and benchmarks, multiple strategies that combine extrinsic and intrinsic motivation are necessary. As shown by our study, the use of a dashboard and pay‐for‐performance can be tailored to an institution's goals, in line with national standards. The specific goal (risk‐appropriate VTE prophylaxis) and benchmarks (80%, 85%, 90%, 95%) can be individualized to a particular institution. For example, if readmission rates are above target, readmissions could be added as a dashboard metric. The specific benchmark would be determined by historical trends and administrative targets. Similarly, the overall financial incentives could be adjusted based on the financial resources available. Other process measures, such as influenza vaccination screening and administration, could also be targeted. For all of these objectives, continued provider feedback and engagement are critical for progressive success, especially to decrease variability in care at the attending physician level. Incorporating the value‐based purchasing philosophy from the Affordable Care Act, our study suggests that the combination of standardized order sets, real‐time dashboards, and physician‐level incentives may assist hospitals in achieving quality and safety benchmarks, especially at higher targets.
Acknowledgements
The authors thank Meir Gottlieb, BS, from Salar Inc. for data support; Murali Padmanaban, BS, from Johns Hopkins University for his assistance in linking the administrative billing data with real‐time physician orders; and Hsin‐Chieh Yeh, PhD, from the Bloomberg School of Public Health for her statistical advice and additional review. We also thank Mr. Ronald R. Peterson, President, Johns Hopkins Health System and Johns Hopkins Hospital, for providing funding support for the physician incentive payments.
Disclosures: Drs. Michtalik and Brotman had full access to all of the data in the study and take responsibility for the integrity of the data and accuracy of the data analysis. Study concept and design: Drs. Michtalik, Streiff, Finkelstein, Pronovost, and Brotman. Acquisition of data: Drs. Michtalik, Streiff, Brotman and Mr. Carolan, Mr. Lau, Mrs. Durkin. Analysis and interpretation of data: Drs. Michtalik, Haut, Streiff, Brotman and Mr. Carolan, Mr. Lau. Drafting of the manuscript: Drs. Michtalik and Brotman. Critical revision of the manuscript for important intellectual content: Drs. Michtalik, Haut, Streiff, Finkelstein, Pronovost, Brotman and Mr. Carolan, Mr. Lau, Mrs. Durkin. Statistical analysis and supervision: Drs. Michtalik and Brotman. Obtaining funding: Drs. Streiff and Brotman. Technical support: Dr. Streiff and Mr. Carolan, Mr. Lau, Mrs. Durkin
This study was supported by a National Institutes of Health grant T32 HP10025‐17‐00 (Dr. Michtalik), the National Institutes of Health/Johns Hopkins Institute for Clinical and Translational Research KL2 Award 5KL2RR025006 (Dr. Michtalik), the Agency for Healthcare Research and Quality Mentored Clinical Scientist Development K08 Awards 1K08HS017952‐01 (Dr. Haut) and 1K08HS022331‐01A1 (Dr. Michtalik), and the Johns Hopkins Hospitalist Scholars Fund. The funders had no role in the design and conduct of the study; collection, management, analysis, and interpretation of the data; preparation, review, or approval of the manuscript; and decision to submit the manuscript for publication.
Dr. Haut receives royalties from Lippincott, Williams & Wilkins. Dr. Streiff has received research funding from Portola and Bristol Myers Squibb, honoraria for CME lectures from Sanofi‐Aventis and Ortho‐McNeil, consulted for Eisai, Daiichi‐Sankyo, Boerhinger‐Ingelheim, Janssen Healthcare, and Pfizer. Mr. Lau, Drs. Haut, Streiff, and Pronovost are supported by a contract from the Patient‐Centered Outcomes Research Institute (PCORI) titled Preventing Venous Thromboembolism: Empowering Patients and Enabling Patient‐Centered Care via Health Information Technology (CE‐12‐11‐4489). Dr. Brotman has received research support from Siemens Healthcare Diagnostics, Bristol‐Myers Squibb, the Agency for Healthcare Research and Quality, Centers for Medicare & Medicaid Services, the Amerigroup Corporation, and the Guerrieri Family Foundation. He has received honoraria from the Gerson Lehrman Group, the Dunn Group, and from Quantia Communications, and received royalties from McGraw‐Hill.
The Affordable Care Act explicitly outlines improving the value of healthcare by increasing quality and decreasing costs. It emphasizes value‐based purchasing, the transparency of performance metrics, and the use of payment incentives to reward quality.[1, 2] Venous thromboembolism (VTE) prophylaxis is one of these publicly reported performance measures. The National Quality Forum recommends that each patient be evaluated on hospital admission and during their hospitalization for VTE risk level and for appropriate thromboprophylaxis to be used, if required.[3] Similarly, the Joint Commission includes appropriate VTE prophylaxis in its Core Measures.[4] Patient experience and performance metrics, including VTE prophylaxis, constitute the hospital value‐based purchasing (VBP) component of healthcare reform.[5] For a hypothetical 327‐bed hospital, an estimated $1.7 million of a hospital's inpatient payments from Medicare will be at risk from VBP alone.[2]
VTE prophylaxis is a common target of quality improvement projects. Effective, safe, and cost‐effective measures to prevent VTE exist, including pharmacologic and mechanical prophylaxis.[6, 7] Despite these measures, compliance rates are often below 50%.[8] Different interventions have been pursued to ensure appropriate VTE prophylaxis, including computerized provider order entry (CPOE), electronic alerts, mandatory VTE risk assessment and prophylaxis, and provider education campaigns.[9] Recent studies show that CPOE systems with mandatory fields can increase VTE prophylaxis rates to above 80%, yet the goal of a high reliability health system is for 100% of patients to receive recommended therapy.[10, 11, 12, 13, 14, 15] Interventions to improve prophylaxis rates that have included multiple strategies, such as computerized order sets, feedback, and education, have been the most effective, increasing compliance to above 90%.[9, 11, 16] These systems can be enhanced with additional interventions such as providing individualized provider education and feedback, understanding of work flow, and ensuring patients receive the prescribed therapies.[12] For example, a physician dashboard could be employed to provide a snapshot and historical trend of key performance indicators using graphical displays and indicators.[17]
Dashboards and pay‐for‐performance programs have been increasingly used to increase the visibility of these metrics, provide feedback, visually display benchmarks and goals, and proactively monitor for achievements and setbacks.[18] Although these strategies are often addressed at departmental (or greater) levels, applying them at the level of the individual provider may assist hospitals in reducing preventable harm and achieving safety and quality goals, especially at higher benchmarks. With their expanding role, hospitalists provide a key opportunity to lead improvement efforts and to study the impact of dashboards and pay‐for performance at the provider level to achieve VTE prophylaxis performance targets. Hospitalists are often the front‐line provider for inpatients and deliver up to 70% of inpatient general medical services.[19] The objective of our study was to evaluate the impact of providing individual provider feedback and employing a pay‐for‐performance program on baseline performance of VTE prophylaxis among hospitalists. We hypothesized that performance feedback through the use of a dashboard would increase appropriate VTE prophylaxis, and this effect would be further augmented by incorporation of a pay‐for‐performance program.
METHODS
Hospitalist Dashboard
In 2010, hospitalist program leaders met with hospital administrators to create a hospitalist dashboard that would provide regularly updated summaries of performance measures for individual hospitalists. The final set of metrics identified included appropriate VTE prophylaxis, length of stay, patients discharged per day, discharges before 3 pm, depth of coding, patient satisfaction, readmissions, communication with the primary care provider, and time to signature for discharge summaries (Figure 1A). The dashboard was introduced at a general hospitalist meeting during which its purpose, methodology, and accessibility were described; it was subsequently implemented in January 2011.
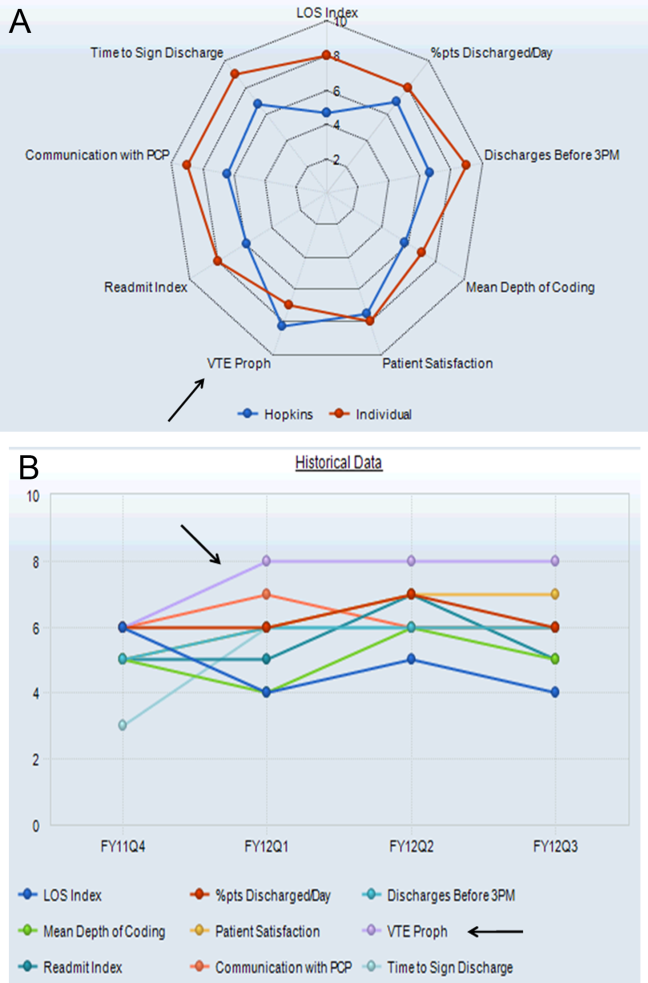
Benchmarks were established for each metric, standardized to establish a scale ranging from 1 through 9, and incorporated into the dashboard (Figure 1A). Higher scores (creating a larger geometric shape) were desirable. For the VTE prophylaxis measure, scores of 1 through 9 corresponded to <60%, 60% to 64.9%, 65% to 69.9%, 70% to 74.9%, 75% to 79.9%, 80% to 84.9%, 85% to 89.9%, 90% to 94.9%, and 95% American College of Chest Physicians (ACCP)‐compliant VTE prophylaxis, respectively.[12, 20] Each provider was able to access the aggregated dashboard (showing the group mean) and his/her individualized dashboard using an individualized login and password for the institutional portal. This portal is used during the provider's workflow, including medical record review and order entry. Both a polygonal summary graphic (Figure 1A) and trend (Figure 1B) view of the dashboard were available to the provider. A comparison of the individual provider to the hospitalist group average was displayed (Figure 1A). At monthly program meetings, the dashboard, group results, and trends were discussed.
Venous Thromboembolism Prophylaxis Compliance
Our study was performed in a tertiary academic medical center with an approximately 20‐member hospitalist group (the precise membership varied over time), whose responsibilities include, among other clinical duties, staffing a 17‐bed general medicine unit with telemetry. The scope of diagnoses and acuity of patients admitted to the hospitalist service is similar to the housestaff services. Some hospitalist faculty serve both as hospitalist and nonhospitalist general medicine service team attendings, but the comparison groups were staffed by hospitalists for <20% of the time. For admissions, all hospitalists use a standardized general medicine admission order set that is integrated into the CPOE system (Sunrise Clinical Manager; Allscripts, Chicago, IL) and completed for all admitted patients. A mandatory VTE risk screen, which includes an assessment of VTE risk factors and pharmacological prophylaxis contraindications, must be completed by the ordering physician as part of this order set (Figure 2A). The system then prompts the provider with a risk‐appropriate VTE prophylaxis recommendation that the provider may subsequently order, including mechanical prophylaxis (Figure 2B). Based on ACCP VTE prevention guidelines, risk‐appropriate prophylaxis was determined using an electronic algorithm that categorized patients into risk categories based on the presence of major VTE risk factors (Figure 2A).[12, 15, 20] If none of these were present, the provider selected No major risk factors known. Both an assessment of current use of anticoagulation and a clinically high risk of bleeding were also included (Figure 2A). If none of these were present, the provider selected No contraindications known. This algorithm is published in detail elsewhere and has been shown to not increase major bleeding episodes.[12, 15] The VTE risk assessment, but not the VTE order itself, was a mandatory field. This allowed the physician discretion to choose among various pharmacological agents and mechanical mechanisms based on patient and physician preferences.
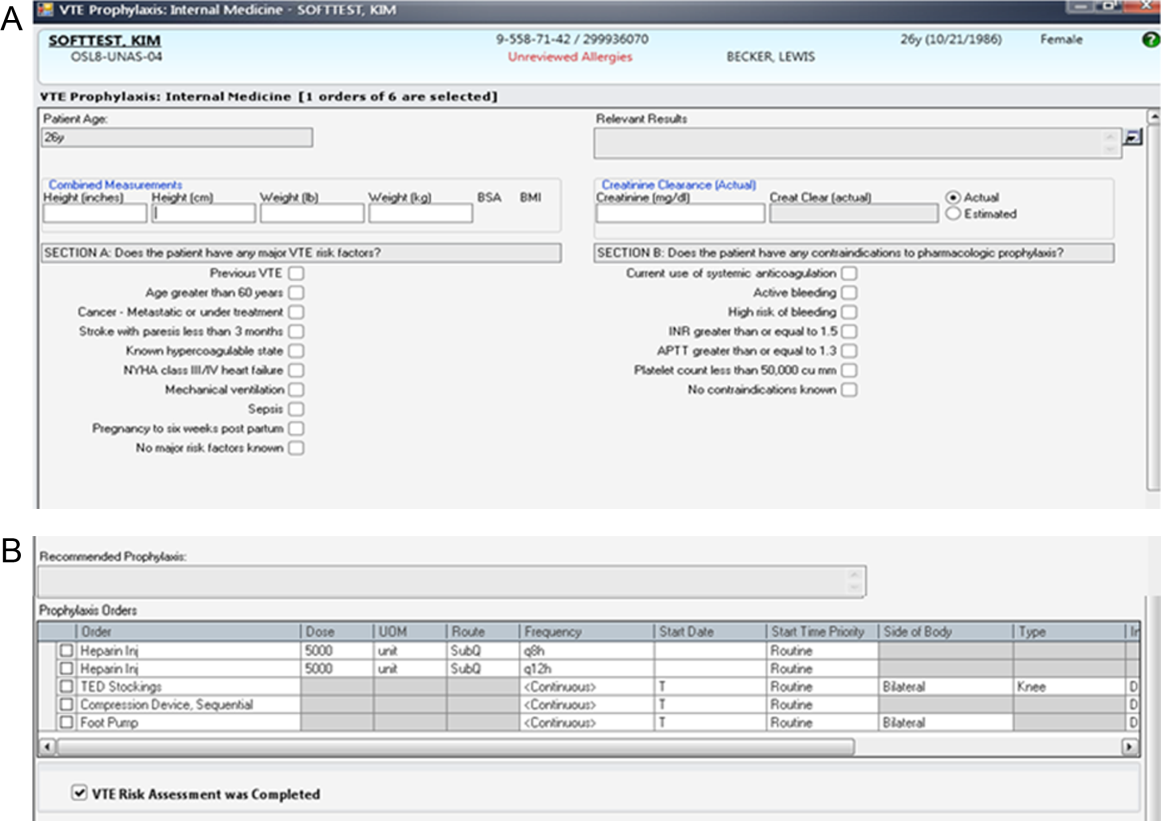
Compliance of risk‐appropriate VTE prophylaxis was determined 24 hours after the admission order set was completed using an automated electronic query of the CPOE system. Low molecular‐weight heparin prescription was included in the compliance algorithm as acceptable prophylaxis. Prescription of pharmacological VTE prophylaxis when a contraindication was present was considered noncompliant. The metric was assigned to the attending physician who billed for the first inpatient encounter.
Pay‐for‐Performance Program
In July 2011, a pay‐for‐performance program was added to the dashboard. All full‐time and part‐time hospitalists were eligible. The financial incentive was determined according to hospital priority and funds available. The VTE prophylaxis metric was prorated by clinical effort, with a maximum of $0.50 per work relative value unit (RVU). To optimize performance, a threshold of 80% compliance had to be surpassed before any payment was made. Progressively increasing percentages of the incentive were earned as compliance increased from 80% to 100%, corresponding to dashboard scores of 6, 7, 8, and 9: <80% (scores 1 to 5)=no payment; 80% to 84.9% (score 6)=$0.125 per RVU; 85% to 89.9% (score 7)=$0.25 per RVU; 90% to 94.9% (score 8)=$0.375 per RVU; and 95% (score 9)=$0.50 per RVU (maximum incentive). Payments were accrued quarterly and paid at the end of the fiscal year as a cumulative, separate performance supplement.
Individualized physician feedback through the dashboard was continued during the pay‐for‐performance period. Average hospitalist group compliance continued to be displayed on the electronic dashboard and was explicitly reviewed at monthly hospitalist meetings.
The VTE prophylaxis order set and data collection and analyses were approved by the Johns Hopkins Medicine Institutional Review Board. The dashboard and pay‐for‐performance program were initiated by the institution as part of a proof of concept quality improvement project.
Analysis
We examined all inpatient admissions to the hospitalist unit from 2008 to 2012. We included patients admitted to and discharged from the hospitalist unit and excluded patients transferred into/out of the unit and encounters with a length of stay <24 hours. VTE prophylaxis orders were queried from the CPOE system 24 hours after the patient was admitted to determine compliance.
After allowing for a run‐in period (2008), we analyzed the change in percent compliance for 3 periods: (1) CPOE‐based VTE order set alone (baseline [BASE], January 2009 to December 2010); (2) group and individual physician feedback using the dashboard (dashboard only [DASH], January to June 2011); and (3) dashboard tied to the pay‐for‐performance program (dashboard with pay‐for‐performance [P4P], July 2011 to December 2012). The CPOE‐based VTE order set was used during all 3 periods. We used the other medical services as a control to ensure that there were no temporal trends toward improved prophylaxis on a service without the intervention. VTE prophylaxis compliance was examined by calculating percent compliance using the same algorithm for the 4 resident‐staffed general medicine service teams at our institution, which utilized the same CPOE system but did not receive the dashboard or pay‐for‐performance interventions. We used locally weighted scatterplot smoothing, a locally weighted regression of percent compliance over time, to graphically display changes in group compliance over time.[21, 22]
We also performed linear regression to assess the rate of change in group compliance and included spline terms that allowed slope to vary for each of the 3 time periods.[23, 24] Clustered analysis accounted for potentially correlated serial measurements of compliance for an individual provider. A separate analysis examined the effect of provider turnover and individual provider improvement during each of the 3 periods. Tests of significance were 2‐sided, with an level of 0.05. Statistical analysis was performed using Stata 12.1 (StataCorp LP, College Station, TX).
RESULTS
Venous Thromboembolism Prophylaxis Compliance
We analyzed 3144 inpatient admissions by 38 hospitalists from 2009 to 2012. The 5 most frequent coded diagnoses were heart failure, acute kidney failure, syncope, pneumonia, and chest pain. Patients had a median length of stay of 3 days [interquartile range: 26]. During the dashboard‐only period, on average, providers improved in compliance by 4% (95% confidence interval [CI]: 35; P<0.001). With the addition of the pay‐for‐performance program, providers improved by an additional 4% (95% CI: 35; P<0.001). Group compliance significantly improved from 86% (95% CI: 8588) during the BASE period of the CPOE‐based VTE order set to 90% (95% CI: 8893) during the DASH period (P=0.01) and 94% (95% CI: 9396) during the subsequent P4P program (P=0.01) (Figure 3). Both inappropriate prophylaxis and lack of prophylaxis, when indicated, resulted in a non‐compliance rating. During the 3 periods, inappropriate prophylaxis decreased from 7.9% to 6.2% to 2.6% during the BASE, DASH, and subsequent P4P periods, respectively. Similarly, lack of prophylaxis when indicated decreased from 6.1% to 3.2% to 3.1% during the BASE, DASH, and subsequent P4P periods, respectively.
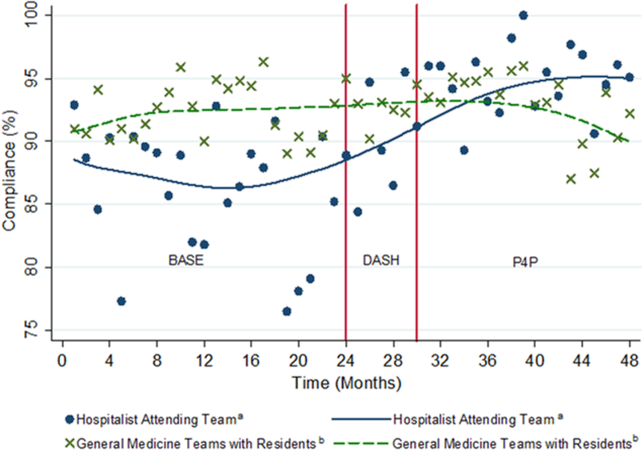
The average compliance of the 4 non‐hospitalist general medicine service teams was initially higher than that of the hospitalist service during the CPOE‐based VTE order set (90%) and DASH (92%) periods, but subsequently plateaued and was exceeded by the hospitalist service during the combined P4P (92%) period (Figure 3). However, there was no statistically significant difference between the general medicine service teams and hospitalist service during the DASH (P=0.15) and subsequent P4P (P=0.76) periods.
We also analyzed the rate of VTE prophylaxis compliance improvement (slope) with cut points at each time period transition (Figure 3). Risk‐appropriate VTE prophylaxis during the BASE period did not exhibit significant improvement as indicated by the slope (P=0.23) (Figure 3). In contrast, during the DASH period, VTE prophylaxis compliance significantly increased by 1.58% per month (95% CI: 0.41‐2.76; P=0.01). The addition of the P4P program, however, did not further significantly increase the rate of compliance (P=0.78).
A subgroup analysis restricted to the 19 providers present during all 3 periods was performed to assess for potential confounding from physician turnover. The percent compliance increased in a similar fashion: BASE period of CPOE‐based VTE order set, 85% (95% CI: 8386); DASH, 90% (95% CI: 8893); and P4P, 94% (95% CI: 9296).
Pay‐for‐Performance Program
Nineteen providers met the threshold for pay‐for‐performance (80% appropriate VTE prophylaxis), with 9 providers in the intermediate categories (80%94.9%) and 10 in the full incentive category (95%). The mean individual payout for the incentive was $633 (standard deviation 350), with a total disbursement of $12,029. The majority of payments (17 of 19) were under $1000.
DISCUSSION
A key component of healthcare reform has been value‐based purchasing, which emphasizes extrinsic motivation through the transparency of performance metrics and use of payment incentives to reward quality. Our study evaluates the impact of both extrinsic (payments) and intrinsic (professionalism and peer norms) motivation. It specifically attributed an individual performance metric, VTE prophylaxis, to an attending physician, provided both individualized and group feedback using an electronic dashboard, and incorporated a pay‐for‐performance program. Prescription of risk‐appropriate VTE prophylaxis significantly increased with the implementation of the dashboard and subsequent pay‐for performance program. The fastest rate of improvement occurred after the addition of the dashboard. Sensitivity analyses for provider turnover and comparisons to the general medicine services showed our results to be independent of a general trend of improvement, both at the provider and institutional levels.
Our prior studies demonstrated that order sets significantly improve performance, from a baseline compliance of risk‐appropriate VTE prophylaxis of 66% to 84%.[13, 15, 25] In the current study, compliance was relatively flat during the BASE period, which included these order sets. The greatest rate of continued improvement in compliance occurred during the DASH period, emphasizing both the importance of provider feedback and receptivity and adaptability in the prescribing behavior of hospitalists. Because the goal of a high‐reliability health system is for 100% of patients to receive recommended therapy, multiple approaches are necessary for success.
Nationally, benchmarks for performance measures continue to be raised, with the highest performers achieving above 95%.[26] Additional interventions, such as dashboards and pay‐for‐performance programs, supplement CPOE systems to achieve high reliability. In our study, the compliance rate during the baseline period, which included a CPOE‐based, clinical support‐enabled VTE order set, was 86%. Initially the compliance of the general medicine teams with residents exceeded that of the hospitalist attending teams, which may reflect a greater willingness of resident teams to comply with order sets and automated recommendations. This emphasizes the importance of continuous individual feedback and provider education at the attending physician level to enhance both guideline compliance and decrease provider care variation. Ultimately, with the addition of the dashboard and subsequent pay‐for‐performance program, compliance was increased to 90% and 94%, respectively. Although the major mechanism used by policymakers to improve quality of care is extrinsic motivation, this study demonstrates that intrinsic motivation through peer norms can enhance extrinsic efforts and may be more influential. Both of these programs, dashboards and pay‐for‐performance, may ultimately assist institutions in changing provider behavior and achieving these harder‐to‐achieve higher benchmarks.
We recognize that there are several limitations to our study. First, this is a single‐site program limited to an attending‐physician‐only service. There was strong data support and a defined CPOE algorithm for this initiative. Multi‐site studies will need to overcome the additional challenges of varying service structures and electronic medical record and provider order entry systems. Second, it is difficult to show actual changes in VTE events over time with appropriate prophylaxis. Although VTE prophylaxis is recommended for patients with VTE risk factors, there are conflicting findings about whether prophylaxis prevents VTE events in lower‐risk patients, and current studies suggest that most patients with VTE events are severely ill and develop VTE despite receiving prophylaxis.[27, 28, 29] Our study was underpowered to detect these potential differences in VTE rates, and although the algorithm has been shown to not increase bleeding rates, we did not measure bleeding rates during this study.[12, 15] Our institutional experience suggests that the majority of VTE events occur despite appropriate prophylaxis.[30] Also, VTE prophylaxis may be ordered, but intervening events, such as procedures and changes in risk status or patient refusal, may prevent patients from receiving appropriate prophylaxis.[31, 32] Similarly, hospitals with higher quality scores have higher VTE prophylaxis rates but worse risk‐adjusted VTE rates, which may result from increased surveillance for VTE, suggesting surveillance bias limits the usefulness of the VTE quality measure.[33, 34] Nevertheless, VTE prophylaxis remains a publicly reported Core Measure tied to financial incentives.[4, 5] Third, there may be an unmeasured factor specific to the hospitalist program, which could potentially account for an overall improvement in quality of care. Although the rate of increase in appropriate prophylaxis was not statistically significant during the baseline period, there did appear to be some improvement in prophylaxis toward the end of the period. However, there were no other VTE‐related provider feedback programs being simultaneously pursued during this study. VTE prophylaxis for the non‐hospitalist services showed a relatively stable, non‐increasing compliance rate for the general medical services. Although it was possible for successful residents to age into the hospitalist service, thereby improving rates of prophylaxis based on changes in group makeup, our subgroup analysis of the providers present throughout all phases of the study showed our results to be robust. Similarly, there may have been a cross‐contamination effect of hospitalist faculty who attended on both hospitalist and non‐hospitalist general medicine service teams. This, however, would attenuate any impact of the programs, and thus the effects may in fact be greater than reported. Fourth, establishment of both the dashboard and pay‐for‐performance program required significant institutional and program leadership and resources. To be successful, the dashboard must be in the provider's workflow, transparent, minimize reporter burden, use existing systems, and be actively fed back to providers, ideally those directly entering orders. Our greatest rate of improvement occurred during the feedback‐only phase of this study, emphasizing the importance of physician feedback, provider‐level accountability, and engagement. We suspect that the relatively modest pay‐for‐performance incentive served mainly as a means of engaging providers in self‐monitoring, rather than as a means to change behavior through true incentivization. Although we did not track individual physician views of the dashboard, we reinforced trends, deviations, and expectations at regularly scheduled meetings and provided feedback and patient‐level data to individual providers. Fifth, the design of the pay‐for‐performance program may have also influenced its effectiveness. These types of programs may be more effective when they provide frequent visible, small payments rather than one large payment, and when the payment is framed as a loss rather than a gain.[35] Finally, physician champions and consistent feedback through departmental meetings or visual displays may be required for program success. The initial resources to create the dashboard, continued maintenance and monitoring of performance, and payment of financial incentives all require institutional commitment. A partnership of physicians, program leaders, and institutional administrators is necessary for both initial and continued success.
To achieve performance goals and benchmarks, multiple strategies that combine extrinsic and intrinsic motivation are necessary. As shown by our study, the use of a dashboard and pay‐for‐performance can be tailored to an institution's goals, in line with national standards. The specific goal (risk‐appropriate VTE prophylaxis) and benchmarks (80%, 85%, 90%, 95%) can be individualized to a particular institution. For example, if readmission rates are above target, readmissions could be added as a dashboard metric. The specific benchmark would be determined by historical trends and administrative targets. Similarly, the overall financial incentives could be adjusted based on the financial resources available. Other process measures, such as influenza vaccination screening and administration, could also be targeted. For all of these objectives, continued provider feedback and engagement are critical for progressive success, especially to decrease variability in care at the attending physician level. Incorporating the value‐based purchasing philosophy from the Affordable Care Act, our study suggests that the combination of standardized order sets, real‐time dashboards, and physician‐level incentives may assist hospitals in achieving quality and safety benchmarks, especially at higher targets.
Acknowledgements
The authors thank Meir Gottlieb, BS, from Salar Inc. for data support; Murali Padmanaban, BS, from Johns Hopkins University for his assistance in linking the administrative billing data with real‐time physician orders; and Hsin‐Chieh Yeh, PhD, from the Bloomberg School of Public Health for her statistical advice and additional review. We also thank Mr. Ronald R. Peterson, President, Johns Hopkins Health System and Johns Hopkins Hospital, for providing funding support for the physician incentive payments.
Disclosures: Drs. Michtalik and Brotman had full access to all of the data in the study and take responsibility for the integrity of the data and accuracy of the data analysis. Study concept and design: Drs. Michtalik, Streiff, Finkelstein, Pronovost, and Brotman. Acquisition of data: Drs. Michtalik, Streiff, Brotman and Mr. Carolan, Mr. Lau, Mrs. Durkin. Analysis and interpretation of data: Drs. Michtalik, Haut, Streiff, Brotman and Mr. Carolan, Mr. Lau. Drafting of the manuscript: Drs. Michtalik and Brotman. Critical revision of the manuscript for important intellectual content: Drs. Michtalik, Haut, Streiff, Finkelstein, Pronovost, Brotman and Mr. Carolan, Mr. Lau, Mrs. Durkin. Statistical analysis and supervision: Drs. Michtalik and Brotman. Obtaining funding: Drs. Streiff and Brotman. Technical support: Dr. Streiff and Mr. Carolan, Mr. Lau, Mrs. Durkin
This study was supported by a National Institutes of Health grant T32 HP10025‐17‐00 (Dr. Michtalik), the National Institutes of Health/Johns Hopkins Institute for Clinical and Translational Research KL2 Award 5KL2RR025006 (Dr. Michtalik), the Agency for Healthcare Research and Quality Mentored Clinical Scientist Development K08 Awards 1K08HS017952‐01 (Dr. Haut) and 1K08HS022331‐01A1 (Dr. Michtalik), and the Johns Hopkins Hospitalist Scholars Fund. The funders had no role in the design and conduct of the study; collection, management, analysis, and interpretation of the data; preparation, review, or approval of the manuscript; and decision to submit the manuscript for publication.
Dr. Haut receives royalties from Lippincott, Williams & Wilkins. Dr. Streiff has received research funding from Portola and Bristol Myers Squibb, honoraria for CME lectures from Sanofi‐Aventis and Ortho‐McNeil, consulted for Eisai, Daiichi‐Sankyo, Boerhinger‐Ingelheim, Janssen Healthcare, and Pfizer. Mr. Lau, Drs. Haut, Streiff, and Pronovost are supported by a contract from the Patient‐Centered Outcomes Research Institute (PCORI) titled Preventing Venous Thromboembolism: Empowering Patients and Enabling Patient‐Centered Care via Health Information Technology (CE‐12‐11‐4489). Dr. Brotman has received research support from Siemens Healthcare Diagnostics, Bristol‐Myers Squibb, the Agency for Healthcare Research and Quality, Centers for Medicare & Medicaid Services, the Amerigroup Corporation, and the Guerrieri Family Foundation. He has received honoraria from the Gerson Lehrman Group, the Dunn Group, and from Quantia Communications, and received royalties from McGraw‐Hill.
- Medicare Program, Centers for Medicare 76(88):26490–26547.
- Quality meets finance: payments at risk with value‐based purchasing, readmission, and hospital‐acquired conditions force hospitalists to focus. Hospitalist. 2013;17(1):31. .
- National Quality Forum. March 2009. Safe practices for better healthcare—2009 update. Available at: http://www.qualityforum.org/Publications/2009/03/Safe_Practices_for_Better_Healthcare%E2%80%932009_Update.aspx. Accessed November 1, 2014.
- Joint Commission on Accreditation of Healthcare Organizations. Approved: more options for hospital core measures. Jt Comm Perspect. 2009;29(4):1–6.
- Centers for Medicare 208(2):227–240.
- Thromboprophylaxis in nonsurgical patients. Hematology Am Soc Hematol Educ Program. 2012;2012:631–637. , .
- Venous thromboembolism risk and prophylaxis in the acute hospital care setting (ENDORSE study): a multinational cross‐sectional study. Lancet. 2008;371(9610):387–394. , , , et al.
- Practices to prevent venous thromboembolism: a brief review. BMJ Qual Saf. 2014;23(3):187–195. , .
- Improving hospital venous thromboembolism prophylaxis with electronic decision support. J Hosp Med. 2013;8(3):115–120. , , , et al.
- Innovative approaches to increase deep vein thrombosis prophylaxis rate resulting in a decrease in hospital‐acquired deep vein thrombosis at a tertiary‐care teaching hospital. J Hosp Med. 2008;3(2):148–155. , , .
- Lessons from the Johns Hopkins Multi‐Disciplinary Venous Thromboembolism (VTE) Prevention Collaborative. BMJ. 2012;344:e3935. , , , et al.
- Improved prophylaxis and decreased rates of preventable harm with the use of a mandatory computerized clinical decision support tool for prophylaxis for venous thromboembolism in trauma. Arch Surg. 2012;147(10):901–907. , , , et al.
- Designing and implementing effective venous thromboembolism prevention protocols: lessons from collaborative efforts. J Thromb Thrombolysis. 2010;29(2):159–166. , .
- Impact of a venous thromboembolism prophylaxis "smart order set": improved compliance, fewer events. Am J Hematol. 2013;88(7):545–549. , , , et al.
- Improving adherence to venous thromoembolism prophylaxis using multiple interventions. BMJ. 2012;344:e3935. , .
- Health Resources and Services Administration of the U.S. Department of Health and Human Services. Managing data for performance improvement. Available at: http://www.hrsa.gov/quality/toolbox/methodology/performanceimprovement/part2.html. Accessed December 18, 2014.
- Improving patient safety by taking systems seriously. JAMA. 2008;299(4):445–447. , .
- Growth in the care of older patients by hospitalists in the United States. N Engl J Med. 2009;360(11):1102–1112. , , , .
- Prevention of venous thromboembolism: American College of Chest Physicians evidence‐based clinical practice guidelines (8th edition). Chest. 2008;133(6 suppl):381S–453S. , , , et al.
- Robust locally weighted regression and smoothing scatterplots. J Am Stat Assoc. 1979;74(368):829–836. .
- Locally weighted regression: An approach to regression analysis by local fitting. J Am Stat Assoc. 1988;83(403):596–610. , .
- Regression Methods in Biostatistics: Linear, Logistic, Survival, and Repeated Measures Models. 2nd ed. New York, NY: Springer; 2012. , , , .
- Regression Modeling Strategies: With Applications to Linear Models, Logistic Regression, and Survival Analysis. New York, NY: Springer‐Verlag; 2001. .
- Med Care. doi: 10.1097/MLR.0000000000000251. , , , et al. Eliminating healthcare disparities via mandatory clinical decision support: the venous thromboembolism (VTE) example [published online ahead of print November 4, 2014].
- Joint Commission. Improving America's hospitals: the Joint Commission's annual report on quality and safety. 2012. Available at: http://www.jointcommission.org/assets/1/18/TJC_Annual_Report_2012.pdf. Accessed September 8, 2013.
- Hospital performance for pharmacologic venous thromboembolism prophylaxis and rate of venous thromboembolism: a cohort study. JAMA Intern Med. 2014;174(10):1577–1584. , , , et al.
- Incidence of hospital‐acquired venous thromboembolic codes in medical patients hospitalized in academic medical centers. J Hosp Med. 2014;9(4):221–225. , , , et al.
- No association between hospital‐reported perioperative venous thromboembolism prophylaxis and outcome rates in publicly reported data. JAMA Surg. 2014;149(4):400–401. , , , , .
- Linking processes and outcomes: a key strategy to prevent and report harm from venous thromboembolism in surgical patients. JAMA Surg. 2013;148(3):299–300. , , , , .
- Patterns of non‐administration of ordered doses of venous thromboembolism prophylaxis: implications for novel intervention strategies. PLoS One. 2013;8(6):e66311. , , , et al.
- Nonadministration of thromboprophylaxis in hospitalized patients with HIV: a missed opportunity for prevention? J Hosp Med. 2014;9(4):215–220. , , , et al.
- Evaluation of surveillance bias and the validity of the venous thromboembolism quality measure. JAMA. 2013;310(14):1482–1489. , , , et al.
- Surveillance bias in outcomes reporting. JAMA. 2011;305(23):2462–2463. , .
- Pay for performance in health care: an international overview of initiatives. Med Care Res Rev. 2012;69(3):251–276. .
- Medicare Program, Centers for Medicare 76(88):26490–26547.
- Quality meets finance: payments at risk with value‐based purchasing, readmission, and hospital‐acquired conditions force hospitalists to focus. Hospitalist. 2013;17(1):31. .
- National Quality Forum. March 2009. Safe practices for better healthcare—2009 update. Available at: http://www.qualityforum.org/Publications/2009/03/Safe_Practices_for_Better_Healthcare%E2%80%932009_Update.aspx. Accessed November 1, 2014.
- Joint Commission on Accreditation of Healthcare Organizations. Approved: more options for hospital core measures. Jt Comm Perspect. 2009;29(4):1–6.
- Centers for Medicare 208(2):227–240.
- Thromboprophylaxis in nonsurgical patients. Hematology Am Soc Hematol Educ Program. 2012;2012:631–637. , .
- Venous thromboembolism risk and prophylaxis in the acute hospital care setting (ENDORSE study): a multinational cross‐sectional study. Lancet. 2008;371(9610):387–394. , , , et al.
- Practices to prevent venous thromboembolism: a brief review. BMJ Qual Saf. 2014;23(3):187–195. , .
- Improving hospital venous thromboembolism prophylaxis with electronic decision support. J Hosp Med. 2013;8(3):115–120. , , , et al.
- Innovative approaches to increase deep vein thrombosis prophylaxis rate resulting in a decrease in hospital‐acquired deep vein thrombosis at a tertiary‐care teaching hospital. J Hosp Med. 2008;3(2):148–155. , , .
- Lessons from the Johns Hopkins Multi‐Disciplinary Venous Thromboembolism (VTE) Prevention Collaborative. BMJ. 2012;344:e3935. , , , et al.
- Improved prophylaxis and decreased rates of preventable harm with the use of a mandatory computerized clinical decision support tool for prophylaxis for venous thromboembolism in trauma. Arch Surg. 2012;147(10):901–907. , , , et al.
- Designing and implementing effective venous thromboembolism prevention protocols: lessons from collaborative efforts. J Thromb Thrombolysis. 2010;29(2):159–166. , .
- Impact of a venous thromboembolism prophylaxis "smart order set": improved compliance, fewer events. Am J Hematol. 2013;88(7):545–549. , , , et al.
- Improving adherence to venous thromoembolism prophylaxis using multiple interventions. BMJ. 2012;344:e3935. , .
- Health Resources and Services Administration of the U.S. Department of Health and Human Services. Managing data for performance improvement. Available at: http://www.hrsa.gov/quality/toolbox/methodology/performanceimprovement/part2.html. Accessed December 18, 2014.
- Improving patient safety by taking systems seriously. JAMA. 2008;299(4):445–447. , .
- Growth in the care of older patients by hospitalists in the United States. N Engl J Med. 2009;360(11):1102–1112. , , , .
- Prevention of venous thromboembolism: American College of Chest Physicians evidence‐based clinical practice guidelines (8th edition). Chest. 2008;133(6 suppl):381S–453S. , , , et al.
- Robust locally weighted regression and smoothing scatterplots. J Am Stat Assoc. 1979;74(368):829–836. .
- Locally weighted regression: An approach to regression analysis by local fitting. J Am Stat Assoc. 1988;83(403):596–610. , .
- Regression Methods in Biostatistics: Linear, Logistic, Survival, and Repeated Measures Models. 2nd ed. New York, NY: Springer; 2012. , , , .
- Regression Modeling Strategies: With Applications to Linear Models, Logistic Regression, and Survival Analysis. New York, NY: Springer‐Verlag; 2001. .
- Med Care. doi: 10.1097/MLR.0000000000000251. , , , et al. Eliminating healthcare disparities via mandatory clinical decision support: the venous thromboembolism (VTE) example [published online ahead of print November 4, 2014].
- Joint Commission. Improving America's hospitals: the Joint Commission's annual report on quality and safety. 2012. Available at: http://www.jointcommission.org/assets/1/18/TJC_Annual_Report_2012.pdf. Accessed September 8, 2013.
- Hospital performance for pharmacologic venous thromboembolism prophylaxis and rate of venous thromboembolism: a cohort study. JAMA Intern Med. 2014;174(10):1577–1584. , , , et al.
- Incidence of hospital‐acquired venous thromboembolic codes in medical patients hospitalized in academic medical centers. J Hosp Med. 2014;9(4):221–225. , , , et al.
- No association between hospital‐reported perioperative venous thromboembolism prophylaxis and outcome rates in publicly reported data. JAMA Surg. 2014;149(4):400–401. , , , , .
- Linking processes and outcomes: a key strategy to prevent and report harm from venous thromboembolism in surgical patients. JAMA Surg. 2013;148(3):299–300. , , , , .
- Patterns of non‐administration of ordered doses of venous thromboembolism prophylaxis: implications for novel intervention strategies. PLoS One. 2013;8(6):e66311. , , , et al.
- Nonadministration of thromboprophylaxis in hospitalized patients with HIV: a missed opportunity for prevention? J Hosp Med. 2014;9(4):215–220. , , , et al.
- Evaluation of surveillance bias and the validity of the venous thromboembolism quality measure. JAMA. 2013;310(14):1482–1489. , , , et al.
- Surveillance bias in outcomes reporting. JAMA. 2011;305(23):2462–2463. , .
- Pay for performance in health care: an international overview of initiatives. Med Care Res Rev. 2012;69(3):251–276. .
© 2014 Society of Hospital Medicine